Jeff Dean: AI isn't as smart as you think -- but it could be | TED
Summary
TLDR私はGoogleのAIリサーチとヘルスを担当しているジェフです。過去20年で、AIが視覚や言語理解などで大きな進歩を遂げましたが、まだ多くの課題があります。神経ネットワークと計算能力の向上が鍵でしたが、現在のモデルはタスクごとに独立しており、学習方法も非効率です。これを改善するために、複数タスク対応のモデルや異なるデータモダリティの統合、スパースなモデルが必要です。さらに、公平性やプライバシーを考慮した責任あるAI開発が重要です。これにより、医療や教育、環境問題の解決に貢献できると信じています。
Takeaways
- 🌟 GoogleのAI研究と健康部門をリードしているジェフ・ディーンは、20年以上前に小さなオフィスで始まり、コンピューティングの変革を多くの目にしてきた。
- 🤖 AIは過去10年間で、画像認識、言語理解、音声認識などの分野で飛躍的な進歩を遂げた。
- 🔮 AIの進歩は、洪水予測、言語翻訳、病気の予測と診断など、人々の生活に変革をもたらしている。
- 🧠 ニューラルネットワークは、過去15年で多くの問題解決のための突破的なアプローチとして現れ、実際には1960年代から考え出されているアイデアである。
- 💪 ニューラルネットワークを効果的に動作させるには、膨大なコンピューティングパワーが必要で、過去15年間でそのパワーが実現された。
- 🔧 AIの学習プロセスは、重み値の微調整を繰り返し行い、システム全体を望ましい行動に導くことで複雑なタスクをこなすことができる。
- 🌐 Googleは、YouTubeのビデオからランダムに選択したフレームを使って非常に大きなニューラルネットワークをトレーニングし、物体認識の能力を開発させた。
- 🛠️ Googleは、ニューラルネットワークの計算に特化したハードウェアであるTensor Processing Unit (TPU)を開発し、検索や翻訳などに使用している。
- 🚧 AI分野では、現在のモデルが1つのタスクに特化し、多タスクモデルのトレーニングが不足していると指摘された。
- 🌈 今後のAIモデルは、複数のモダリティ(画像、テキスト、音声など)を扱い、それらを融合してより包括的な世界理解を持つことを目指している。
- 🏛️ 倫理的なAIの重要性が強調され、AIの開発と使用には、公平性、解釈可能性、プライバシー、セキュリティなどの原則が適用されるべきである。
- 🌍 AIの進歩は、医療、教育、環境問題など、人類が直面する最大の課題に取り組む力となる可能性がある。
Q & A
ジェフはどの企業でどのような役職を務めていますか?
-ジェフはGoogleでAI研究とヘルスのリーダーを務めています。
ジェフがGoogleに入社してからどのくらいの年月が経ちましたか?
-ジェフは20年以上前にGoogleに入社しました。
AIが過去10年間でどのような進歩を遂げていますか?
-過去10年間で、AIはコンピュータビジョン、言語理解、音声理解などの分野で大きな進歩を遂げています。
AIの進歩がもたらした具体的な応用例には何がありますか?
-AIの進歩は洪水の予測、多言語翻訳、病気の予測と診断などに応用されています。
最近のAIシステムの進歩の2つの重要な要素は何ですか?
-最近のAIシステムの進歩の2つの重要な要素は神経ネットワークとコンピューテーションパワーです。
神経ネットワークとはどのような概念ですか?
-神経ネットワークは、実際の神経系のプロパティを緩やかに模倣する、相互接続された人工神経のシリーズです。
ジェフがなぜ神経ネットワークに興味を持ち始めましたか?
-ジェフは1990年代に大学で神経ネットワークの授業を受け、その時点では小さな問題では印象的な結果を示していたものの、実際の大きな問題には拡張できませんでしたが、コンピュートパワーが増加すれば神経ネットワークの可能性が広がると感じ始めました。
GoogleのTPUとは何を意味していますか?
-TPUはTensor Processing Unitの略で、神経ネットワークの計算に特化したハードウェアです。
ジェフがAIの現在の取り組み方で何が間違っていると思いますか?
-ジェフは現在多くのAIモデルが1つのタスクに特化しており、多タスクに対応するモデルのトレーニングが不足していると指摘しています。また、データのモダリティやモデルの密度についても改善の余地があると言っています。
AIの将来のビジョンとしてジェフはどのようなシステムを提案していますか?
-ジェフは多タスクに対応し、すべてのデータモダリティを扱い、効率的なスパースなモデルを用いるAIシステムを提案しています。
GoogleのAI原則とはどのようなものですか?
-GoogleのAI原則は、AI技術を開発する際に公平性、解釈可能性、プライバシー、セキュリティを考慮する原則であり、これらの原則は常に更新されています。
ジェフがAIが直面している最大の課題としているものは何ですか?
-ジェフはAIが既知のタスクから新しいタスクへの一般化をどのように効率的に行うかが最大の課題だとしています。
AIの進歩がもたらす可能性についてジェフは何を期待していますか?
-ジェフはAIが新しいタスクに非常に少数の例で学習できるシステムを構築し、より多くの問題を解決できると期待しています。
AIの適用においてジェフはどのようなリスクや問題に注意を払うべきだと述べていますか?
-ジェフはAIの適用において、その強力なシステムが善や悪のどちらに用いられるかに注意を払い、AIの原則に基づいた慎重で深思熟慮された適用が重要だと述べています。
GoogleのAI研究グループはどのようにして研究の独立性を保っていますか?
-GoogleのAI研究グループは広告グループ、検索グループ、マップグループなどと協力しながらも、基本的な研究を行っており、その多くは公開されています。
Outlines
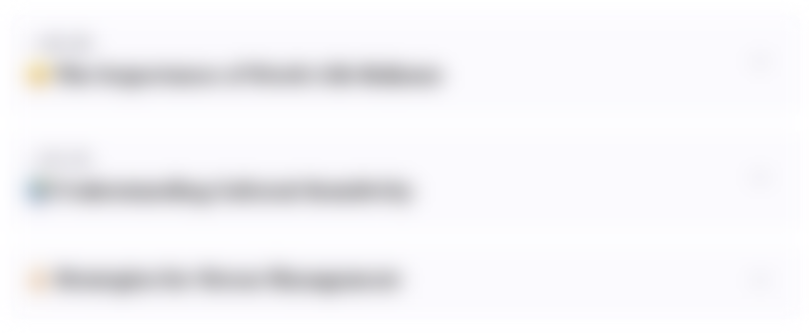
This section is available to paid users only. Please upgrade to access this part.
Upgrade NowMindmap
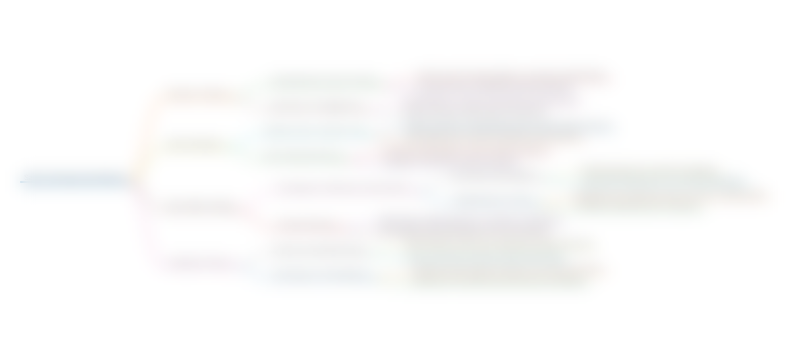
This section is available to paid users only. Please upgrade to access this part.
Upgrade NowKeywords
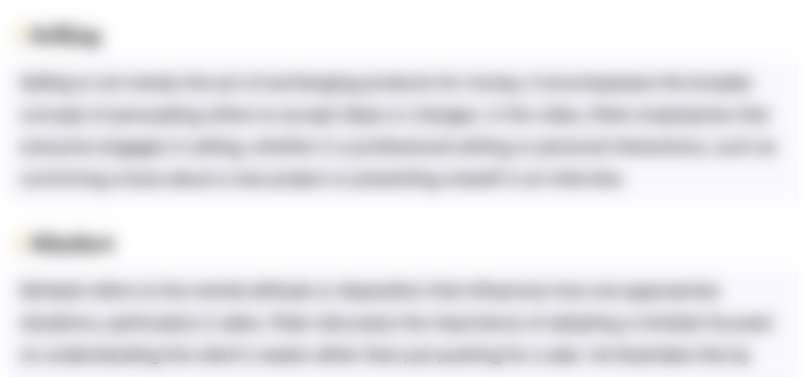
This section is available to paid users only. Please upgrade to access this part.
Upgrade NowHighlights
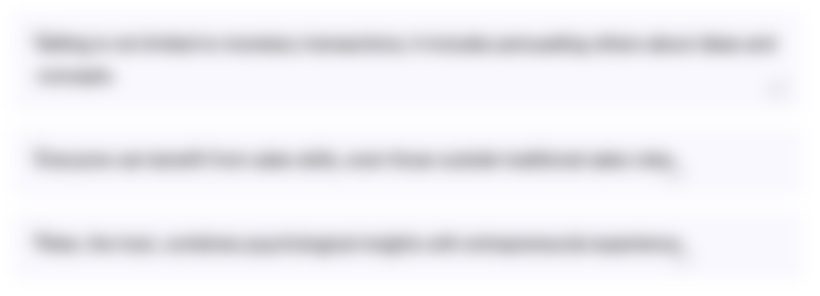
This section is available to paid users only. Please upgrade to access this part.
Upgrade NowTranscripts
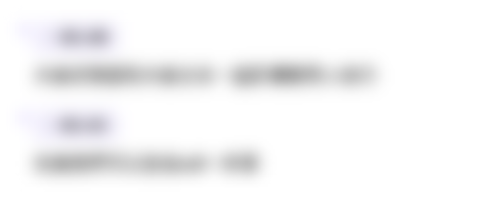
This section is available to paid users only. Please upgrade to access this part.
Upgrade NowBrowse More Related Video
5.0 / 5 (0 votes)