How to Become an AI Product Manager - AI PM Community Session #42
Summary
TLDRThe video script discusses the high demand for AI talent and the importance of AI product management (AIPM) skills. Vian, founder of PM exercises, introduces their AIPM learning program designed to equip product managers with AI expertise. The program includes ongoing education, community access, and practical tools. Guest speaker Kai, from Uber's AI platform team, shares insights on AI's practical applications and the significance of product management in translating AI visions into reality. The presentation highlights the need for a deep understanding of AI technologies and the opportunity to network with influential figures in the AI space.
Takeaways
- π The demand for AI talent is high, with many enterprise clients struggling to fund it, indicating the growing importance of AI in the industry.
- π Vian, the founder of PM exercises, emphasizes the mission to help people become better product managers, especially in the realm of AI product management.
- π The company initially focused on interview preparation but has expanded to ongoing education, recognizing the rapid changes in AI and the need for continuous learning.
- π Success stories from PM exercises' platform highlight the effectiveness of their approach in helping individuals prepare for AI product manager roles.
- π Ongoing access to learning materials for three years is offered to ensure that skills remain up-to-date with the evolving AI landscape.
- π οΈ PM exercises is focused on creating the best AI product manager learning program, being the first to establish a dedicated cohort for this complex field.
- π€ The program provides access to a community, ongoing learning, and an AI assistant to increase productivity, as well as opportunities to connect with influential figures in AI.
- π± The transition from 'AI product managers' to just 'product managers' is anticipated, as understanding AI is expected to become a core competency for all product managers.
- πΌ The importance of not just getting hired but also excelling in the role is underscored, with the need for a deep technical understanding to manage AI products effectively.
- π The script discusses the process of identifying and creating job opportunities, with an emphasis on self-sourcing and the power of hackathons to build networks and skills.
- π The content of the AI product management cohort is outlined, detailing the skills required and the structure of the program to develop a comprehensive understanding of AI in product management.
Q & A
What is the primary focus of PM exercises?
-The primary focus of PM exercises is to help people become better product managers, with a special emphasis on AI product management as the next big thing for the PM community.
What is the significance of the statement 'today we call them AI product managers, but tomorrow we're just going to call them product managers'?
-This statement signifies the belief that understanding AI will become a fundamental expectation for all product managers in the future, making the 'AI' prefix unnecessary.
How does PM exercises measure success in helping users prepare for interviews?
-PM exercises measures success by tracking the success rate in landing jobs among people who have used their platform to prepare for interviews.
What is the importance of ongoing education in the AI product management field according to the transcript?
-Ongoing education is crucial because the AI space is rapidly changing, and taking a course or cohort now might not be sufficient to keep one's skill set updated a year later.
What does PM exercises provide to users for ongoing learning?
-PM exercises provides access to everything developed on an ongoing basis for a three-year period, ensuring that users' knowledge and skills in AI product management stay updated.
Who is Kai and what is his role in the context of the transcript?
-Kai is the lead product manager for the AI platform team at Uber, managing Uber's internal machine learning platform called Michelangelo. He is involved in leading the AI product management cohort.
What is the role of hackathons in creating opportunities for AI product managers?
-Hackathons are important for creating opportunities as they allow individuals to meet others interested in sharing knowledge, build products, and demonstrate thought leadership, making candidates more attractive for AI product management roles.
Why is it necessary to have a deep technical understanding as an AI product manager?
-A deep technical understanding is necessary to apply product management skills effectively, make informed trade-offs, and turn visions or ideas into real AI products that solve real-world problems.
What are some of the hidden opportunities in the AI industry for product managers?
-Hidden opportunities include positions that are not advertised and may only become available when a company identifies a perfect candidate for a problem they were not clear how to address.
How can an AI product manager candidate make themselves attractive to potential employers?
-Candidates can make themselves attractive by gaining relevant experience, participating in hackathons, publishing thought leadership content, and demonstrating passion and willingness to learn and adapt in the AI space.
What is the importance of the interview evaluation process in securing a job as an AI product manager?
-The interview evaluation process is crucial as it is where candidates often fall short. It is not enough to just get an introduction or referral; candidates must pass a rigorous interview process to demonstrate their AI product management skills and knowledge.
Outlines
π€ Introduction to AI Product Management and PM Exercises
The speaker, Ban, introduces himself as the founder of PM Exercises, a company focused on helping individuals become better product managers. He discusses the company's mission, which is not centered on profitability but on educational value. Ban highlights the increasing importance of AI in product management and the demand for AI talent. He mentions the success of their platform in helping people prepare for interviews and the ongoing education they provide, which is crucial given the rapidly changing AI landscape. The speaker also introduces Kai, who will be leading the AI product management cohort, and shares his excitement about networking with influential people in the AI space.
π The Evolution of AI and the Role of Product Managers
Kai, the lead product manager for Uber's AI platform, discusses the evolution of AI from a data science and engineering task to a field with practical applications that can change the world. He emphasizes the importance of product managers in turning AI visions into reality, especially with the abundance of capital and resources available. Kai also talks about the challenges of delivering on promises made by companies in the AI space and the necessity for product managers to have a deep technical understanding to succeed.
πΌ Navigating the AI Job Market and the Importance of Self-Sourcing
The speaker discusses the strategies for landing a job in the AI field, noting that traditional methods such as submitting resumes or getting referrals are not enough. Instead, he suggests becoming an interesting candidate by self-sourcing opportunities, leveraging referrals, and showcasing a strong track record. The speaker shares personal anecdotes of how he created opportunities for himself through outreach and emphasizes the importance of being proactive and taking risks to stand out in the job market.
π Building Expertise and Crafting a Compelling AI Narrative
The speaker advises on how to build expertise in AI and become an attractive candidate for AI product management roles. He suggests participating in hackathons, attending events, and publishing content to demonstrate thought leadership. The speaker also highlights the importance of being able to articulate a strong story during interviews, which can be achieved by building products, practicing skills, and engaging in intellectual conversations about technology.
π The AI Product Manager Learning Program and Community Support
The speaker outlines the AI product manager learning program offered by PM Exercises, which includes access to learning materials, experts, and a community of AI product managers. He discusses the importance of ongoing learning in a rapidly evolving field and the benefits of being part of a community that provides support, feedback, and job opportunities. The speaker also mentions the hands-on nature of the program, which includes team-based workshops and product building.
π Deep Dive into AI Product Management Curriculum and Expectations
Kai provides an overview of the curriculum for the AI product management cohort, which covers AI and ML technical foundations, problem definition, data strategy, MOps, and responsible AI. He explains that the program is designed to help participants develop the necessary skills to become successful AI product managers, including understanding AI models, managing the model lifecycle, and ensuring responsible AI practices. The curriculum is structured to build a comprehensive PRD and includes live workshops and practical applications.
π Personal Support and Community Engagement in the Cohort Program
The speaker discusses the personal support available to participants in the cohort program, including access to the instructors' calendars for one-on-one advice. He emphasizes the importance of community engagement through a forum where questions can be posted and answered. The speaker also mentions the availability of learning materials for three years, ensuring that participants stay updated with the latest developments in the AI field.
π Overview of the Cohort Structure and Application Process
The speaker provides details about the structure of the cohort, including the number of weeks, the content covered each week, and the bonus materials provided. He explains that the program is designed to build an end-to-end PRD and that participants will have the opportunity to present their product ideas. The speaker also shares the application process, the start date of the next cohort, and encourages interested individuals to apply.
π Final Thoughts and Closing Remarks
In the closing remarks, the speaker thanks the participants for attending the session and expresses excitement for the next cohort. He invites questions and offers to share the presentation and learning materials afterward. The speaker also provides his contact information for further inquiries related to the cohort and encourages participants to reach out for personal support.
Mindmap
Keywords
π‘AI Talent
π‘Product Manager (PM)
π‘AI Product Managers
π‘Ongoing Education
π‘AI Platform
π‘MLOps
π‘Hackathons
π‘
π‘Evaluation Process
π‘Technical Understanding
π‘Community
π‘PRD (Product Requirements Document)
Highlights
High demand for AI talent is causing many enterprise clients to struggle with funding.
PM Exercises, founded by Ban, aims to help people become better product managers with a focus on AI.
AI Product Managers are expected to have a deep understanding of AI, which will become a standard for all product managers.
PM Exercises provides ongoing education for AI Product Managers and has seen success in job placements.
Access to the PM Exercises platform includes a community, ongoing learning, and an AI assistant for three years.
Ban emphasizes the importance of having the best AI product manager learning program.
Kai, the lead product manager for Uber's AI platform, shares his experience and passion for growing the AI PM community.
The AI industry has shifted from theoretical discussions to practical applications, impacting various fields.
Product managers play a crucial role in turning AI visions into real products.
Hidden PM opportunities in the AI industry are often not advertised and require a proactive approach.
Referrals and self-sourcing are effective methods for uncovering unadvertised job opportunities.
Hackathons are a powerful way for aspiring AI PMs to gain experience and network.
Passing the evaluation process is key to landing a job, not just getting referrals or submitting resumes.
AI PMs need to demonstrate both traditional PM skills and AI-specific knowledge during interviews.
Building a strong story and showcasing AI expertise can help candidates stand out in interviews.
The PM Exercises AI PM learning program includes hands-on workshops and team-based product building.
Cohort participants gain access to a community of AI PMs and opportunities for personal support.
The program covers AI technical foundations, ML problem definition, data strategy, MOps, and responsible AI.
By the end of the cohort, participants aim to have a complete PRD ready for an engineering team.
The cohort includes optional content and recordings for deeper learning, requiring a time commitment of 5-15 hours.
The next cohort starts in May, with a structure that includes foundational knowledge and bonus materials.
Transcripts
[Music]
% of their Enterprise clients are
struggling with funding AI talent and
that really speaks to the high demand
that's out there so um first of all I'll
introduce myself I'm I'm ban founder of
PM exercises I we've been in business
for about six years now and uh since the
beginning it was a kind of a mission
driven uh company that is not really
optimizing for profitability or anything
like that it's optimizing for uh helping
people become better product managers it
really started from my own uh set of
experiences that I had in the industry
um trying to prepare for interviews so
that was what we were focused on for the
first few years and uh with AI we
decided this is kind of the next big
thing uh for the PM Community um I think
that we're going to enter a world where
um just like communication skills having
a deep understanding of how AI works is
going to be one of the expectations for
any product manager in any job and uh
today we call them AI product managers
but tomorrow we're just going to call
them product managers and we have some
good stats on the success in bending
jobs among people that have used our
platform to prepare for their interviews
um we we provide ongoing education this
really applies to a couple different
things not only that um we have a lot of
users who go through our platform use
our platform to prepare for interviews
but they also use it on an ongoing basis
to become better PMS uh but we actually
do do this also on the AI product
management side in fact you get access
to everything uh for a few years
afterwards um going through the cohort
and the reason for it is we understand
um there's a lot that's happening in the
space things are changing a lot and um
you taking a course or cohort now um
might not be sufficient uh for your
skill sets to be updated like a year
later right so anything that we share
and develop on an ongoing basis will be
available to you for threeyear period uh
and right now PM exercis is really
focused on um having the best AI product
manager learning program out there um I
can say with confident we were the first
ones in the world um that decided this
is so complex that needs its own cohort
and um we've had five successful
learning programs so far but now we're
expanding it um when you're part of the
program you get things like you know
access to a community ongoing learning
you get access to an AI assistant that
helps you become more productive uh but
uh what I'm also very excited about is
um the opportunity for me to get to meet
um other people other very influential
people in the AI space uh for example
Kai is one of them um I've been very
lucky and honored to get to know him um
I want to actually pass it to him uh do
his own intro and then I I'll come back
and continue so go ahead Kai sure thanks
Vian he folks uh my name is Kai I'm
currently the lead product manager for
the AI platform team here Uber I manage
the Uber's internal N2 machine learning
platform called mangel that offs you
know 100% of Uber's machine use cases
these are these are the use case such as
you know rider rider pricing uh driver
incentives promotions rer driver Mion is
delivery uh ETA uh map CTA and also
things like our East home feed
recommendations all the way to customer
services and also fraud detection so it
covers both the traditional machine
learning and also the recent Genera a
use cases at Uber um I will be leading
this cohort uh aipm cohort uh and uh
joined by a x aipm from Google Corey uh
I don't think Corey is here today right
no yeah it's not on the call
okay um why am I here I am super
passionate about the AI ml space and I
do want to help grow the AI mlpm Comm
Community because I think we're still at
a very early stage for uh for this for
this field and uh I just love sharing I
want to share my past experience some of
My Success a lot of my failures to help
you jumpstart your aipm career so
welcome I mean nice to meet all of you
guys back to you all right thank you so
much so um let's talk a little bit about
um what we're going to um go over and I
think this is very interesting uh slide
that um I think it kind of captures
what's really happening in the industry
especially in the world of AI um for a
long time um this was more of a um data
science or um engineering task um this
was like driven by engineering and data
scientists they were doing a lot of
research uh trying to kind of figure out
um you know how do you actually have um
some sort of a Technology stock that
delivers um at least artificial
intelligence in some like basic forms
shapes and form and one could argue that
um you know rule based systems that we
have been working with over the past 20
years maybe there were like kind of the
initial versions of it but um AI in the
form that we understand today uh was not
really available to us um until very
recently and about four or five years
ago things started shifting um there was
kind of more of
focus on talking about Ai and really
talking about the potential because
people could start imagining how Ai and
ml is going to change the world but um
given the technology wasn't there
initially this Focus was mostly on the
marketing side so um a lot of companies
got funded um lot of Visions were
communicated people could start
imagining how things are going to work
but a lot of them started u kind of not
delivering on their promises um until
about um a couple couple years ago when
um the world was kind of shocked at how
Ai and ml can actually start having
practical applications and this is when
I'm talking about the world I'm talking
about kind of people outside um the AI
ml community and um all of a sudden you
had a product um that basically
showcased how um Ai and ml could
actually solve real world problems
whether it's education healthare um
image detection and all these other
things and um with the rise of geni and
chat gbt a lot of companies actually
started getting also funded because they
could start imagining even more use
cases and um in a world where um there's
kind of abundance of funds available
abundance of capital and um there is
like an abundance of uh engineering
resources and marketing resources uh
what becomes important is um delivering
on those promises and like really
turning those Visions into reality and
uh who does that better than anybody
else is product managers right like you
need to kind of apply product management
skills to uh turn a vision or an idea
into a real product um do the tradeoffs
and in order for you to do that you have
to have really deep technical
understandings of how that part works
and um that's what we're all about at PM
exercises really kind of building that
foundation so um what we're going to
talk about today to kind of see how that
um you know could translate into an
opportunity for us at a very high level
is uh what are some of the Hidden PM
opportunities from an artificial
intelligent industry perspective um I'll
talk a little bit about um you know why
I'm talking about hidden and like what I
mean by that um the other part is um if
you come across as an opportunity um is
that sufficient right like you know if
you get intros introduced to another
company or um you know you send your res
uh is that sufficient um reality is
that's not sufficient because um there
are many other
uh competitors uh who are also aiming
for the same gole so um not only that um
you have to kind of make yourself look
attractive to them uh so that they're
willing to kind of pick up the phone or
like send send you an email to have a
conversation you have to be able to also
pass the interview and the evaluation
process right uh and we'll talk a little
bit about um the AI product management
learning um later um during this session
so this is what we're going to cover
cover at a very high level and um what's
interesting is that uh typically um we
think of like getting hired funnel as
you send your resume or you get a
referral and you get hired right um but
the reality is that's not the case this
is not how things are done even though
uh we continue thinking um maybe in our
subconscious level that this is how um
you can land a job this is not the
reality you just getting submitting your
resume um or you know getting a referral
um does not mean that um you've had
you're going to land the job and um the
best way for me to kind of um you know I
think make you kind of realize this as a
fact is to like think back about the
last time you were actually able to land
a job this way right or or do you know
anybody um who's ever landed a job this
way and probably the answer is no right
um just because you get a referral or
you get a resume um sent you're not
going to land your job you're not going
to get hired um the right way to do it
is somehow you become an interesting
candidate date um that comes under their
radar and there's so many ways for us to
do this resume is only one of them right
um but more important is for you to pass
the evaluation step right um and guess
what any company that's good um that's
really trying to build a meaningful team
in the space of AI and ml they're going
to make sure that they're doing a pretty
good job in hiring people um on the AI
ml side and what it means is that um
they're going to make they're going to
be very careful with their hiring
process they're going to be very
selective they're going to make you go
through a rigorous um hire interview
process um and um that's usually where
we fall short this is where we usually
fail so the only way for you to really
land the job is for you to pass that
evaluation process and um we're going to
talk a little bit about U what that
means over the next uh few slides I
think this is going to be pretty helpful
for um the existing uh cohort members on
the alumni and also like people that are
uh currently um evaluating um whether or
not they want to be part of the next
learning program on AI product
management
so the first thing that we're gonna kind
of ask ourselves is okay um how do I
come across as an opportunity right um
for me to actually even be an
interesting candidate um in the eyes of
um the potential hiring manager right
and the reality is that um there are a
lot of positions out there right that
you can apply for um but the real
opportunities are not the ones that are
advertised um they're the ones that
nobody knows about maybe the company
hasn't even decided um that they're
going to hire an individual and um they
would only like get that uh position
available once they see that oh there's
somebody who if they come on board um
they would be perfect for this problem
that they were dealing with and they
were not clear how to address right and
um there are a couple different ways to
kind of go about um coming across this
opportunity I think one of those
opportunities is um getting referrals
yes that's very important this doesn't
mean that you're going to actually land
a job that's very helpful um I've
basically received lot of offers from um
friends or um other um connections to my
previous um employers staff because
somebody said oh ban came in did this
like you should talk to them right
that's a great way way and that usually
means that you get that third party
approval that makes things easier but
this referral model works really well
when you have a strong track record
right you have a strong track record
then it makes sense because they're like
oh we want you because we heard you've
done a great job um in this particular
area in this fi right and the other part
is self Source right and when I say self
Source I'll kind of give you an example
on how you can self it self Source these
opportunities kind of create these
opportunities yourself and um once you
create those opportunities uh you still
have to basically make sure that you
pass the evaluation phase right uh so I
got like a couple examples of like you
know how um these opportunities could
exist in the real world uh this is like
a kind of a real example of my situation
right like I sent out an email to
somebody and I'm like hey I'm very
interested in um you know working with
your company and um I would like to
learn more about you and guess what they
replied back and
um we ended up actually talking and um I
ended up doing a Consulting gig for them
for a while I was very interested in
their technology and um here's another
example um this turned into a full-time
opportunity um I reached out basically a
company that I was very interested in uh
I told them about I heard about you guys
um I would like to know if there's a
chance for me to be part of your team I
just heard you guys raised money uh and
they didn't even have an opening right
and the CEO emailed back and said yes
I'm very interested in being part of
your team um so um I'm very interested
in you joining our team they invited me
I flew down to San Francisco and next
thing you know I join them as a
full-time staff so I think this is kind
of an example of how I create
opportunities for myself it's something
that definitely works and um the way I
do it the way I come across them is I
know what I'm interested in um maybe I
use like Google alert to be notified
about every single uh content that's
generated in my area of Interest I spend
a lot of time on like you know link
or um X or any other platform or like
popular Tech related sites to be
informed of any developments in that
space and if I see something I just
reach out to them right and you know
I've also not listed a lot of examples
of situations where I didn't get a
response right so um it's very important
for us to kind of realize it's not
guaranteed to be a success but it just
shows that this method works and you
have to kind of be willing to uh put
yourself out there um and reach out so
that they start evaluating you and I
want to emphasize and say this doesn't
mean that you're going to get the job it
just means that now you've soled the
very first step which is okay this is an
interesting candidate and we're willing
to evaluate that right so I kind of like
you know a couple things to highlight um
how does cold approaches work first of
all um you don't have to have um a job
experience in that particular area you
need to have an experience right um and
it's very important for us to realize
this ESP especially in emerging um
Industries like artificial intelligence
because um a lot of you who are thinking
about breaking into AI you're probably
thinking in your head I don't have a
full-time AI product manager experience
but that's okay um you can actually
create that um by becoming experts
spending time to read on things that you
think are important um generating
content that represents you have some
sort of a thought leadership and your
experience and knowledge has reached a
point where um you can actually have
your own independent opinion and you
have other ways for you
to gain that experience and one thing
that I'll highlight throughout this
presentation is hackathons like the
power of hackathons you going into
hackathons where you're going to meet a
bunch of other people who are actually
very interested in sharing their
knowledge with you like how great is
that like they're actually passionate
about finding somebody who's willing to
listen to them U and is willing to learn
from them and they're very happy to do
that and what this will do is um over
time it'll make you more attractive as a
candidate um it'll help you kind of
develop a deeper understanding of what
different companies do um particularly
the company that you're aiming for right
um and this will allow you also be more
selective on the type of companies that
you want to go after right it also shows
that you're passionate right it shows
that uh you actually are interested in
this not just because of the salary job
you're not going to be a 9 to5 type of a
person you're going to be somebody U
who's actually very interested even once
you land your job you're going to
continue um developing your skill set
and your knowledge maybe outside the the
working hours and that you know that's
exactly what an employer wants right um
and you also want to kind of show that
you're willing to make sacrifices when
you're entering a new space um in an
area where um you don't have necessarily
a lot of full-time experience and what I
mean by sacrificing is for example
sometimes it means making a sacrifice on
a title sometimes it means making a
sacrifice on your salary because let's
face it they're taking a risk on you and
um if you really want to kind of
maximize on your compensation uh you're
better off working in an area where you
already have a lot of expertise and
you're kind of like CCH capturing all
the fruits that are um already grown
right so whereas here you're kind of
entering into a new space um given that
they're taking a risk on you um there's
going to be a discounted price you can
think of think of it
um on your services and you have to be
realistic and accept that um in the hope
that in the long run right you will
become an attractive candidate to more
companies and eventually you become an
expert in the field that everybody wants
so that's something for you to kind of
keep in mind like you know when does
Whole approaches work well um before I
send those emails I already went to a
lot of hackathons I already attended a
lot of events I published a um my own
paper on a particular topic that I was
in interested in I could kind of refer
to it uh it made them see that I'm a
thought leader um and I I told them I
just want to be part of your team I
don't care what I'm going to be doing
right uh and that was very important
so now the question is going to become
you know how do you pass the um
evaluation process right uh and the
reality is um on a Surface um it sounds
like they basically want you to have all
these um great
attributes um that sometimes are kind of
conflicting with each other right for
example oh you're it's a new space like
how can you expect um all your hires to
have a lot of um you know experience in
this field right until three years ago
um maybe the number of people that were
working as AI product managers were
three digits so that's a reality and
there you know many other kind of
examples like this and that you know we
as PMS would probably experience but
this doesn't mean that um there's no um
there's no opportunity so there's a
couple ways for you to kind of become
that attractive candidate um one like I
mentioned um really becoming an
aipm um before um you land a job right
and the way you do that is by practicing
those set of skill sets wherever you get
a chance right um through um community
events or like hackathons um and
building products like there's nothing
more important than actually building
something that you can present and you
can kind of talk about because when you
actually build a product um that's when
you start realizing some of the
limitations of the technology so you can
have an intelligent conversation um it's
somebody actually mentioned to me once
that people that do well in interviews
um they think of a job interview as um
an intellectual conversation between two
smart people and I thought it was very
interesting U especially when you're
aiming for a company that's very
attractive um to a large number of
intelligent people that's good way of
thinking about it and the best way for
you to handle that conversation uh is
for you to be able to talk intelligently
about uh kind of the nuances of the tech
stack and the best way for you to learn
that is through building things right
like building a PRD um and if you're if
it's possible especially with no code
tools or um attending like a hackathon
even turning that into some sort of a
prototype um that allows you to um kind
of see those like limitations in the
real world uh and then writing about it
there's nothing like um writing when it
comes to learning it's very hard uh it's
very hard to be authentic and um good
news is that in the world of a ml um
there's so many areas um that are kind
of just like kind of scratching the
surface right now you can kind of uh go
really uh deep in one particular area U
we had somebody that actually went
really deep on one particular metric for
one particular company and he published
a um interesting blog post about it and
after the blog post was published uh he
started receiving a lot of contacts a
lot of interest from people on LinkedIn
like recruiter saying oh we really like
this blog post that you published we'
like to talk to you uh but it needs to
be genuinely um written by you and
should represent that you actually have
your independent thoughts and um once
you kind of reach that level um then
you're actually able to um see yourself
um available for many opportunities and
this is going to be a marathon more than
a Sprint that's the reality but it is
possible so then how do you actually Ace
the interview um I think there's a
couple things that it's really important
for us to realize is um you know there's
like the kind of the traditional PM
skills that are very relevant like you
know knowing how to do product sense and
how to do uh strategy questions and
behavioral you have to continue U being
able to pass those kinds of questions
and PM exercises has a lot of good
examples but you also need to have a
very AI specific knowledge right um and
this really comes down to you
understanding uh what's important for an
AI product manager right for example how
does an AI product manager get involved
on the data strategy side or how much
does an AI product manager get involved
on mlops uh because you're G to have to
be able to have uh a meaningful
conversation with some of your future
colleagues and some of those future
colleagues are you know the data
scientists and ml Engineers right um
here's the good news you can actually um
simulate those conversations before the
interviews and you can do that like I
said whether through like a physical
event like hackathon or through your um
you know personal contacts uh attending
online communities or physical physical
communities or events uh but that's what
you need to do um in order for you to be
able to have a comfortable conversation
uh with these people because
uh people like data scientists and ml
Engineers are very likely or at least
their um their criteria for a good
hiring process is very likely to be part
of the interview process right um so
what you have to do is kind of like you
know practice for that um and make sure
that you can you can do well and you
need to have a very strong story to
share and that strong story um ideally
if it's very attractive it will actually
take most of the interview time and
you'll be able to kind of um Define the
rules of the game during the interview
instead of letting them kind of take you
in any direction that they like uh and
like kind of trying to find a gotcha
moment right um and the best way to do
that is to like I said um build
something that you find interesting
that's probably better than um investing
even in a learning program and um you
still need to kind of practice a lot um
it's interesting to me like I've seen
people that I've landed um great jobs at
top tech companies uh like for example
Google and Facebook uh many of them just
on the mock interview side they've done
over 100 mock interviews um that means
over 100 hours right of course that's
not the case with everybody um but it's
important for us to be aware of and
couple notes here for the cohort U
members on on cohort 5 um if you guys
are interested um if you're kind of
practicing for your PM aipm interviews
uh feel free to reach out um to me or
Audrey and um I'll be happy to uh we'll
be happy to match you guys with um some
of the other uh alumni of the previous
cohorts who are also practicing for
their interviews um that's one of the
benefits of the community you guys can
practice and I kind of improve help each
other grow and um get better in your
interviews and the other thing that
we'll be doing is that um we will be
also U providing you with um a um the
the cohort five people um with an aipm
interview guide um sometime next week
this is um almost ready we're very
excited about sharing it with you and we
will also share this with some of the um
Al with the alumni of the previous
cohorts as well so hopefully that'll
help you guys U become more prepared for
the um interviews to come so um I I'll
kind of quickly talk about um what's
going on on the um AI product management
site at PM exercises we think that we
try to um bring everything that I talked
about together um during the cohort um
some people have been going through it
right now um so I want to talk about
this slide quickly and then I'm going to
pass it to Kai to kind of dig more into
it uh but like one thing that I'll also
mention is that um you will get access
to all the learning material for a
period of three years this is very
important I think especially given the
industry is evolving and you get access
to experts and um there are a lot of
experts right there is like um the
instructors in the learning program um
there will be um guest speaker opportun
unities that will also involve invite
people from the previous cohorts too so
you'll also be able to kind of attend
those uh and then there will be like a
good opportunity for you to kind of
learn about um some of the emerging
applications in Ai and um you know what
sort of PM roles are evolving um in each
of those areas because you want to kind
of play in in an area where not a lot of
players are out there or like um I had a
a teacher back in school uh he used to
say you want to fish in a lake where
nobody else is fishing fishing right so
that's kind of like something for you to
Define and you know what do you define
fishing maybe it's your expertise maybe
it's the fact that the industry is not
being paid attention to but hopefully
you'll get a sense of that uh you'll get
access to the community of AI product
managers um we we meet every Saturday as
you're aware um there'll be some of job
opportunities but I want to kind of
emphasize and say um you know this is
very important for you to kind of be
aware of because we're happy to do the
intros but um if you kind of you're not
attracted to the um employer and you
know you won't pass the evaluation right
you're just going to burn those chances
so uh there's no guarantees or anything
in here but um you know if one of the
cohort members right now or alumni are
interested please reach out to us and
we're happy to um do intros and help you
out but um let's make sure that your
package is attractive um and it's almost
like a no-brainer for the other side to
hire you right um and we do a lot of
kind of like team based workshops and
product building um anybody who
graduates will have we can talk about
like a particular PRD that they worked
on um and it's got a very kind of a VC
style format towards the end where you
actually get to present your idea um to
um a panel of judges and uh they'll
evaluate you and they'll give you real
feedback I'm really impressed by um the
quality of the projects that are being
um developed right now we have about um
10 projects and um they're really
amazing projects a couple of them are
totally fundable so uh very impressed
and here's the good news because you
work in a form in a team um you'll learn
a lot uh from your colleagues um a
couple of people asked that we assigned
them like uh study partners and we've
done that actually more than a couple
like about 10 people and um you know
that's one way for you kind of get more
from the community and another way is
you're going to be part of a team um
that's going to be uh developing a PRD
that you can even turn into a product
after the graduation
we'll also give you some additional
bonuses some access to the PM excises
platform you get access to um the
product the assistant for AI for product
managers on product monkey it's a tool
that converts your uh product manage uh
any design files into detail enging Tas
and uh prds and this is a really good
tool for anybody who wants to uh
basically save time and kind of
Outsource some of their uh monkey tasks
um and these are everything here is very
Hands-On as some of the people in the
current cohort can tell we do spend some
time kind of going over your resumΓ©s uh
giving you feedback and we're pretty
honest to be honest like sometimes it
might feel uh very harsh but we we
optimize for your success so we tell you
look you need to go and like really
improve your resume this way uh for
example if your resume doesn't mention
anything about AI uh there's no hint of
it uh it's very unlikely that you going
to be successful right um in learning an
aipm job so um if now you have to have a
resume that really speaks and breath um
AI product management then that means
you have to do a different set of
activities and sometimes means a couple
months of work from you to kind of reach
a point where you can proudly present
your resume for an aipm role and uh
we're happy to provide all that
guidance and um like I said uh we will
be happy to do intros um if you're ready
and um you know at some point um I've
said to people if you're very interested
I'm happy to go ahead and do it right
now but you got to make sure that you're
ready if you want to be successful at
least on your side uh so that's a little
bit about um you know the aipm learning
program that's starting um in May uh
good news is that uh we're going to have
three different tracks like one is on
Tuesday Tuesday evenings another one is
on Thursday morning and another one is
on Saturday morning so hopefully um
anybody who's kind of considering
attending and they're worried about
their time zones and whether or not
they're not they're they're able to
attend they'll probably find one of
these sessions uh to work for them and
they can attend that and um over time
they'll kind of build their team and
they'll become more familiar uh with the
rest of the community and um this is
going to be a great great uh Community
for you and for your career going
forward uh so with that said I want to
pass it um to um Kai I'll quickly talk
about this one last slide too uh but
like who are the cohort participants
really a couple people asked that
question um earlier uh these are mostly
people that are existing product
managers I would say about 90% of or so
um of our uh cohort participants are
existing product managers that worked
for um you know anywhere between two to
three or even like 105 years as product
managers sometimes they're directors or
VPS um we have a few entrepreneurs
um in the world of um AI product
management um but one thing I can tell
you is you're not going to get um the um
kind of basic um product management 101
that's not what this cohort is all about
um this is about U much kind of further
than that much deeper than that um so
thank you so much for listening to me
I'll pass it to Kai maybe Kai can talk a
little bit about um the um the program
and we can go from there yeah thanks V
so uh let's talk about a little bit more
about the the content of this of this
cohort but before that let's actually
take a look at what the what are the you
know desired or required skill sets for
aipm uh just want to make it clear all
the uh all the skill sets for the for
traditional software product managers
are still required for being a aipm this
are you know uh user empathy you know
business sense product design technical
depth but additionally to be a
successful
aipm uh you need additional skill sets
uh first of all your AI ml technical
foundations this is besides the
traditional software development uh
technical foundations now you need to
know uh what is AI what are different
types of models how to build the models
and their applications how to manage the
model life cycle all things like that
and then the ml problem definition so
after Define your defining your business
problem how do you actually translate
that into a machine learning problem and
more importantly do you even need
machine learning to solve this problem
do you just use the traditional
traditional way or do you actually need
machine learning so that's something as
a PM you need to decide so you need to
know how to do that and third is about
data do you understand data where to
collect data because the data is a key
part of any of the machine learning
systems do you do you know how to come
up with a successful data strategy
things like what are the data sources
how to collect the data what type of
data do you need to solve this exact
Machinery problem and how do you process
data and uh all the way to how do you
visualize the data at the end right and
then uh very importantly how do you
actually manage the machine Le life
cycle from uh starting from collecting
data as we just mentioned to training a
model writing a model deploying mod
model and the monitoring model and if
the model performance de rates how do
you retrain model with a fresh data and
the risk risk medication your machine
systems will make mistake that's just
the nature of machine learning when that
happens what do you do do you have do
you have a fullback plan and also uh
risk uh management also applies to the
the project management side of of ai ai
product development and because the
product development life cycle is is
very iterative it's uh more way more
complicated compared to the traditional
software development so how do you
manage that uh project efficient AI
project efficiently that's something we
we need to talk about and
explainability um usually the
traditional software is easy to explain
because it's just followed by rules and
but AI product sometimes we treat is
treated as a blackbox but a lot of times
you need to explain the your model
behaviors why your model is making this
prediction uh especially when you apply
AI in space like uh
Financial uh you know
Financial finance um and also you know
uh when you apply this to safety or
legal to uh and law so you have to
explain your model model model
performance that's something as aipm
need to understand how to do it and
lastly responsible AI how do you detect
the bias in your system how do you make
sure it's actually fair to different uh
user cohorts
um can go to the next
St so to help you develop all these uh
skill set we just mentioned uh we
purposely designed the agenda for this
cohort we will start with the a IML um
Foundation we talk about what are data
way to collect data what are features
how to do feature engineering different
types of machine learning models and
algorithms how where to apply them how
to build them and also how do you
evaluate your perform your model
performance and your application
performance and a lot more and then in
week one we start with the AI problem uh
product problem
definition and we introduce a framework
to help you decide whether your problem
uh is um is good for need to solve or
you can just use the traditional
traditional rule based system and we'll
run a live Workshop in the live session
to apply that framework to solve some of
the uh you know the scenarios and use
cases and in week two we move on once
you have the problem defined in week two
we move on to the to the design we
introduce the key principles to design
the AI products and again we'll
introduce you a design framework for AI
products they are 15 Steps I think it's
more than 15 steps you need to follow to
Define to uh to design a AI product
properly and again we run a live
Workshop uh there's some uh scenarios
you you will see how do we apply this
design framework to solve those use
cases and after Design After product
design is done after your requirements
are already now you can pass it down for
your engineering to build a product and
that's why that's what we're going to
talk about week three the M Ops how do
you actually build and launch the uh you
know build the machine plat build a
machine product and launching into
production and also maintain this exper
uh its performance in
production and in week four we finally
talk about the once the model is ready
application is built now we talk about
AI product launch and also we introduce
a framework for to for you to apply the
responsible AI to your product make sure
it actually works as as um as expected
so you can probably tell uh through week
one and week four we are actually focus
on each part some some part of your
PRD right we start from prop definition
then move on to design requirements then
move on to Builder product and then
product launch so that's exactly what
we're doing we aim to actually build a
End to endend
PRD uh through this cohorts and that's a
PRD you can actually take to engineering
team to build a real product and at the
end of the cohort we have a bonus week
this is where this is when all the teams
got to present their product ideas just
like a product pitch so yeah very
exciting content uh hopefully I'll see
all of you there back to
you all right thank you very much so um
at a very high level um anybody who is
interested in applying please use this
URL so um I've shared this on LinkedIn
and anybody who's on Zoom I'm just going
to share it with you um in a second
after I stop sharing my screen uh but uh
next cohort starts on the second week of
May um and uh please feel free to submit
your applications we're reviewing them
we've already got a lot of people who
have signed up for the next cohort so
very excited um that uh we're going to
be a part of this uh program with you
guys um so yes I'm going to share the
link in a second uh chatan I can see
your question uh your comment uh but uh
let me kind of take a second and then
see if uh people have any questions I we
would be happy to um answer them here's
the link um I'm not sure
why give me one second let just like
quickly share that I'm not sure why it's
kind of sharing it the way you're seeing
it um the ending is supposed to have ai
product manager um but uh let me see if
there are any other questions that are
posted um does anybody have any
questions anything that we need to
discuss hopefully to raise your
hand I'm going to stop sharing my screen
kind of make it easier for myself to see
what's going on oh perfect thank you Kai
um and I've shared um the um the link
the link on comments as
well um okay somebody messaged me asking
um what what does like kind of personal
support look like so um as part of the
program uh you're more than welcome to
uh book at time in my calendar or Kai's
calendar or Corey's calendar and we're
happy to kind of give you um any advice
or any help that you would need at a
personal
level um but one thing you know we kind
of emphasize on is to make sure that um
you come to us for like things that are
very important otherwise um there will
be a community to support you we'll have
a forum that you can post questions now
um we start kind of rolling it out
halfway through cohort 5 but now cord
six The Forum is going to be kind of the
default so uh that'll work great as well
um and yes um somebody's asking if I can
send them a copy of the presentation yes
I'm happy to do that as well um so we'll
share that link afterwards uh but yeah
so like you would be able to um have
access to us as well and um as you're
kind of working through your PRD
sometimes um you might come across
questions or you kind of get stuck
somewhere um we will be giving you
feedback we'll kind of guide you through
it but uh we intentionally not get
involved in like certain steps like um
giving you an idea for example for a
product right uh because um I brought up
a really good point earlier in the
school where is like their PMS they're
supposed to be able to do this
themselves so um we're happy to help you
with those things if if you kind of get
stuck but um I think the goal is for you
to kind of over time get more and more
comfortable with um asking these
questions yourself um and another person
asked if the learning material will be
available afterwards um the answer is
yes they'll be available and like I said
because the industry is like kind of
evolving so quickly um there will be a
lot of like new learning material uh as
time goes by and um all this new
learning material will be available to
you for the next three years so um
hopefully that kind of put you a these
that what you're going to go through um
is going to be relevant like a year or
two from now because you can kind of
rely back on it and kind of stay updated
with what's going on um okay one more
private question like um how many hours
really you need I think yeah we talked
about that like I would say five to
seven to get the basics and like kind of
really understand what's going on in the
cohort but if you want to get the most
out of it like like you can spend like
10 to 15 hours to be honest like we have
a lot of content that we call them
optional um you don't have to
necessarily like read them or like spend
time on them but um if you do I think
it's a good thing um and um there's a
lot of like recordings from like
previous U sessions that we've had or
like guest speakers where we had like a
CEO of a company come in and kind of
shared their experience from a product
perspective you don't really have to
listen to those things but I think what
happens is you kind of spend more time
in the space uh you get more and more
comfortable uh with the language and and
you get better at it uh so that was
another question that somebody asked let
me see if there's anything else that's
coming in okay no I think that's it uh
for now um anything else that anybody
would like to add otherwise I will be
wrapping up the
session okay well thank you so much
everybody for coming in I really
appreciate it um and uh I'm very excited
to go through this with Kai again the
next cohort I think this cord was
amazing hopefully people got a lot of
value out of it and um yeah looking
forward to um having a bigger uh
Community um of AI product managers
right um so thank you so much somebody
asked how many weeks is the cohort that
starts in May so yes like like I said it
would be um four weeks um but it's four
weeks um we have one week of like kind
of the we call the Bonus Week at the end
uh where we still present give you guys
some additional learning material that's
kind of optional you can take it don't
take it if you don't want to but there
will be a demo period That's when you
actually be presenting to the rest of
the uh cohort and um hopefully this will
be an opportunity for you to also um
learn more about uh what everybody else
is doing and um there will be also like
kind of a we call it like a week zero U
which is um the week before we actually
start um going deep into both Ai nml and
product management side of it basically
we spend one week U making sure that you
have like some foundational knowledge
right like you need to know how neural
networks work uh before we can even have
any sort of meaningful conversation uh
so if you add all this really it's like
six weeks um but um technically it's
four weeks because uh technically it's
six weeks but officially it's like four
weeks because there's a pre-read week
and then there's a bonus week that we
offer um let me see what other questions
people are still asking me sending me
questions I'm going to answer um so
somebody asked how can I connect with
you um shoot me an email ban pme right
um You guys probably have my personal
email uh just shoot me an email um and
we can go from there um if there's
anything related to the cohort um I'd be
happy to answer um let me see what else
if anybody else is posting anything else
one second
okay I think this is it for now uh thank
you so much everybody I hope you enjoyed
today's session I call any any final
words before I wrap it up no I think
you've covered
everything in the cohort 6 yeah hope to
see you guys in cohort 6 and uh have a
great great weekend take care
bye
Browse More Related Video
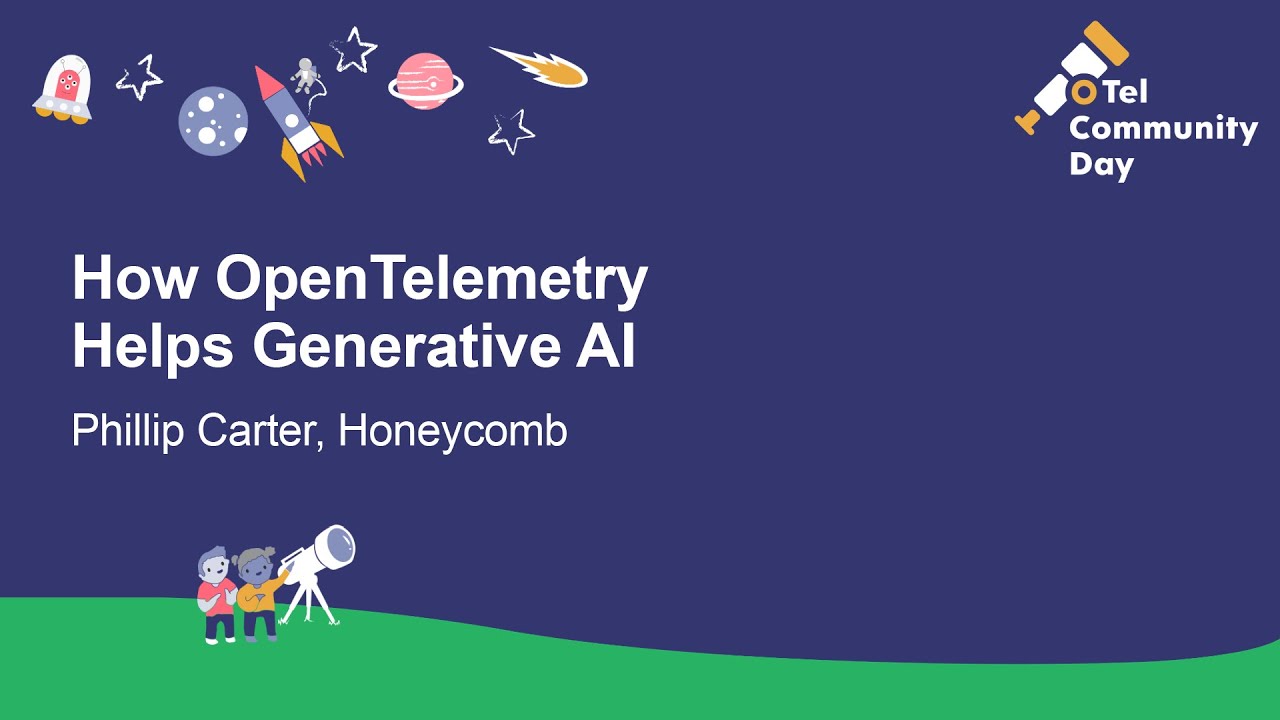
How OpenTelemetry Helps Generative AI - Phillip Carter, Honeycomb
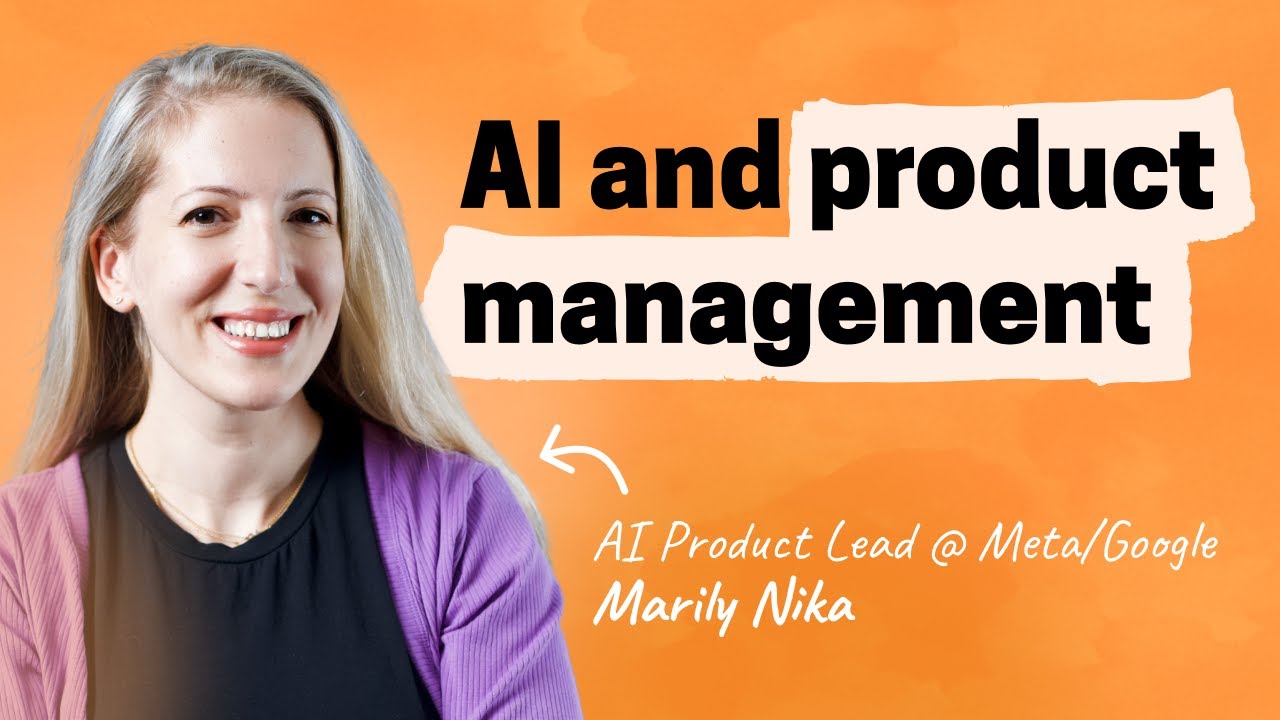
AI and product management | Marily Nika (Meta, Google)

Config 2024: How to bring the craft back to product management (Peter Yang, Product Lead, Roblox)
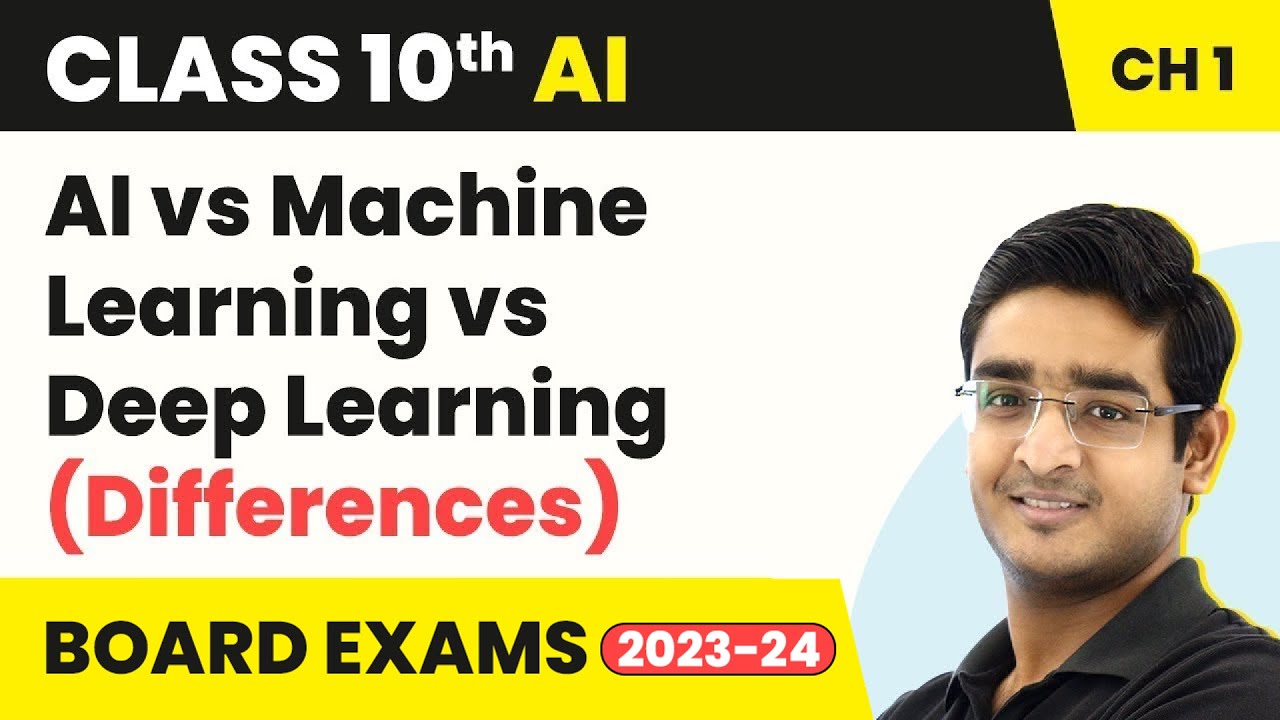
Artificial Intelligence Class 10 Ch 1 |AI vs Machine Learning vs Deep Learning (Differences) 2022-23
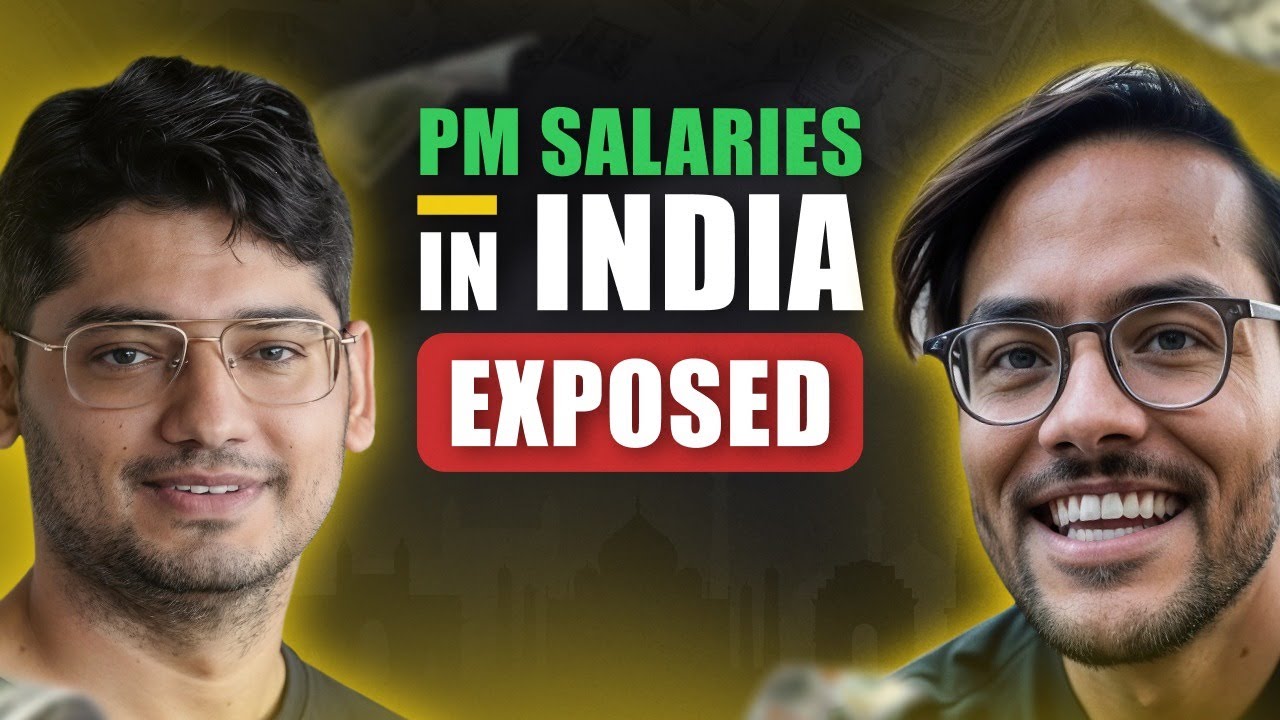
The Indian Product Manager Salary Report with Aakash Gupta (Ex-VP Apollo.io) | HelloPM Podcast
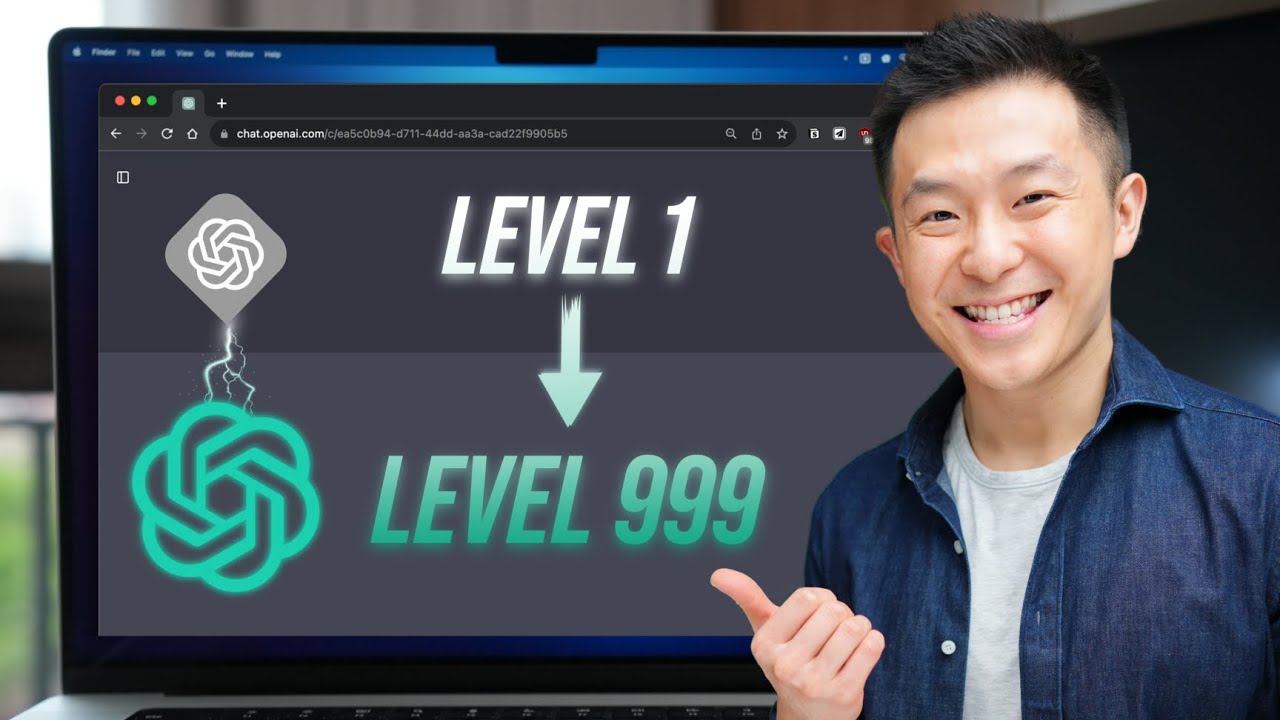
Beginner to Pro with ChatGPT in One Video!
5.0 / 5 (0 votes)