SIR Modell - Teil II
Summary
TLDRDieses Video erklärt das SIR-Modell, eine mathematische Methode zur Simulation der Ausbreitung von Epidemien. Es zeigt, wie durch numerische Methoden die Entwicklung von infizierten, genesenen und anfälligen Personen über Zeit modelliert wird. Das Ziel ist es, die Kurve zu flach zu halten, indem der Infektionsindex gesenkt wird, um die Belastung des Gesundheitssystems zu verringern. Die Wichtigkeit von Hygienemaßnahmen und sozialer Distanz wird hervorgehoben, um die Verbreitung zu verlangsamen.
Takeaways
- 🧑🏫 Das Skript stellt das SIR-Modell vor und erklärt, wie dieses mathematische Modell abgeleitet wird.
- 🔍 Die Materialien dienen ausschließlich der Bildung und enthalten keine offiziellen Daten; das Modell ist stark vereinfacht.
- ⚠️ Die Ergebnisse des Modells dürfen nicht für politische Entscheidungen, Meinungsbildung oder entsprechende Maßnahmen genutzt werden.
- 📈 Die Differentialgleichungen des SIR-Modells beschreiben die Veränderungen der Gruppen Suszeptible, Infizierte und Genesene.
- 📊 Die Lösung dieser Gleichungen erfolgt numerisch durch Diskretisierung, also durch die Verwendung eines Algorithmus, der von einem Computer ausgeführt werden kann.
- 📚 Die Diskretisierung betrachtet die Veränderung innerhalb eines Zeitintervalls, z.B. die Veränderung von Suszeptiblen (ΔS) innerhalb eines Tages (Δt).
- 🌐 Die anfänglichen Werte für S, I und R sowie die Konstanten c und w sind für die Lösung der Gleichungen erforderlich.
- 📉 Das Modell zeigt die zeitabhängige Entwicklung der drei Gruppen: Suszeptible in grün und gestrichelt, Infizierte in rot und Genesene in blau und punktiert.
- 📈 Die Kurve des Infizierten zeigt ein exponentielles Wachstum in der frühen Phase der Epidemie, das nicht unendlich andauern kann.
- 🛑 Die Maßnahmen, die zur Flachstellung der Kurve führen, beinhalten die Reduzierung des Infektionsgrades durch die Reduzierung von Kontakten zwischen Individuen.
- 🔬 Durch das Modell können verschiedene Szenarien simuliert werden, ohne dass tatsächliche Infektionen stattfinden müssen.
- 🔍 Die Reduzierung des Infektionsgrades auf die Hälfte verschiebt das Hoheitsmaximum der Epidemie und reduziert die Anzahl der Infizierten pro 100.000 Einwohner.
- 🌱 Nach etwa 100 Tagen ist die Epidemie gemäß der Simulation vorüber, da alle Individuen immun sind.
- 🤝 Es ist wichtig, die Empfehlungen von Wissenschaftlern und Politikern zu befolgen, um die Belastung des Gesundheitssystems zu verringern und die Epidemie zu überwinden.
Q & A
Was ist das SIR-Modell?
-Das SIR-Modell ist ein mathematisches Modell, das verwendet wird, um die Verbreitung von Infektionskrankheiten in einer Bevölkerung zu beschreiben und zu verfolgen.
Welche drei Gruppen sind im SIR-Modell enthalten?
-Die drei Gruppen sind Suszeptible (S), Infizierte (I) und Genesene (R).
Was sind die beiden Konstanten c und w im SIR-Modell?
-Die Konstanten c und w repräsentieren die Infektionsrate und die Wiederherstellungsrate im SIR-Modell.
Wie wird das SIR-Modell in der Praxis gelöst?
-Das SIR-Modell wird numerisch gelöst, indem Algorithmen verwendet werden, die von Computern ausgeführt werden und die Diskretisierung der Differentialgleichungen beinhalten.
Was bedeutet die Diskretisierung im Zusammenhang mit dem SIR-Modell?
-Die Diskretisierung ist ein numerisches Verfahren, bei dem die Veränderung innerhalb eines Zeitintervalls (z.B. eines Tages) statt der Ableitung verwendet wird, um die Differentialgleichungen zu lösen.
Wie wird die Veränderung der Suszeptiblen (S) im SIR-Modell berechnet?
-Die Veränderung der Suszeptiblen wird durch die Formel Delta S = -2 / (4.5 * T / N * I) berechnet, wobei T die Anzahl der Tage und N die Gesamtbevölkerung ist.
Was zeigt das SIR-Modell in Bezug auf die Verbreitung einer Epidemie?
-Das SIR-Modell zeigt die zeitabhängige Entwicklung der drei Gruppen Suszeptible, Infizierte und Genesene und wie sie sich während einer Epidemie verändern.
Was ist die Bedeutung von 'flatten the curve' im Kontext des SIR-Modells?
-'Flatten the curve' bezieht sich darauf, die Kurve der Infizierten zu verlangsamen, um die Belastung des Gesundheitssystems zu verringern und die Spitzenlast zu reduzieren.
Wie kann die Infektionsrate im SIR-Modell reduziert werden?
-Die Infektionsrate kann reduziert werden, indem die Kontakte zwischen Individuen verringert werden, was die Wahrscheinlichkeit eines Kontakts zwischen Suszeptiblen und Infizierten vermindert.
Was zeigt das Modell, wenn die Infektionsrate halbiert wird?
-Wenn die Infektionsrate halbiert wird, verschiebt sich der Höhepunkt der Epidemie in der Regel, und die Anzahl der Infizierten pro 100.000 Einwohner sinkt, was die Belastung des Gesundheitssystems reduziert.
Was ist die Hauptbotschaft des Videos über das SIR-Modell?
-Die Hauptbotschaft ist, dass das Verständnis mathematischer Modelle wie das SIR-Modell uns hilft, die Verbreitung von Epidemien zu verstehen und zu simulieren, um die Auswirkungen verschiedener Maßnahmen zu bewerten.
Outlines
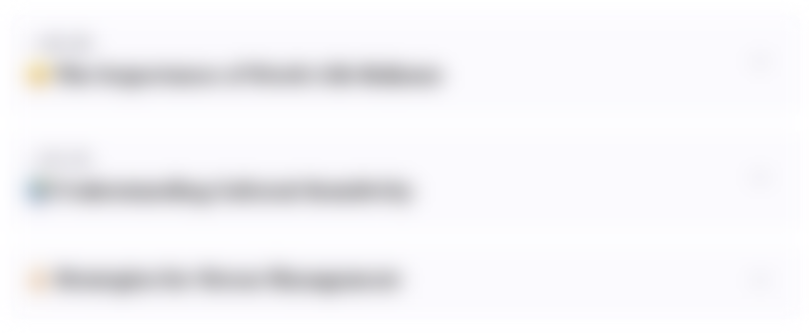
This section is available to paid users only. Please upgrade to access this part.
Upgrade NowMindmap
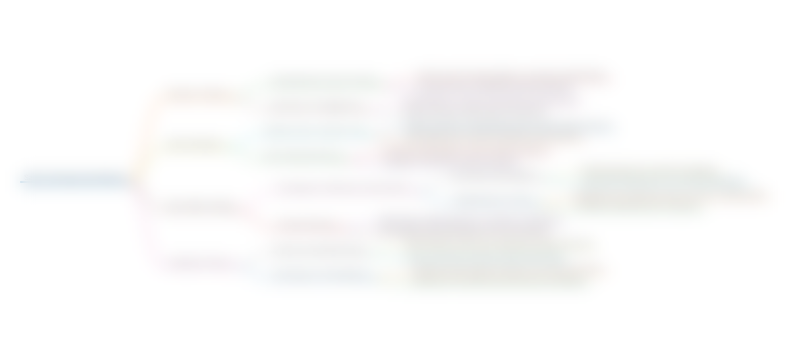
This section is available to paid users only. Please upgrade to access this part.
Upgrade NowKeywords
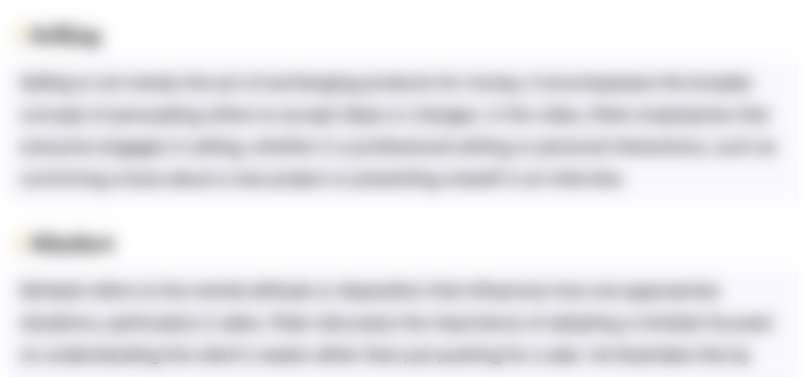
This section is available to paid users only. Please upgrade to access this part.
Upgrade NowHighlights
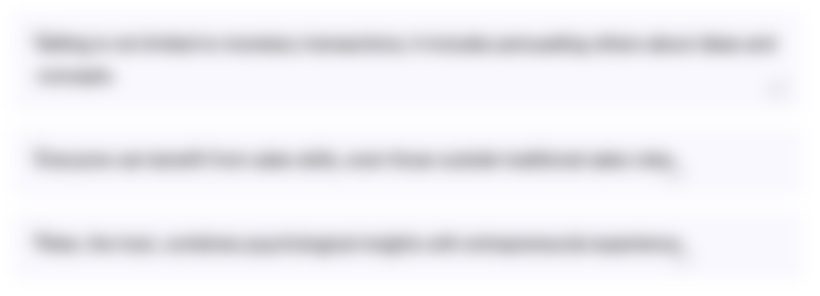
This section is available to paid users only. Please upgrade to access this part.
Upgrade NowTranscripts
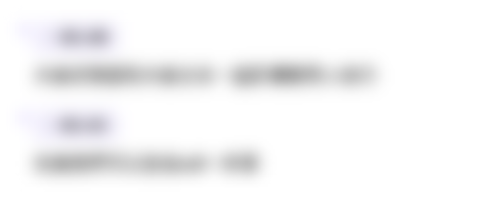
This section is available to paid users only. Please upgrade to access this part.
Upgrade NowBrowse More Related Video
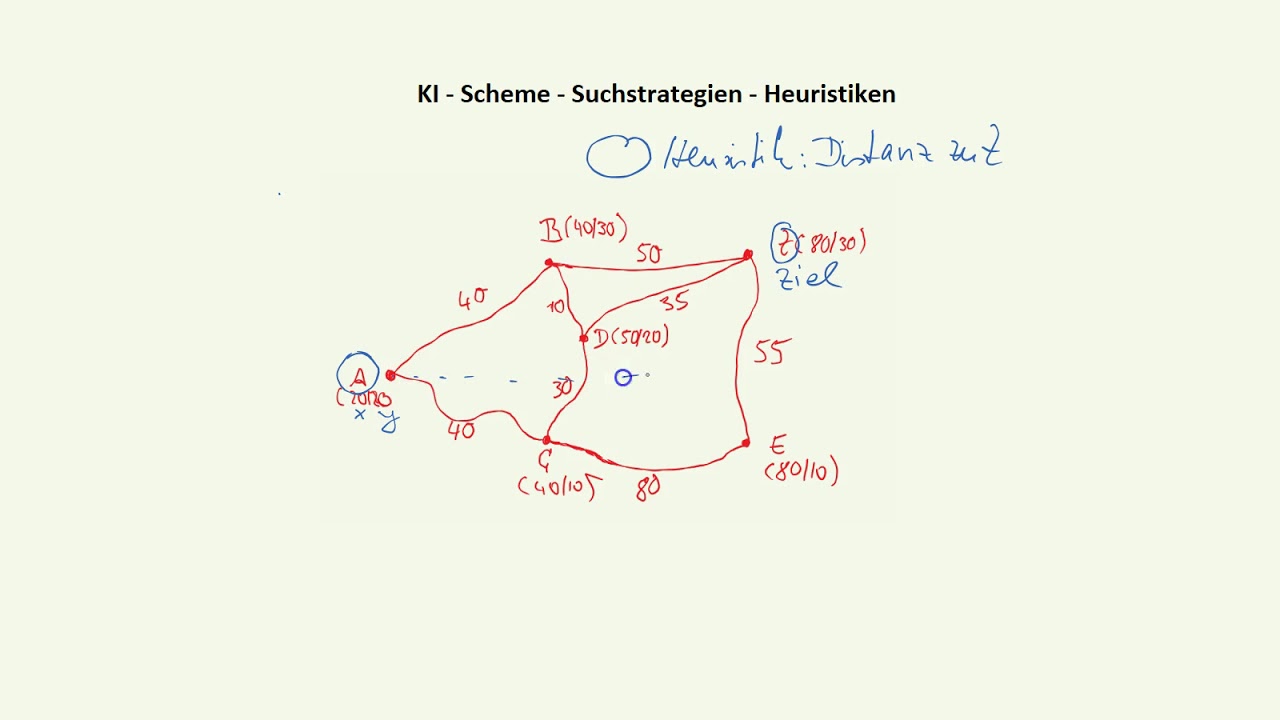
Ki und Scheme - Suchstrategien - Heuristiken
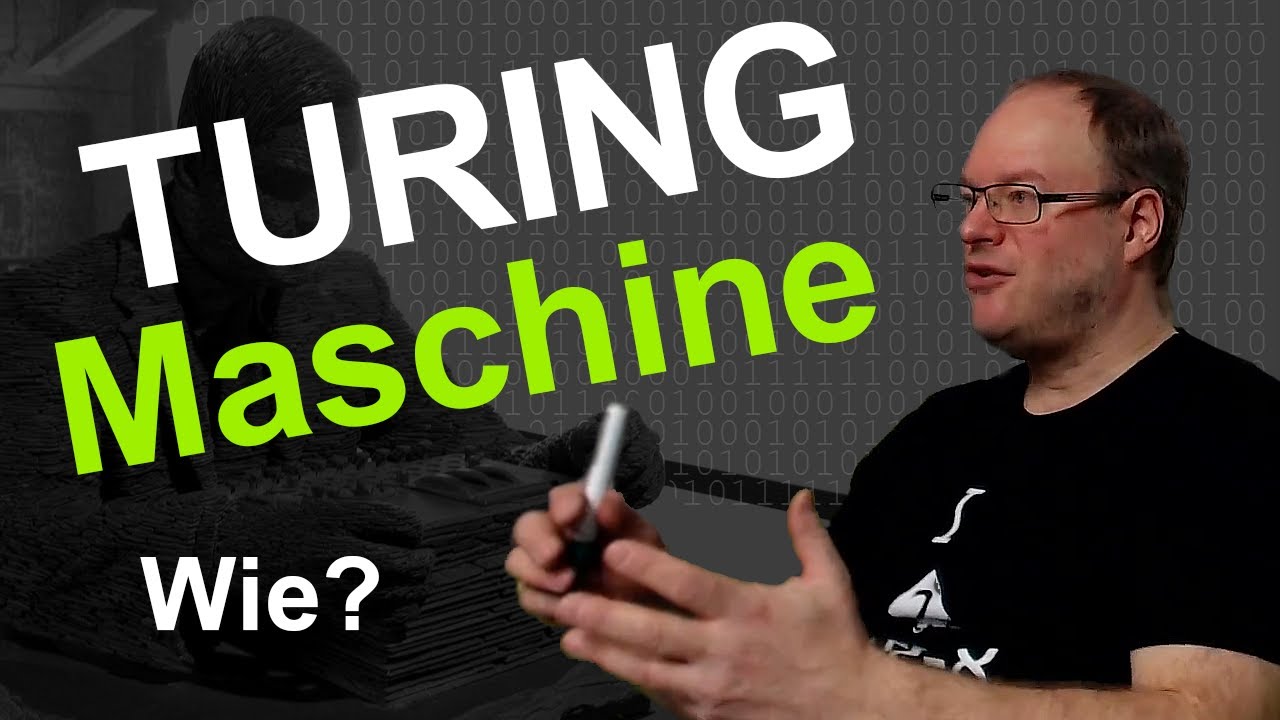
Wie funktioniert die Turingmaschine von Alan Turing? - Einfach erklärt auf Deutsch (German)
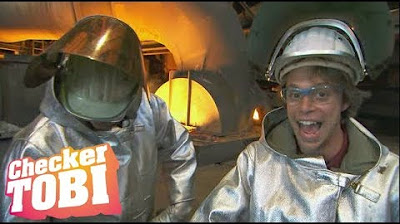
Der Stahl-Check | Reportage für Kinder | Checker Tobi
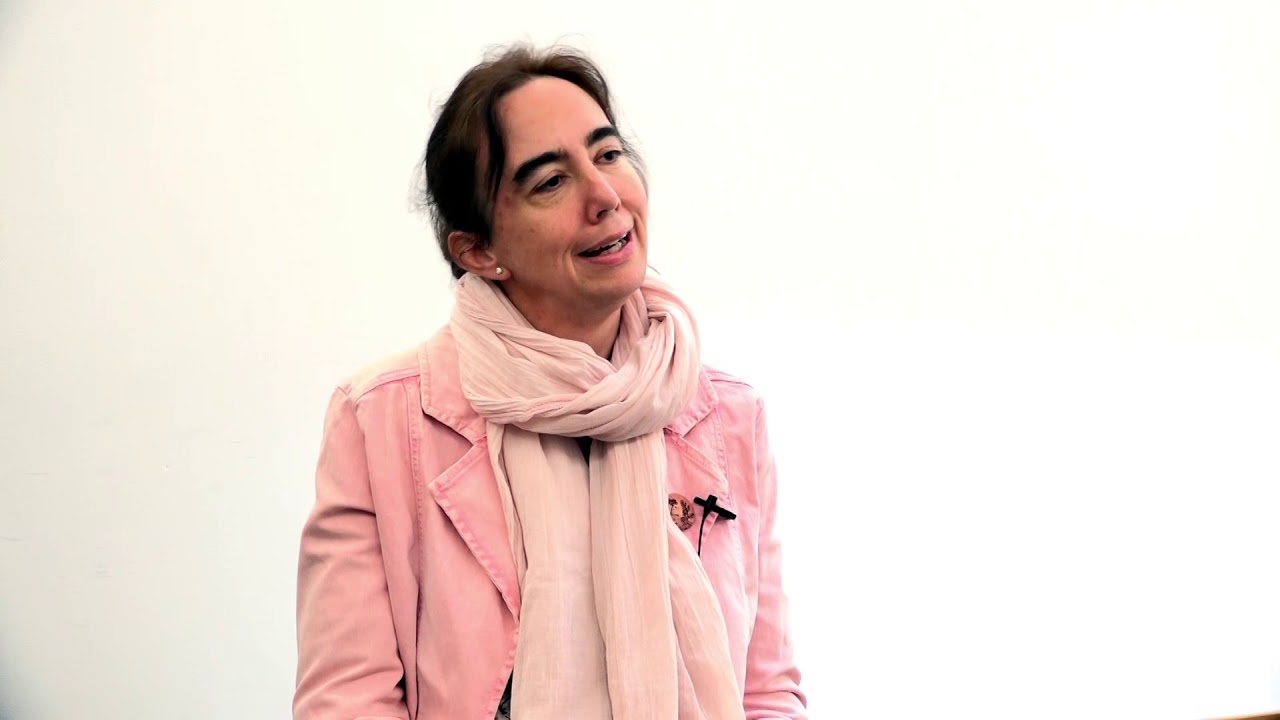
Methodisches Arbeiten in der Beratung – Zirkuläre Fragen
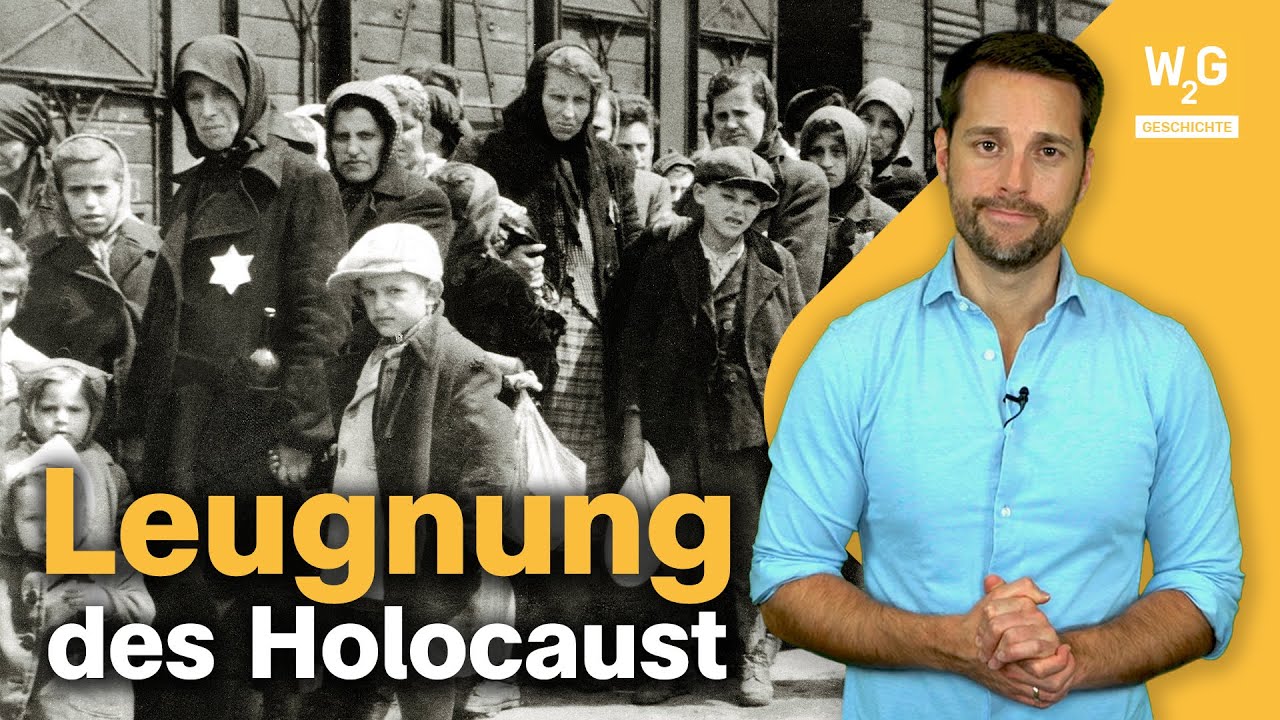
Holocaustleugnung: Welche Lügen kursieren und wie ihr sie erkennt
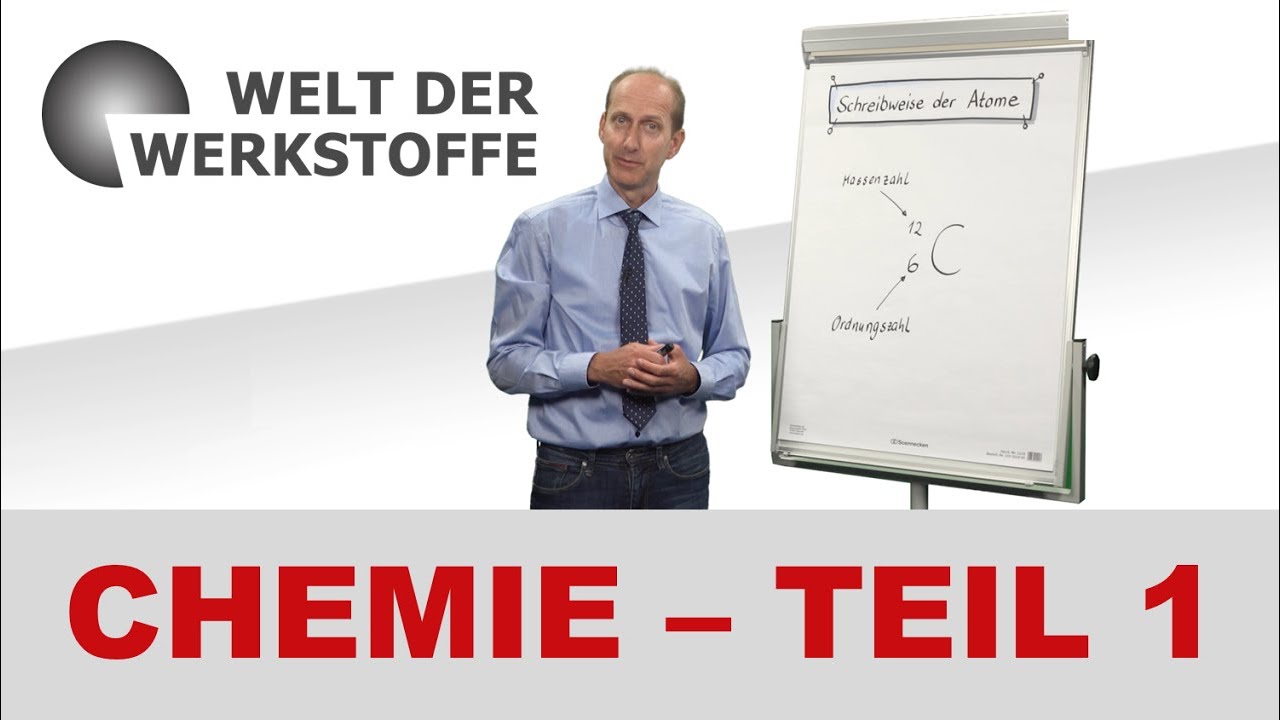
Die Welt der Werkstoffe, Chemie, Teil 1
5.0 / 5 (0 votes)