Where AI is today and where it's going. | Richard Socher | TEDxSanFrancisco
Summary
TLDRDie Präsentation untersucht die Entwicklung und den aktuellen Stand der künstlichen Intelligenz (KI). Ursprünglich konzentrierte sich die KI-Forschung auf Spiele wie Schach, doch inzwischen hat sie sich auf komplexere Herausforderungen wie Spracherkennung und Computer Vision verlagert. Fortschritte in der Bildklassifizierung und Sprachverarbeitung haben bedeutende Anwendungen in Bereichen wie medizinischer Diagnostik ermöglicht. Es wird betont, dass KI ein Werkzeug bleibt, das sowohl positive als auch negative Auswirkungen haben kann, abhängig von seiner Nutzung und den zugrunde liegenden Daten. Zukünftige Entwicklungen könnten unsere Kommunikation und Effizienz erheblich verbessern, erfordern jedoch auch sorgfältige Regulierung, um Verzerrungen zu vermeiden.
Takeaways
- 🔍 Die Definition von Künstlicher Intelligenz (KI) ist fließend und verändert sich, wenn Probleme gelöst werden.
- 🤖 Frühere KI-Forschung konzentrierte sich auf das Lösen von Spielen wie Schach, was als Prolog-Umgebung ohne die Komplexitäten der realen Welt angesehen wurde.
- 🗣️ Spracherkennung war einst ein großes Problem, bis Deep Learning 2010 Fortschritte erzielte und es zu einer Routine-Technologie machte.
- 👀 Computer Vision, insbesondere Bildklassifizierung, hat durch Deep Learning in den letzten Jahren enorme Fortschritte gemacht.
- 🌟 End-to-End-Modelle in der KI ermöglichen es, von Rohdaten zu abstrakten Darstellungen zu lernen, was früher manuell definiert werden musste.
- 🧠 Die Schichtenkomplikation in KI-Modellen spiegelt die Verarbeitung im menschlichen Gehirn wider, von einfachen Kanten bis zu komplexen Objektteilen und vollständigen Objekten.
- 🎨 Kombination von Computer Vision und Sprachverarbeitung ermöglicht innovative Anwendungen wie das Beschreiben von Bildern und das Beantworten von Fragen zu ihnen.
- 🏥 KI hat auch in der Medizin, insbesondere in der Onkologie, großes Potenzial, z.B. bei der automatischen Zählung von Blutzellen.
- 🏗️ In der Zukunft könnte KI bei der Automatisierung grundlegender menschlicher Bedürfnisse wie der Landwirtschaft und dem Bauwesen helfen.
- 🤖 Motorische Kontrolle ist ein noch aktiver Forschungsbereich in der KI, da Roboter oft nicht so flexibel sind wie erhofft.
- 📈 Die Anwendung von KI in der Sprachverarbeitung, insbesondere beim Textklassifizierung, hat sich verbessert und kann nun Kontext besser erfassen.
- 📚 Fortschritte in der KI, insbesondere in der Sprachverarbeitung, eröffnen neue Möglichkeiten für Aufgaben wie Textzusammenfassung und Fragebeantwortung.
- 🌐 KI ist ein Werkzeug und sollte sorgfältig eingesetzt werden, um negative Auswirkungen zu vermeiden, die durch voreingestellte Daten oder Entscheidungsprozesse entstehen könnten.
- 👥 Die KI-Gemeinschaft ist sich der Bedeutung von Vielfalt und der Notwendigkeit ihrer Förderung im Forschungsprozess bewusst.
Q & A
Was ist die grundlegende Herausforderung beim Definieren von künstlicher Intelligenz?
-Die Definition der künstlichen Intelligenz ist schwierig, da sie ständig sich bewegt. Sobald ein Problem gelöst wird, wird es oft nicht mehr als künstliche Intelligenz bezeichnet.
Warum haben frühere Forschungen in der künstlichen Intelligenz mit Schach begonnen?
-Frühere Forschungen begannen mit Schach, weil Forscher glaubten, dass das Lösen solcher Probleme viele andere Probleme lösen könnte, da Schach ein komplexes Spiel ist, das Mathematik, Logik und strategisches Denken erfordert.
Was hat das tiefe Lernen für das Spracherkennungsproblem gebracht?
-Seit dem Jahr 2010 hat das tiefe Lernen erhebliche Fortschritte im Bereich der Spracherkennung gemacht, was ein Problem war, das vorher als besonders schwierig galt.
Was sind die Hauptkomponenten eines End-to-End-Lernmodells in der Bildverarbeitung?
-Ein End-to-End-Lernmodell nimmt Rohdaten wie die Pixel eines Bildes und lernt komplexe Repräsentationen, um z.B. Objekte im Bild zu identifizieren, von einfachen Kanten bis hin zu vollständigen Objekten.
Wie funktioniert die Farbcodierung in der visuellen Wahrnehmung, um die Aufmerksamkeitsbereiche eines Modells zu visualisieren?
-Die Farbcodierung visualisiert, auf welche Teile des Bildes das Modell beim Generieren einer Beschreibung schaut, indem es die Bereiche hervorhebt, auf die das Modell beim Erzeugen von Wörtern konzentriert.
Was ist das visuelle Fragen-Beantwortungs-System und wie nützlich kann es sein?
-Das visuelle Fragen-Beantwortungs-System ist eine Aufgabe, bei der das Modell mit einem Bild, einer Frage und einer Antwort trainiert wird und dann versucht, die Frage anhand des Bildes zu beantworten. Es kann dabei helfen, das Bild besser zu verstehen und ist in Bereichen wie der Medizin oder der Bildbeschreibung nützlich.
Welche Anwendungen hat die künstliche Intelligenz im medizinischen Bereich, insbesondere in der Onkologie?
-In der Onkologie kann künstliche Intelligenz dazu beitragen, das Zählen von Blutzellen zu automatisieren, was die Onkologie-Versorgung verbessern und die Identifizierung von Infektionen unterstützen kann.
Welche Herausforderungen gibt es im Bereich der motorischen Steuerung in der künstlichen Intelligenz?
-Im Bereich der motorischen Steuerung haben wir noch viele Herausforderungen zu meistern, da selbst teure Roboter oft Schwierigkeiten haben, einfache Aufgaben wie das Öffnen von Türen oder das Drehen von Hebeln zu erledigen.
Welche Fortschritte hat die natürliche Sprachverarbeitung in Bezug auf die Textklassifizierung gemacht?
-Die natürliche Sprachverarbeitung hat erhebliche Fortschritte gemacht, indem sie den gesamten Kontext von Sätzen erfasst und nicht nur einzelne Wörter betrachtet, was zu einer korrekteren Klassifizierung von Sätzen führt.
Was sind die Herausforderungen im Bereich der Textzusammenfassung?
-Die Textzusammenfassung ist eine schwierige Aufgabe, da Modelle normalerweise nur kurze, kohärente Sätze generieren können. Die Automatisierung der Zusammenfassung längerer Dokumente ist noch ein offenes Forschungsproblem.
Welche Rolle kann die künstliche Intelligenz in der Fragebeantwortung spielen?
-Fragebeantwortung kann als eine Aufgabe angesehen werden, die fast alle anderen NLP-Aufgaben umfasst, da sie Übersetzungen, Stimmungen, Zusammenfassungen und mehr beinhalten kann.
Welche Bedeutung hat die Qualität der Trainingsdaten für die künstliche Intelligenz?
-Die Qualität der Trainingsdaten ist entscheidend, da sie die Fähigkeiten der künstlichen Intelligenz formt. Wenn die Daten voreingenommen sind, kann die AI diese Muster aufnehmen und sogar verstärken.
Welche Auswirkungen kann die künstliche Intelligenz auf die Arbeitswelt haben?
-Die künstliche Intelligenz kann die Arbeitswelt revolutionieren, indem sie grundlegende menschliche Bedürfnisse wie Landwirtschaft und Bauwesen automatisiert und so die Produktivität erhöht.
Was bedeutet die Vielfalt im Zusammenhang mit der künstlichen Intelligenz?
-Die Vielfalt bezieht sich auf die Notwendigkeit, verschiedene Perspektiven und Hintergründe in die Entwicklung und Anwendung der künstlichen Intelligenz einzubeziehen, um Vorurteile und Bias zu vermeiden.
Outlines
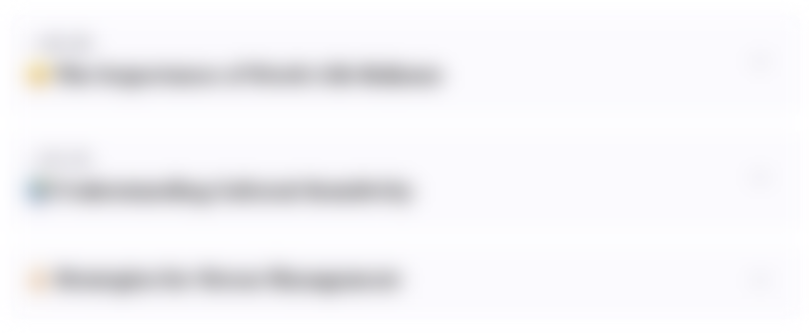
This section is available to paid users only. Please upgrade to access this part.
Upgrade NowMindmap
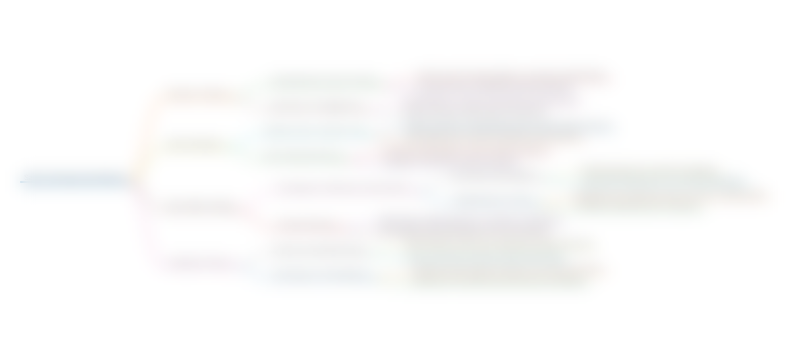
This section is available to paid users only. Please upgrade to access this part.
Upgrade NowKeywords
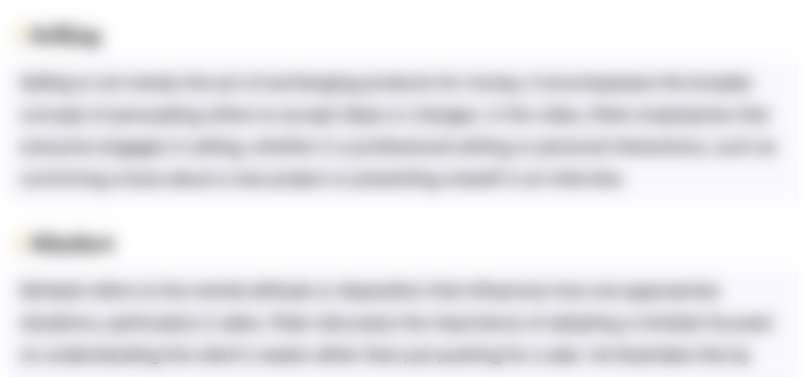
This section is available to paid users only. Please upgrade to access this part.
Upgrade NowHighlights
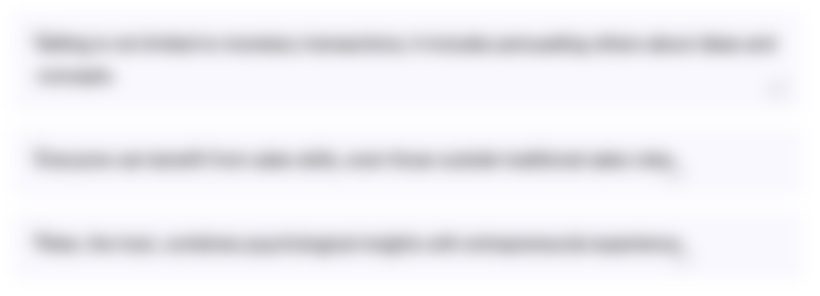
This section is available to paid users only. Please upgrade to access this part.
Upgrade NowTranscripts
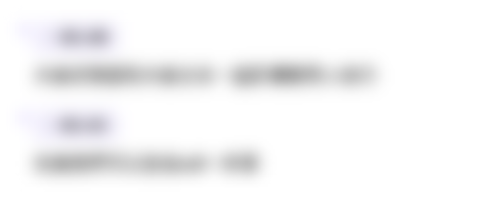
This section is available to paid users only. Please upgrade to access this part.
Upgrade Now5.0 / 5 (0 votes)