Michał Kudelski (TCL): Inpainting using Deep Learning: from theory to practice
Summary
TLDRThe speaker from TCL Research Europe introduces their AI project focused on image and video inpainting using deep learning, a technique to reconstruct lost or deteriorated parts of visual media. Applications include restoring old photos, scene editing, and even uncensoring animations. The talk covers the use of partial convolutions, challenges in training, and practical issues like batch normalization and high-resolution inpainting. The presentation concludes with sample results and an invitation to learn more about TCL's innovative projects.
Takeaways
- 📍 The speaker is from TCL Research Europe, a new R&D center focusing on AI methods, particularly in computer vision for smart devices like TVs and smartphones.
- 🎨 'Inpainting' is the process of reconstructing lost or deteriorated parts of images or videos, which is the main topic of the presentation.
- 🤖 Deep learning, specifically partial convolutions, is the approach used in the speaker's project for image inpainting, which is more advanced than traditional methods.
- 🔍 The project's practical applications include restoring old photos, automatic scene editing, and even uncensoring images, demonstrating the versatility of inpainting.
- 🛠️ Training data for inpainting models can be obtained from existing databases or by generating random masks to simulate missing parts of images.
- 🌟 The architecture of the inpainting model is based on an encoder-decoder structure, with partial convolutions accounting for missing data in the input image.
- 🔧 The model's loss function is a combination of several elements, including pixel-wise loss, perceptual loss, style loss, and total variation loss, each contributing to the quality of the inpainted output.
- 🚀 Challenges in inpainting include issues with batch normalization due to varying mask sizes and the increased computational demand of high-resolution images.
- 🔍 Solutions to these challenges include training with diversified masks, using instance normalization, or removing normalization layers altogether.
- 🔄 The speaker also discusses the potential of using adversarial losses and a new loss function called IDM-RF to improve the realism and diversity of inpainted images.
- 📈 TCL Research Europe is actively working on advancing inpainting technology, with a focus on practical applications and overcoming technical hurdles for real-world use.
Q & A
What is TCL Research Europe and what is its primary focus?
-TCL Research Europe is a new R&D center established by TCL in Warsaw. It primarily focuses on AI methods, specifically in the area of computer vision, as TCL is a major manufacturer of Smart TVs and smartphones.
What is the concept of 'inpainting' in the context of the presented project?
-Inpainting refers to the process of reconstructing lost or deteriorated parts of images or videos. It involves using an input image with a mask indicating the missing parts, and then reconstructing those parts based on the surrounding context.
Why is the topic of inpainting considered interesting and important?
-Inpainting is considered interesting due to its applications in various fields such as restoring old photos and videos, automatic scene editing, retouching, denoising, and even entertainment like uncensoring Japanese animations. It was also a topic at the prestigious NIPS conference, indicating its significance in the AI community.
What is the role of deep learning in the inpainting project presented?
-Deep learning is used to build an inpainting model that can effectively reconstruct missing image parts. It is based on a recent paper introducing partial convolutions, which is a technique that takes into account the masks indicating missing areas during the convolution process.
What are partial convolutions and how do they differ from traditional convolutions?
-Partial convolutions are a modification of traditional convolutions that account for missing data by multiplying the input patch with a mask before performing the convolution. This means that during the convolution, only the pixels outside of the mask are considered, and the mask is updated after each layer to reflect the reconstructed pixels.
What are some practical issues encountered during the inpainting project?
-Some practical issues include problems with batch normalization due to varying mask sizes, difficulties with high-resolution inpainting due to increased computational cost, and challenges with reconstructing detailed textures at higher resolutions.
How can batch normalization issues be addressed in the inpainting model?
-Batch normalization issues can be addressed by using techniques such as freeze training, where batch normalization layers are frozen after initial training, allowing the model to adapt to different mask sizes during fine-tuning. Other methods include using instance normalization or removing batch normalization layers altogether.
What are some approaches to handle high-resolution inpainting challenges?
-To handle high-resolution inpainting challenges, one can reduce model size, optimize the model for inference, use quantization techniques, or leverage specialized hardware like DSP processors. Additionally, increasing the receptive fields of the model or using architectures with different receptive field sizes can help improve results.
What is the significance of mask generation in the inpainting process?
-Mask generation is crucial as it defines the areas of the image that need to be inpainted. Specialized masks can be generated using techniques like semantic segmentation or object detection to focus on specific elements like faces or objects, which can be useful for automatic scene editing or fine-tuning the model for specific applications.
Can you provide an example of how the inpainting model can be applied to facial images?
-The inpainting model can be trained on facial images to reconstruct missing parts of faces realistically. It can also be used for facial retouching, such as smoothing out wrinkles or removing imperfections, resulting in a retouched and more aesthetically pleasing facial image.
Outlines
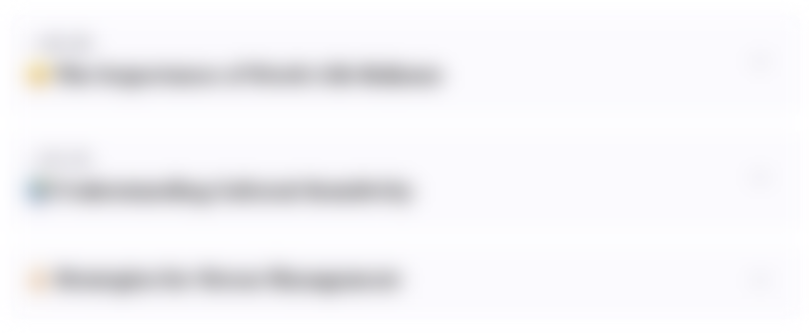
This section is available to paid users only. Please upgrade to access this part.
Upgrade NowMindmap
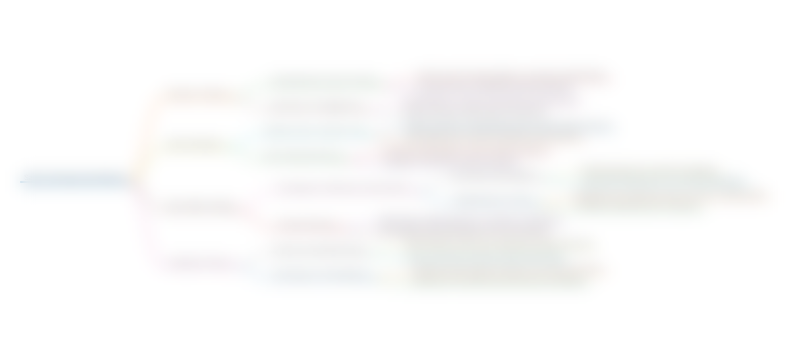
This section is available to paid users only. Please upgrade to access this part.
Upgrade NowKeywords
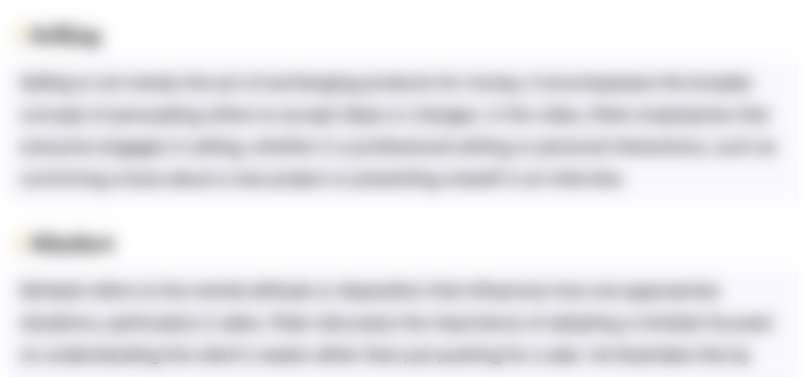
This section is available to paid users only. Please upgrade to access this part.
Upgrade NowHighlights
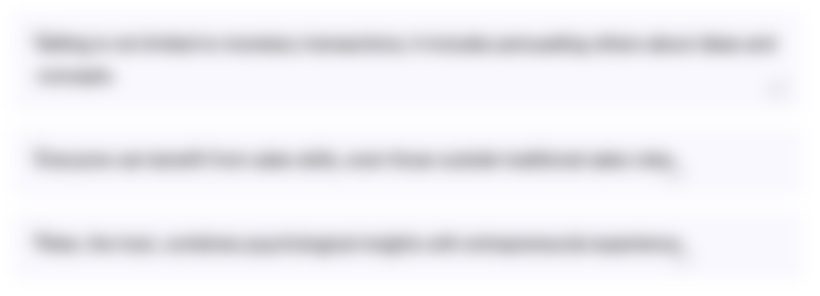
This section is available to paid users only. Please upgrade to access this part.
Upgrade NowTranscripts
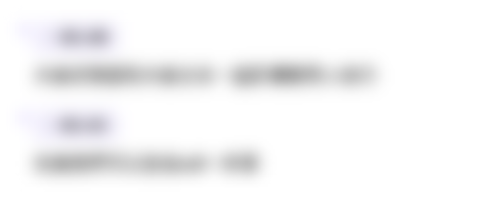
This section is available to paid users only. Please upgrade to access this part.
Upgrade NowBrowse More Related Video
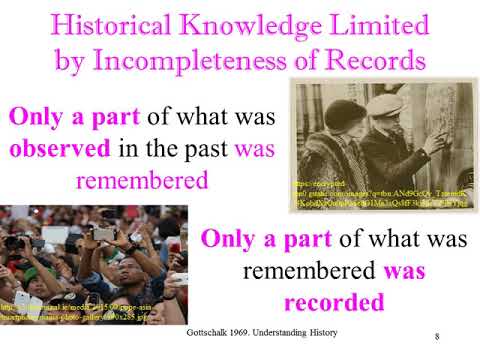
Lecture 1 Historical Method
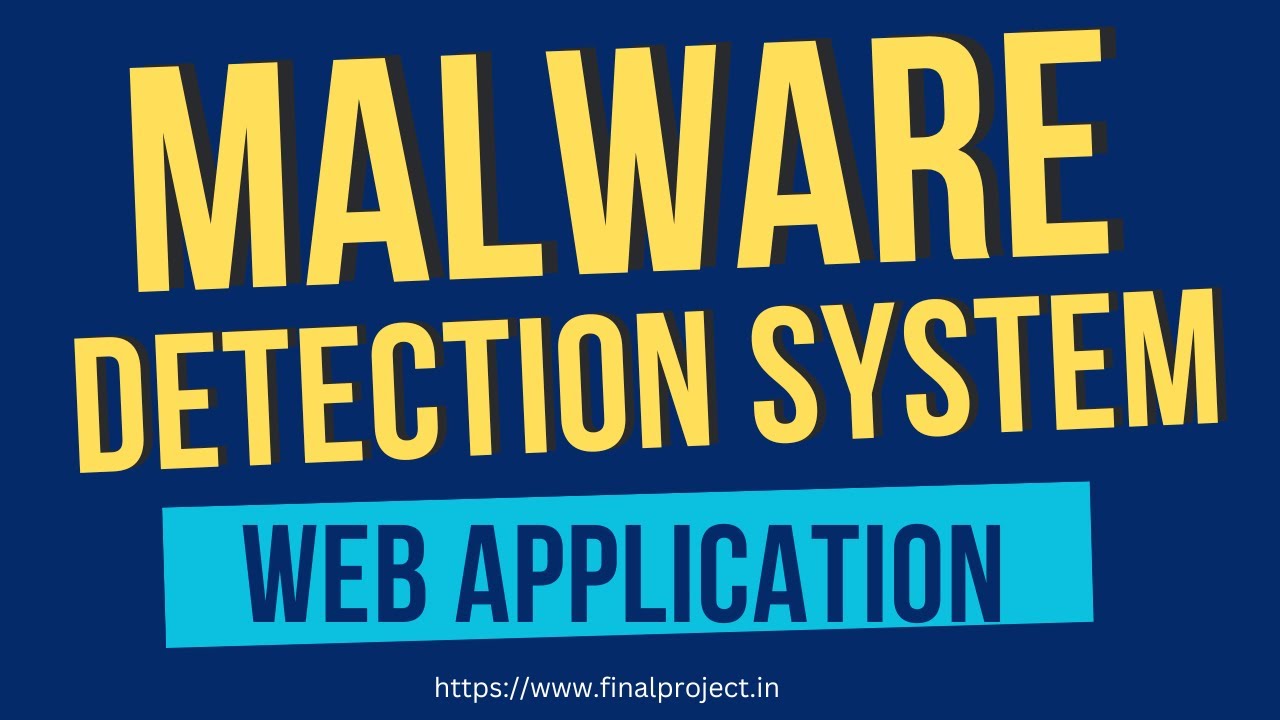
Malware Detection Using Machine learning and Deep Learning #finalyearproject
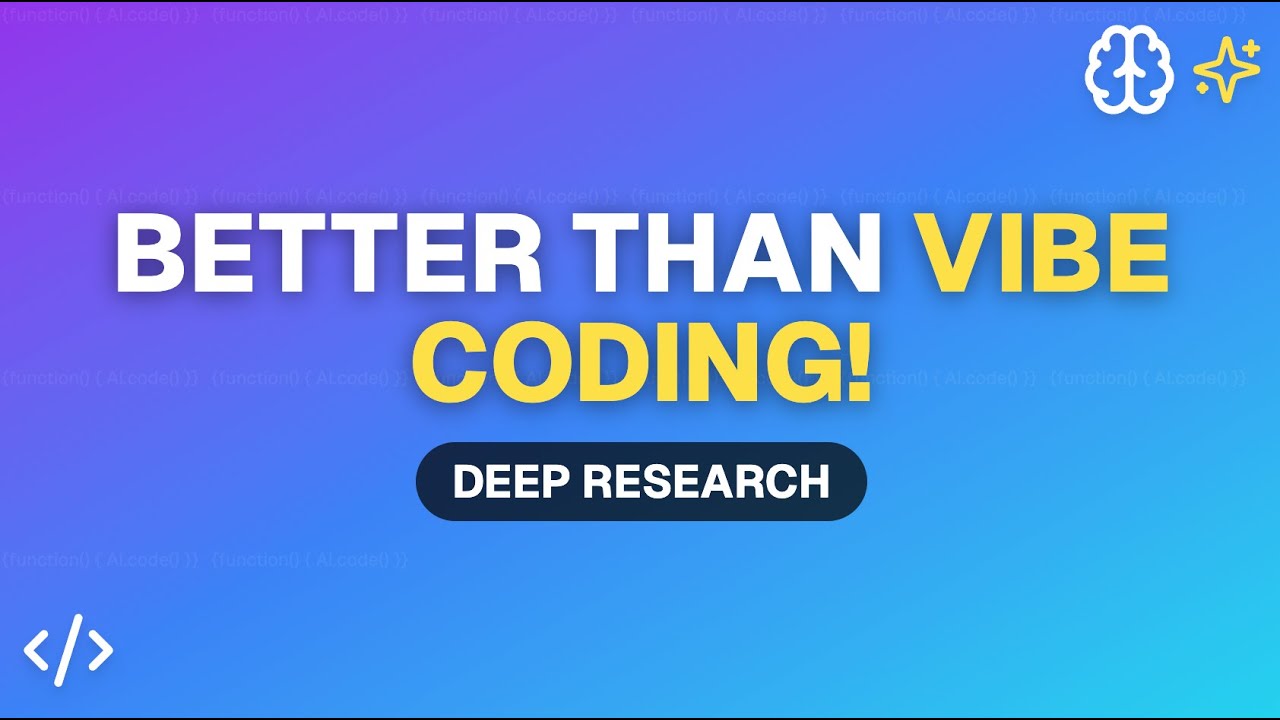
Try this instead of vibe coding!
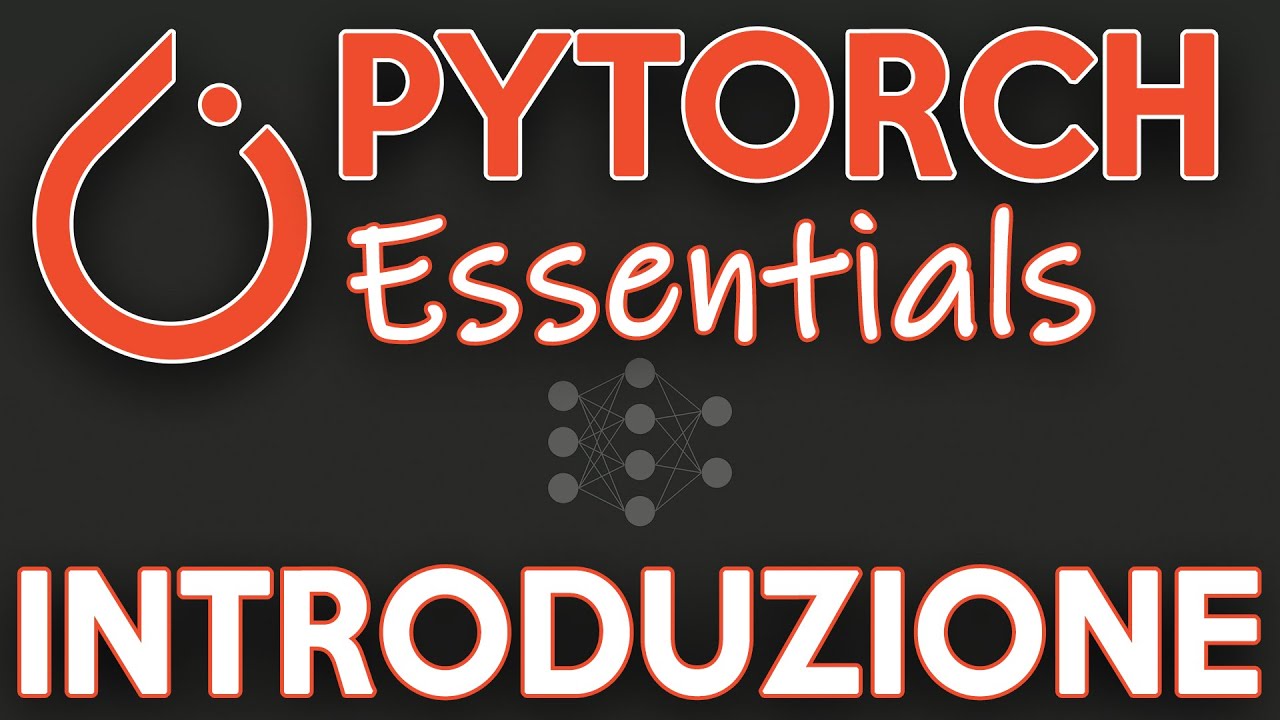
Cos'è PyTorch e Perché Tutti Lo USANO? INTRODUZIONE Al Potere dei Tensori! 🤔💡

SSR2093 Lab Practical: Task 1
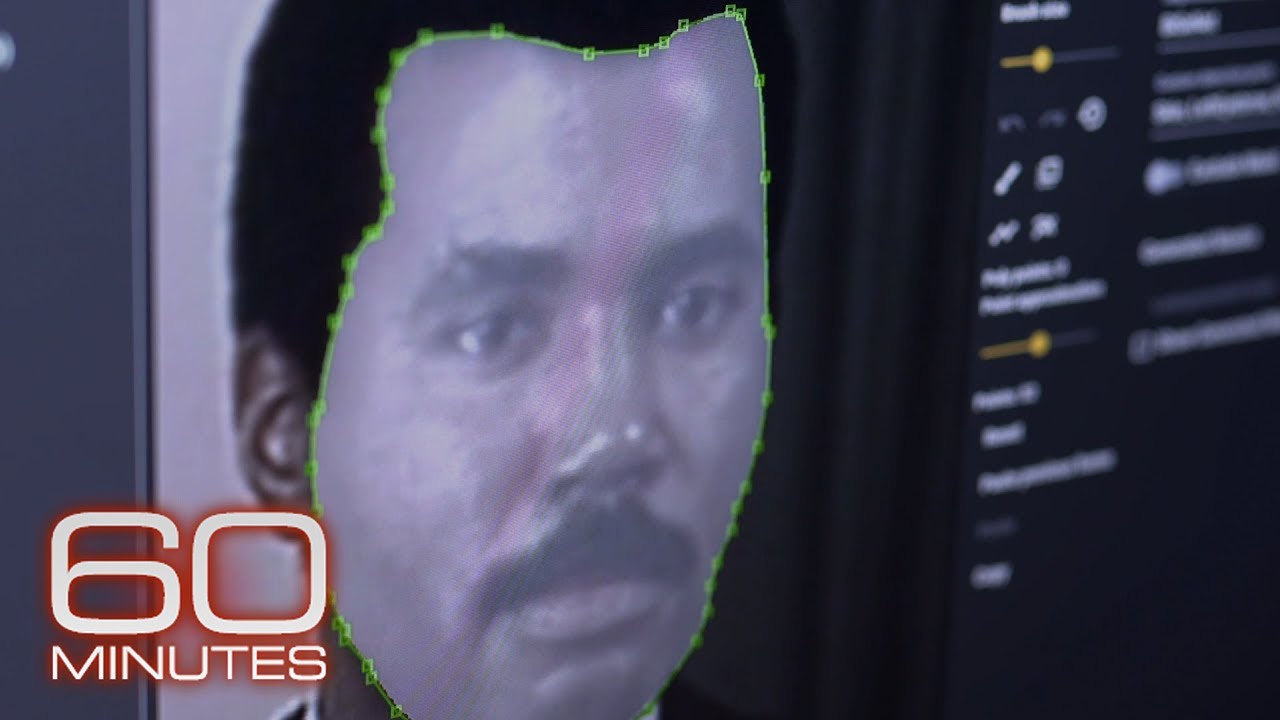
How synthetic media, or deepfakes, could soon change our world
5.0 / 5 (0 votes)