Lecture 4.2 - Association between two categorical variables - Introduction
Summary
TLDRThe script discusses the concept of association between variables, particularly focusing on the relationship between categorical and numerical variables. It explores how to understand and represent these associations through contingency tables, emphasizing the importance of order in ordinal variables. The example of smartphone ownership in relation to gender and income levels illustrates the process of summarizing data and creating cross-tabulations to analyze associations effectively.
Takeaways
- 📊 The script discusses the concept of association between variables, emphasizing that association does not imply causation.
- 🔍 It focuses on understanding associations between two numerical variables, particularly in the context of categorical and numerical variables.
- 📈 The module aims to teach how to interpret the association between two variables using plots and how to create a contingency table to analyze these associations.
- 👥 A market research example is used to explore whether there is an association between gender and smartphone ownership.
- 📝 Data is presented in a contingency table format, capturing gender and smartphone ownership among 100 college students.
- 👩🦳👦 Gender is captured as a nominal variable with two categories: male and female, while smartphone ownership is a binary categorical variable with 'yes' or 'no'.
- 🔢 The data summary reveals the count of male and female students, as well as the number who own smartphones and those who do not.
- 📉 The script explains how to create a pivot table in Google Sheets to analyze the association between gender and smartphone ownership.
- 📝 The importance of recording data accurately is highlighted, especially when dealing with categorical variables like gender and binary outcomes like smartphone ownership.
- 📚 The script also touches on ordinal variables, such as income levels categorized as low, medium, or high, and how they differ from nominal variables.
- 📋 The final takeaway is about the importance of maintaining the order in the contingency table when dealing with ordinal variables to accurately reflect the data's hierarchy.
Q & A
What is the main focus of the module discussed in the script?
-The main focus of the module is to understand the association between two categorical and numerical variables, specifically how to capture and analyze these associations.
What is the significance of understanding the association between variables?
-Understanding the association between variables is important for identifying patterns, making predictions, and drawing meaningful conclusions from data analysis.
What is the purpose of creating a contingency table in the context of this script?
-The purpose of creating a contingency table is to organize data in a way that makes it easier to analyze the relationship between two categorical variables, such as gender and smartphone ownership.
How is the data in the script collected and represented?
-The data is collected through a survey of 100 college students, capturing information about their gender and whether they own a smartphone, and is represented in a contingency table format.
What is the meaning of 'nominal variable' in the context of the script?
-In the script, a nominal variable refers to a categorical variable that can take on two or more values without any intrinsic order or ranking, such as gender.
How is the smartphone ownership variable captured in the script?
-Smartphone ownership is captured as a binary categorical variable with two values: 'yes' or 'no', indicating whether a student owns a smartphone.
What is the term used to describe a table that shows the relationship between two categorical variables?
-The term used to describe such a table is a 'contingency table', also commonly referred to as a 'cross-tabulation' or 'crosstab'.
What is the importance of considering the order in the contingency table when dealing with ordinal variables?
-When dealing with ordinal variables, considering the order is important because it reflects a ranking or sequence that can influence the analysis and interpretation of the data.
How does the script suggest to handle ordinal variables in a contingency table?
-The script suggests coding ordinal variables with numbers that represent their order, such as 1 for high income, 2 for medium income, and 3 for low income, to maintain the sequence in the contingency table.
What is the goal when analyzing the contingency table in the script?
-The goal is to determine whether there is an association between the categorical variables, such as whether gender is associated with smartphone ownership, and to understand the distribution of these associations across different categories.
How can one use the contingency table to explore the relationship between income and smartphone ownership?
-One can use the contingency table to explore the relationship by comparing the distribution of smartphone ownership across different income levels, thus analyzing if there is a correlation between income and the likelihood of owning a smartphone.
Outlines
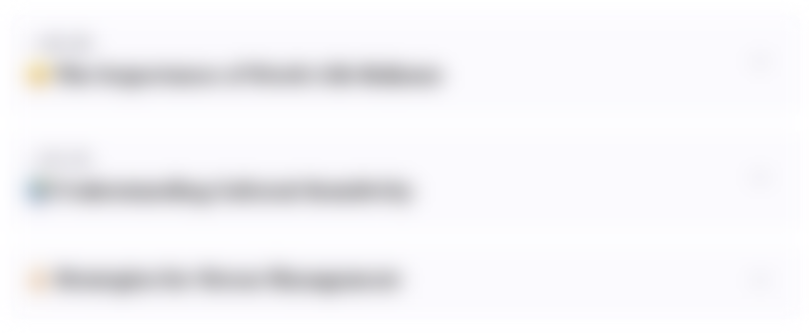
This section is available to paid users only. Please upgrade to access this part.
Upgrade NowMindmap
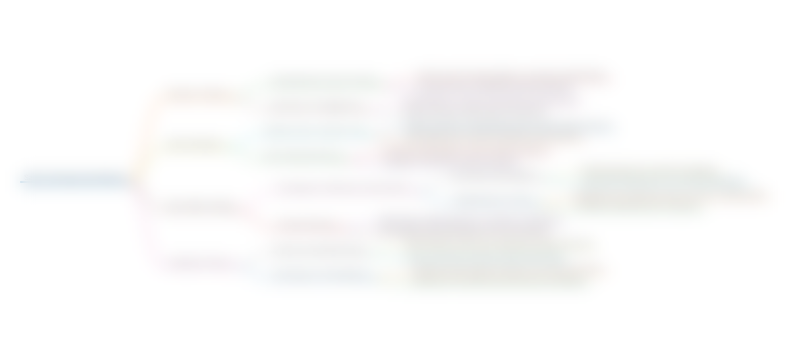
This section is available to paid users only. Please upgrade to access this part.
Upgrade NowKeywords
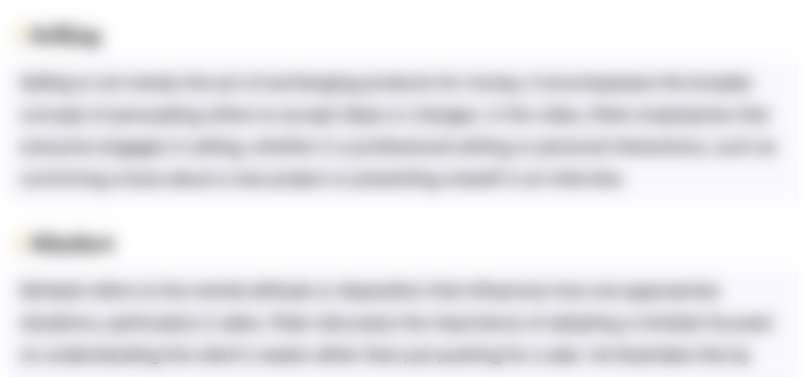
This section is available to paid users only. Please upgrade to access this part.
Upgrade NowHighlights
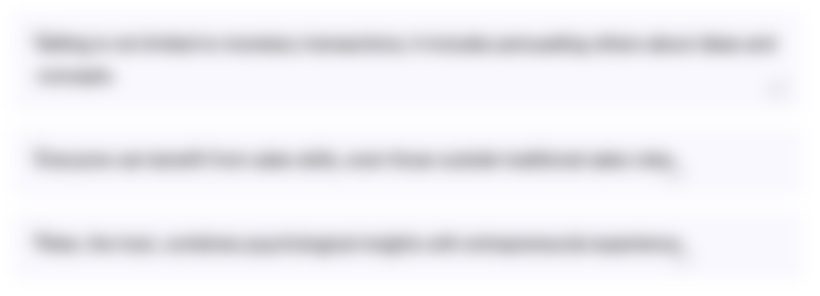
This section is available to paid users only. Please upgrade to access this part.
Upgrade NowTranscripts
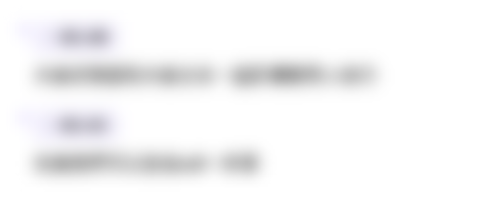
This section is available to paid users only. Please upgrade to access this part.
Upgrade NowBrowse More Related Video
5.0 / 5 (0 votes)