10 People + AI = Billion Dollar Company?
Summary
TLDRIn this episode of 'Light Cone,' hosts Gary, Jared Harge, and Diana debate the future of programming in the age of AI advancements. They discuss the impact of AI on job automation, the necessity for learning computer science, and the potential for AI to transform the way we build software. The conversation touches on the state of AI programmers, the importance of human creativity in coding, and the possibility of smaller teams creating billion-dollar companies. The hosts agree that despite AI's growing capabilities, the need for human ingenuity in programming remains crucial.
Takeaways
- ๐งโ๐ป The script discusses the evolving role of AI in programming and its implications for the tech industry, suggesting that AI may not fully replace the need for human programmers.
- ๐ It highlights the potential for AI to automate certain aspects of coding, particularly for junior developers, but acknowledges the limitations in creating complex systems.
- ๐ The conversation references the impact of AI advancements like GitHub Copilot and the sbench dataset, which have spurred interest in AI programming capabilities.
- ๐ The importance of learning computer science and coding is debated, with the argument that even if AI becomes advanced, understanding programming can enhance logical thinking and problem-solving skills.
- ๐ The script suggests that the future might see a rise in smaller teams or even solo founders capable of creating successful startups due to the democratizing effect of technology and AI.
- ๐ค The discussion touches on the idea that AI could change the nature of work, possibly reducing the need for large teams and allowing for more efficient, smaller companies.
- ๐ก It is suggested that AI and automation may lead to a shift in the types of jobs available, with a potential decrease in junior programming roles and an increase in demand for more senior or specialized skills.
- ๐ ๏ธ The script emphasizes that despite AI advancements, there is still a need for human creativity and craftsmanship in building products, particularly in the early stages of a startup.
- ๐ฑ The conversation speculates on the potential for AI to enable more people to pursue entrepreneurial ventures by reducing the barriers to entry in terms of technical skill and resource requirements.
- ๐ฎ The script concludes with a general agreement that learning to code is still valuable, as it provides a foundational understanding necessary for innovation and effective communication with AI tools.
- ๐ The participants express a collective hope for a future where AI contributes to a flourishing of entrepreneurship, allowing more individuals to realize their ideas and contribute to societal progress.
Q & A
What is the main topic discussed in the transcript?
-The main topic discussed is the impact of AI on programming and the future of software companies, particularly in relation to whether learning to code is still necessary.
What controversial statement did Jensen make that sparked discussion?
-Jensen stated that it is vital that children learn computer science and programming is almost exactly the opposite of what is necessary because computing technology should be advanced enough so that nobody has to program, making everyone in the world a programmer.
What are some companies mentioned that are working on AI programming tools?
-Companies mentioned include Sweep and Fume, which are developing coding assistance tools for developers.
What significant advancement in AI programming occurred about eight months ago?
-The significant advancement was the release of the benchmarking dataset called sbench by the Princeton NLP group, which includes real programming problems and has spurred interest and development in AI programming.
What historical dataset is compared to sbench in terms of its impact on AI development?
-sbench is compared to the ImageNet dataset, which was a groundbreaking dataset in deep learning and computer vision from Stanford's lab led by Fei-Fei Li.
What is one reason programming tasks are challenging for AI, according to the transcript?
-Programming tasks are challenging for AI because real-world problems are messy and complex, requiring not just programming skills but also the ability to understand and model the intricacies of real-world systems.
What argument does Jared make against Jensen's statement?
-Jared argues that even if AI can eventually build great apps from English descriptions, learning to code is still valuable because it makes people smarter and improves their logical thinking skills.
What is the Jevons Paradox and how does it relate to the discussion?
-The Jevons Paradox states that increasing the efficiency of a service leads to an increase in demand for that service. It is used to explain why the demand for programmers has not decreased despite advancements in programming tools.
What prediction is made about the future of unicorn companies?
-The prediction is that AI will lead to an increase in the number of unicorn companies started each year because it will be easier to get ideas off the ground and into prototype and initial user stages.
What is a key takeaway about the importance of learning to code?
-The key takeaway is that learning to code remains essential because it provides foundational knowledge and good taste needed to build great products, even in a future where AI might handle many programming tasks.
Outlines
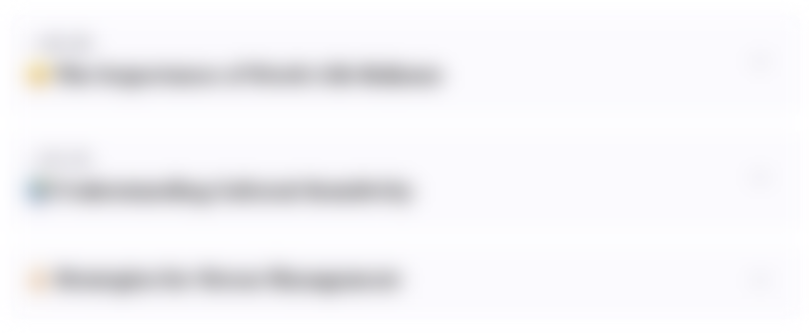
This section is available to paid users only. Please upgrade to access this part.
Upgrade NowMindmap
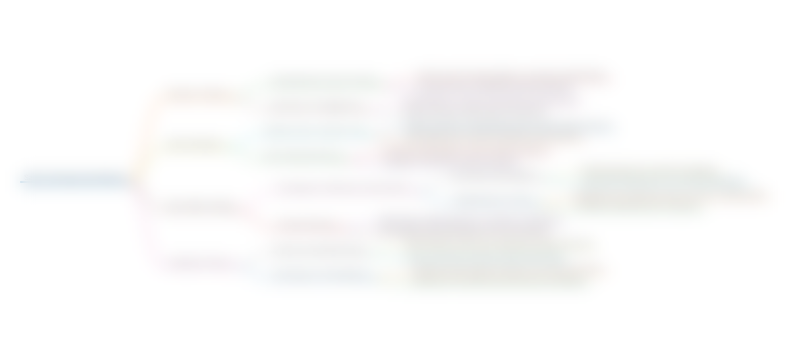
This section is available to paid users only. Please upgrade to access this part.
Upgrade NowKeywords
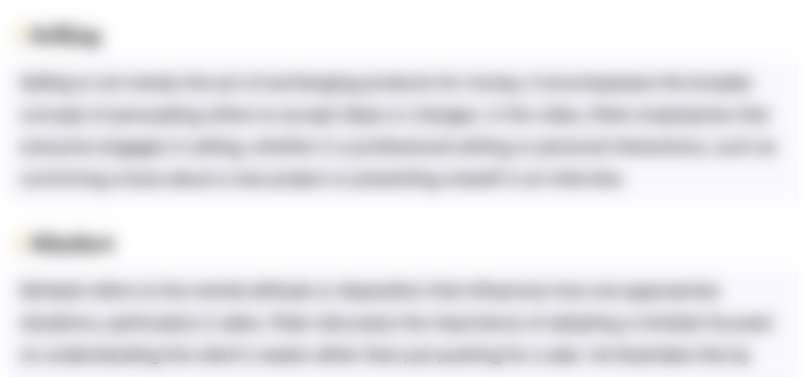
This section is available to paid users only. Please upgrade to access this part.
Upgrade NowHighlights
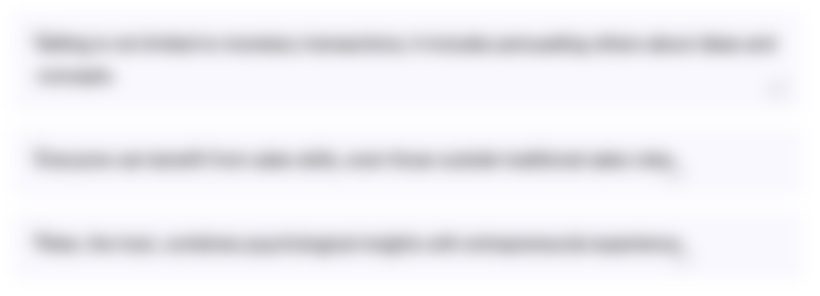
This section is available to paid users only. Please upgrade to access this part.
Upgrade NowTranscripts
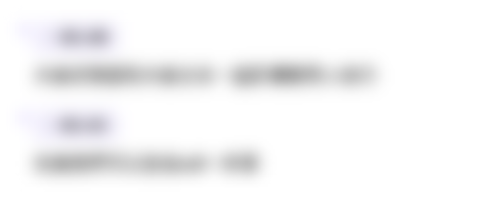
This section is available to paid users only. Please upgrade to access this part.
Upgrade Now5.0 / 5 (0 votes)