AI for Business: #3 Generative AI Use-cases
Summary
TLDRThe video script explores the rapid rise and diverse applications of generative AI, from writing articles and generating code to creating realistic images, videos, and music. It discusses the technology's economic impact, highlighting sectors like sales, marketing, and software engineering. The script delves into use cases like Microsoft's Office 365 co-pilot, marketing content creation, and data analysis, emphasizing the potential of generative AI in transforming business operations and everyday tasks through natural language interfaces and autonomous AI agents.
Takeaways
- π Chad GPT became the fastest-growing app, reaching 100 million users and showcasing the potential of generative AI in various fields.
- π Generative AI is predicted to contribute between $2.6 to $4.4 trillion annually, impacting 63 different use cases across various sectors.
- π‘ Large language models are capable of generating not just text, but also code, images, videos, 3D models, voice, and music, expanding creative and productive possibilities.
- π§ Tools like GitHub Copilot and Amazon CodeWhisperer are assisting software engineers in writing and debugging code more efficiently.
- π¨ AI tools are generating highly realistic images and videos from text inputs, with companies like Runway making strides in video generation.
- πΌ Music creation is also being revolutionized by AI, as demonstrated by tools like Stable Audio, which can produce original music compositions.
- π Generative AI is being used for knowledge retrieval, allowing employees to interact with enterprise data through natural language queries.
- π The economic impact of generative AI is substantial, with sectors like sales, marketing, software engineering, customer operations, and product R&D showing significant potential.
- π οΈ Microsoft's Office 365 co-pilot is an example of how AI can automate tasks in presentation creation, data analysis, and document writing.
- π Generative AI is streamlining marketing tasks, from crafting copy to generating social media content and personalized outreach, enhancing personalization and content richness.
- π’ Enterprise generative AI systems are integrating with proprietary company data to create smart chatbots and internal tools that can provide the latest information and insights.
Q & A
What milestone did Chad GPT achieve in January 2023?
-In January 2023, Chad GPT became the fastest-growing app of all time, reaching 100 million users just two months after its launch.
What are some capabilities of Chad GPT mentioned in the script?
-Chad GPT is capable of writing entire articles, generating software code, and aiding students in learning about virtually any topic.
What economic potential does generative AI hold according to the McKenzie report?
-Generative AI holds the potential to contribute between $2.6 to $4.4 trillion annually across 63 different use cases.
How are organizations leveraging large language models?
-Organizations use large language models to transform their business by automating customer operations, crafting marketing content, and assisting software engineers in writing code 56% faster.
What types of data can large language models generate beyond text?
-Large language models can generate highly realistic images, videos, 3D models, voice, music, and more.
Which tools assist software engineers with code generation?
-Tools like GitHub Copilot, Amazon CodeWhisperer, and Meta's Code Llama assist software engineers in writing software code, writing unit tests, and finding bugs.
What are some tools mentioned for generating realistic images from text inputs?
-Stable Diffusion, OpenAI's DALL-E, and Midjourney are mentioned as tools that can generate highly realistic and beautiful images from text inputs.
How is generative AI being used in the music industry?
-Tools like Stable Audio from Stability AI are used to produce music, demonstrating AI's capability to generate nice music from text inputs.
What is one significant use case of generative AI in the marketing domain?
-Generative AI is used to automate the creation of marketing content, such as crafting marketing copy, generating social media content, writing personalized outreach emails, and producing visual elements.
What are the two major ways of integrating generative AI with enterprise data?
-The two major ways are fine-tuning, which involves refining a pre-trained model on a specific dataset, and retrieval-augmented generation (RAG), which allows models to access up-to-date and verifiable knowledge sources.
Outlines
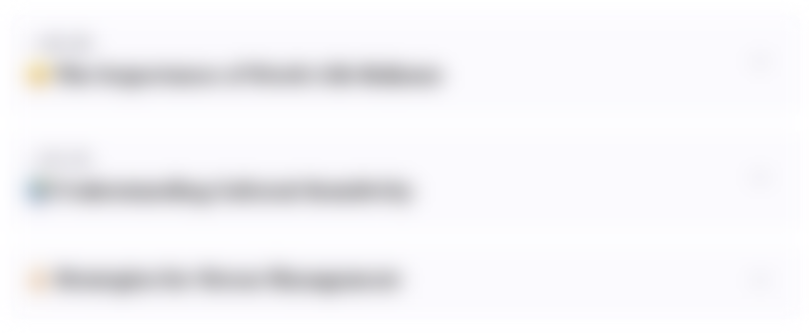
This section is available to paid users only. Please upgrade to access this part.
Upgrade NowMindmap
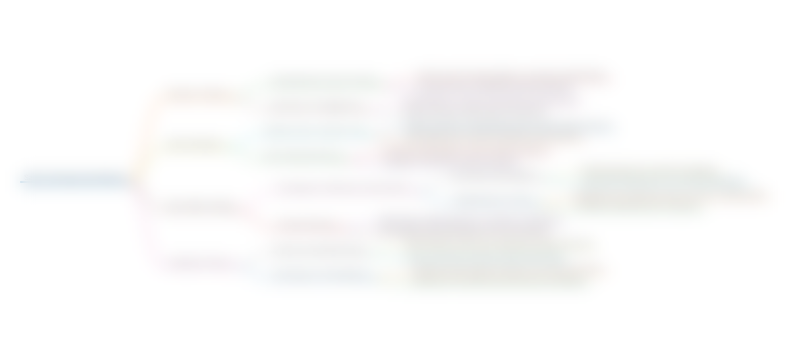
This section is available to paid users only. Please upgrade to access this part.
Upgrade NowKeywords
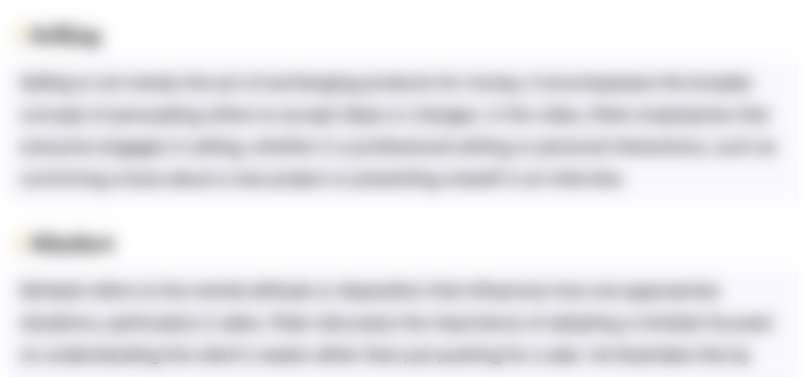
This section is available to paid users only. Please upgrade to access this part.
Upgrade NowHighlights
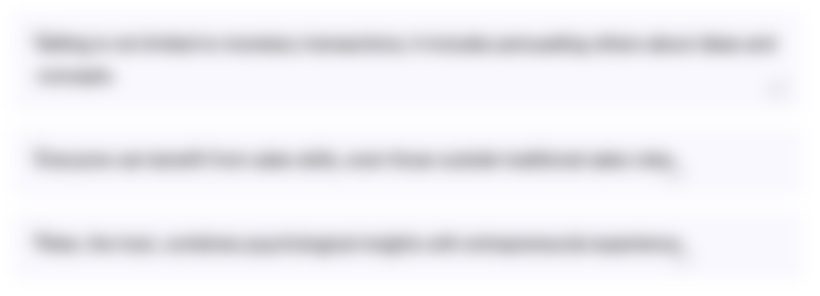
This section is available to paid users only. Please upgrade to access this part.
Upgrade NowTranscripts
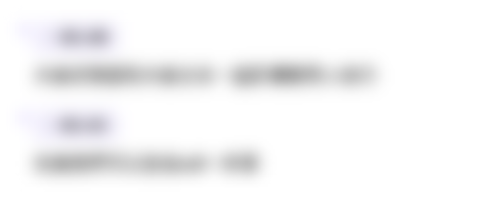
This section is available to paid users only. Please upgrade to access this part.
Upgrade NowBrowse More Related Video
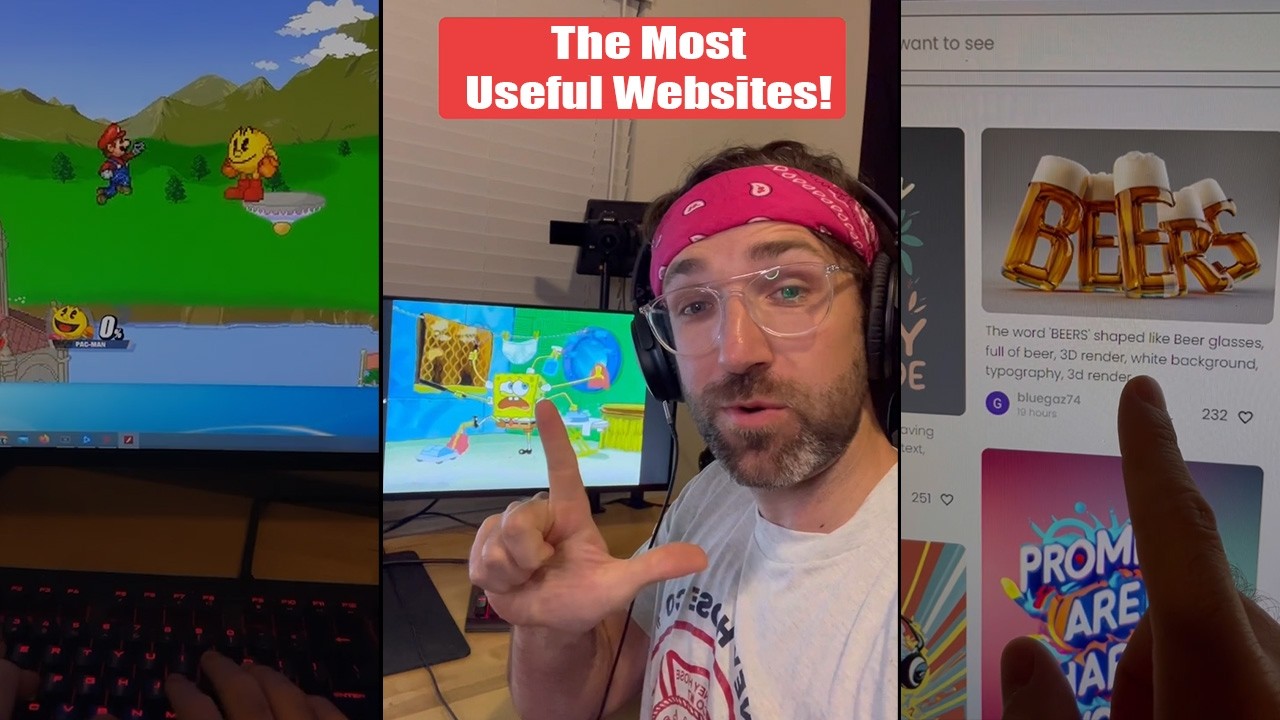
These Are The Most Useful Websites
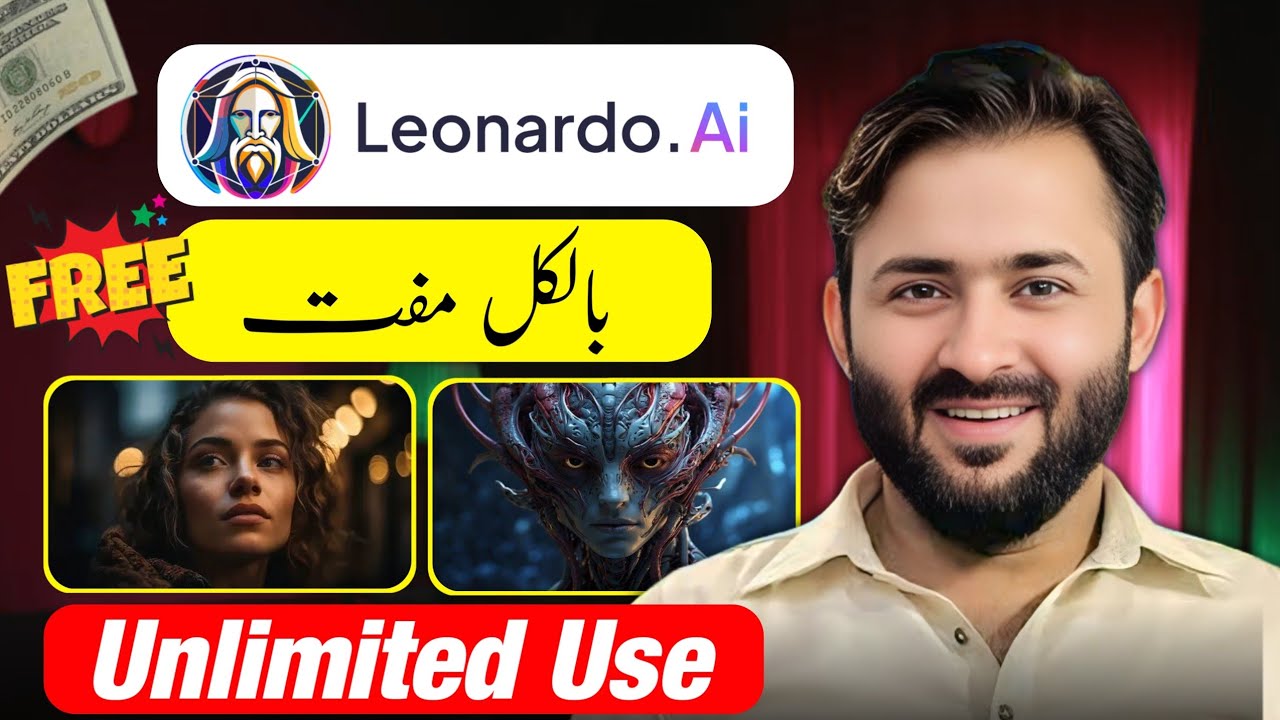
Can You Use Leonardo AI Unlimited? π€― Learn How to Create Limitless AI Images & Earn Money!
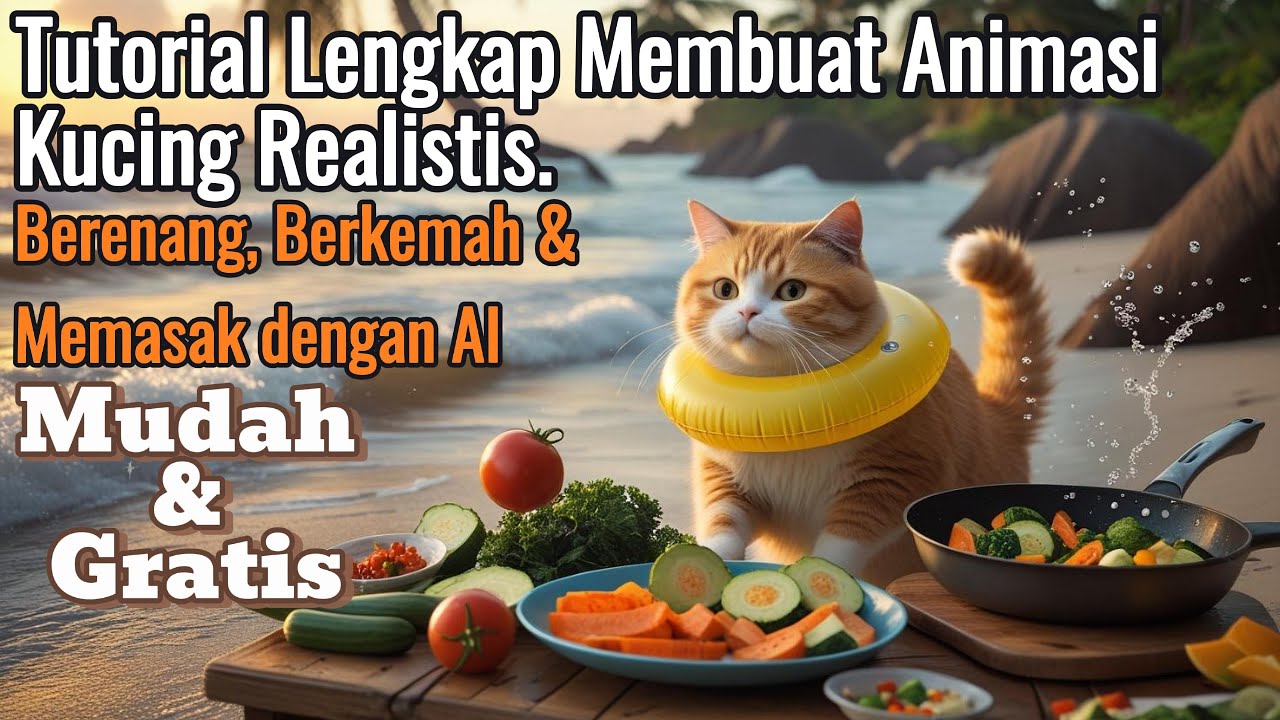
Tutorial Lengkap Membuat Animasi Kucing Berenang, Berkemah & Memasak dengan AI, Mudah Dan Gratis
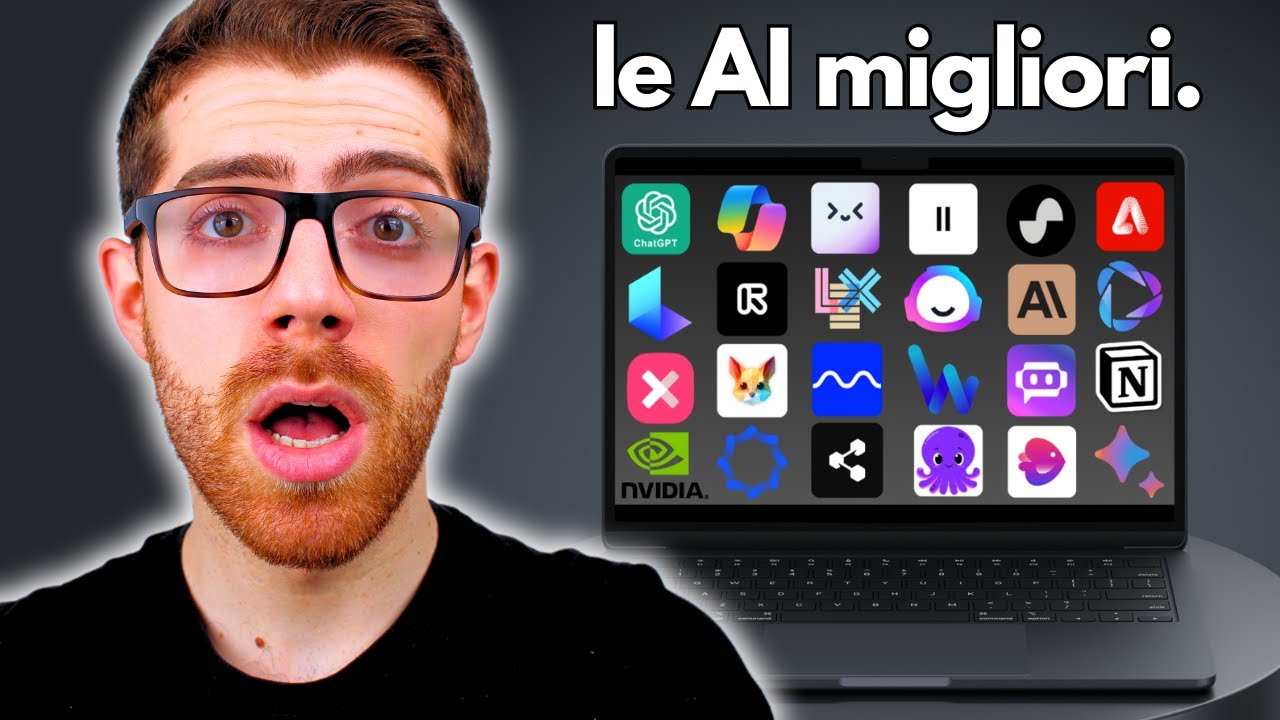
24 Intelligenze Artificiali PAZZESCHE da provare nel 2024
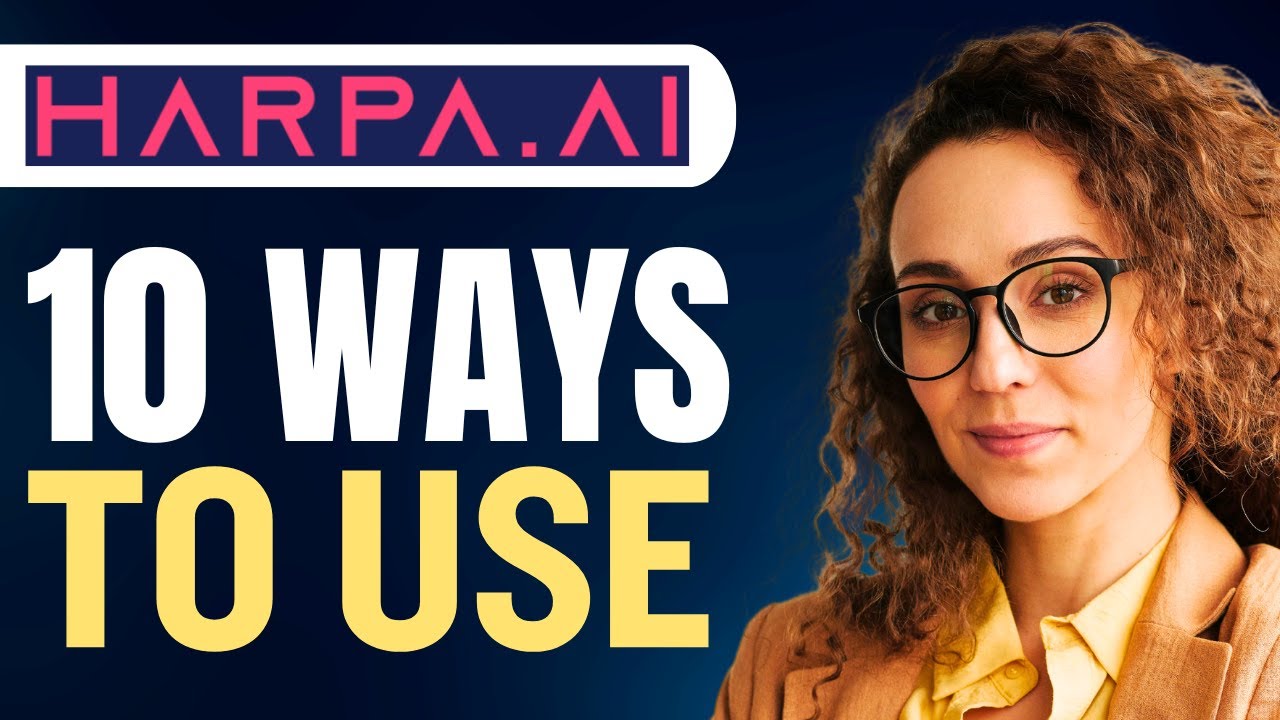
10 Ways to Use Harpa AI Tool (Harpa Ai Tool l Harpa AI Tutorial)

AI influencers are getting filthy rich... let's build one
5.0 / 5 (0 votes)