Open AI's Q* Is BACK! - Was AGI Just Solved?
Summary
TLDRこのビデオスクリプトでは、最近のAIコミュニティにおける興味深い動きを紹介しています。特に、大きな言語モデル(LLM)が数学問題を解決する能力が注目されています。研究によると、Googleがアルファゴを解決するために使用したモンテカルロ木探索技術を用いたLLMは、GSM数学ベンチマークで高い正解率を記録しました。また、OpenAIが数学問題を解決する能力を示したQARの話題も取り上げられており、AIの進化とその可能性が熱く語られています。
Takeaways
- 🧠 LLM(Large Language Models)は数学問題を解決する能力を示しており、これは技術的なマイルストーンとして注目されています。
- 📈 Googleがアルファ狗を解決するために使用したモンテカルロ木探索技術を用いた研究により、パラメータ数が1000倍少ないLLMでも高い数学問題解決率を達成できることがわかりました。
- 🔍 QAR(Quantum AI Research)は、AIコミュニティを騒然とさせ、Sam Almanが退職する直前に話題になりました。
- 🤖 GPT-0は、DeepMindのアルファゼロプログラムを模倣して、言語モデルが数学や科学の問題を解決する方法を模索する秘密プロジェクトでした。
- 🎯 Monte Carlo tree searchとbackpropagationを組み合わせた手法により、Llama 3はGSM-8K数学ベンチマークで高いスコアを記録しました。
- 🚀 QARは、見たことのない数学問題を解決する能力を示し、AIの安全性に関心を集めています。
- 🔑 Andrej Karpathyが語ったように、未来のモデルではモンテカルロ木探索のような手法が必要不可欠で、これはAIの改善に役立ちます。
- 📚 AlphaGoは、人間を超えるために自己改善を通じて学習し、これはLLMにとっても重要なステップです。
- 🛠️ コンピュートのコストが、LLMと検索を組み合わせた手法の普及を阻む主な障害物となっており、これは最適化の必要性を示しています。
- 🌐 Arc AGIは、新しい基準として提唱されており、システムがAGI(人工一般知能)を達成するかどうかを評価するための重要な指標となっています。
- 🔮 将来のモデルでは、視覚やコーディング、長いコンテキストの理解を改善することで、Arc AGIのベンチマークを超える可能性が示唆されています。
Q & A
QARとは何ですか?また、なぜAIコミュニティに注目されていますか?
-QARは数学問題を未見olvedのものを解決できるという重要な技術的マイルストーンを持っており、AIコミュニティに注目される要因の一つです。QARはSam Almanが退職する直前にOpenAIで突破を果たしたとされる情報記事の話題となりました。
最近の研究で、大きな言語モデルが数学にどのように役立つか説明してください。
-最近の研究では、大きな言語モデルがMonte Carlo tree searchとback propagationを用いて数学問題を解決することができ、Llama 8 billion parametersでは96.7%の正解率を記録しました。これは、パラメータ数が200倍少ないにもかかわらず、GPT 4 ClaudeやGeminiよりも優れていることを意味します。
GPT 0プロジェクトとは何で、なぜ秘密裏に行われたのですか?
-GPT 0は、言語モデルが数学や科学の問題を含む推論タスクを解決できるようにするため、OpenAIが2021年に開始した秘密プロジェクトです。Alpha Zeroプログラムにちなんでおり、チェスや囲碁をプレイするプログラムとして開発されました。
Monte Carlo tree searchとは何であり、どのようにAIに適用される可能性があると述べていますか?
-Monte Carlo tree searchは、Alpha Goで使用されたアルゴリズムで、AIシステムがすべての可能な構成を探索し、次に最も良い手を決定するものです。この手法は、言語モデルに適用され、数学問題の解決に役立つとされています。
Andrej Karpathyが言語モデルの未来について何を述べていますか?
-Andrej Karpathyは、言語モデルの未来においてはMonte Carlo tree searchのような手法が必要であると述べています。彼は1時間の講演で、これらのモデルが改善されるために必要なものであると語っています。
Alpha Goの成功の鍵は何でしたか?
-Alpha Goの成功の鍵は、自己改善とMonte Carlo tree searchでした。Alpha Goは人間エキスパートプレイヤーを模倣することから始まりましたが、後には自己改善を通じて超人的なレベルに達しました。
現在の言語モデルが直面している課題は何ですか?
-現在の言語モデルは、基本的な視覚、コーディング能力、長いコンテキストの扱いについて課題に直面しています。これらの制限を克服することは、AIの性能を向上させる上で重要なステップとなります。
ARC AIとは何であり、なぜ重要なのですか?
-ARC AIは、視覚推論のベンチマークであり、新しいルールを推測する必要があります。重要なのは、AIが新しい状況に適応し、基本的な知識のみを使って問題を解決できることを証明できるためです。
GPT-4の現在の限界はどこにありますか?
-GPT-4は、グリッドの視覚理解、コーディングでの基本的な誤り、長いコンテキストの使用において限界があります。これらの限界を超えることが、AIの性能向上に寄与すると予想されています。
AIがARC AIベンチマークを超えるために必要なものは何ですか?
-AIがARC AIベンチマークを超えるためには、基本的な視覚理解の向上、コーディング能力の向上、長いコンテキストの扱い方改善などが必要です。また、より多くのサンプルを用いた学習も重要な要素です。
Outlines
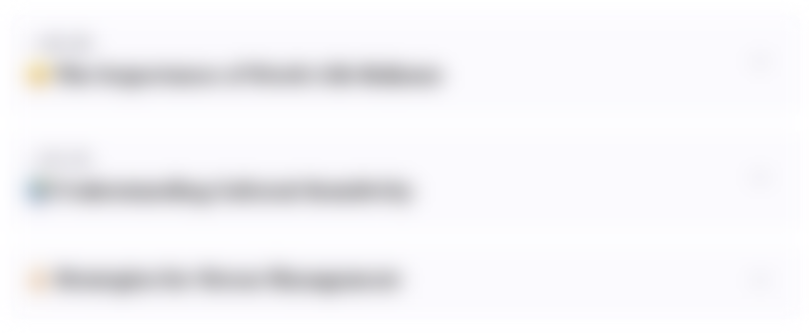
This section is available to paid users only. Please upgrade to access this part.
Upgrade NowMindmap
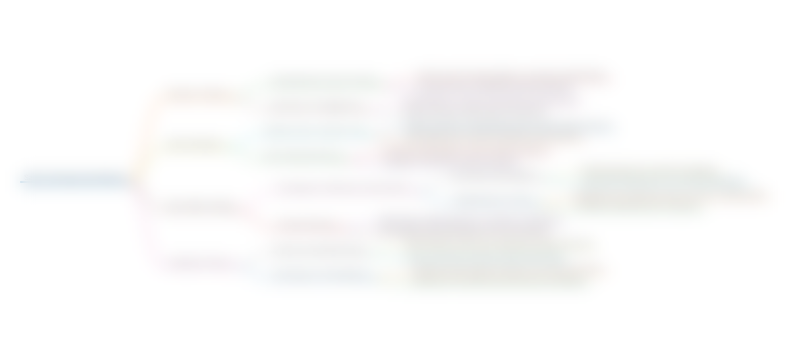
This section is available to paid users only. Please upgrade to access this part.
Upgrade NowKeywords
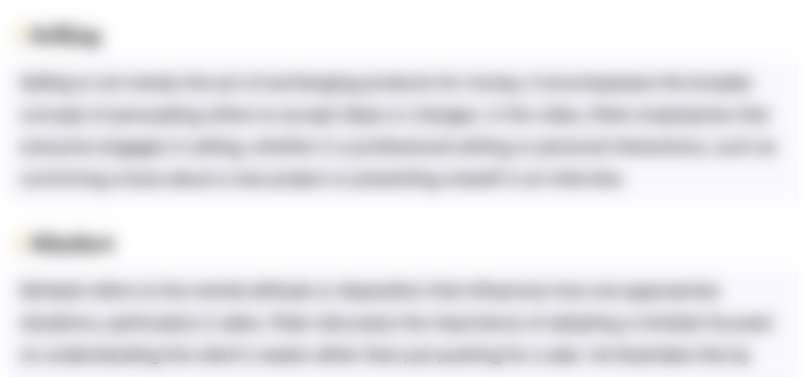
This section is available to paid users only. Please upgrade to access this part.
Upgrade NowHighlights
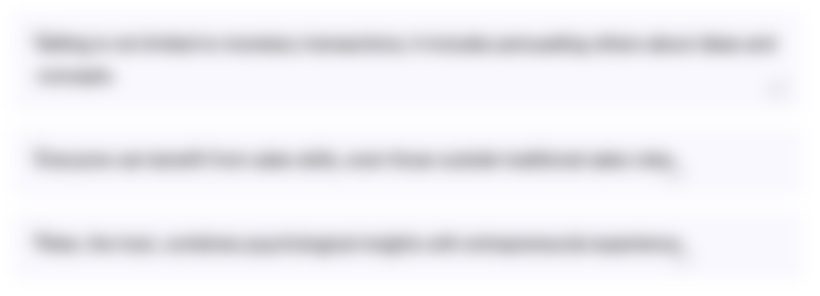
This section is available to paid users only. Please upgrade to access this part.
Upgrade NowTranscripts
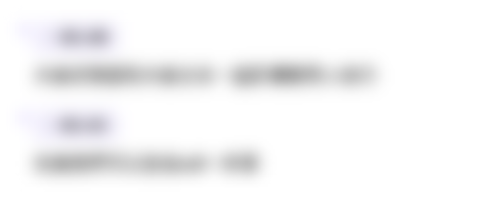
This section is available to paid users only. Please upgrade to access this part.
Upgrade NowBrowse More Related Video
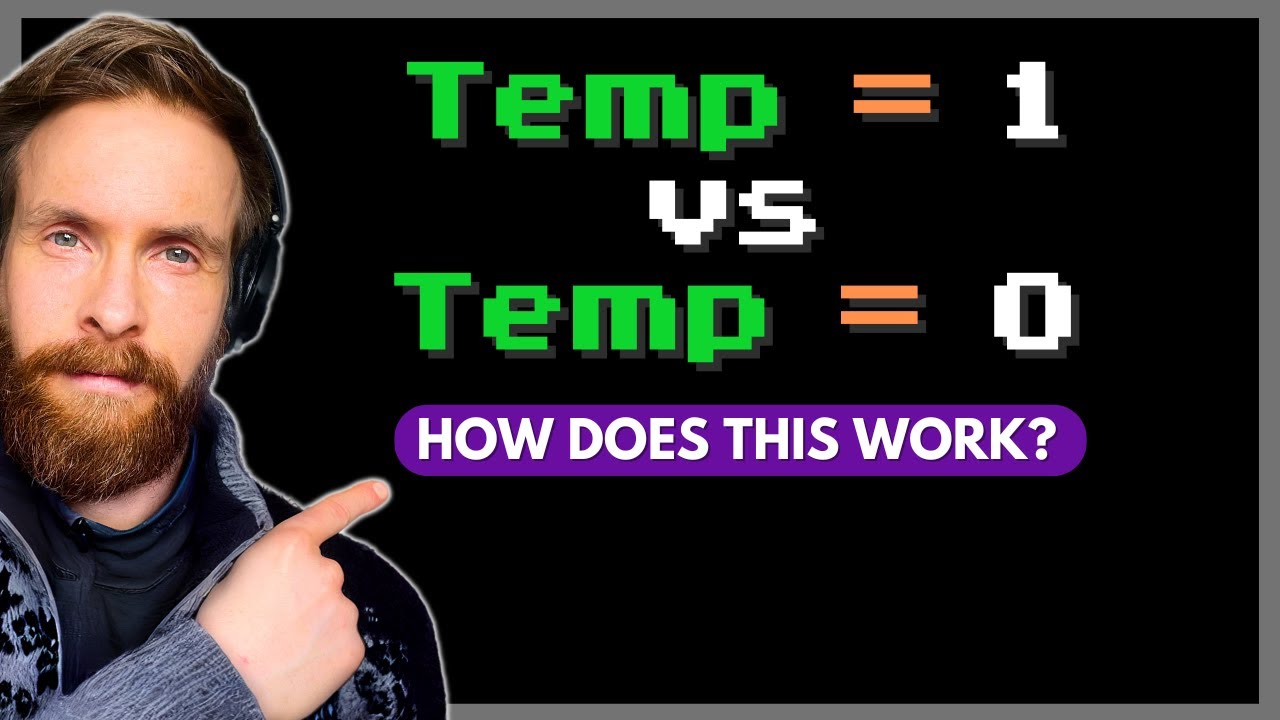
Determinism in the AI Tech Stack (LLMs): Temperature, Seeds, and Tools
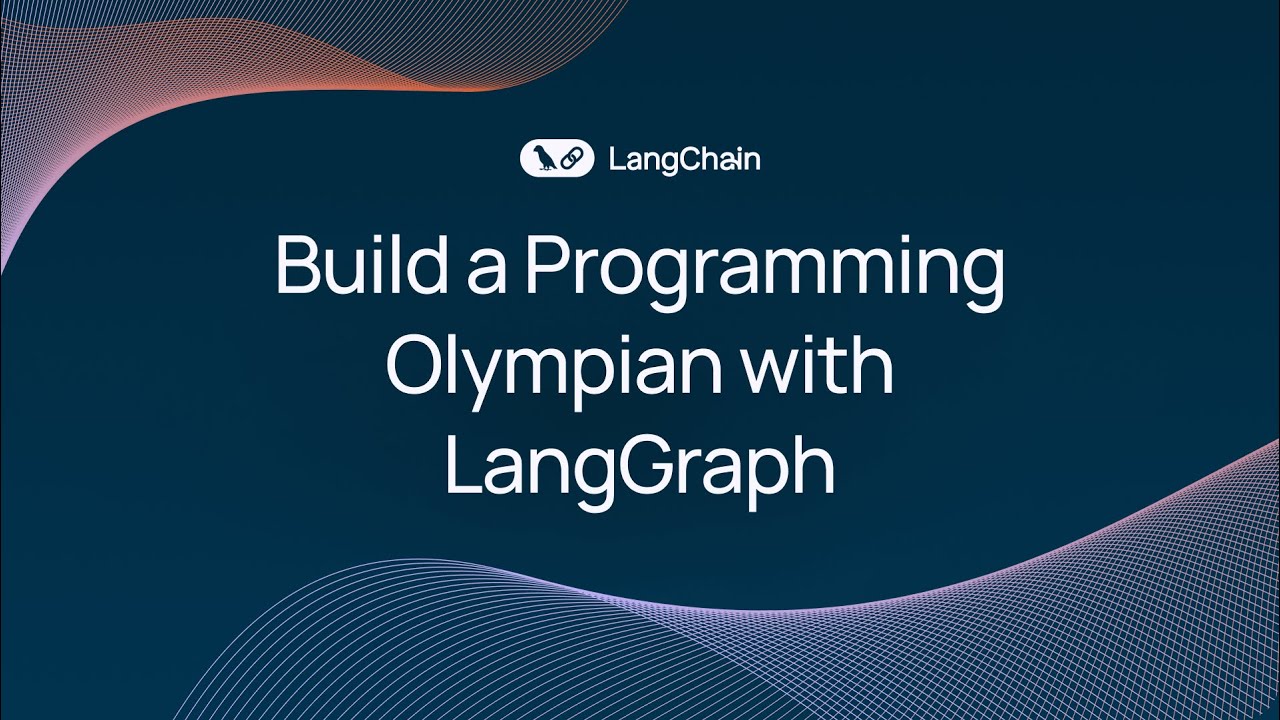
Build Computing Olympiad Agents with LangGraph

これさえ押さえておけばOK! 生成AI時流を解説 〜4月前半の生成AIトレンドをご紹介〜(2024/04/17)
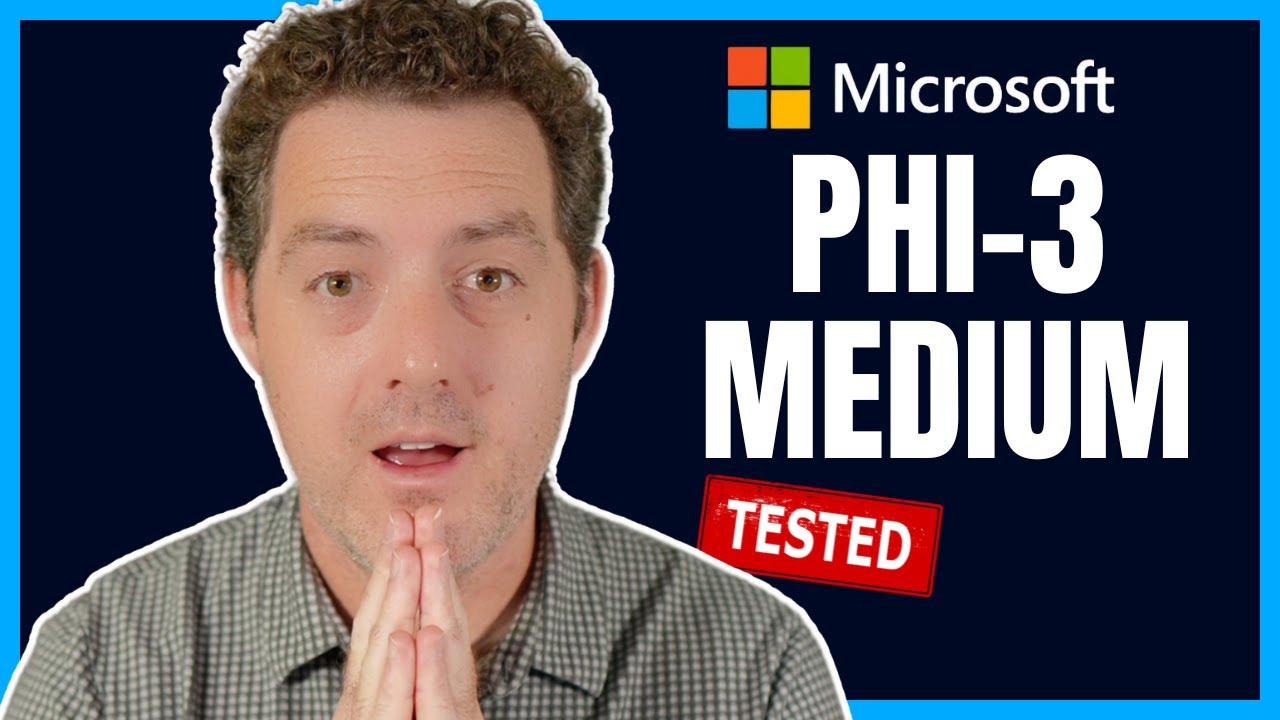
Phi-3 Medium - Microsoft's Open-Source Model is Ready For Action!
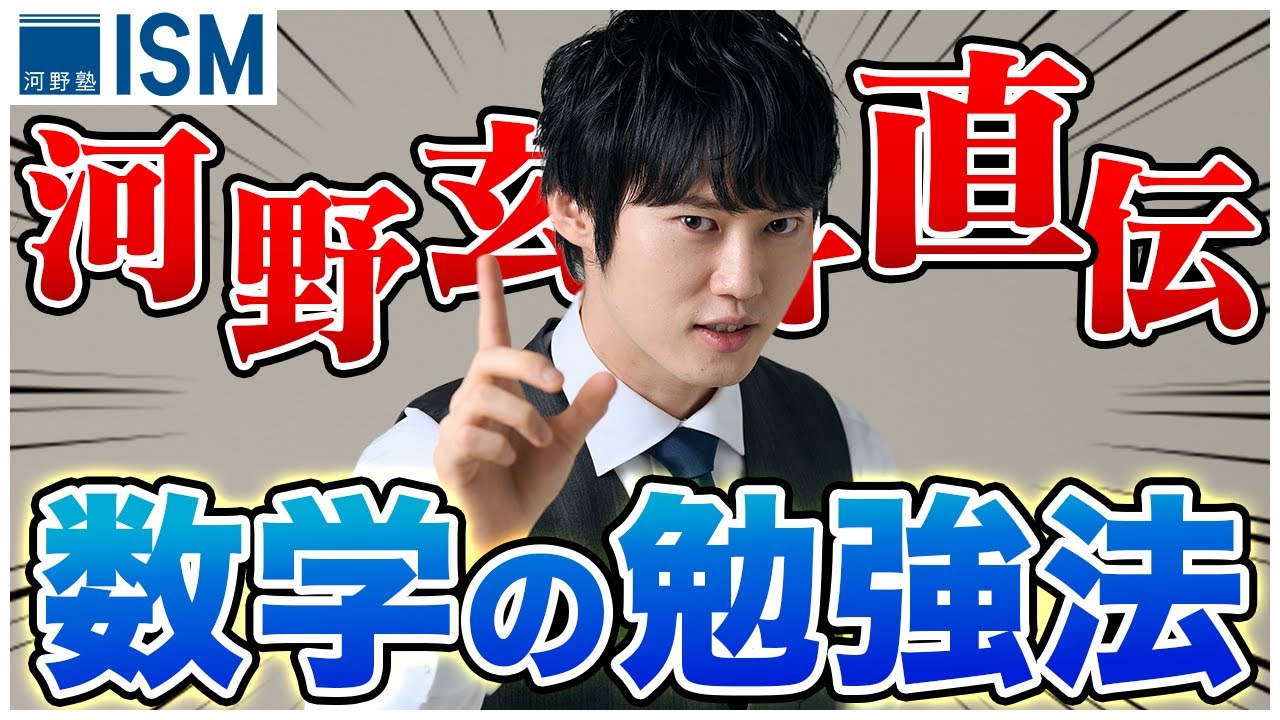
誰でも数学で無双できます。【数学の勉強法】

Did OpenAI Just Secretly Release GPT-5?! ("GPT2-Chatbot")
5.0 / 5 (0 votes)