Sampling: Simple Random, Convenience, systematic, cluster, stratified - Statistics Help
Summary
TLDRThis script introduces five common sampling methods for population studies: simple random sampling, convenience sampling, systematic sampling, cluster sampling, and stratified sampling. It emphasizes the importance of unbiased and representative samples, acknowledges the inevitability of sampling error, and discusses the practicality and potential biases of each method. The goal is to choose a sampling method that balances accuracy with available resources, and to account for any known bias in analysis.
Takeaways
- 📌 A sample is a subset of a population selected for study, aiming to represent the whole group's characteristics.
- 🔍 The ideal sample should be unbiased, meaning every member of the population has an equal chance of being selected.
- 🍏 In practical scenarios, such as measuring the size of apples in an orchard, it's not feasible to measure every single apple, hence sampling is necessary.
- 💰 The choice of sampling method depends on the nature of the population and the available resources like time and money.
- 🔄 There will always be some sampling error due to the fact that only a part of the population is being studied.
- 🎯 Simple random sampling is the theoretical ideal, providing an unbiased sample, but it can be impractical and costly, especially with human populations.
- 🏢 Convenience sampling is quick and easy but often biased, and may suffer from self-selection bias when participants have a vested interest.
- 🌐 Systematic sampling involves selecting members at regular intervals, which is easier to administer but can be affected by patterns in the population.
- 📚 Cluster sampling involves dividing the population into groups and randomly selecting groups for the sample, which can be practical but may introduce bias if groups differ significantly.
- 📊 Stratified sampling divides the population into subgroups with specific characteristics and takes random samples from each, potentially providing a highly representative sample but requires detailed information about the population.
- 🛠️ Different sampling methods have their advantages and disadvantages, and the choice should be based on the best result achievable with the available resources.
- ⚖️ If a sample is known to have bias, this should be considered during analysis and reporting to ensure accurate representation.
Q & A
What is the primary purpose of taking a sample from a population?
-The primary purpose of taking a sample is to gather information about a population of interest when it is impractical or impossible to measure or observe the entire population.
Why is it important for a sample to be unbiased?
-An unbiased sample is important because it ensures that every object in the population has an equal chance of being selected, which helps in obtaining a representative sample and reducing sampling error.
What is a sampling frame and why is it necessary for simple random sampling?
-A sampling frame is a complete list of all the individuals or objects within the population of interest. It is necessary for simple random sampling to ensure that every member of the population has an equal chance of being selected.
What are the potential issues with convenience sampling?
-Convenience sampling can be biased because it selects individuals or objects based on their availability or accessibility rather than randomly, which may not accurately represent the entire population.
How does systematic sampling differ from simple random sampling?
-Systematic sampling involves selecting a starting point randomly and then choosing every nth object in a list or sequence, making it easier to administer than simple random sampling but potentially susceptible to bias if there is a pattern in the population.
What is cluster sampling and how does it work?
-Cluster sampling involves dividing the population into clusters and then randomly selecting some of these clusters. All members within the chosen clusters are included in the sample, which can be more practical than simple random sampling but may lead to bias if clusters are not representative of the population.
How does stratified sampling aim to improve the representativeness of a sample?
-Stratified sampling divides the population into subgroups or strata based on specific characteristics and then takes a random sample from each stratum. This method can produce a very good representative sample by ensuring that each subgroup is proportionally represented.
What is the potential drawback of stratified sampling compared to other methods?
-Stratified sampling can be complex to administer and requires a sampling frame with detailed information about the population. It may also be challenging to define the strata in a way that accurately represents the population.
Why is it inevitable to have some sampling error when taking a sample?
-Sampling error is inevitable because a sample only includes a part of the population. Even with the best sampling methods, there will always be some variation between the sample and the entire population.
How should known bias in a sample be addressed in the analysis and reporting of results?
-Known bias in a sample should be acknowledged and taken into account during the analysis and reporting process. This may involve adjusting the results or interpreting them with caution to reflect the potential impact of the bias.
What should be considered when choosing a sampling method for a study?
-When choosing a sampling method, one should consider the nature of the population, the resources available in terms of time and money, the need for representativeness, and the potential for bias. The goal is to select a method that provides the best results given the available resources.
Outlines
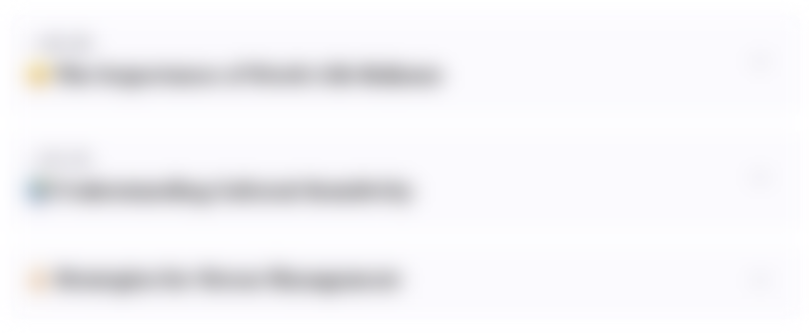
This section is available to paid users only. Please upgrade to access this part.
Upgrade NowMindmap
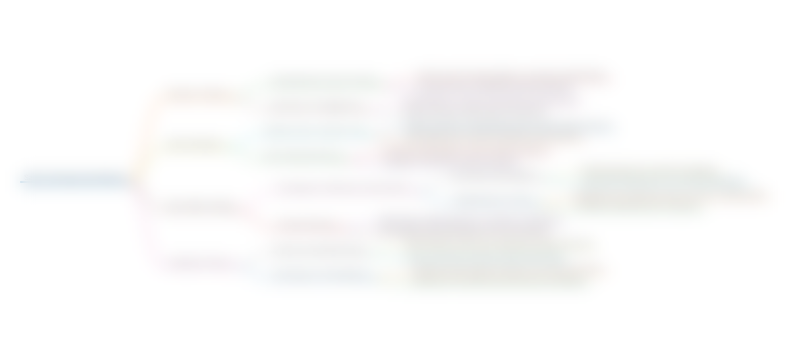
This section is available to paid users only. Please upgrade to access this part.
Upgrade NowKeywords
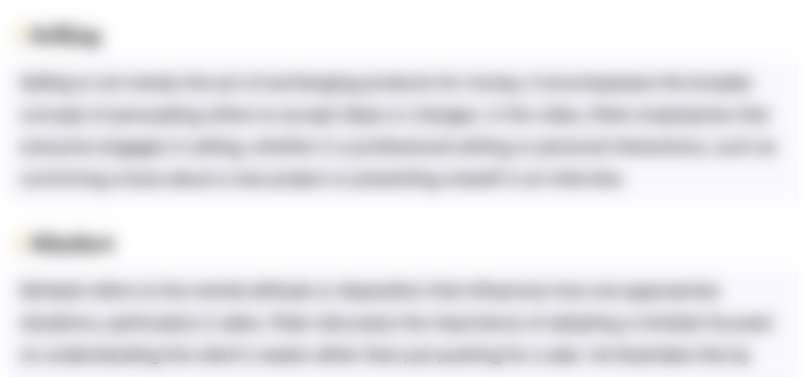
This section is available to paid users only. Please upgrade to access this part.
Upgrade NowHighlights
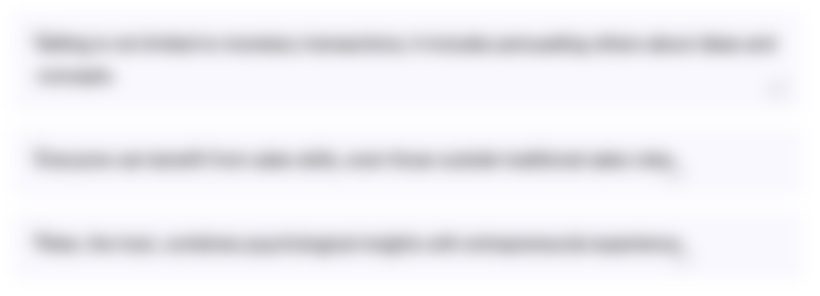
This section is available to paid users only. Please upgrade to access this part.
Upgrade NowTranscripts
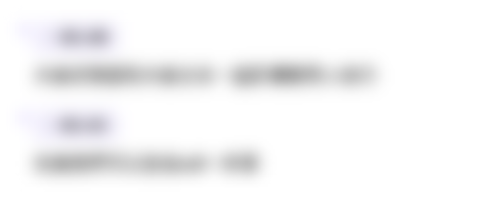
This section is available to paid users only. Please upgrade to access this part.
Upgrade NowBrowse More Related Video

Research Methods 1: Sampling Techniques
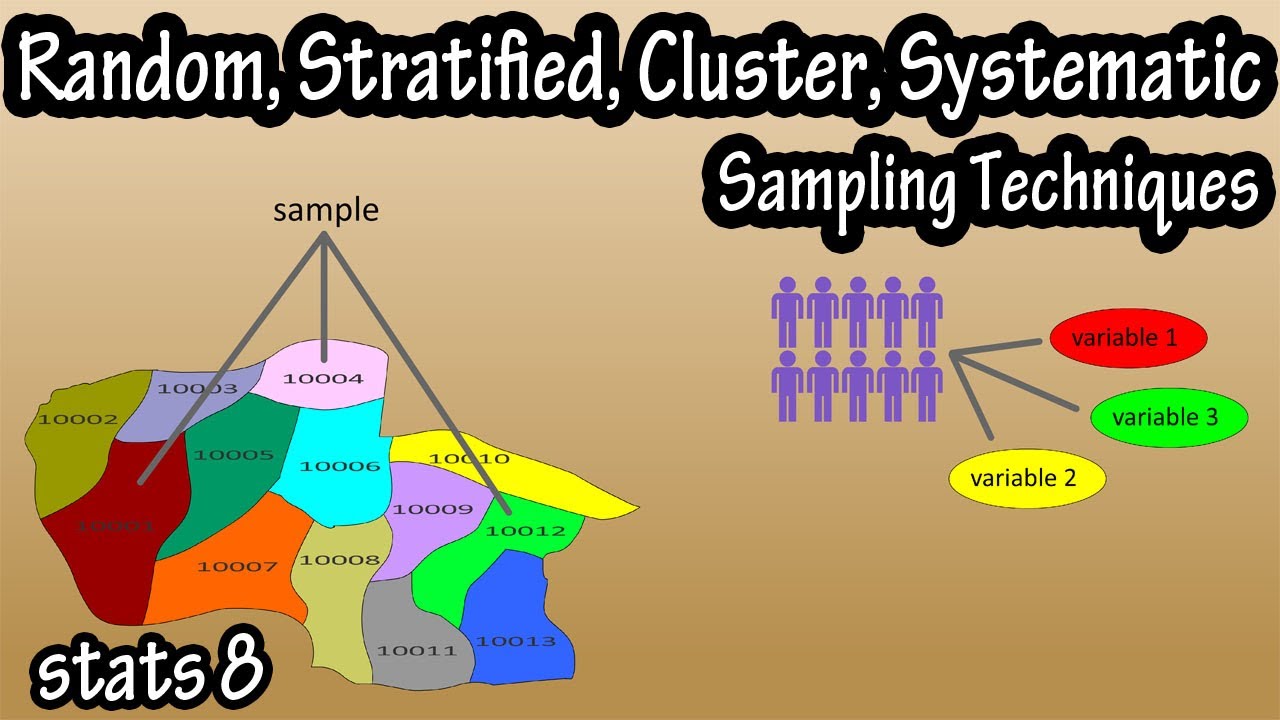
What Are The Types Of Sampling Techniques In Statistics - Random, Stratified, Cluster, Systematic
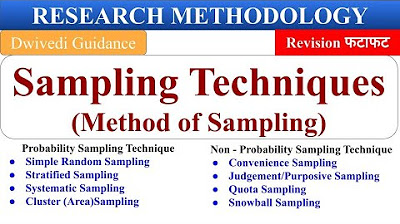
sampling techniques, types of sampling, probability & non probability sampling, Research methodology
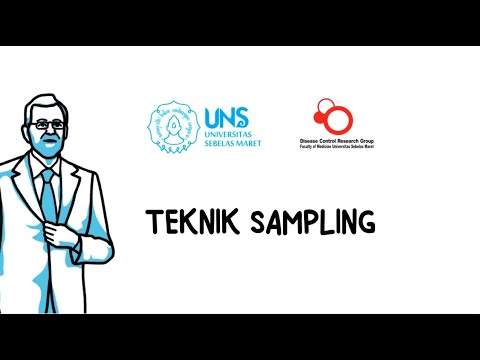
Teknik Sampling

Kuliah Statistika Industri | Teknik Sampling
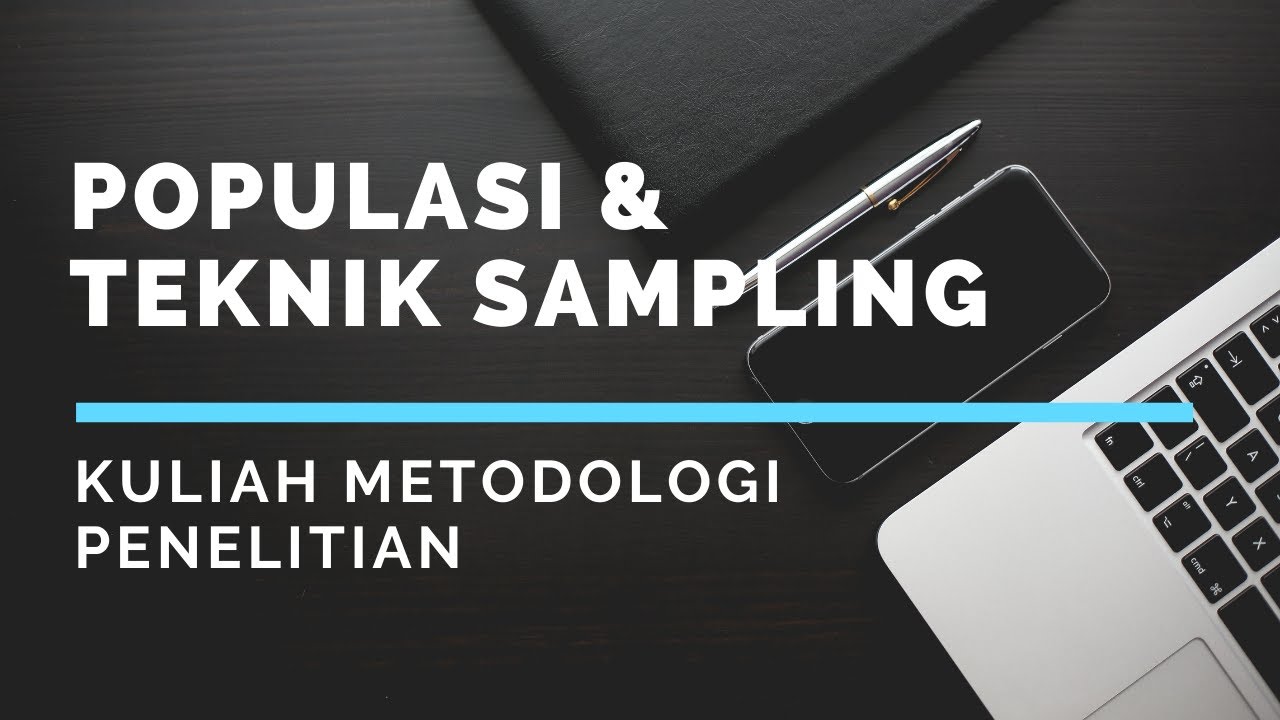
KULIAH METODE PENELITIAN (5) - POPULASI DAN TEKNIK SAMPLING
5.0 / 5 (0 votes)