Unlocking Value with AI | WSO2Con USA 2024
Summary
TLDRThe speaker emphasizes that the value of AI lies not in its complexity but in its practical applications to create tangible outcomes. They recount a story from a fishing company to illustrate the importance of showing positive results and discuss various use cases where AI enhances products, customer support, and operational efficiency. The talk also addresses the challenges of AI implementation, advocating for a clear understanding of the problem, the availability of simpler solutions, and the need for ongoing monitoring and risk management.
Takeaways
- ๐ The importance of applying technology to create value rather than just using it for the sake of it was emphasized, illustrated by a fishing company's data science team focusing on showing charts that went up.
- ๐ AI's value is not inherent but depends on how it's applied to create outcomes that matter, rather than the complexity of the technology itself.
- ๐ A real-world example of AI's value was provided in the apparel manufacturing industry, where image processing technology helped improve quality control significantly.
- ๐๏ธ AI can be used to make products or websites seamless by anticipating user needs and personalizing experiences, enhancing user satisfaction.
- ๐ฌ AI has shown success in customer support, with LLM-based solutions handling a large portion of queries and improving efficiency.
- ๐ AI can simulate physical systems for analysis and troubleshooting, as demonstrated by NASA's use of physical models to solve problems during space missions.
- ๐ In retail, AI can be used to optimize store layouts and checkout processes, improving customer experience and operational efficiency.
- ๐ AI can remove friction from user experiences, such as with biometric authentication, making interactions more natural and less intrusive.
- ๐ฎ When considering AI, it's crucial to first determine if the problem can be solved without it, as simpler solutions are often more cost-effective.
- ๐ A checklist for applying AI models was discussed, including verifying the value, determining if AI is necessary, assessing data availability and legality, and planning for implementation and monitoring.
- ๐ The speaker highlighted the risks and challenges of AI, such as the potential for biased predictions and the need for careful management to avoid unintended consequences.
Q & A
What was the fishing company's approach to data science and insights?
-The fishing company's manager instructed the data science team to only show charts that went up, indicating a positive trend. The team tried hard to find such insights, eventually discovering a chart that showed the weight of fish increasing with age, which was a positive trend they could report.
What is the key takeaway from the fishing company's story about applying technology?
-The key takeaway is that not every application of technology creates value. It's important to focus on how technology is applied and the value it creates rather than just the effort or investment put into the technology itself.
What is the speaker's main focus for the discussion on AI?
-The speaker's main focus is not on how great AI is or how it will evolve, but rather on how to create value with AI. They aim to discuss practical use cases and the importance of applying AI effectively.
Can you provide an example of a successful AI application in the apparel manufacturing industry?
-An example of a successful AI application is in the quality control of apparel manufacturing, where image processing technology is used to check if garments are made in the correct proportions. This reduces the risk of mistakes in quality control and helps factories meet the standards of top brands.
What is the concept of digital transformation as discussed in the script?
-Digital transformation refers to the idea of using technology to make a significant difference in various aspects of a business. It includes using AI in various use cases to improve products, services, and operational efficiency.
What are some examples of AI applications in improving customer support?
-AI can be used to handle a significant portion of customer queries through LLM-based solutions, improving the efficiency of internal support teams, or providing direct assistance to users. It can also be used for churn prediction to align with business efforts to retain customers.
How does the speaker describe the use of AI in creating a seamless user experience?
-The speaker describes using AI to make products or websites seamless by anticipating user needs, guiding them, and even performing actions on their behalf without requiring explicit input, thus creating a smooth and intuitive user experience.
What is the importance of verifying the effectiveness of an AI model?
-Verifying the effectiveness of an AI model is crucial to ensure it is solving the intended problem and providing value. It involves checking if the model works with the available data, understanding the implications of its predictions, and managing potential risks.
What is the speaker's advice when considering an AI use case?
-The speaker advises to first consider if there is a simpler solution that can achieve the desired outcome without AI, as AI can be expensive and complex. If AI is necessary, it's important to clarify the value, verify its effectiveness, and manage the risks associated with its application.
What is the significance of the toothpaste manufacturing company story in the context of AI application?
-The toothpaste manufacturing company story illustrates the importance of checking if an AI solution is actually needed and if it's working as intended. It highlights the potential for simpler, more effective solutions and the need to verify the impact of AI implementations.
How does the speaker suggest managing the risks associated with AI applications?
-The speaker suggests managing risks by understanding the AI model's predictions, setting guardrails for its operation, and being vigilant about its performance. It's also important to consider the potential for models to be gamed and to monitor for unintended consequences.
Outlines
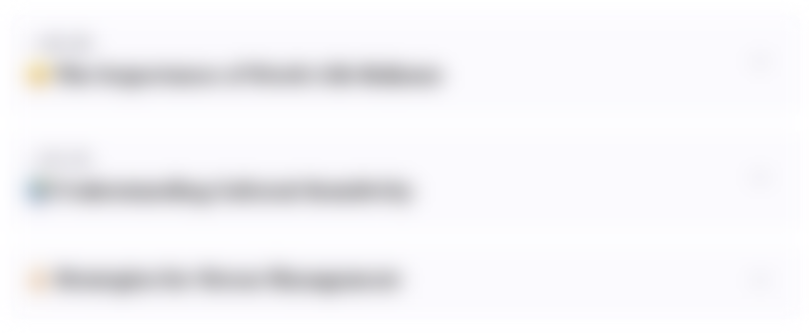
This section is available to paid users only. Please upgrade to access this part.
Upgrade NowMindmap
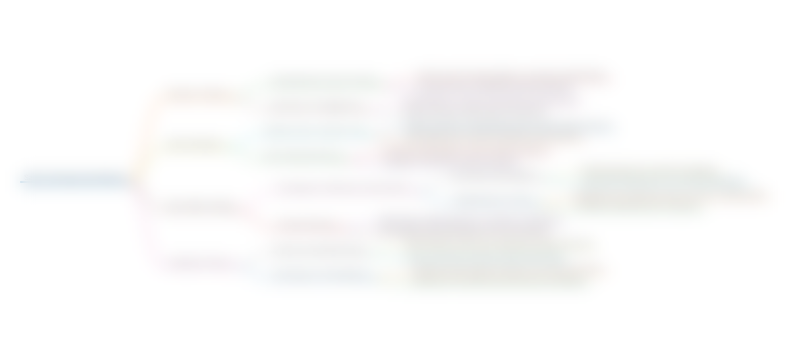
This section is available to paid users only. Please upgrade to access this part.
Upgrade NowKeywords
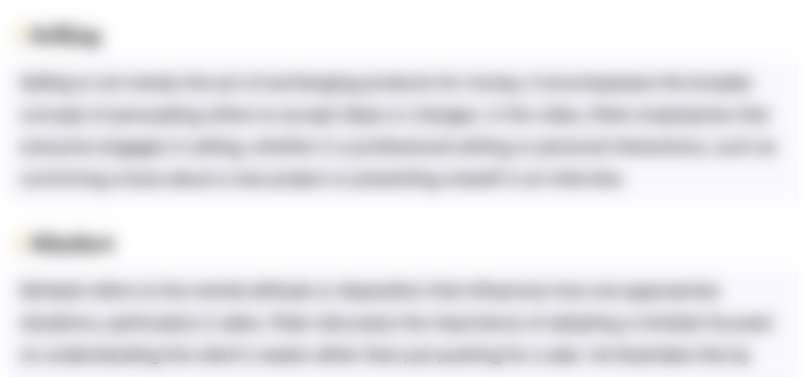
This section is available to paid users only. Please upgrade to access this part.
Upgrade NowHighlights
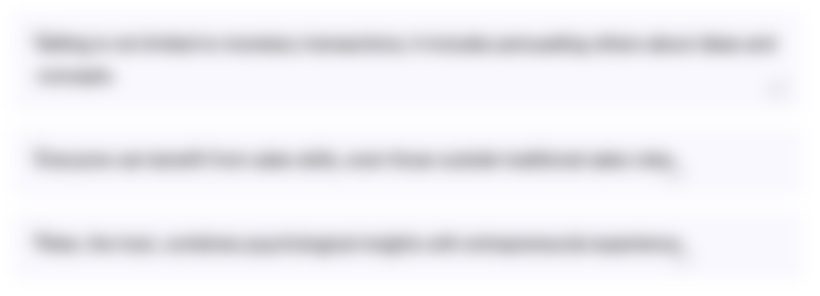
This section is available to paid users only. Please upgrade to access this part.
Upgrade NowTranscripts
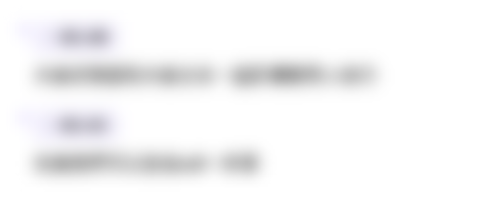
This section is available to paid users only. Please upgrade to access this part.
Upgrade NowBrowse More Related Video
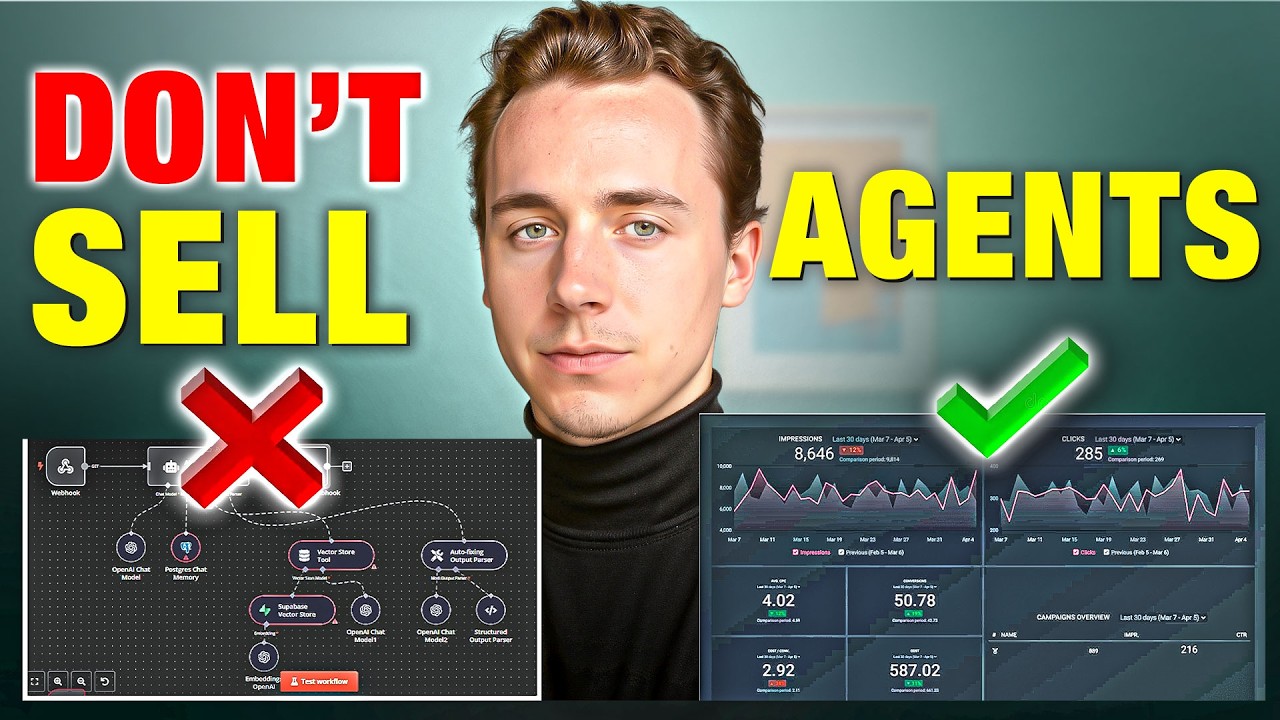
Don't Sell N8N Workflows, Sell AI Infrastructure - Here's How (Massive Opportunity)
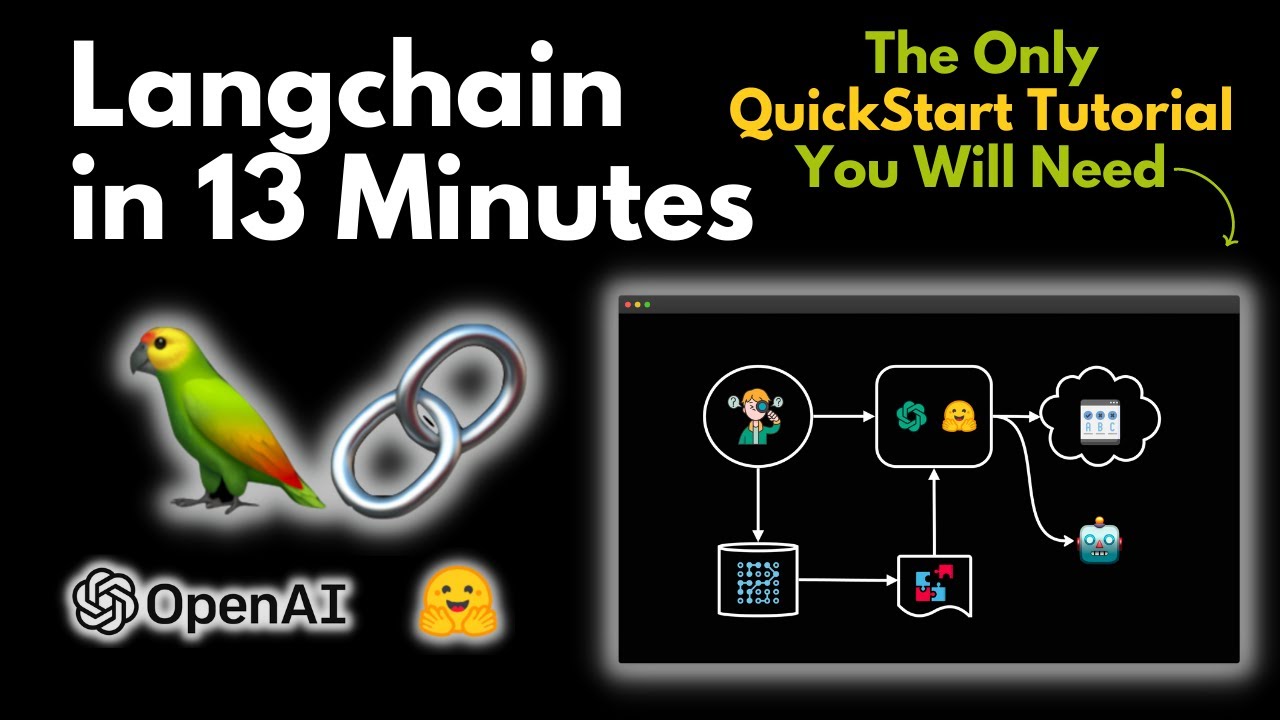
LangChain Explained in 13 Minutes | QuickStart Tutorial for Beginners

AI || ARTIFICIAL INTELIGENCE DALAM PENDIDIKAN

'Too many MBAs ruining companies,' Elon Musk explains.
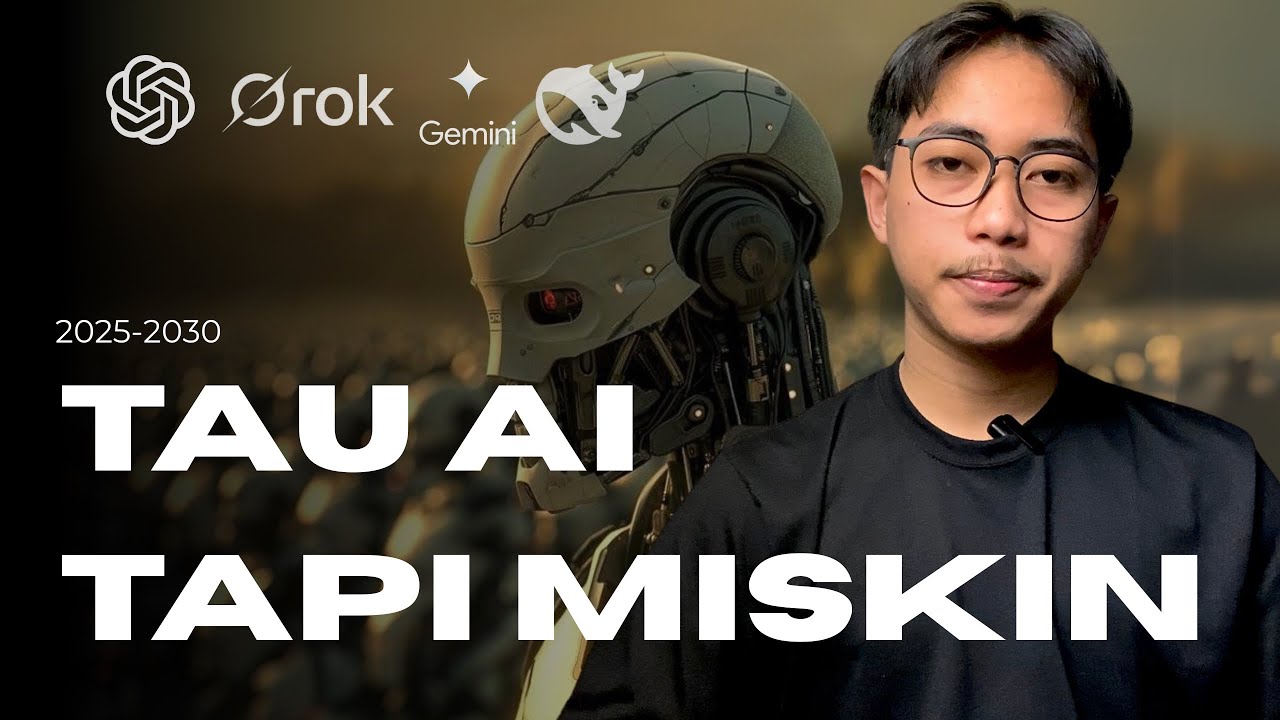
CARA KAYA DI INDUSTRI AI (2025-2030)
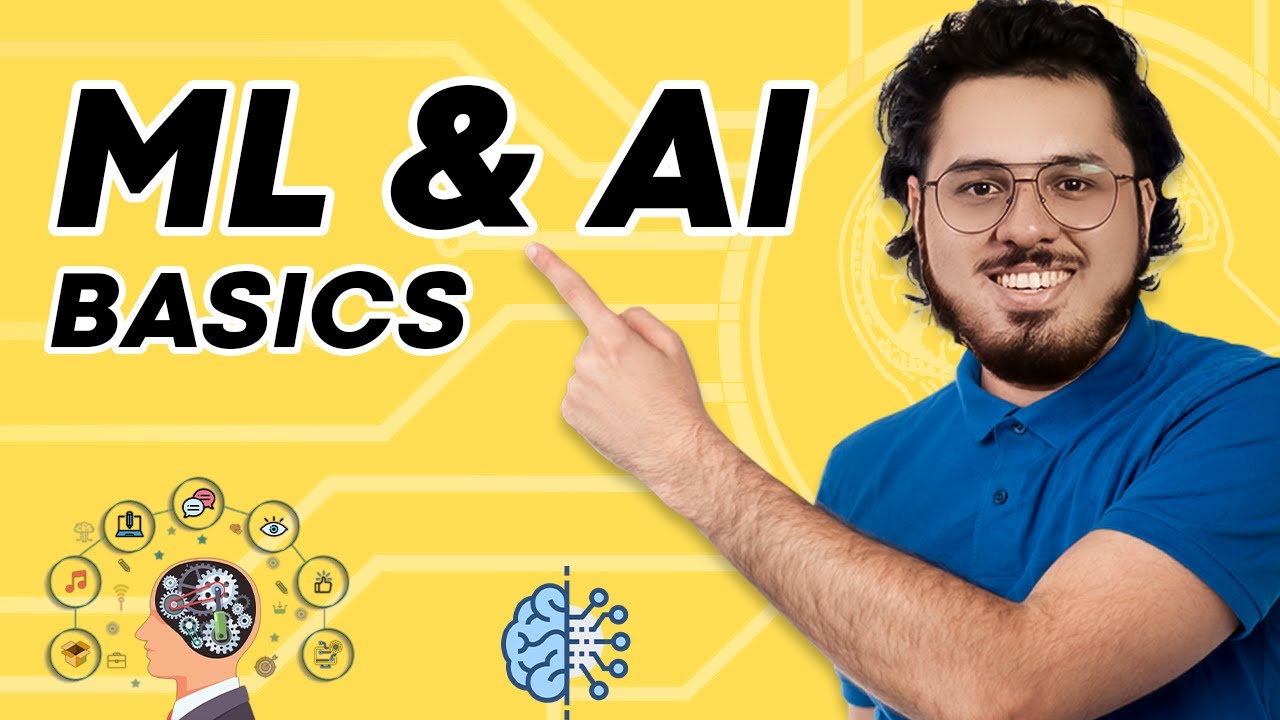
All About Machine Learning & Deep Learning in 2024 ๐ฅ
5.0 / 5 (0 votes)