ARTIFICIAL INTELLIGENCE IN CREDIT ANALYSIS: CHALLENGES AND PROSPECTS - GROWTHPAL
Summary
TLDRThis video explores the role of AI in financial credit analysis, highlighting its benefits such as improved accuracy, faster processing, better risk assessment, and customized lending solutions. AI helps financial institutions make informed decisions while reducing operational costs. However, the video also addresses challenges, including limited data availability, lack of transparency, biases, the 'black box' problem, and the need for human judgment in some cases. The key takeaway is that while AI can enhance credit analysis, financial institutions must address its limitations to ensure fairness and accuracy in lending decisions.
Takeaways
- 😀 AI is enhancing credit analysis by improving the accuracy of financial assessments.
- 😀 AI can analyze large datasets, leading to more accurate creditworthiness evaluations.
- 😀 Automation through AI speeds up the loan processing, improving the customer experience.
- 😀 AI helps financial institutions identify hidden patterns in data for better risk assessment.
- 😀 Customized lending solutions can be created by analyzing individual borrower behavior using AI.
- 😀 Cost savings are achieved by automating processes and reducing the need for manual intervention.
- 😀 One challenge of AI in credit analysis is limited or inaccurate data availability.
- 😀 Lack of transparency in AI algorithms can make it difficult for borrowers to understand credit decisions.
- 😀 AI algorithms may replicate human biases, leading to discrimination against certain groups of borrowers.
- 😀 The 'black box' nature of some AI models makes it hard to explain credit decisions to regulatory authorities.
- 😀 While AI excels in processing data, human judgment is still crucial for making certain lending decisions.
Q & A
What is financial credit analysis?
-Financial credit analysis is the process of assessing the creditworthiness of a borrower by evaluating their financial situation and determining their ability to repay a loan. It involves analyzing factors like income, assets, liabilities, credit history, and other relevant financial data.
How does AI improve the accuracy of credit analysis?
-AI improves the accuracy of credit analysis by using algorithms that can process vast amounts of data, extracting meaningful insights to lead to more accurate credit assessments. This enables financial institutions to make better-informed lending decisions.
What role does AI play in speeding up credit application processing?
-AI speeds up the credit application process by automating various steps, which reduces the time required to evaluate credit applications. This enables financial institutions to process loans faster, improving the overall customer experience.
How does AI help in risk assessment in credit analysis?
-AI helps in risk assessment by identifying patterns and trends in data that are not easily noticeable to humans. This allows financial institutions to better assess potential risks of default and take proactive actions to mitigate these risks.
How can AI provide customized lending solutions?
-AI can analyze individual borrower behavior and offer customized lending solutions tailored to the specific needs of each borrower. This personalization leads to better customer satisfaction and more appropriate loan offerings.
In what ways can AI reduce operational costs for financial institutions?
-AI reduces operational costs by automating several processes and minimizing the need for manual intervention. This allows financial institutions to offer more competitive interest rates while maintaining profitability.
What is the challenge of limited data availability in AI-powered credit analysis?
-AI algorithms require large volumes of data to function effectively. However, if financial institutions do not have access to sufficient or accurate data, this can lead to biased or incorrect credit assessments, affecting the reliability of AI in credit analysis.
What is the lack of transparency in AI credit analysis algorithms?
-The lack of transparency occurs because AI algorithms can be highly complex and difficult to interpret. This can result in borrowers not understanding why their loan applications were accepted or rejected, reducing trust in the AI-driven decision-making process.
How can AI algorithms exhibit biases and discrimination in credit assessments?
-AI algorithms may replicate human biases if the training data they use is biased. For instance, if the data reflects discrimination against certain groups, the AI could unjustly favor or disadvantage certain demographics during credit assessments.
What is the Black Box problem in AI credit analysis?
-The Black Box problem refers to the opaqueness of AI algorithms, meaning it is often difficult to understand how they arrive at certain decisions. This lack of clarity can make it hard to explain credit assessments to borrowers or regulatory authorities.
Outlines
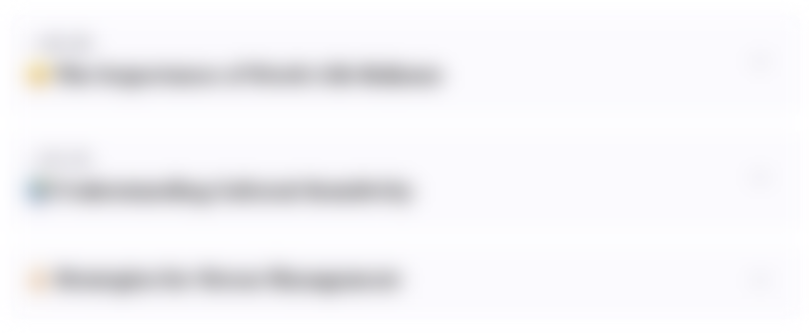
This section is available to paid users only. Please upgrade to access this part.
Upgrade NowMindmap
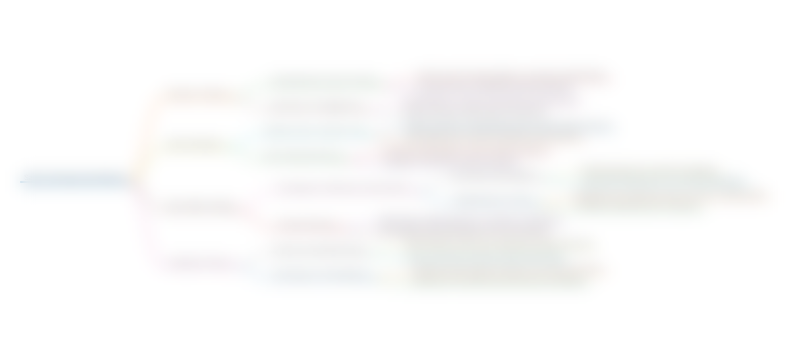
This section is available to paid users only. Please upgrade to access this part.
Upgrade NowKeywords
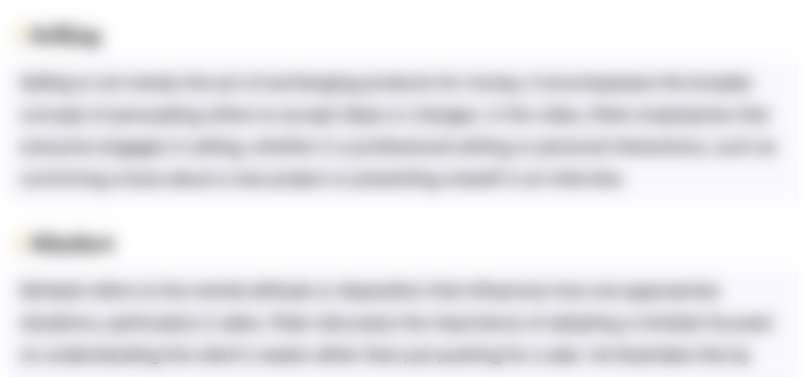
This section is available to paid users only. Please upgrade to access this part.
Upgrade NowHighlights
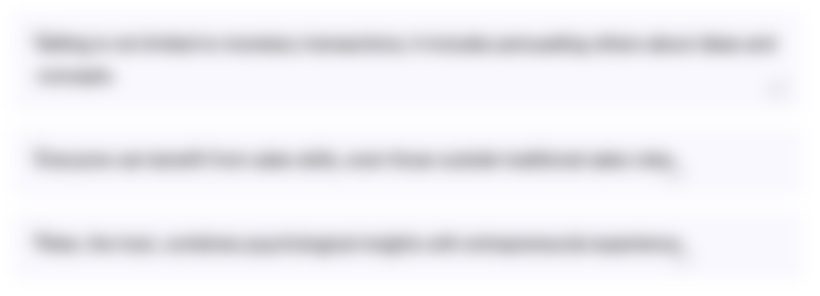
This section is available to paid users only. Please upgrade to access this part.
Upgrade NowTranscripts
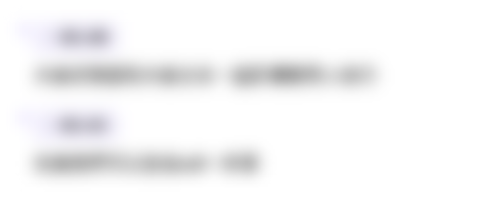
This section is available to paid users only. Please upgrade to access this part.
Upgrade NowBrowse More Related Video
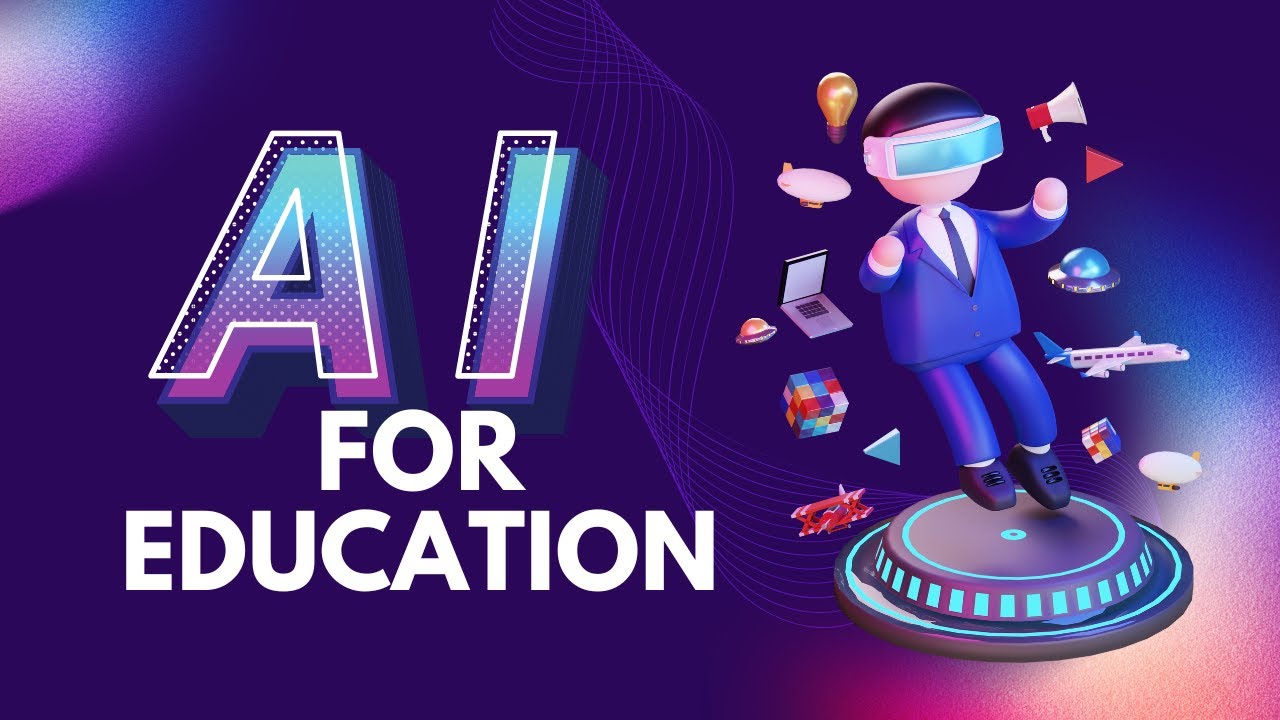
AI In Education: Personalized Learning And Intelligent Tutoring Systems
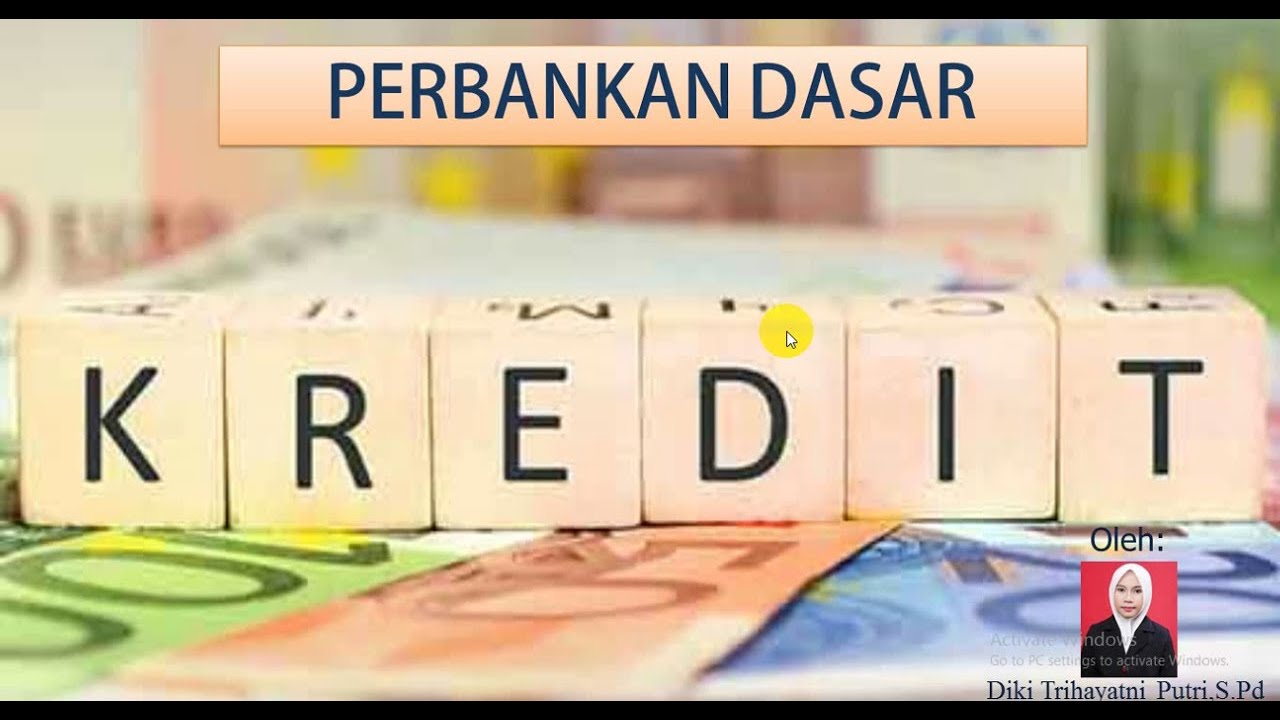
Perbankan Dasar Kelas X SMK | Kredit Perbankan 1
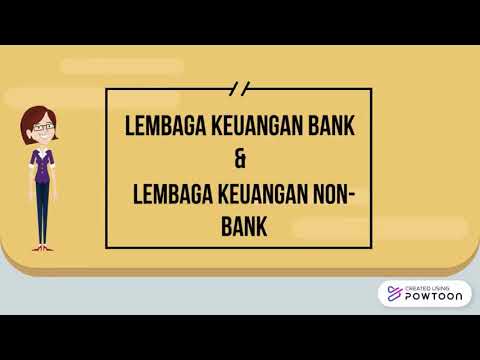
Lembaga Keuangan Bank dan Lembaga Keuangan Non-Bank
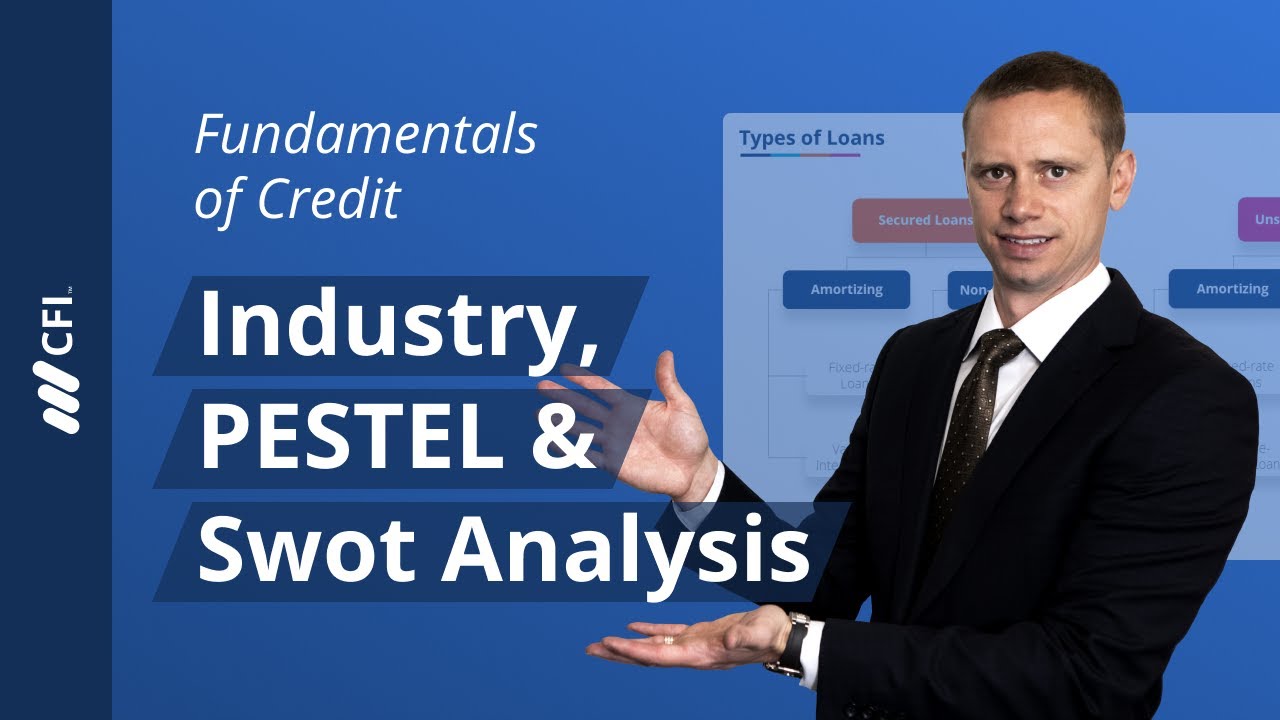
Credit Analysis | Fundamentals of Credit (Part 4)
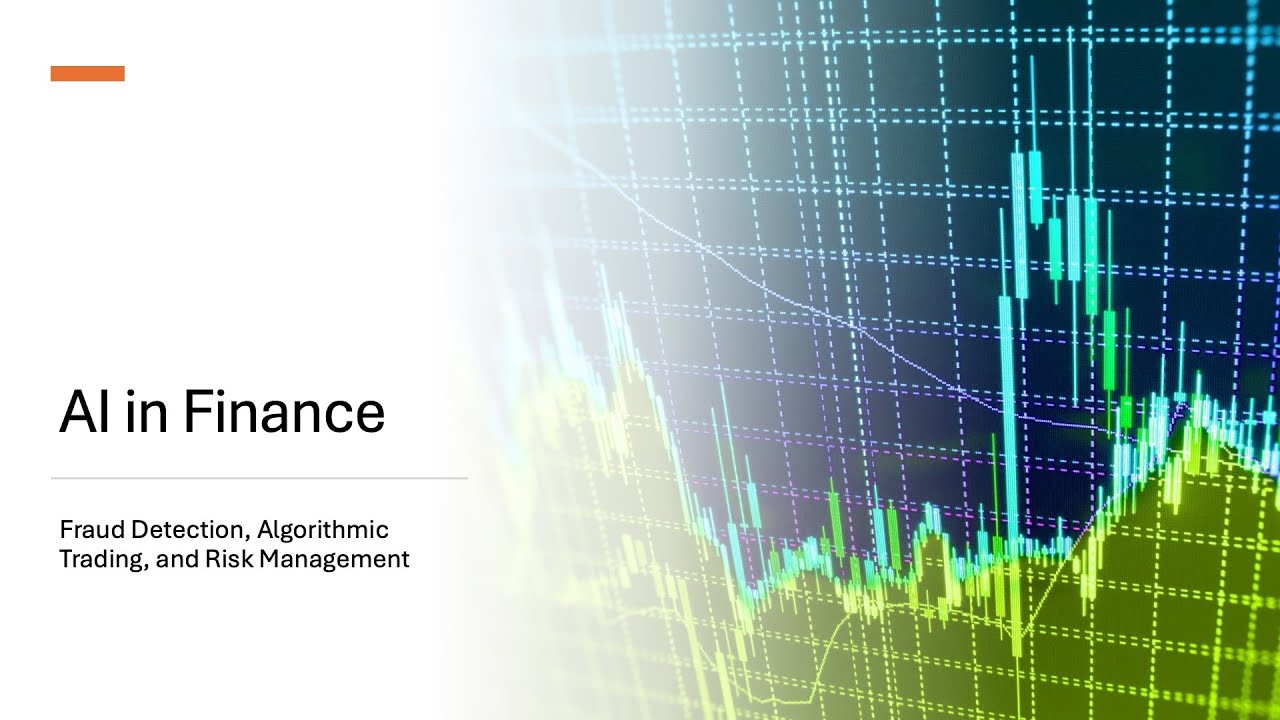
AI in Finance - Fraud Detection, Algorithmic Trading, and Risk Management

The mysterious new industry cranking out billionaires
5.0 / 5 (0 votes)