Realtime Age and Gender estimation using MLKit and Tensor Flow Android Studio | Visitor Analysis
Summary
TLDRIn this video, the presenter demonstrates how to build a machine learning-based Android app for visitor analysis, combining Google ML Kit's face detection with custom TensorFlow models for age and gender estimation. The app is designed to track visitors in real-time, analyzing demographics and emotions like smiling or non-smiling. Using face detection, the app predicts the age and gender of people captured by security or store cameras, offering valuable insights into customer happiness and demographics. The app also provides tracking and analysis features, useful for businesses to enhance customer experience and tailor marketing strategies.
Takeaways
- 😀 This video is about adding a 'visitor analysis' feature to an Android app using machine learning.
- 😀 The app analyzes age, gender, and facial expressions (smiling or not) of individuals captured on camera.
- 😀 Google’s ML Kit is used for face detection, while custom TensorFlow models are employed for age and gender estimation.
- 😀 The purpose of the app is to track customer demographics and sentiment in environments like stores and malls.
- 😀 A large dataset (over 20,000 images) is used to train the models for age, gender, and ethnicity predictions.
- 😀 The app provides a real-time visitor analysis by detecting faces and calculating their age, gender, and smiling probability.
- 😀 By combining Google ML Kit and custom TensorFlow models, the app can track and display demographic data such as age ranges and sentiment.
- 😀 The 'visitor analysis' feature can be used in business contexts to analyze customer mood, demographics, and sales correlation.
- 😀 A key feature of the app is face tracking, which prevents duplicate analysis of the same individual in a crowd.
- 😀 The speaker simplifies the code, focusing on the integration of age and gender estimation models with minimal complexity.
- 😀 The code for this app is available on GitHub, with links to the source and further details about the models used.
Q & A
What is the primary purpose of the ML series demonstrator app discussed in the video?
-The primary purpose of the ML series demonstrator app is to perform age and gender analysis, as well as to determine whether individuals are smiling or not, using facial detection and machine learning models.
How does the app process the images for age and gender estimation?
-The app uses Google’s ML Kit for face detection, extracts the face from the image, and then passes it to custom TensorFlow models for age and gender estimation.
What machine learning models are combined to create the visitor analysis feature in the app?
-The app combines three models: Google’s ML Kit for face detection, a custom TensorFlow model for age estimation, and another for gender estimation.
What are the potential uses of the visitor analysis feature?
-The visitor analysis feature can be used for various purposes, such as tracking customer demographics (age, gender) and their emotional state (smiling or not), which can be combined with sales data to analyze customer satisfaction.
What does the age and gender estimation model in the app use as input?
-The model takes an image or video frame, detects faces using the ML Kit, and then passes the cropped face to the custom TensorFlow models for age and gender prediction.
What is the significance of the smiling probability in the app’s functionality?
-The smiling probability helps to determine the emotional state of individuals in the captured faces, which can provide insights into customer satisfaction or other emotional states in a business setting.
What is the role of the tracking ID in the face detection process?
-The tracking ID ensures that the same face is not reanalyzed multiple times, helping to avoid duplicate processing of the same person and maintaining accurate data.
What changes were made to simplify the code for better understanding?
-The code was simplified by removing unnecessary files and streamlining the vision-based processor, making it easier to understand and follow.
Where can developers access the code for this ML demonstrator app?
-The code for the app is available on GitHub, and the link to the repository is provided in the video description.
What dataset was used to train the custom TensorFlow models for age and gender estimation?
-The custom models were trained on the UTKFace dataset, which includes over 20,000 images with labeled data for age, gender, ethnicity, and other factors.
Outlines
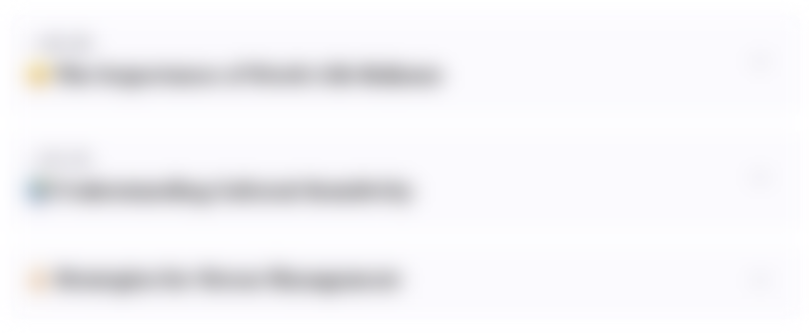
This section is available to paid users only. Please upgrade to access this part.
Upgrade NowMindmap
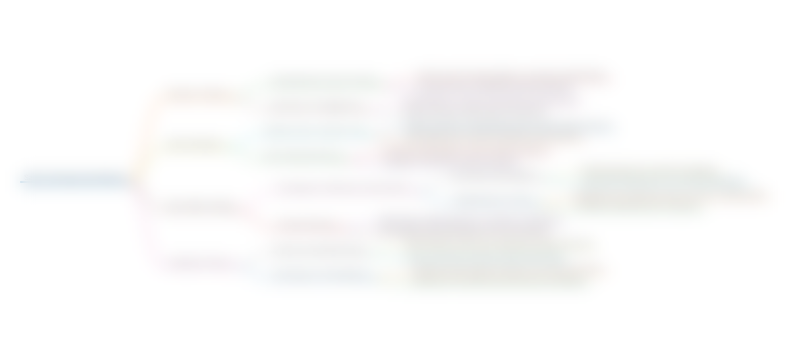
This section is available to paid users only. Please upgrade to access this part.
Upgrade NowKeywords
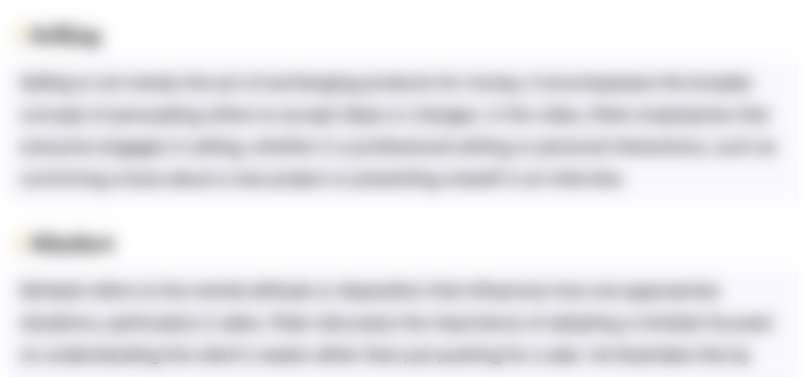
This section is available to paid users only. Please upgrade to access this part.
Upgrade NowHighlights
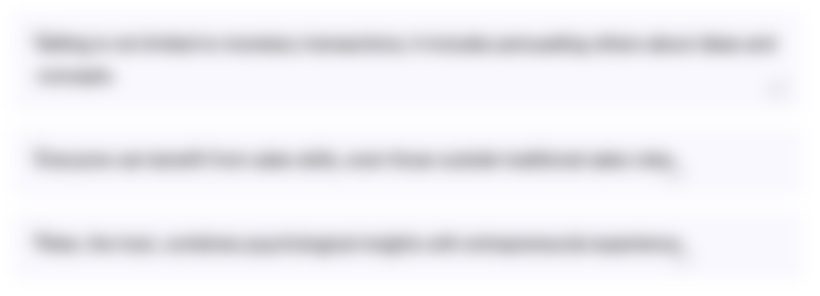
This section is available to paid users only. Please upgrade to access this part.
Upgrade NowTranscripts
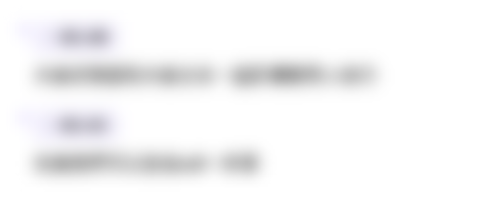
This section is available to paid users only. Please upgrade to access this part.
Upgrade NowBrowse More Related Video
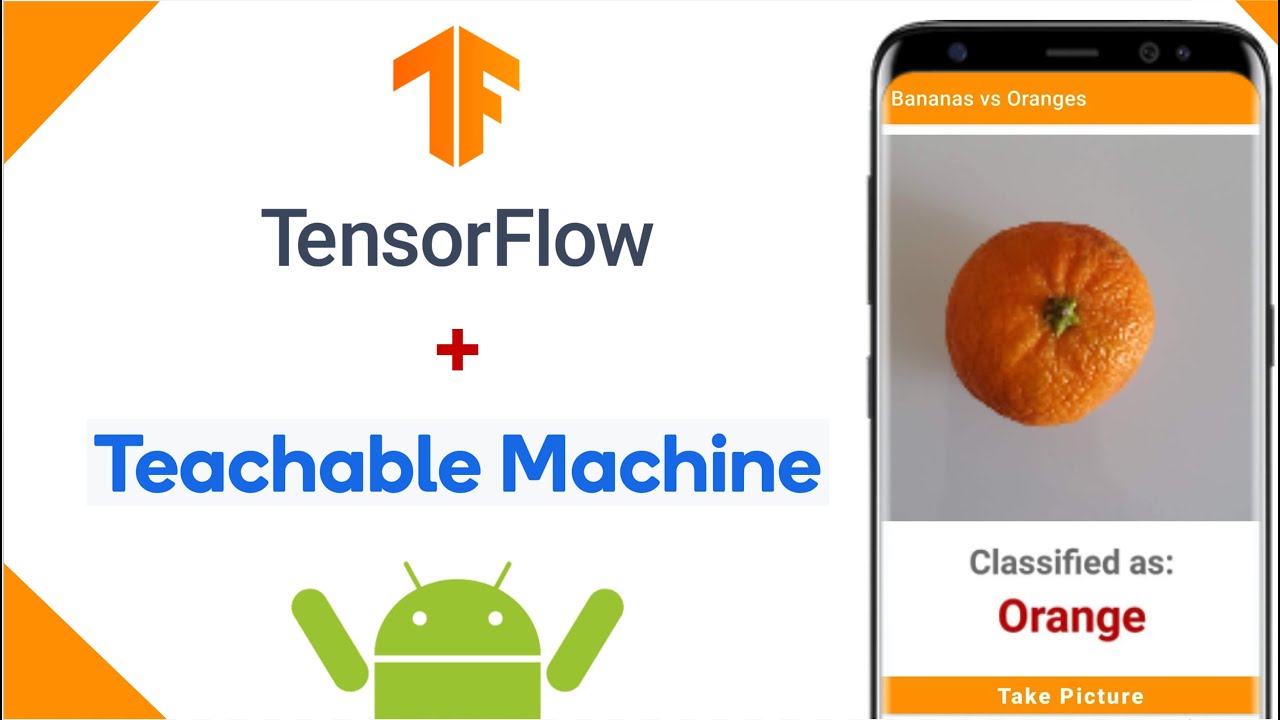
Image Classification App | Teachable Machine + TensorFlow Lite
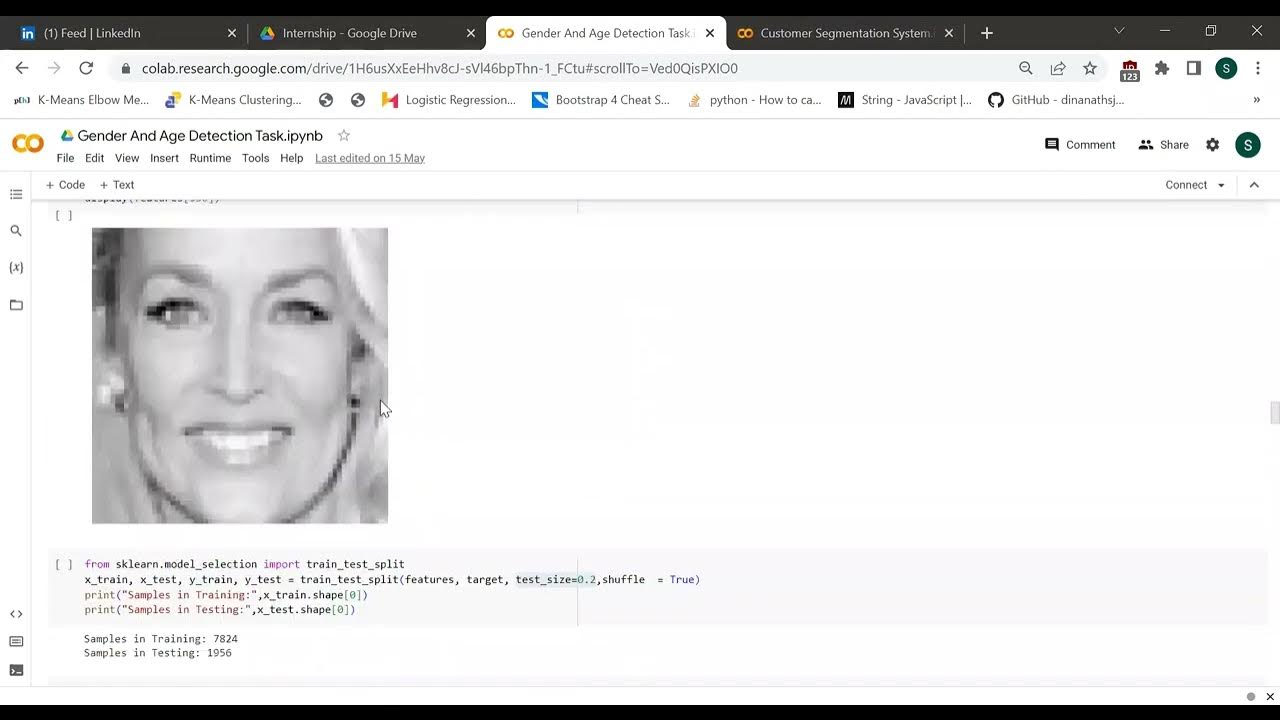
Gender and Age Detection using CNN and SVM classifier | DL Project.
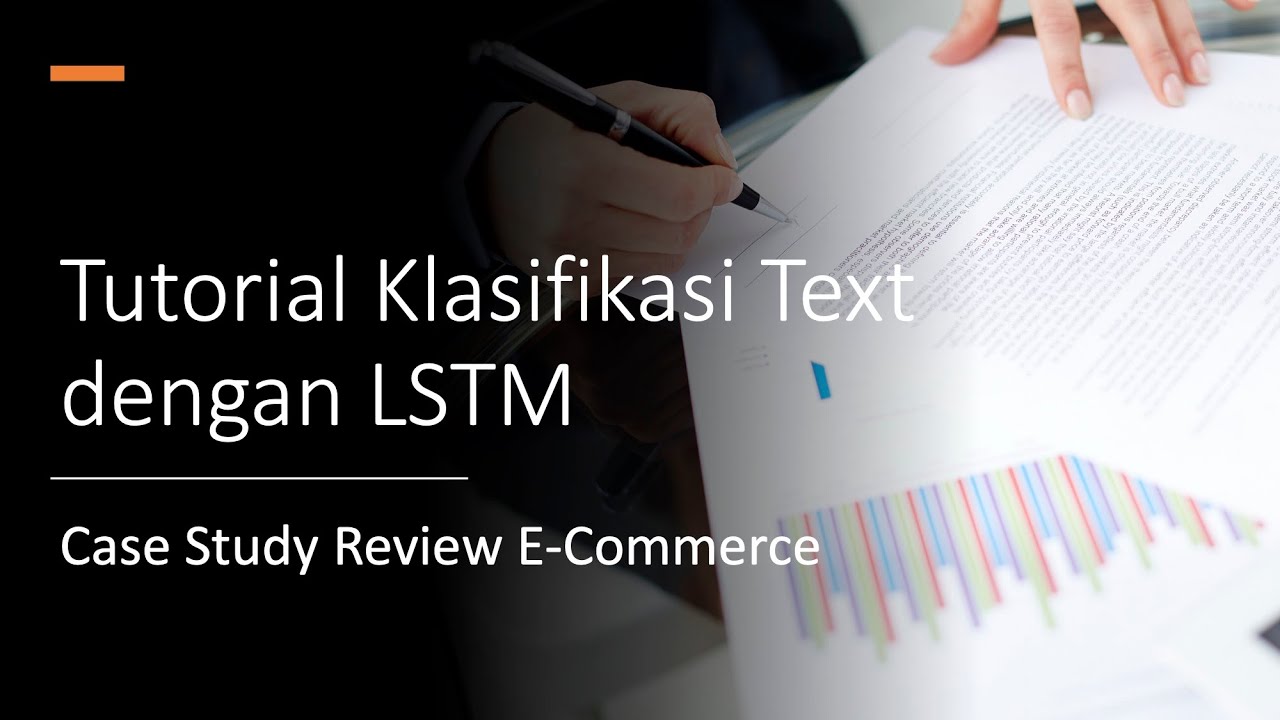
Tutorial Klasifikasi Teks dengan Long Short-term Memory (LSTM): Studi Kasus Teks Review E-Commerce
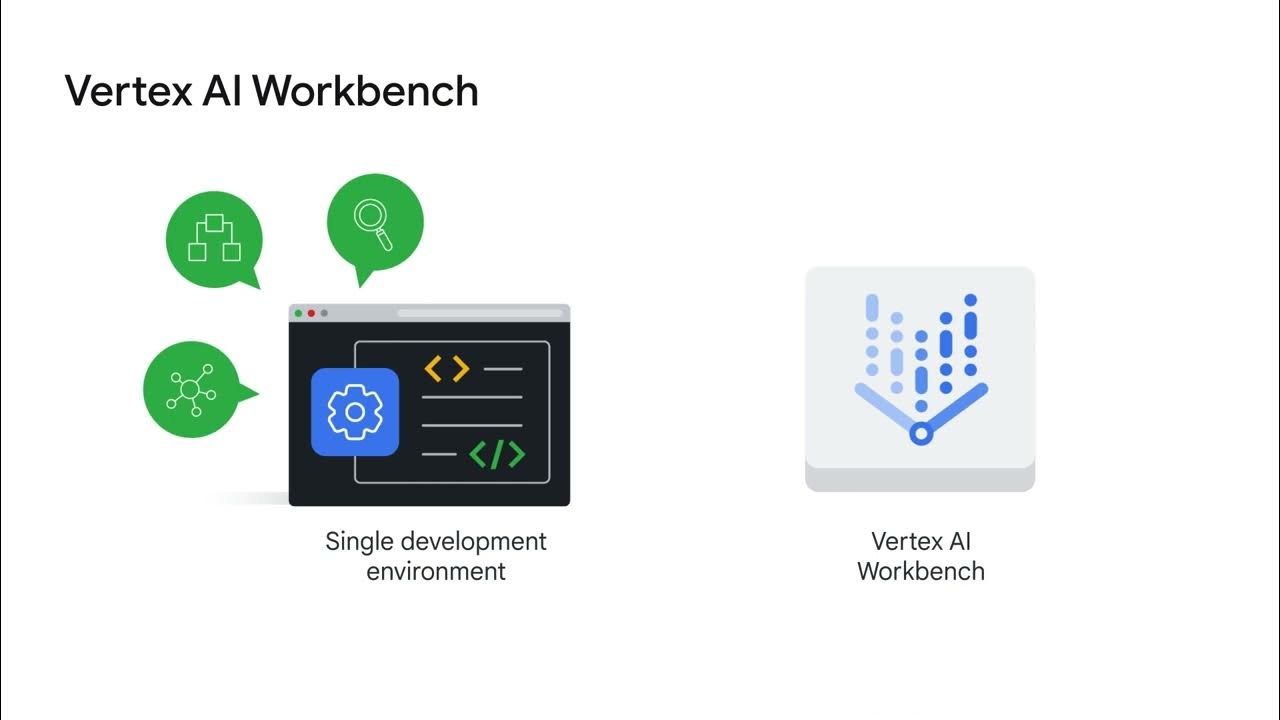
Custom training
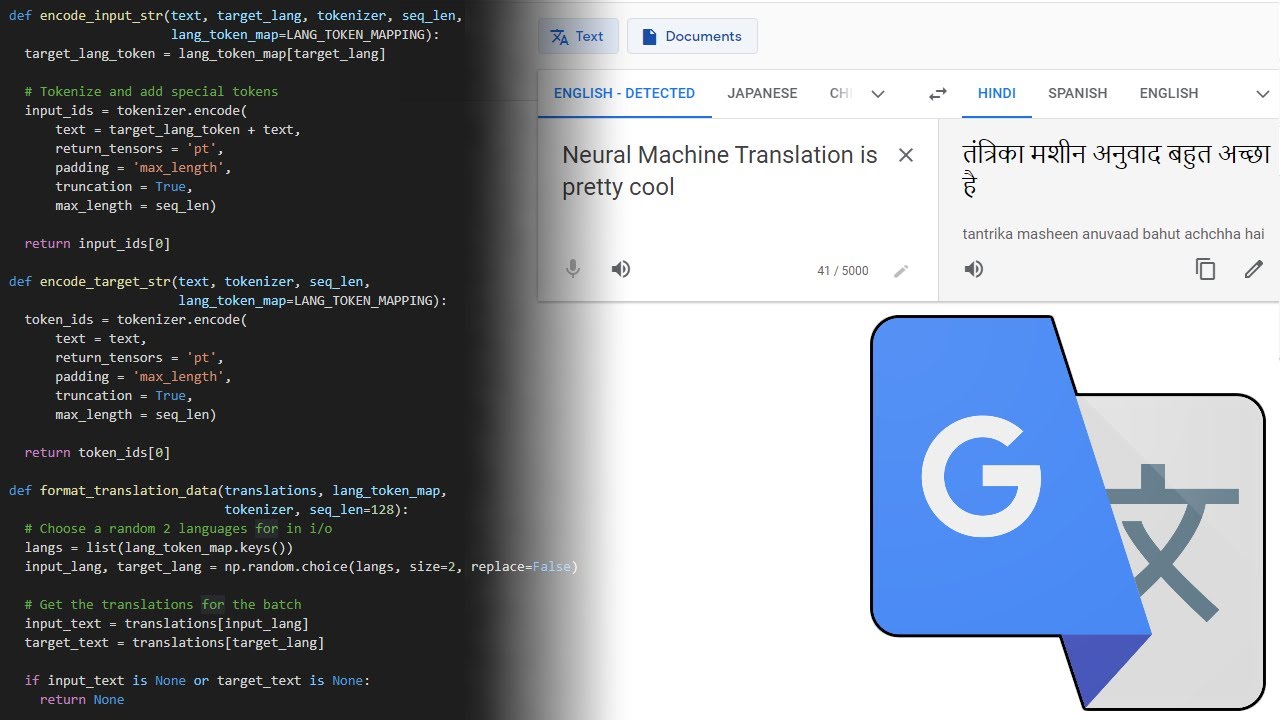
Let's Recreate Google Translate! | Neural Machine Translation
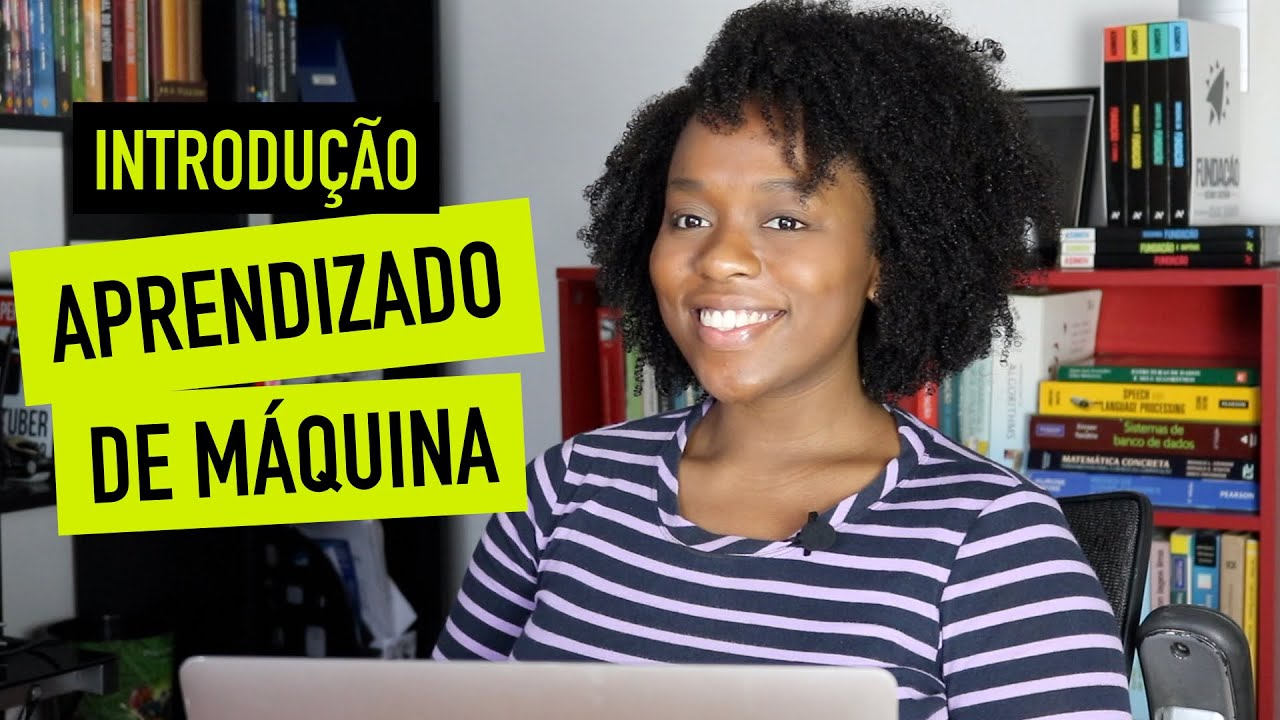
O que é MACHINE LEARNING? Introdução ao APRENDIZADO DE MÁQUINA | Machine Learning #1
5.0 / 5 (0 votes)