Managing machine learning projects( coursera )
Summary
TLDRThis video discusses the application of machine learning (ML) in financial advisory and portfolio management. It highlights the challenge of economic volatility and the need for informed investment decisions. ML can help analyze large financial data, optimize asset allocation, and create personalized investment strategies. The system aims to provide higher returns than traditional fixed deposits while targeting a portfolio growth of X% over one year. The solution includes cloud-based processing, periodic predictions, and the use of A/B testing to validate the approach. Risks such as data drift and economic policy changes are also addressed.
Takeaways
- 😀 The project focuses on financial advisory and portfolio management using machine learning (ML) to help individuals maximize wealth creation.
- 😀 Economic volatility makes it essential to make informed investment decisions to ensure personal wealth growth and avoid financial losses.
- 😀 A personal financial advisor for everyone is expensive and often inaccessible, creating a need for alternative, affordable solutions.
- 😀 Machine learning is relevant because it can process large amounts of financial data, helping to make decisions about asset allocation and investment strategies.
- 😀 The target users are adult, working individuals who are busy and often forget to manage their investments properly.
- 😀 The solution aims to optimize investment strategies based on user needs, risk profiles, and market conditions to ensure better returns than traditional options like bank deposits.
- 😀 The goal is to help users avoid large financial losses by ensuring their investments are properly allocated and risk-managed.
- 😀 A key metric for success is increasing the overall portfolio value by a specified percentage over a year.
- 😀 The proposed system will use machine learning to generate personalized investment strategies and will involve A/B testing for validation of different strategies.
- 😀 The ML system design considers cloud-based solutions for scalability, offline versus online decision-making, and periodic updates to investment predictions, ensuring timely and relevant advice.
- 😀 Potential risks in the production model include issues like data latency, concept drift, and the need to adapt to new investment trends such as cryptocurrencies or changes in taxation policies.
Q & A
What is the main objective of the project presented in the script?
-The main objective is to use machine learning to help individual investors make informed decisions regarding financial advisory and portfolio management, particularly in volatile economic environments.
Why is it difficult for individuals to access personal financial advisors?
-Personal financial advisors are often too costly and not easily accessible to everyone, especially for individuals who need regular, affordable consultations.
How does machine learning help in financial decision-making?
-Machine learning helps by analyzing large, complex financial data to generate optimized investment strategies, making it possible to automate decisions based on risk tolerance, asset performance, and personal financial goals.
Who is the target audience for the proposed machine learning solution?
-The target audience includes adult working professionals who are too busy to manage their finances, forgetful individuals, and traders who need to make decisions based on market changes and economic fluctuations.
What are some of the challenges faced by the target audience?
-Challenges include understanding the right allocation for investments, risk management, optimizing spending, and facing the high costs and limited availability of personal financial advisors.
Why is the solution important in the context of the current financial environment?
-The solution is important because it helps individuals avoid large financial losses due to poor investment choices, providing a cost-effective alternative to personal financial advisors while ensuring better financial decision-making.
What are the key features of the machine learning system design?
-The system design includes a cloud-based solution for scalability, offline learning for investment reasoning on a bi-weekly basis, and batch prediction to make predictions at specific intervals.
What does 'concept drift' refer to in the context of the model?
-Concept drift refers to the phenomenon where changes in financial trends, such as new asset classes or economic shifts, may cause the model to become less accurate, requiring periodic updates.
What are some potential risks identified in the production phase of the machine learning model?
-Potential risks include concept drift, latency issues when processing large datasets, and changes in financial regulations or investment trends that could affect the model’s accuracy.
What are the desired metrics and outcomes for the proposed solution?
-The desired outcomes include portfolio growth, a targeted return on investment (e.g., X% over one year), and the need for individuals to regularly update their asset details and financial goals to improve the model’s recommendations.
Outlines
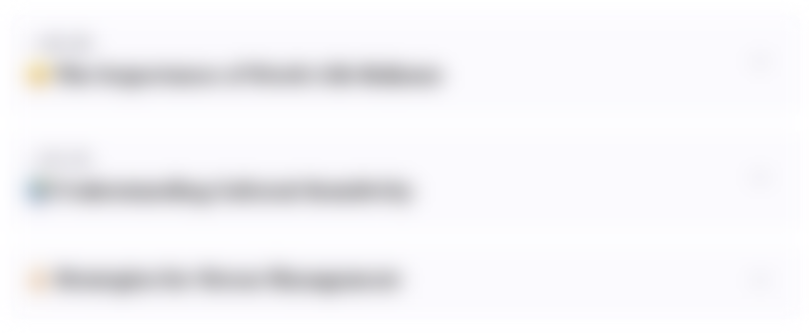
This section is available to paid users only. Please upgrade to access this part.
Upgrade NowMindmap
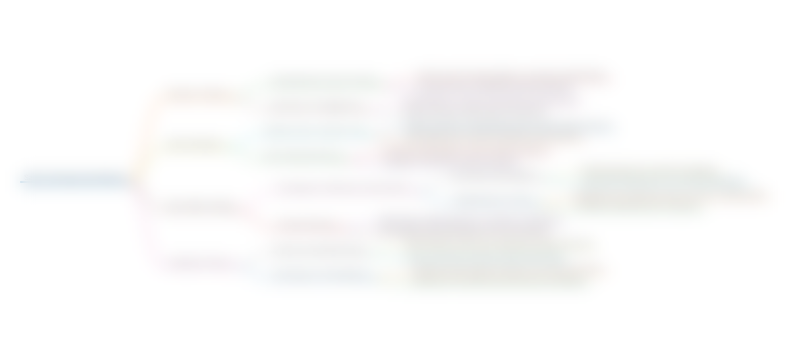
This section is available to paid users only. Please upgrade to access this part.
Upgrade NowKeywords
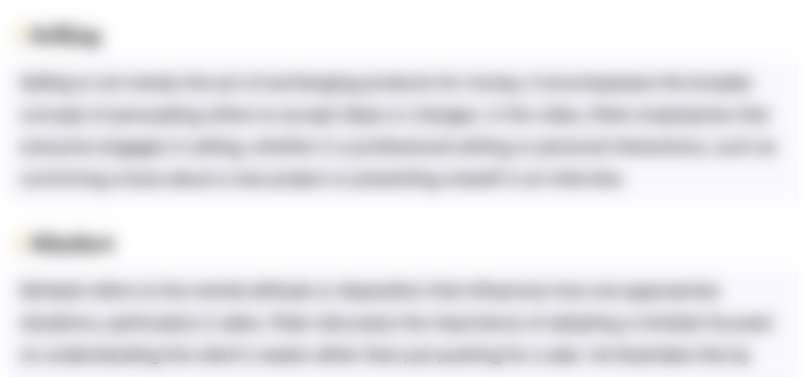
This section is available to paid users only. Please upgrade to access this part.
Upgrade NowHighlights
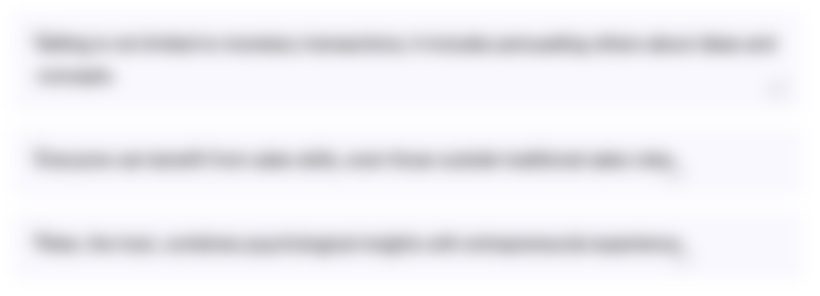
This section is available to paid users only. Please upgrade to access this part.
Upgrade NowTranscripts
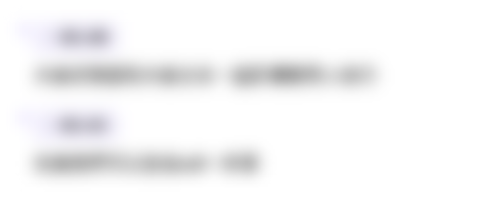
This section is available to paid users only. Please upgrade to access this part.
Upgrade NowBrowse More Related Video

AWS re:Invent 2020: Understand ML model predictions & biases with Amazon SageMaker Clarify
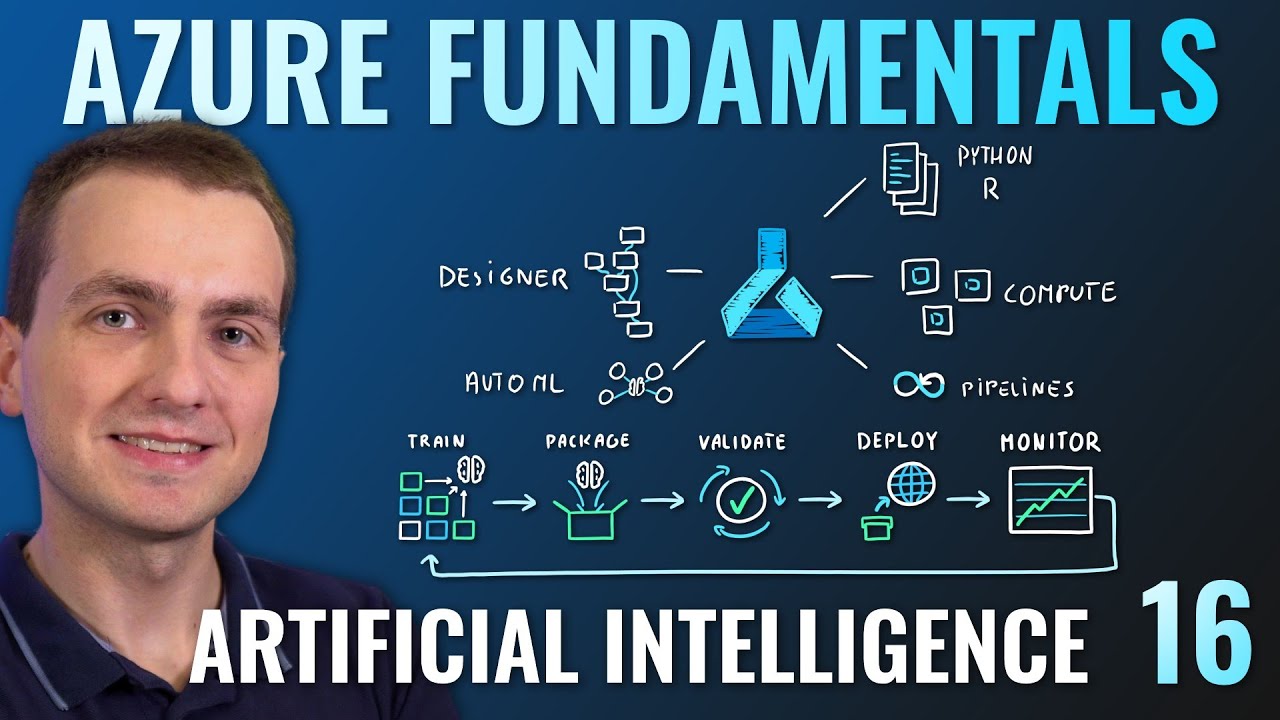
AZ-900 Episode 16 | Azure Artificial Intelligence (AI) Services | Machine Learning Studio & Service
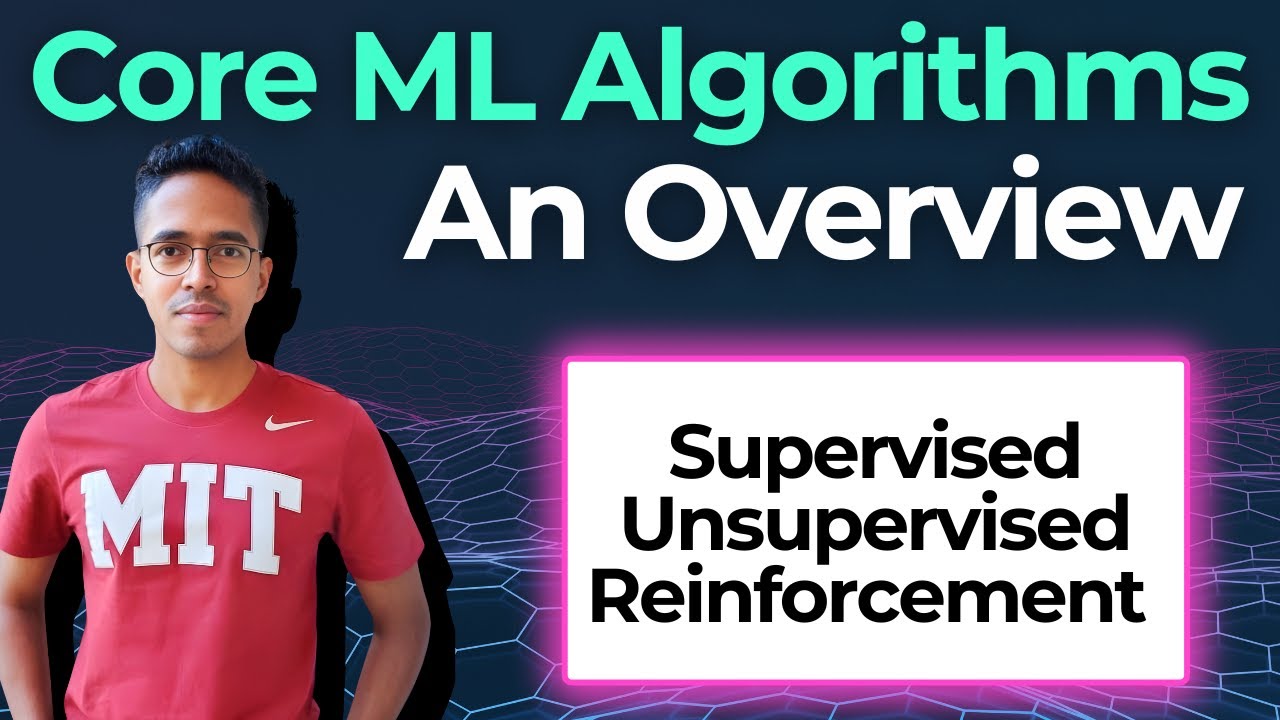
Machine Learning Algorithms Overview - What all exist out there?
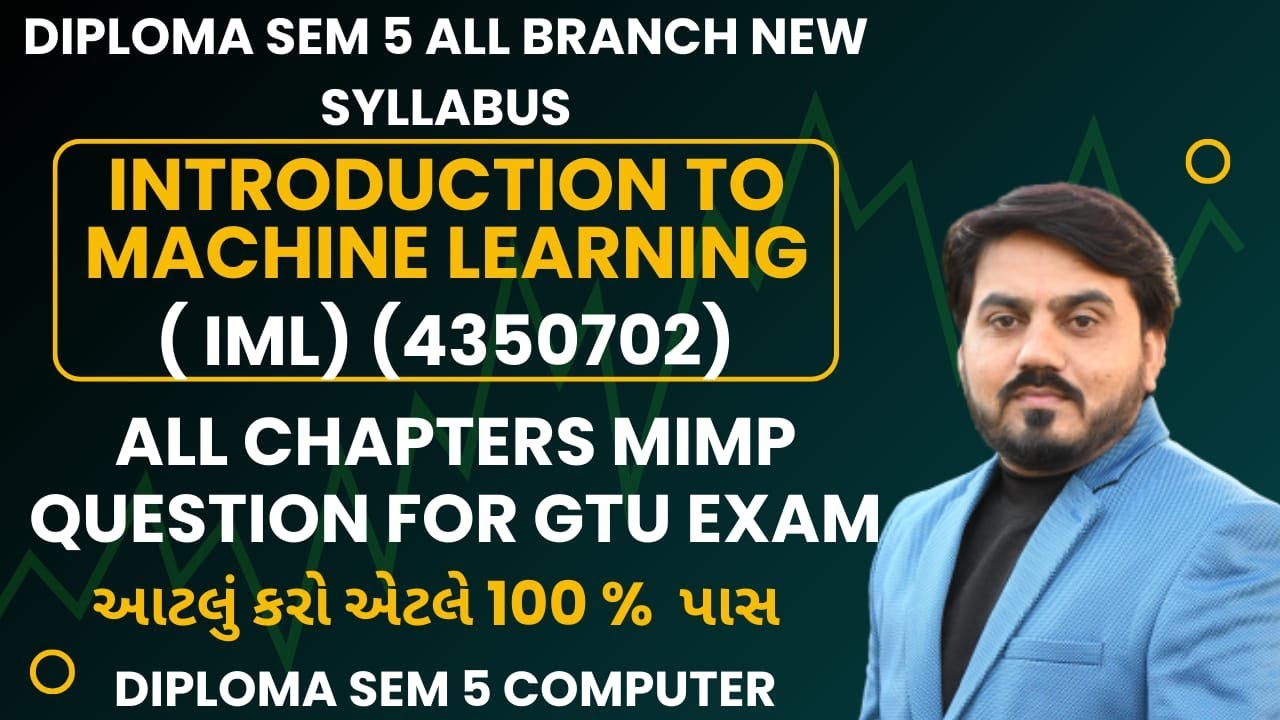
INTRODUCTION TO MACHINE LEARNING (IML) MIMP QUESTION FOR GTU EXAM | SEM 5 COMPUTER MIMP FOR GTU #gtu
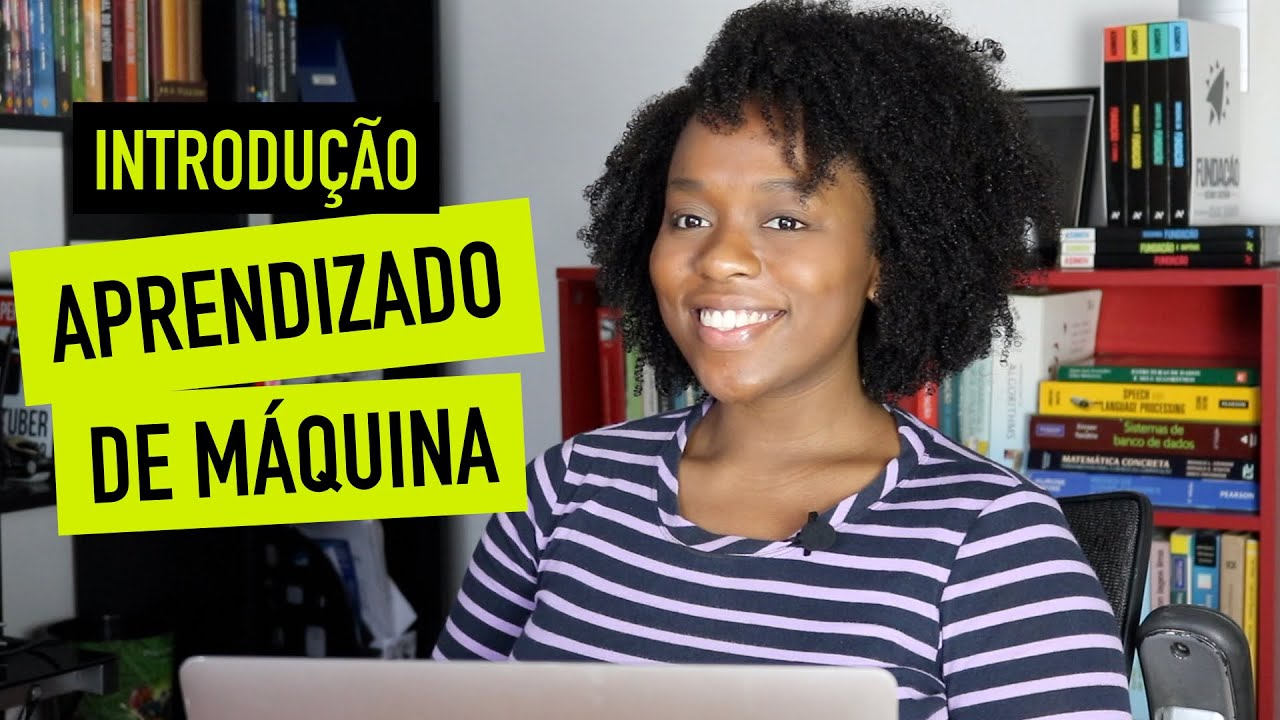
O que é MACHINE LEARNING? Introdução ao APRENDIZADO DE MÁQUINA | Machine Learning #1
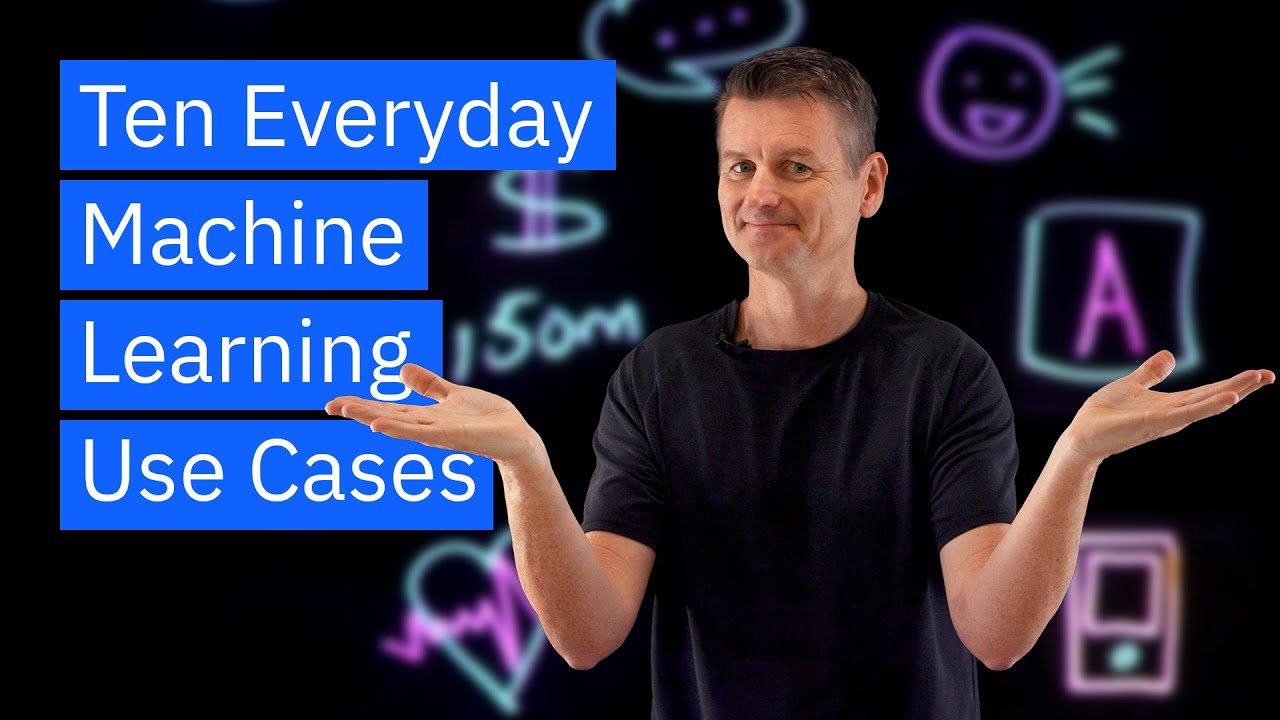
Ten Everyday Machine Learning Use Cases
5.0 / 5 (0 votes)