ME401 Group1 Application of Artificial Intelligence In Fatigue and Fracture
Summary
TLDRThis video explores the transformative role of artificial intelligence (AI) in fatigue and fracture analysis within engineering. The team discusses AI-driven predictive models for fatigue life estimation, AI-based crack detection, and reinforcement learning for optimized material design. Using machine learning techniques like neural networks, deep learning, and support vector machines, AI enhances the precision of structural health monitoring, reduces testing costs, and improves safety across industries like aerospace, automotive, and civil engineering. The video highlights how AI is reshaping material design, crack detection, and predictive maintenance for more durable and efficient systems.
Takeaways
- ๐ AI is revolutionizing fatigue and fracture analysis by providing faster, more precise predictions of material failure in real-world conditions.
- ๐ Machine learning models, including artificial neural networks, support vector machines, and deep learning techniques, enhance fatigue life estimation by learning from experimental, sensor, and simulation data.
- ๐ Neural networks can predict crack growth and fatigue life by analyzing complex, nonlinear relationships between input factors like stress, temperature, and loading cycles.
- ๐ AI-based methods are more adaptive and precise than traditional methods, such as SN curves and fracture mechanics, particularly in handling noisy and incomplete data.
- ๐ Real-time sensor data, like strain gauges and accelerometers, allows AI systems to update fatigue life estimations dynamically and predict failure more accurately.
- ๐ AI technologies are being applied across industries, including aerospace, automotive, and civil engineering, to improve structural reliability and optimize material design.
- ๐ Convolutional neural networks (CNNs) and other image processing techniques are being used for real-time crack detection, reducing human error and improving inspection safety.
- ๐ Reinforcement learning accelerates material design by exploring and optimizing microstructures for fatigue resistance, leading to stronger, lighter, and longer-lasting materials.
- ๐ AI helps in creating smarter, more efficient structural health monitoring systems that can detect issues early, prevent failures, and save both time and money.
- ๐ The future of engineering involves smarter, AI-powered systems that not only predict fatigue but also optimize material properties and enhance the safety of critical infrastructure.
Q & A
How is AI transforming the way we estimate fatigue life in engineering?
-AI is revolutionizing fatigue life estimation by enabling models to learn from real-world data, including simulations and sensor information. Unlike traditional methods, AI can adjust to varying conditions and provide quicker and more precise predictions, especially in complex real-world loading situations.
What machine learning techniques are used to predict fatigue life?
-Machine learning techniques like artificial neural networks, support vector machines, and deep learning methods such as convolutional and recurrent neural networks are used. These methods can handle large datasets, including sensor data, and adjust to complex and noisy real-world conditions.
What are some advantages of AI in fatigue analysis over traditional methods?
-AI offers advantages like lower testing expenses, enhanced precision in complex scenarios, and real-time monitoring and adjustments. It also improves predictive accuracy in cases with multi-axial or varying amplitude loads, which conventional methods struggle to handle.
How does AI contribute to real-time monitoring and maintenance?
-AI models can update fatigue life estimations dynamically using real-time sensor data such as strain gauges and accelerometers. This enables instant monitoring and helps engineers identify parts or components close to failure, allowing for proactive maintenance and extending the lifespan of equipment.
What industries benefit from AI-driven fatigue analysis?
-Industries like aerospace, automotive, civil engineering, and offshore structures benefit from AI-driven fatigue analysis. AI helps predict fatigue life for aircraft components, engine parts, bridge decks, and pipelines, improving structural integrity and safety.
How are neural networks used in fracture mechanics?
-Neural networks are used in fracture mechanics to analyze stress points, predict crack growth, and identify weaknesses in materials. They adjust their internal settings based on past data, enabling faster and more accurate predictions of material failure.
What are the potential applications of AI in material design?
-AI is used to design stronger, lighter, and more durable materials by optimizing microstructures. Reinforcement learning, in particular, accelerates material discovery by exploring design possibilities and learning which structures offer the best resistance to fatigue.
What is the role of AI in crack detection and classification?
-AI, especially convolutional neural networks (CNNs), is used to analyze images and detect cracks with high precision. It helps identify small cracks early, monitor the health of structures like bridges and buildings, and classify cracks based on severity, all while improving inspection safety and speed.
What are some challenges in implementing AI for crack detection?
-Challenges include the need for large, high-quality data sets, potential misidentification of shadows or stains as cracks, and high setup costs for equipment like cameras, GPUs, and drones. Additionally, some models may struggle with different environments.
How does AI in fracture mechanics help engineers design safer systems?
-AI allows engineers to predict material failures early, which helps in designing safer systems. By forecasting when and how materials will crack, engineers can make informed decisions, reduce downtime, and avoid accidents, ultimately saving both time and money.
Outlines
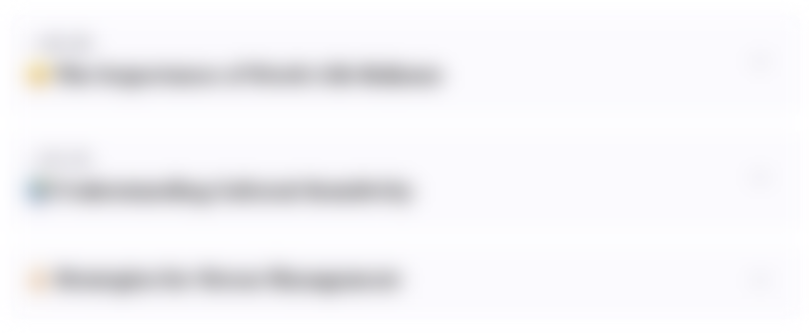
This section is available to paid users only. Please upgrade to access this part.
Upgrade NowMindmap
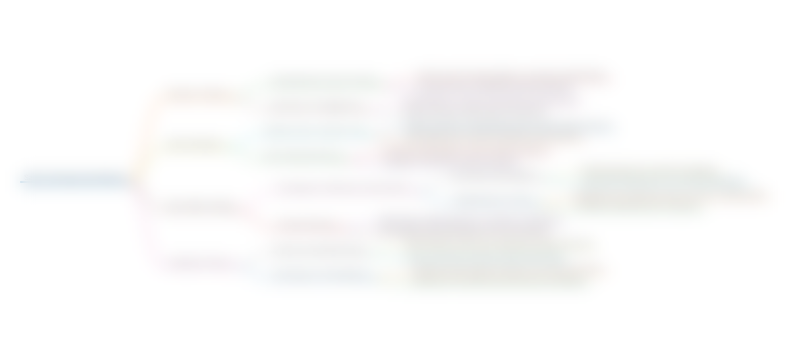
This section is available to paid users only. Please upgrade to access this part.
Upgrade NowKeywords
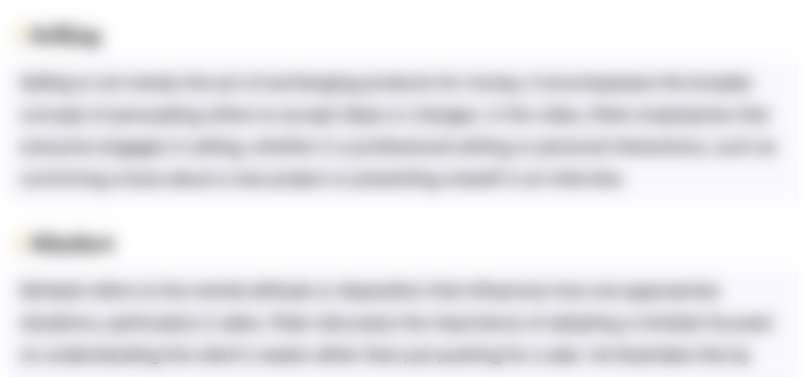
This section is available to paid users only. Please upgrade to access this part.
Upgrade NowHighlights
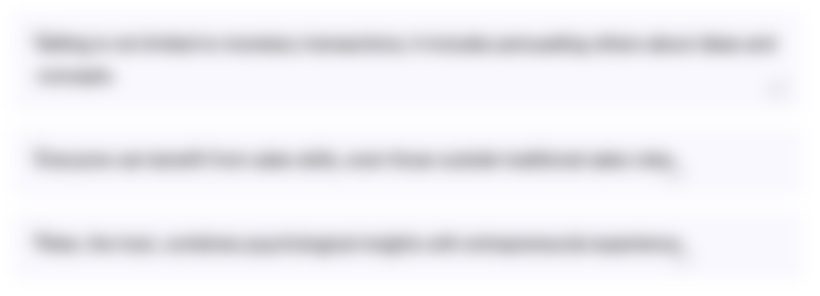
This section is available to paid users only. Please upgrade to access this part.
Upgrade NowTranscripts
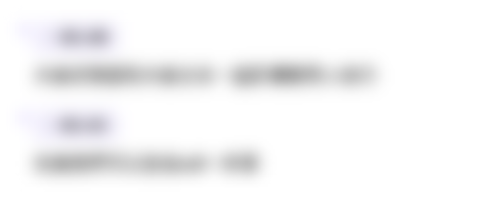
This section is available to paid users only. Please upgrade to access this part.
Upgrade NowBrowse More Related Video
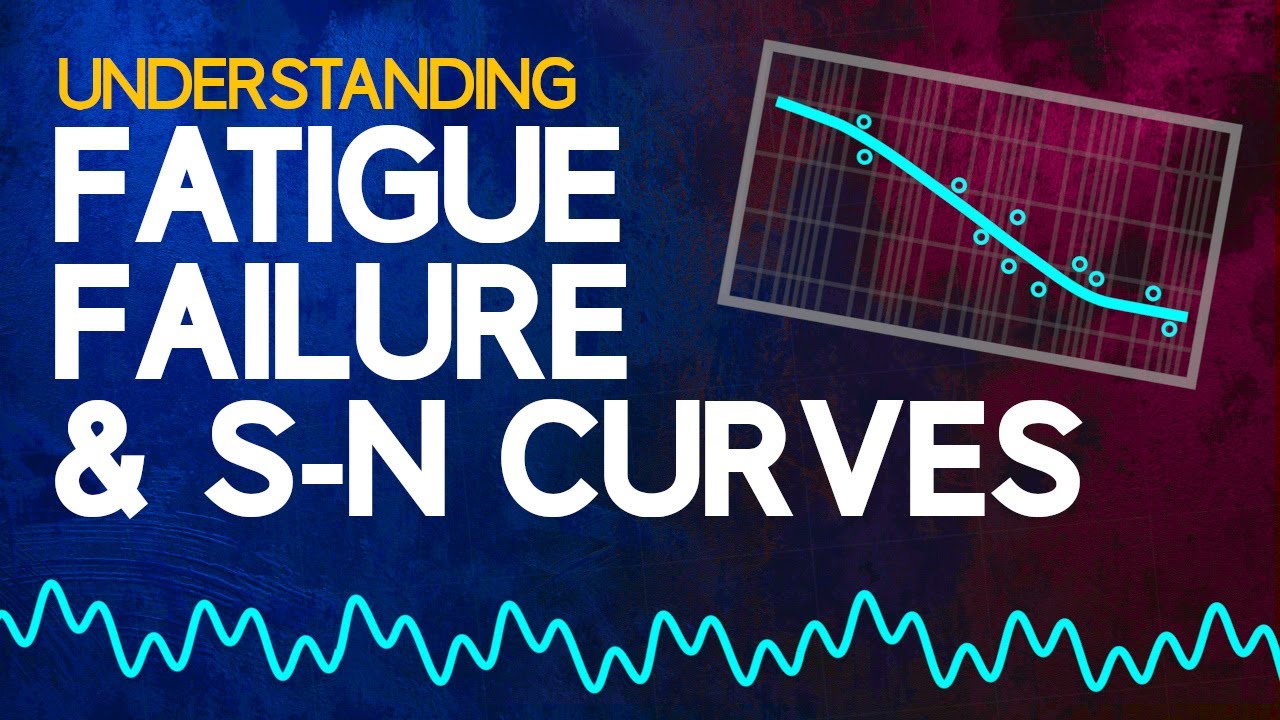
Understanding Fatigue Failure and S-N Curves
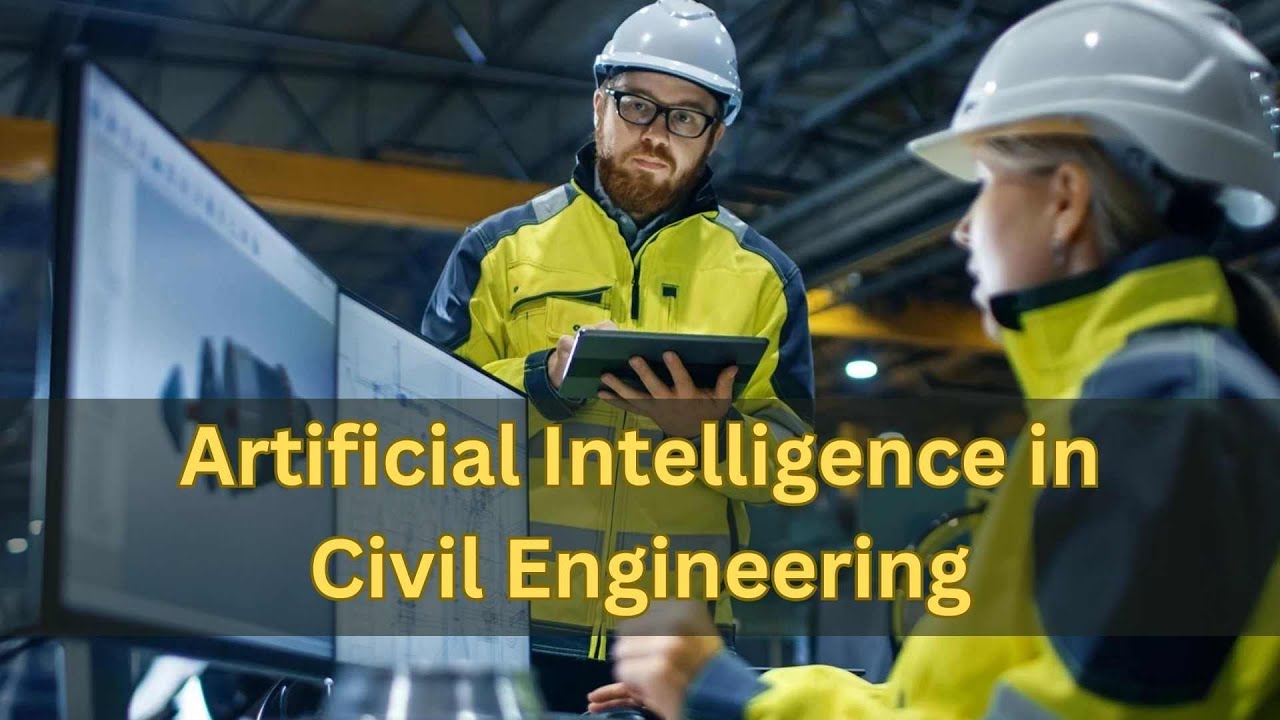
The Role of Artificial Intelligence in Civil Engineering

How AI is Revolutionizing Finance and Accounting
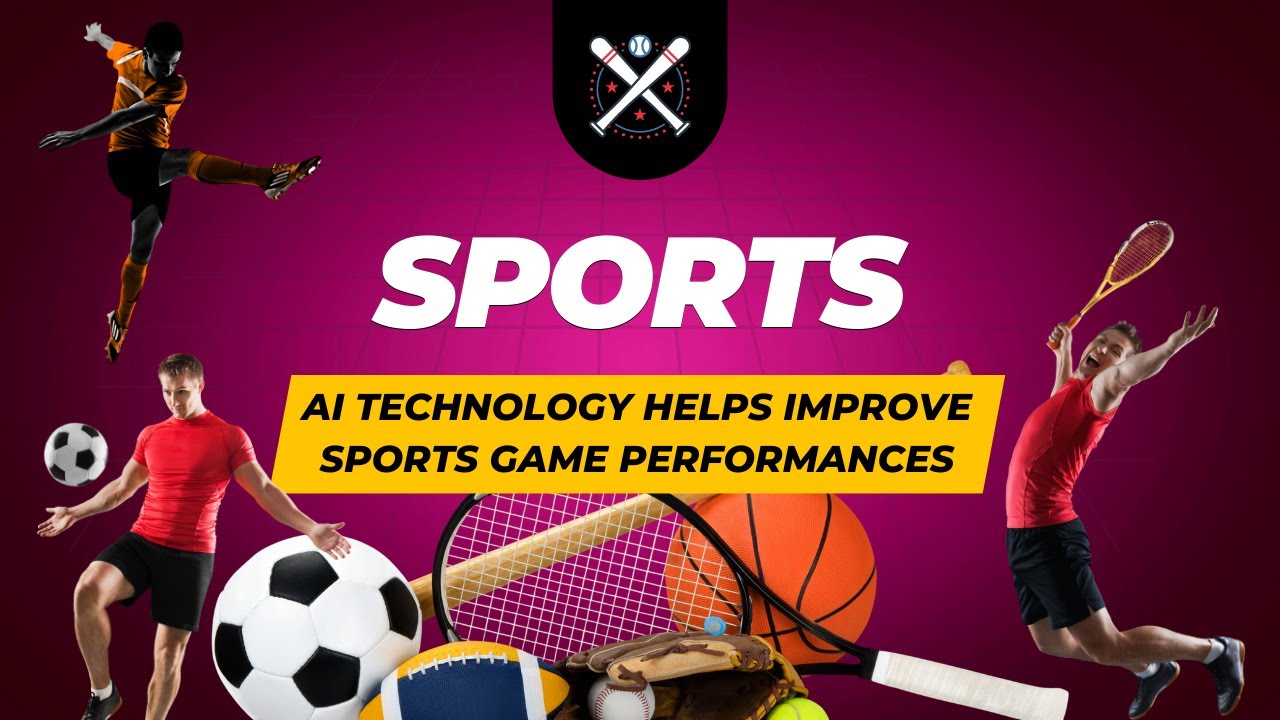
How AI Technology Helps Improve Sports Game Performances || AI Studio ||
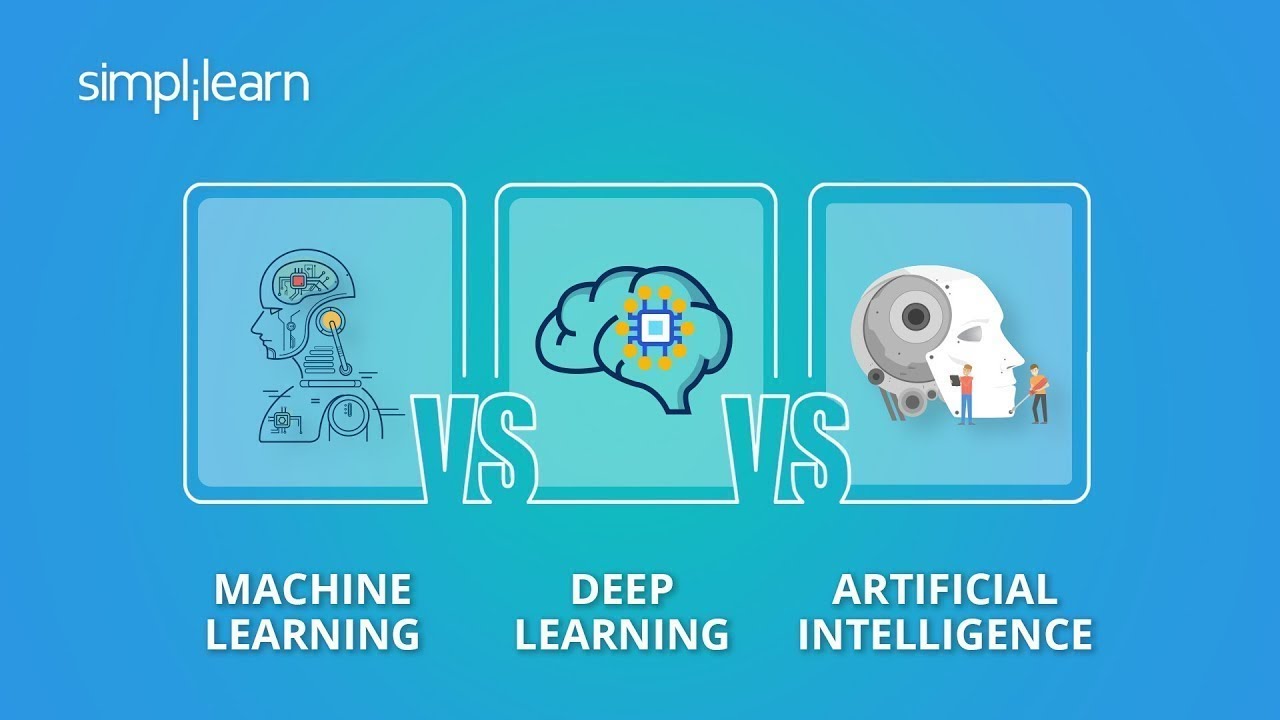
Machine Learning vs Deep Learning vs Artificial Intelligence | ML vs DL vs AI | Simplilearn

Artificial Intelligence and the Environment | Scott Switzer | TEDxPaloAltoHighSchool
5.0 / 5 (0 votes)