Everything You Ever Wanted to Know About Bayes' Theorem But Were Afraid To Ask.
Summary
TLDRThis video uncovers the fascinating story of how an 18th-century Presbyterian minister, Thomas Bayes, unknowingly laid the groundwork for artificial intelligence. His probability theory, initially used in the insurance industry, evolved through the work of Pierre-Simon Laplace and later influenced Alan Turing’s wartime codebreaking efforts. In the 1980s, Judea Pearl applied Bayes' theory to AI, leading to modern applications like IBM's Watson, which famously defeated humans on Jeopardy. The video highlights how Bayes' revolutionary insights on conditional probability helped transform the world of data, predictions, and artificial intelligence.
Takeaways
- 😀 Thomas Bayes, an 18th-century Presbyterian minister, developed a groundbreaking theory of probability that would eventually influence artificial intelligence (AI).
- 😀 Bayes' work on making educated guesses in uncertain situations led to the concept of Bayesian probability, which revolutionized decision-making models.
- 😀 After Bayes' death, his notebooks were discovered by Richard Price, who recognized the value of the theory and published it in 1763.
- 😀 Conditional probability, developed by Pierre-Simon Laplace, is an extension of Bayes' ideas and allows for updating guesses with new information.
- 😀 An example of conditional probability is how the accuracy of a dengue fever test is evaluated, considering both false positives and true positives.
- 😀 Despite its usefulness, Bayesian probability faced criticism from frequentist statisticians, who preferred data-driven approaches relying on large datasets.
- 😀 Frequentists argued that probabilities could only be reliably known with large data sets, while Bayesian theory was seen as based on uncertain assumptions.
- 😀 Bayesian probability played a crucial role in breaking secret codes during World War II, with Alan Turing using it to crack encrypted transmissions.
- 😀 The rivalry between Bayesian and frequentist methods persisted throughout the 20th century, with Bayesian methods gaining increasing recognition in AI.
- 😀 In the 1980s, Judea Pearl used Bayesian reasoning to develop AI algorithms that could make decisions based on incomplete or uncertain data, helping bring AI closer to reality.
- 😀 In 2011, IBM's Watson used Bayesian probability to win on *Jeopardy!*, showcasing the lasting impact of Bayes' work on AI and its decision-making capabilities.
Q & A
Who was Thomas Bayes, and why is he important in the history of probability theory?
-Thomas Bayes was an 18th-century Presbyterian minister who developed a theory of probability based on updating beliefs with new evidence, now known as Bayesian probability. His work laid the foundation for modern statistical reasoning and later played a crucial role in artificial intelligence.
How did Richard Price contribute to the spread of Bayes's work?
-Richard Price, a philosopher, discovered Thomas Bayes's notebooks after his death. He recognized the significance of Bayes's theory, cleaned up the math, and published it in an obscure journal in 1763, helping to bring attention to the idea.
What is Bayesian probability, and how is it used in real-world applications?
-Bayesian probability is a statistical method that allows you to update the probability of an event occurring based on new evidence. For example, in medical testing, Bayesian probability helps refine the accuracy of test results by considering both the test’s accuracy and the rarity of the disease.
How does Bayesian probability differ from frequentist probability?
-Frequentist probability relies on large sample sizes and objective data to infer true probabilities, while Bayesian probability incorporates subjective beliefs and updates them with new data. The key difference lies in how they handle uncertainty and unknown information.
What was the issue with using frequentist probability in early artificial intelligence development?
-In early AI, the frequentist approach struggled because it required large datasets to make accurate predictions, which was difficult in real-world scenarios where data was limited or unreliable. Bayesian probability offered a solution by working with available data and updating predictions as new information came in.
How did Alan Turing apply Bayesian methods during World War II?
-Alan Turing used Bayesian probability to help break encrypted German messages during World War II. By updating the likelihood of different encryption methods based on incoming data, Turing’s use of Bayesian methods played a crucial role in deciphering enemy communications.
What role did Judea Pearl play in the development of AI and its connection to Bayesian probability?
-Judea Pearl was a key figure in the 1980s who developed a new approach to AI based on Bayesian probability. His work enabled AI systems to reason under uncertainty and update their beliefs as new data became available, marking a significant step forward in AI development.
How did Bayesian probability contribute to Watson's success on Jeopardy?
-Watson, the AI computer developed by IBM, used Bayesian probability to answer questions intelligently during its appearance on Jeopardy. By continually updating its beliefs about the most likely correct answer based on the available data, Watson was able to outperform human contestants.
What is conditional probability, and how is it used in Bayesian reasoning?
-Conditional probability is the likelihood of an event occurring given that another event has already occurred. In Bayesian reasoning, it is used to update the probability of an event based on new evidence. For example, it helps assess the probability of a disease given the results of a medical test.
Why is Bayesian probability especially useful in situations with limited or uncertain data?
-Bayesian probability is particularly useful in uncertain situations because it allows for the incorporation of prior knowledge and new data to make educated guesses. This flexibility makes it applicable in fields like AI, medicine, and decision-making where data may be scarce or incomplete.
Outlines
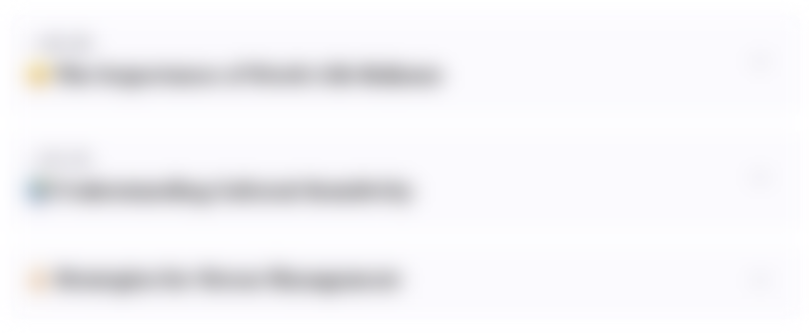
This section is available to paid users only. Please upgrade to access this part.
Upgrade NowMindmap
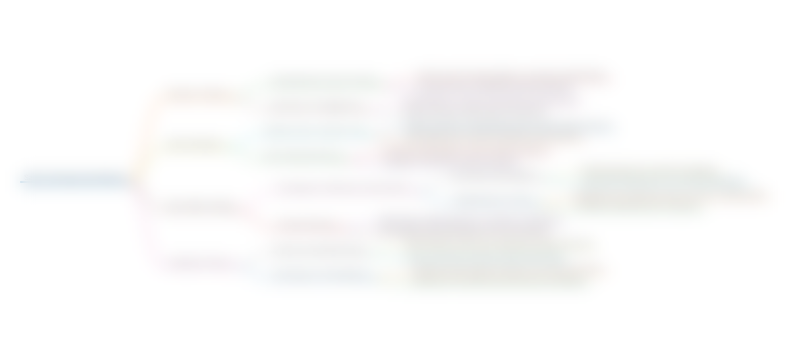
This section is available to paid users only. Please upgrade to access this part.
Upgrade NowKeywords
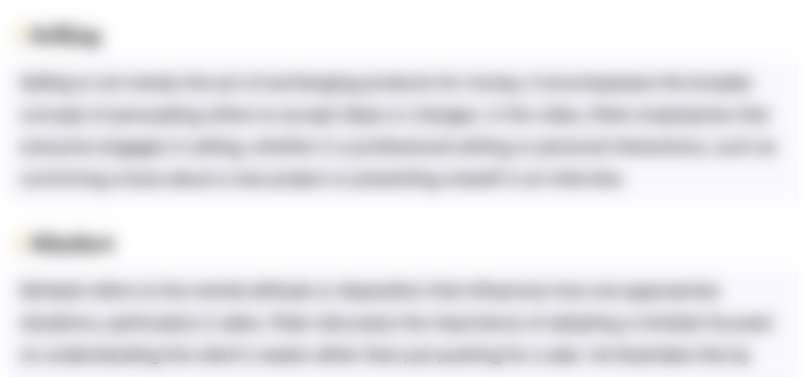
This section is available to paid users only. Please upgrade to access this part.
Upgrade NowHighlights
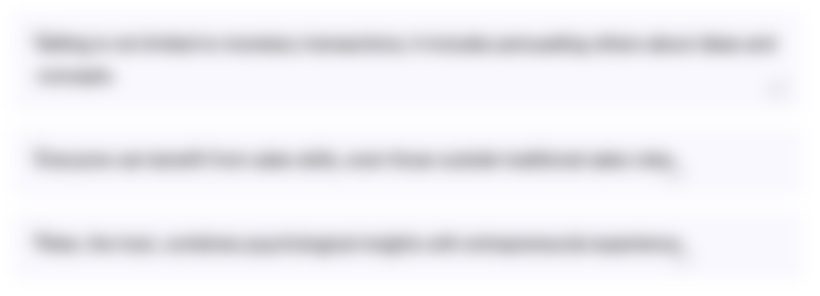
This section is available to paid users only. Please upgrade to access this part.
Upgrade NowTranscripts
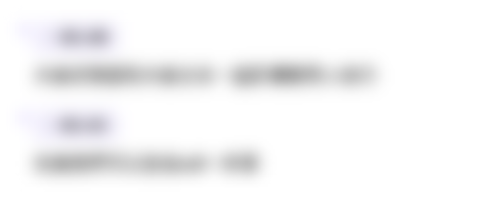
This section is available to paid users only. Please upgrade to access this part.
Upgrade Now5.0 / 5 (0 votes)