Stanford Med LIVE: The State of AI in Healthcare and Medicine
Summary
TLDRThis panel discussion explores the integration of AI into healthcare, focusing on its potential to enhance patient care while addressing challenges such as biases, privacy, and transparency. Experts highlight the importance of ensuring that AI systems are developed ethically, with input from affected communities, and stress the need for collaboration across disciplines. They discuss the balance between optimism for AI's benefits and the caution needed to prevent harm, emphasizing transparency, accountability, and the need for continual monitoring to ensure equitable outcomes. The conversation underscores the role of academic institutions in leading responsible AI innovation.
Takeaways
- 😀 AI in healthcare holds great potential, but it requires collaboration across different sectors to ensure its responsible application.
- 😀 Data security and privacy are major concerns in AI adoption, with ongoing efforts to secure patient data and develop technologies that increase privacy.
- 😀 Discrimination and bias in AI, particularly in healthcare, stem from social determinants of health, and while AI can help identify these biases, societal changes are needed for true equity.
- 😀 To prevent discrimination in AI, it's important to ask about the data, the algorithm, and the actions expected from the AI, and ensure equity in all three aspects.
- 😀 AI can help identify flaws missed by physicians, but it requires training specific to each task (e.g., detecting small polyps in colonoscopies).
- 😀 Transparent engagement with communities and patients is crucial to ensure that AI systems are developed with their interests in mind.
- 😀 There is a push for more patient involvement in AI development, particularly in governance, to ensure their concerns and needs are met.
- 😀 Collaboration between academic medical centers, startups, and industry partners is essential to create lasting, effective AI solutions in healthcare.
- 😀 Continuous monitoring of AI algorithms is necessary to ensure their reliability and to detect any potential issues, especially as data and conditions evolve.
- 😀 Optimism about AI in healthcare is rooted in the fact that it offers opportunities for innovation and improvement, especially when driven by a commitment to equity and accountability.
Q & A
What are the main concerns related to AI and healthcare discussed in the transcript?
-The primary concerns include ensuring data security and privacy, preventing bias and discrimination in AI models, the ethical use of AI in healthcare, and the need for transparency and accountability in AI development. There is also a focus on the importance of engaging all stakeholders, especially patients, in the development and deployment of AI.
How does AI contribute to biases in healthcare, according to the discussion?
-AI can perpetuate biases by reflecting the social determinants of health, such as poverty and race, leading to discriminatory outcomes. These biases are often rooted in real-world disparities, and while AI can be adjusted to reduce bias, the underlying societal issues must also be addressed for lasting change.
What role does transparency play in AI healthcare applications?
-Transparency is crucial in AI healthcare applications to ensure that patients and stakeholders understand how AI models work, what data is used, and how decisions are made. It helps prevent inequities and ensures accountability in how AI is applied to healthcare decisions.
What is the significance of community engagement in AI development for healthcare?
-Community engagement is vital to ensure that the needs and concerns of those impacted by AI, particularly patients, are addressed. It is necessary for creating more equitable AI systems and ensuring that AI technologies align with the values and expectations of society.
What specific example of AI use in healthcare is mentioned in the transcript?
-An example mentioned is an algorithm developed by Sarah Alorn at Hopkins, which better predicts which cancer patients will benefit from palliative radiation based on social determinants of health. This AI model helps avoid unnecessary treatments for patients with shorter life expectancies.
What approach should be taken when developing AI algorithms for healthcare?
-AI algorithms should be developed with a focus on their data sources, the algorithms themselves, and the actions users are expected to take based on the algorithm’s output. These elements must be scrutinized for potential biases and inequities to ensure that AI is applied fairly and responsibly in healthcare.
How are industry partnerships and collaborations being approached in AI healthcare?
-Industry partnerships are approached with careful consideration, especially when startups are involved. Stanford, for example, ensures that its partners have a long-term commitment and are focused on supporting technologies that can be sustained in the healthcare ecosystem without being sold off or shut down prematurely.
What is the significance of 'learning health systems' in AI healthcare?
-Learning health systems refer to the continuous integration of new knowledge, where AI and data are used to constantly improve healthcare practices. The goal is to create systems that learn from each patient interaction and apply that knowledge to improve future care, with a focus on transparency and accountability.
What is the main source of optimism about AI's future in healthcare?
-The optimism stems from the collaboration among various experts, including clinicians, bioethicists, and technologists, who are working together to ensure that AI is developed with ethical considerations in mind. The interdisciplinary approach at institutions like Stanford offers hope for creating equitable and effective AI solutions.
What does the panel suggest about the future of AI in monitoring healthcare practices?
-The panel suggests that AI could play a significant role in monitoring and improving healthcare practices, such as identifying missed diagnoses or ensuring proper post-treatment follow-ups. However, the challenge lies in prioritizing which issues to address first and scaling AI solutions effectively.
Outlines
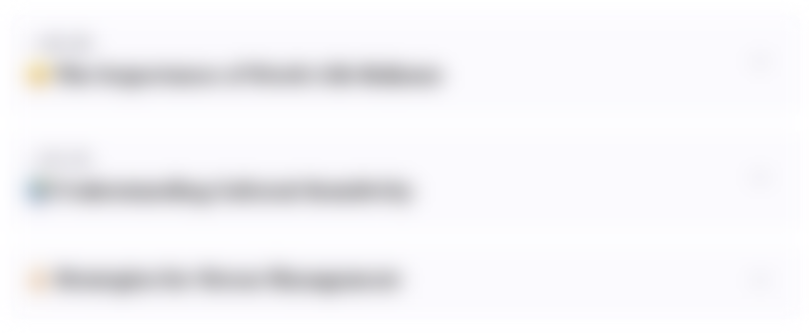
This section is available to paid users only. Please upgrade to access this part.
Upgrade NowMindmap
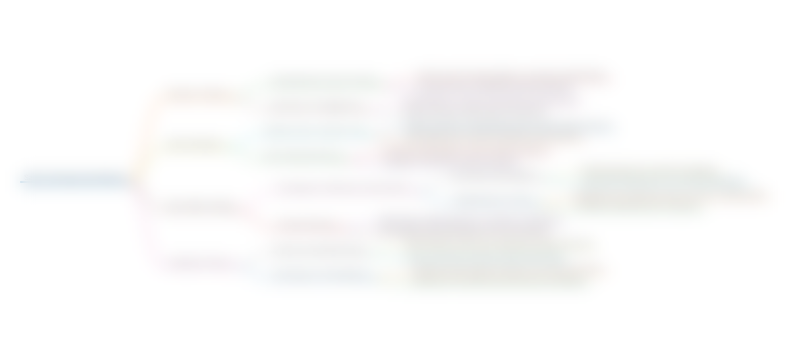
This section is available to paid users only. Please upgrade to access this part.
Upgrade NowKeywords
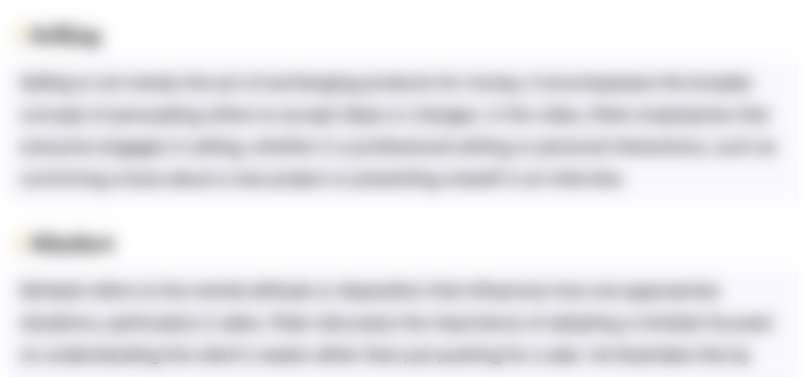
This section is available to paid users only. Please upgrade to access this part.
Upgrade NowHighlights
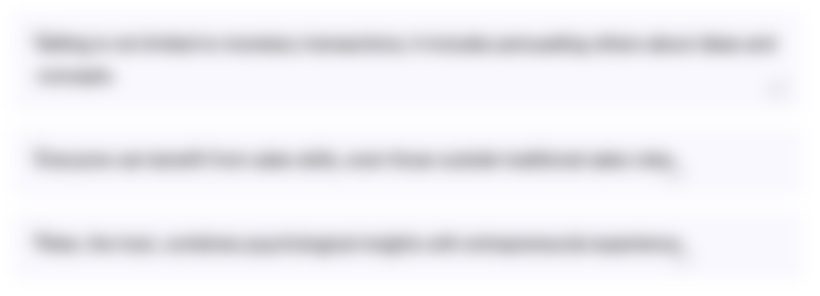
This section is available to paid users only. Please upgrade to access this part.
Upgrade NowTranscripts
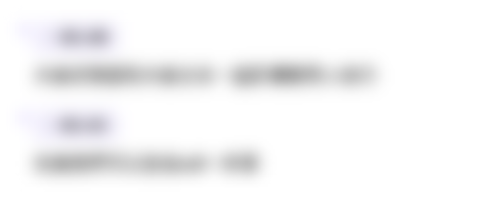
This section is available to paid users only. Please upgrade to access this part.
Upgrade NowBrowse More Related Video
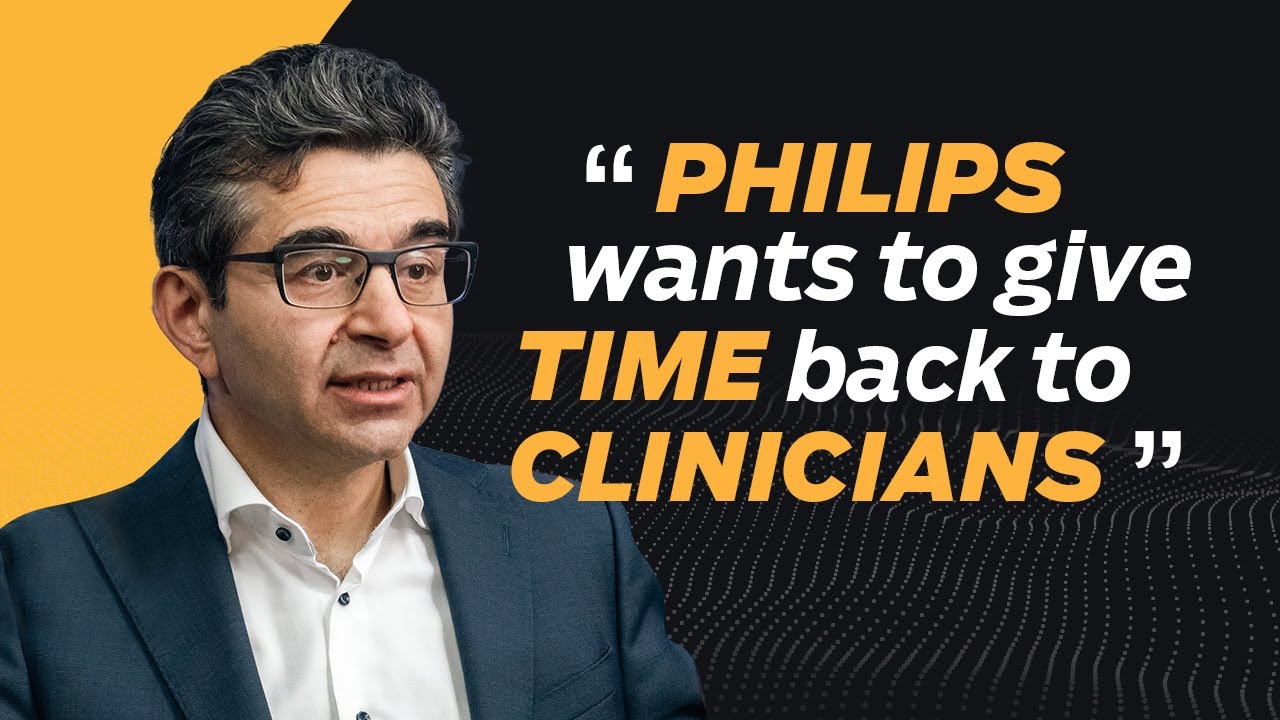
Tell me why time is a barrier to better health?
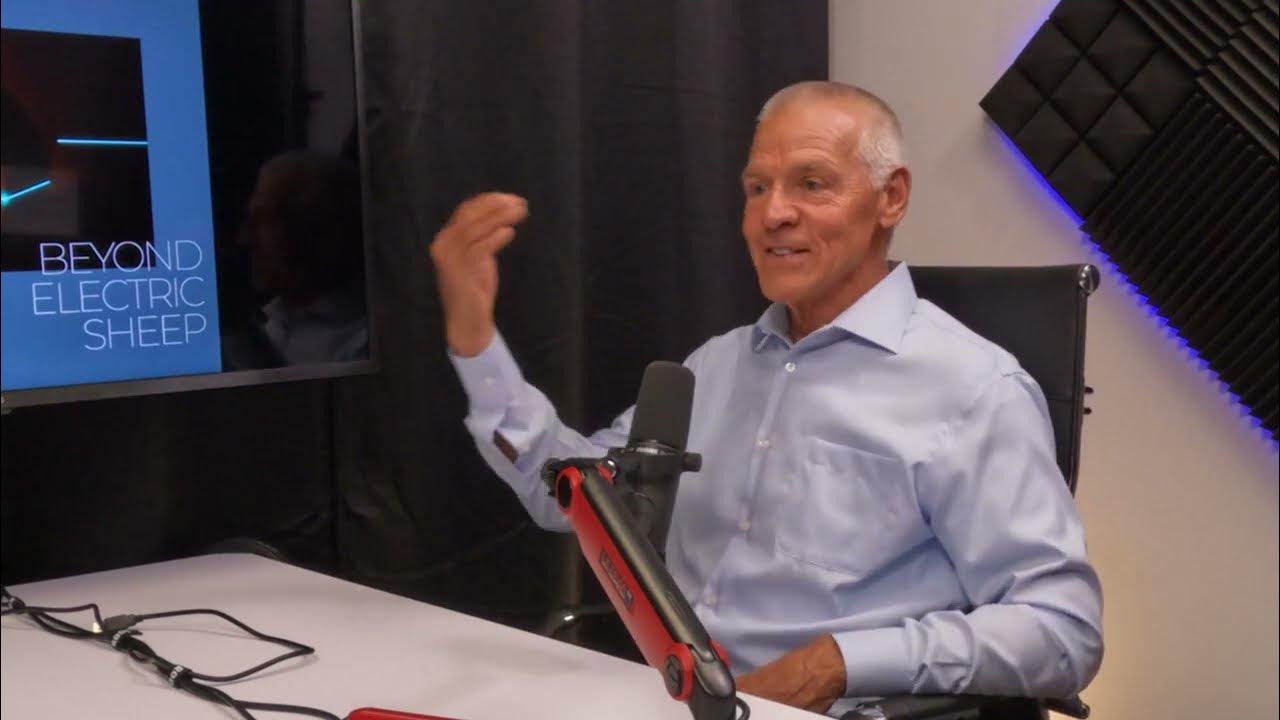
Episode 19 – AI in Clinical Medicine - Beyond Electric Sheep - A Podcast on AI
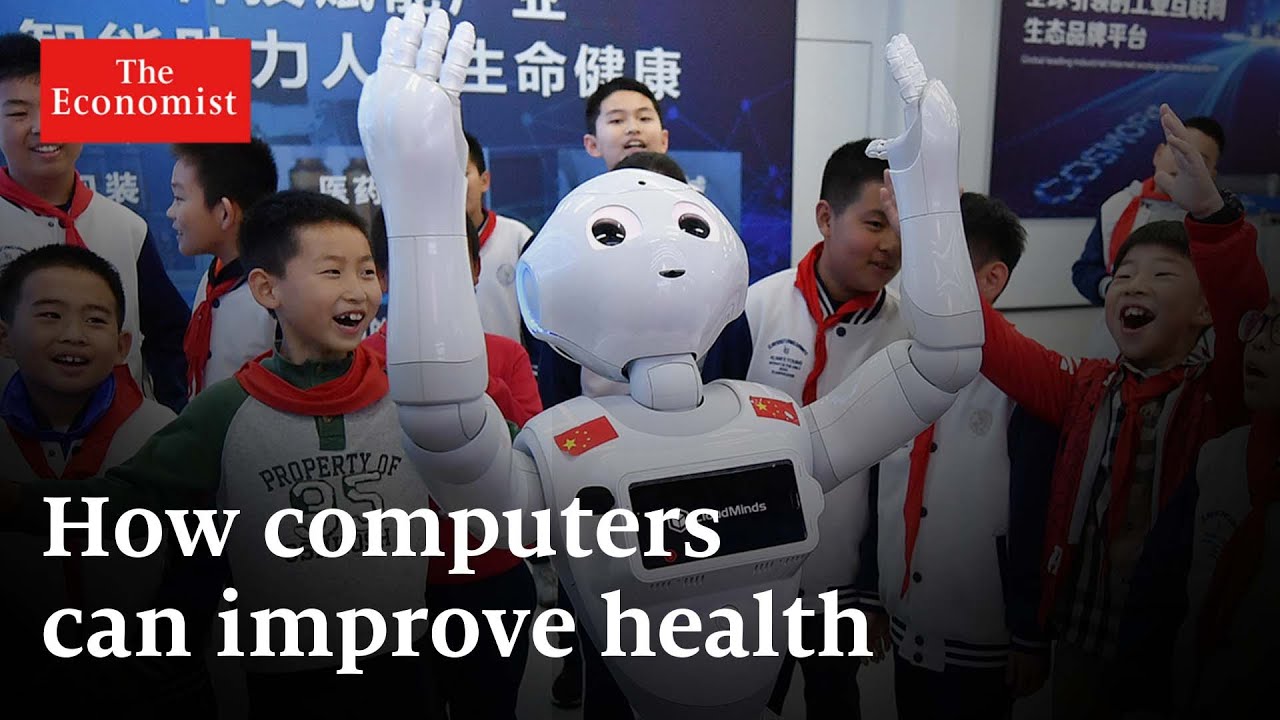
How AI can make health care better

GEF Madrid 2024: Ethical implications of AI
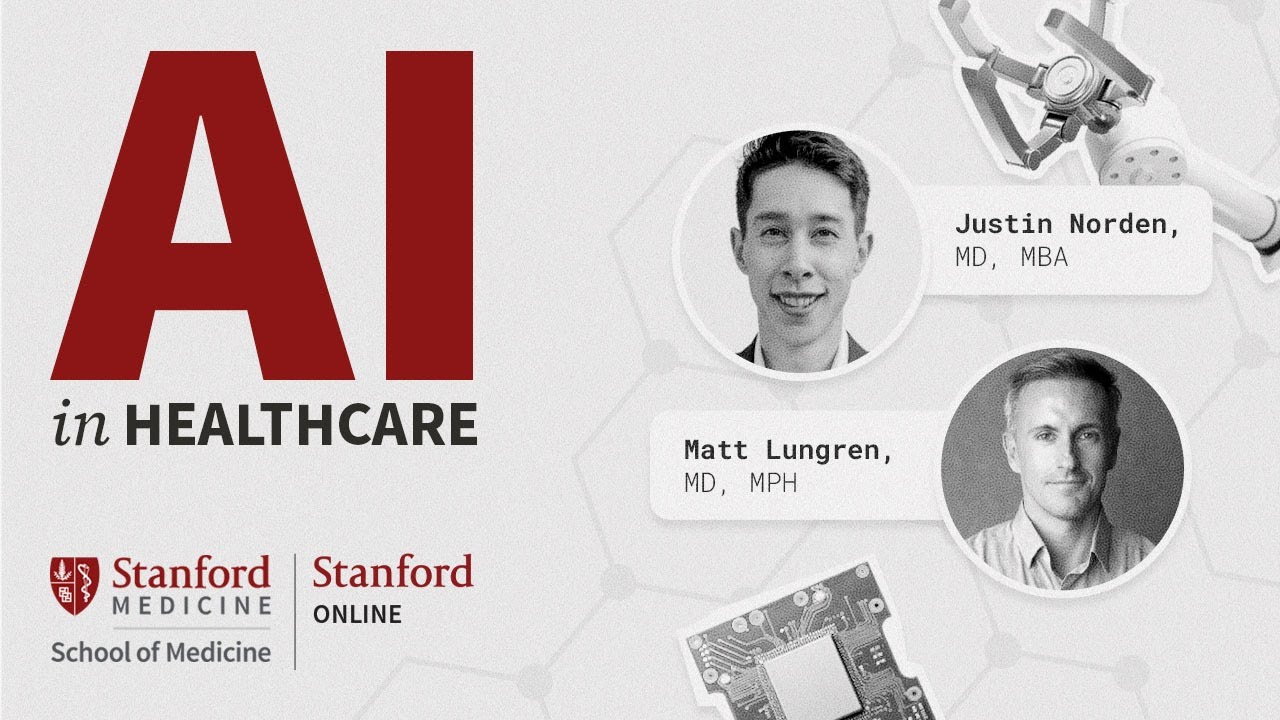
AI in Healthcare Series: State of Gen AI in Healthcare, Troy Tazbaz Former Head Digital Health FDA
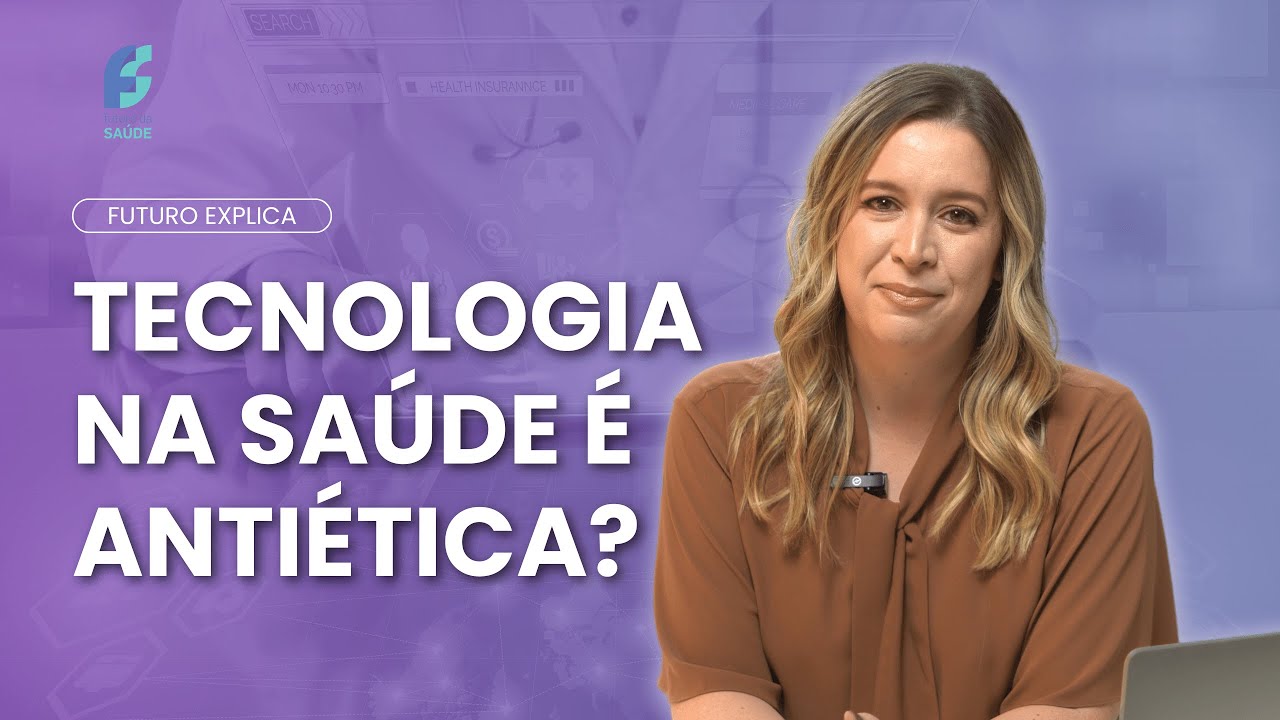
Os limites éticos da tecnologia na saúde
5.0 / 5 (0 votes)