What is Descriptive Analytics? - Data Science Wednesday
Summary
TLDRIn this video, Tessa, a data scientist at Decisive Data, explains the importance of descriptive analytics in business decision-making. Using the example of a grocery store optimizing shelf space, she discusses how descriptive analytics helps identify top and least-selling products. Tessa outlines the four essential steps for creating effective dashboards: cleaning, relating, summarizing, and visualizing data. While not considered traditional data science, descriptive analytics provides critical insights that enable businesses to make informed decisions and drive optimization. The video highlights how descriptive analytics is a key first step before advanced analytics can be performed.
Takeaways
- π Descriptive analytics serves as a launching pad for advanced analytics like predictive, prescriptive, and diagnostic analytics.
- π In the grocery store example, descriptive analytics helps optimize shelf space by identifying top and least-selling products.
- π Descriptive analytics typically involves using dashboards in tools like Tableau or Power BI to drill into data and answer business questions.
- π An interactive dashboard allows users to click through to understand specific product performance and drive decision-making.
- π Key components of building a good dashboard include cleaning, relating, summarizing, and visualizing data.
- π Cleaning data involves addressing issues like inconsistent text, invalid dates, and improperly handled null values to ensure accurate reporting.
- π Relating data helps connect various tables and datasets, such as linking Captain Crunch to the pantry category in the dashboard example.
- π Summarizing data allows for a broader view of performance, such as viewing sales over a month or by category, rather than focusing on individual transactions.
- π Visualization is the intersection of technical data work and business decision-making, requiring clear and accurate presentations for non-technical users.
- π Descriptive analytics answers questions that describe a business, often involving data preparation and creating insightful visualizations.
- π Effective descriptive analytics is crucial for businesses to make informed decisions and optimize operations, such as inventory management and revenue maximization.
Q & A
What is the main topic of the video?
-The main topic of the video is descriptive analytics, which is discussed as a necessary starting point before diving into advanced analytics in data science.
Why does the speaker mention descriptive analytics even though it's not considered 'data science'?
-Descriptive analytics is emphasized because it is a crucial foundation for understanding data before proceeding with more advanced predictive, prescriptive, or diagnostic analytics.
What example is used to explain descriptive analytics?
-The speaker uses the example of a grocery store owner wanting to optimize shelf space by identifying top-selling and least-selling products through descriptive analytics.
How does descriptive analytics help in the grocery store example?
-Descriptive analytics helps the store owner identify which products are selling well and which are not, allowing for better decisions on product placement and inventory management.
What tools are commonly used for descriptive analytics visualizations?
-The speaker mentions tools like Tableau and Power BI as common platforms used for creating interactive dashboards for descriptive analytics.
What are the four key steps in building a dashboard for descriptive analytics?
-The four key steps mentioned are cleaning, relating, summarizing, and visualizing the data.
What does data cleaning involve in the context of descriptive analytics?
-Data cleaning involves handling dirty data, such as correcting text formatting issues, managing inconsistencies in dates, and properly addressing missing or null values, to ensure accurate reporting.
Why is relating data important in descriptive analytics?
-Relating data is important because it allows different data points, such as product names and categories, to be connected properly, ensuring that the data in the dashboard is accurate and meaningful.
What is the purpose of summarizing data in descriptive analytics?
-Summarizing data helps provide an overview of trends, such as sales over a month or across product categories, rather than focusing on granular, day-to-day data.
What role does visualization play in descriptive analytics?
-Visualization helps in presenting the summarized and cleaned data in an accessible and actionable way, bridging the gap between complex data analysis and business decision-making.
Outlines
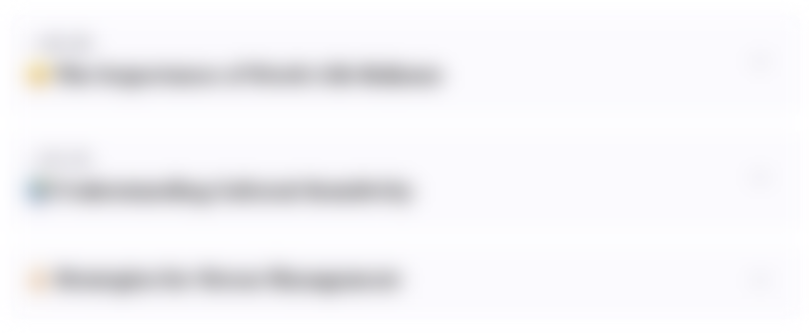
This section is available to paid users only. Please upgrade to access this part.
Upgrade NowMindmap
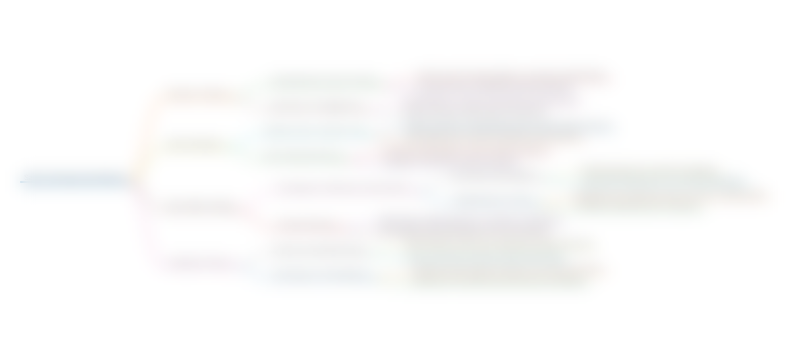
This section is available to paid users only. Please upgrade to access this part.
Upgrade NowKeywords
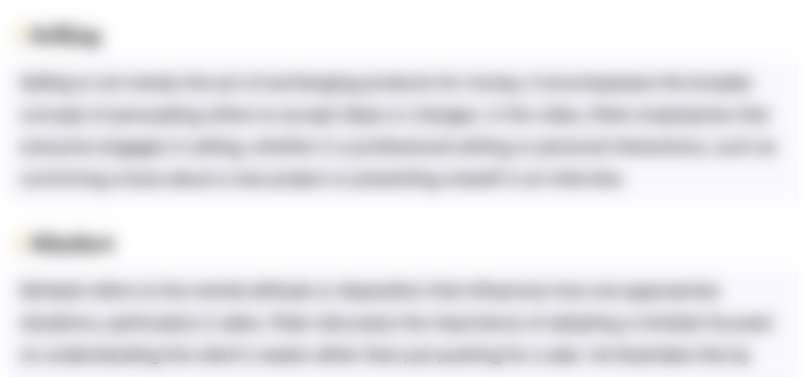
This section is available to paid users only. Please upgrade to access this part.
Upgrade NowHighlights
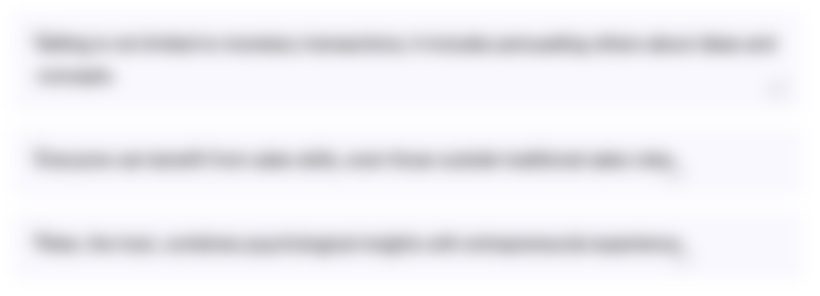
This section is available to paid users only. Please upgrade to access this part.
Upgrade NowTranscripts
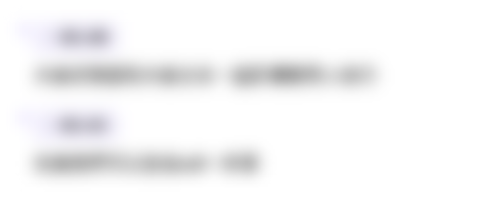
This section is available to paid users only. Please upgrade to access this part.
Upgrade NowBrowse More Related Video

Types of Business Analytics (Descriptive, Predictive, and Prescriptive)
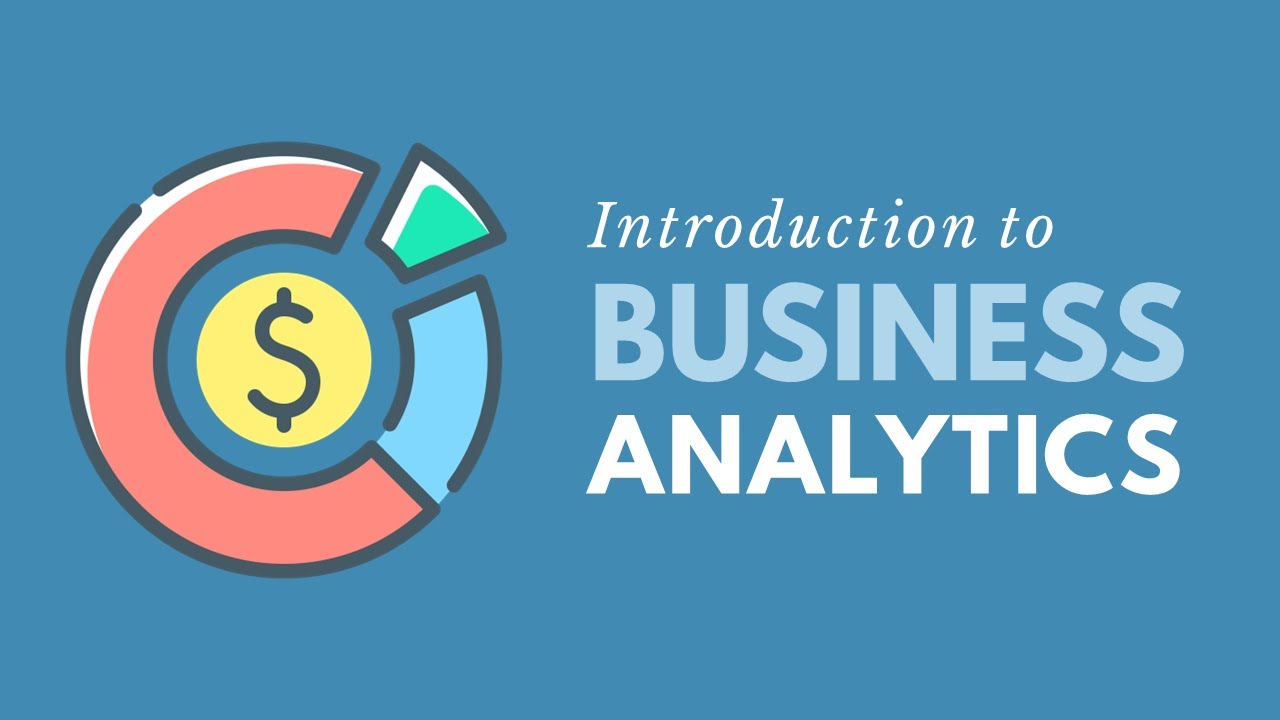
Introduction to Business Analytics (Updated Edition)
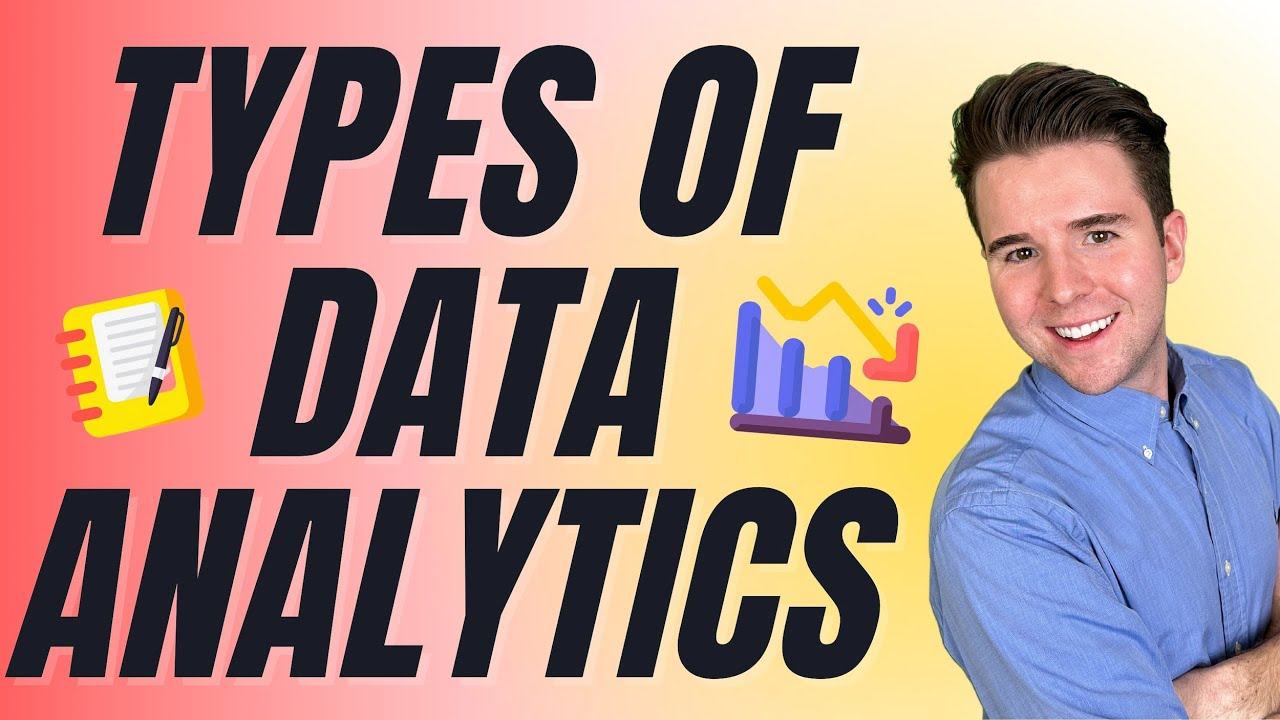
Types of Data Analytics
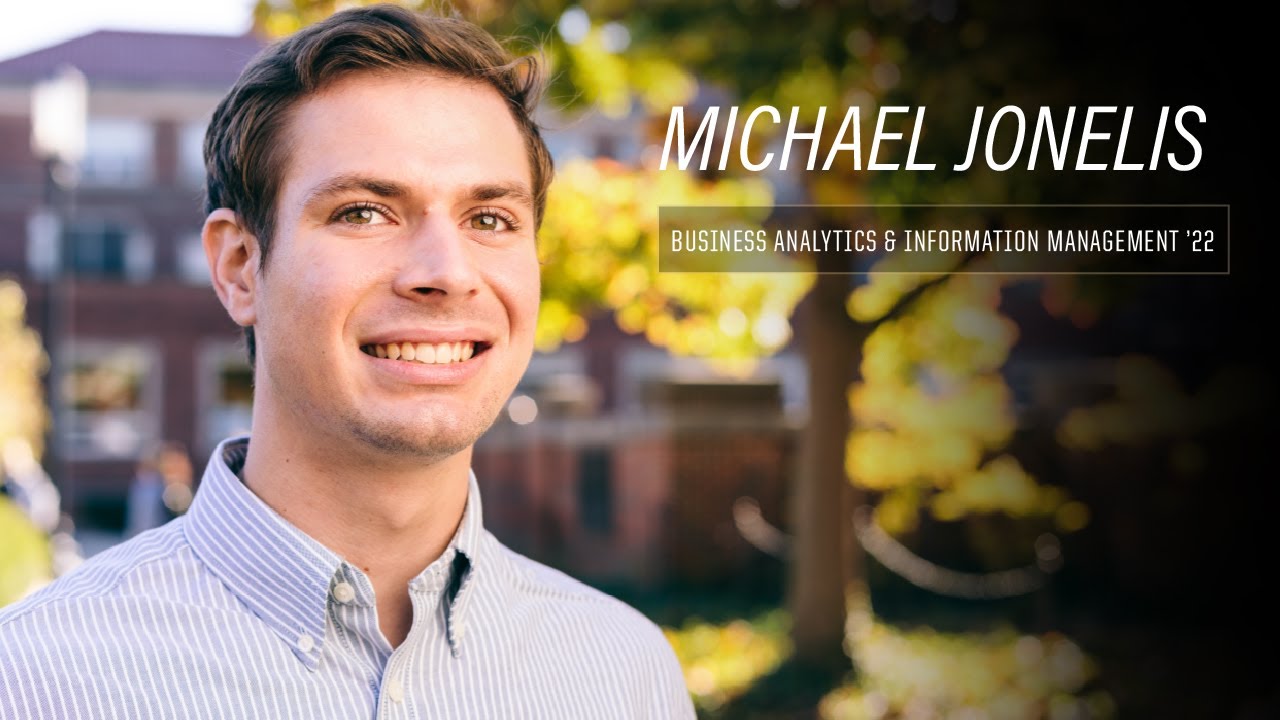
My Experience as MS Business Analytics & Information Management Student at Purdue | Michael Jonelis

Understand Prescriptive Analytics in 20 Minutes

Pengantar Statistika
5.0 / 5 (0 votes)