Max Tegmark | On superhuman AI, future architectures, and the meaning of human existence
Summary
TLDRThe speaker reflects on their journey from pondering the cosmos to delving into artificial intelligence and neuroscience at MIT. They draw parallels between the unexpected advancements in AI and the history of flight, emphasizing the importance of humility in the face of rapid technological progress. The discussion explores the potential of AI to revolutionize education, the need for safety standards in AI development, and the future where AI could help solve humanity's most pressing issues. The speaker advocates for a future where humans remain in control, with AI as a tool for societal betterment, and stresses the importance of creating meaning in a universe that doesn't inherently provide it.
Takeaways
- 🌌 The speaker's lifelong fascination with the mysteries of the universe and the human mind led them to a career in AI and neuroscience research at MIT.
- 🤖 The rapid development of AI, particularly in language mastery, has surprised many experts, highlighting the importance of remaining humble and open to unexpected advancements.
- 🔄 The speaker compares the evolution of AI to the development of flight, suggesting that sometimes simpler solutions emerge before fully understanding complex biological counterparts.
- 💡 AI research can benefit from a broader perspective that includes both the vastness of cosmology and the intricacies of the human brain.
- 🔗 The speaker believes that future AI advancements will likely incorporate elements from the brain's structure, such as loops and recurrence, which are missing in current transformer models.
- 🧠 Human brains are efficient, using significantly less power than current AI data centers, indicating a need for more efficient AI models that can learn from fewer examples.
- 🔑 The speaker suggests that the key to creating superhuman AI lies in combining neural networks with symbolic reasoning, similar to human cognitive processes.
- 🌐 AI's ability to make analogies and generalize from one domain to another is a significant area of research, with implications for how models understand and translate knowledge.
- 🚀 The potential for AI to revolutionize education by personalizing learning and providing deep insights is an exciting prospect for the future.
- ⚠️ There is a call for safety standards and regulations in AI development to ensure that powerful technologies are used responsibly and ethically.
- 🛠️ The speaker emphasizes the need for AI systems to be more interpretable and trustworthy, suggesting that AI could help us understand itself through automated reasoning and symbolic regression.
Q & A
What were the two biggest mysteries that inspired the speaker's career?
-The speaker was inspired by the mysteries of the universe and the universe within the mind, which refers to intelligence and the workings of the human brain.
How has the speaker's research focus evolved over time?
-The speaker started with researching the outer universe and then shifted their focus to artificial intelligence and neuroscience, conducting research at MIT for the past eight years.
What comparison does the speaker make between the development of AI and the history of flight?
-The speaker compares the unexpected advancements in AI to the development of flight, noting that just as mechanical birds were not necessary to invent flying machines, understanding the human brain may not be necessary to create advanced AI.
What does the speaker think about the current state of AI and its potential future developments?
-The speaker believes that current AI technologies, like transformers, will be seen as primitive in the future, and that we will develop much better AI architectures that require less data, power, and electricity.
How does the speaker view the relationship between the human brain and AI systems?
-The speaker suggests that while AI systems like transformers are powerful, the human brain operates differently, using loops and less data. They believe future AI will incorporate elements of how the brain works.
What role do analogies play in the development of AI according to the speaker?
-Analogies are crucial for both human reasoning and AI development. They allow AI models to derive higher abstractions and perform transfer learning between different domains.
How does the speaker perceive the future of AI in terms of its ability to supersede human knowledge?
-The speaker is confident that AI will not only match but surpass human knowledge, especially with its ability to draw analogies across different disciplines and integrate vast amounts of data.
What potential changes in AI architecture does the speaker foresee?
-The speaker anticipates a shift from the current focus on transformers to new AI architectures that may be more efficient and powerful, possibly incorporating symbolic reasoning and other elements of human cognition.
What is the speaker's perspective on the integration of AI with tools and its implications for the future?
-The speaker sees the integration of AI with tools as a way to enhance AI capabilities, allowing AI to perform tasks and make decisions that combine the strengths of both AI and traditional software systems.
How does the speaker view the role of AI in education and knowledge dissemination?
-The speaker believes AI can revolutionize education by providing personalized learning experiences, understanding students' knowledge gaps, and presenting information in engaging ways.
What is the speaker's stance on the importance of safety and ethical considerations in AI development?
-The speaker emphasizes the need for safety standards and ethical considerations in AI development, advocating for regulations similar to those in other industries to ensure the responsible advancement of AI.
What historical event does the speaker draw a parallel with the current state of AI development?
-The speaker compares the current state of AI to the moment when the first nuclear reactor was built, suggesting that significant advancements and potential risks are on the horizon.
What does the speaker believe the best-case scenario for the future of AI looks like?
-In the best-case scenario, the speaker envisions a future where humans are still in control, major problems like famine and wars are solved, and AI is used responsibly for the betterment of society.
Outlines
🤖 Journey from Cosmology to AI and Neuroscience
The speaker reflects on their early fascination with the mysteries of the universe and the human mind, leading to a career that began with cosmology and transitioned into artificial intelligence and neuroscience research at MIT. They highlight the rapid advancements in AI, particularly in language and knowledge mastery, drawing parallels to the early days of flight. The speaker emphasizes the importance of humility in the face of technological progress and the potential for AI to evolve beyond current understanding, much like the transition from mechanical birds to flying machines.
🧠 The Future of AI Architecture and Learning from the Brain
The speaker discusses the limitations of current AI models, such as transformers, and suggests that future architectures will likely incorporate elements from the human brain, such as loops and recurrence, to improve consciousness and learning. They argue that AI can learn from fewer examples and with less energy than current systems, and that while understanding the brain is beneficial, it's not necessary to replicate its complexity. The speaker also touches on the potential 'missing ingredients' for AI to perform more advanced reasoning.
🔗 The Power of Analogies in AI and Human Reasoning
The speaker explores the concept of analogies in AI, drawing a comparison between the human brain's use of symbols and languages for reasoning and the way AI models can learn and transfer knowledge between different domains. They discuss the importance of pattern recognition and how AI systems can discover and apply these patterns to answer novel questions, even without explicit training. The speaker also delves into the idea of AI systems superseding human knowledge by drawing analogies across various disciplines.
🚀 The Potential of AI as Autonomous Agents and Tools
The speaker predicts that AI will evolve from language models to autonomous agents capable of performing tasks and making decisions to achieve goals. They discuss the potential for AI to use tools and databases effectively, similar to how humans use calculators and other aids. The speaker also considers the implications of AI in education, suggesting that AI could revolutionize teaching by deeply understanding students' knowledge and misconceptions, thus providing personalized and effective learning experiences.
🛠 The Path to Self-Improving AI and its Societal Impact
The speaker addresses the gradual transition of AI towards self-improvement, using the analogy of technological progress and productivity growth. They discuss the current state of AI development, where humans are still involved in the loop, but foresee a future where AI systems become increasingly independent. The speaker also emphasizes the importance of safety and transparency in AI, advocating for precautionary measures and responsible development to ensure AI is used for the greater good.
🌐 The Vision for a Safer and More Transparent AI Future
The speaker recounts the 2015 AI Safety Conference, which aimed to bring together leading figures in AI to discuss safety and ethical considerations. They highlight the importance of having a proactive conversation about AI's impact on society and the need for research into making AI safe and controllable. The speaker also reflects on the collective responsibility to shape the future of AI and the potential for a few dedicated individuals to make a significant difference.
💡 The Search for Novel AI Architectures and Innovations
The speaker advocates for thinking beyond the current transformer models and exploring entirely new AI architectures that could lead to more efficient and powerful systems. They draw parallels to historical shifts in computing architecture and suggest that AI could discover these innovative architectures, leading to significant advancements in AI capabilities with less hardware and energy consumption.
🔍 The Quest for Interpretability and Trust in AI Systems
The speaker discusses ongoing research aimed at making AI systems more interpretable and trustworthy. They describe their work in symbolic regression, which seeks to understand the complex formulas learned by neural networks. The speaker also talks about the potential for AI to help verify and prove the correctness of other AI systems, emphasizing the importance of safety and understanding in AI development.
🛑 The Call for AI Safety Standards and Ethical Considerations
The speaker compares the current state of AI to the early days of nuclear power, emphasizing the need for safety standards and ethical considerations. They argue for the establishment of regulations that would ensure AI technologies are safe before they are released to the public. The speaker also discusses the importance of balancing the benefits of AI with the potential risks and the need for a measured approach to AI development.
🌟 Envisioning a Future Where AI Contributes to Human Well-Being
The speaker paints a hopeful picture of a future where AI has been harnessed to solve some of humanity's most pressing issues, such as disease, climate change, and conflict. They emphasize the importance of creating a world where humans are still in control and where AI serves to enhance human life and well-being. The speaker also reflects on the meaning of life and the role of consciousness in creating meaning in the universe.
Mindmap
Keywords
💡Artificial Intelligence (AI)
💡Cosmology
💡Neural Networks
💡Transformers
💡Language Models
💡Consciousness
💡Recurrent Neural Networks (RNNs)
💡Symbolic AI
💡Transfer Learning
💡Self-Driving Cars
💡AI Safety
Highlights
The speaker's early fascination with the mysteries of the universe and intelligence, which directed their career path.
The unexpected advancements in AI, with chatGPT-4 being smarter than anticipated, reflecting the ease of creating thinking machines compared to understanding the brain.
The influence of cosmology on AI research, emphasizing the importance of looking for the bigger picture beyond current AI models.
The comparison between transformers and the human brain, suggesting future AI architectures may incorporate elements of how the brain works.
The potential for AI to learn from fewer examples and be more energy-efficient, taking inspiration from the human brain's efficiency.
The idea that future AI systems may combine symbolic reasoning with neural networks, similar to human cognitive processes.
The importance of analogies in AI, enabling transfer learning between different domains.
The discovery of patterns by AI systems, such as representing information in geometric patterns, contributing to their generalization capabilities.
The potential for AI to supersede human knowledge by drawing analogies across different disciplines.
The evolution of AI from language models to multimodal agents capable of performing actions to achieve goals.
The vision of using AI to revolutionize education by understanding learners' needs and tailoring teaching methods accordingly.
The concept of self-improving AI and the gradual transition towards systems that require fewer humans in the loop.
The importance of having a conversation about AI safety and the role of the 2015 AI Safety Conference in starting this dialogue.
The potential for AI to automate the process of understanding its own workings, contributing to increased trustworthiness.
The discovery of new scientific knowledge using AI, as demonstrated by the re-discovery of physics formulas and a new finding in climate chemistry.
The future potential of AI to meet safety standards, allowing for the development of more powerful and trustworthy systems.
The comparison of AI development to historical technological advancements, such as the nuclear reactor, signaling the need for caution and preparation.
The importance of establishing safety standards for AI to ensure beneficial outcomes and avoid potential risks.
Transcripts
I remember when I was a teenager out on Julittarvägen in Bromma lying in a hammock
between two apple trees. And I realized then that I just loved thinking about big questions
and mysteries. And I always felt that the, the two biggest mysteries of all were our universe
out there and the universe in here, intelligence in the mind. And, and so throughout my career I
started with a, the outer universe and worked on that a whole bunch. And then I got too excited
about artificial intelligence and neuroscience, and that's what I've been researching here
at MIT for the past eight years. And, um, it's just a crazy exciting time. You know,
just four years ago, most of my colleagues thought that, uh, we were decades away from something as
smart as chatGPT-4, because they thought we can never make machines that master language
and human knowledge until we figured out how our brain works and we're nowhere near it still
. And it turned out there was an easier way to make
machines that think, well, and this reminds me a lot of, uh, the situation with flight. You know,
in the year 1900, someone could have said, oh, we're never gonna figure out how to make flying
machines until we figured out mechanical birds. But that was completely wrong. 'cause there was a
much easier way to build machines that could fly much faster than birds even. And I think that's
where we're seeing, um, that transformers that are powering LLMs today are just incredibly simple
compared to the brain. And I, I think, um, we should be very humble and never be too confident
that something is impossible to do soon, because, um, there might be easier ways than we realized.
How did those ideas from cosmology influence your AI research?
They influenced me by, I think make me always look at the book for the bigger picture. I think today,
um, especially young people, almost put an equal sign between artificial intelligence
and artificial neural networks. Some even put an e equal sign between AI and transformers,
the particular kind of neural networks, the powers, uh, chatGPT. I think that's way too
narrow-minded. I think, uh, transformers are gonna be remembered as the vacuum tubes of AI. You know,
vacuum tubes was the first technology that really let us build electronic computers.
But then we found better ones. And I'm pretty confident that in not too many years we'll
have found much better AI architectures than what we have now. Which let you do
the same thing with much less data, much less power, much less electricity use.
Do you think the brain does something different than a next token or next word prediction?
For sure. Our brain, for example, is a, so-called recurrent neural network where information flows
around in loops. There are no loops in, in a transformer. And um, that's very interesting
actually, because one of the more detailed theories of what causes consciousness,
the subjective experiences of colors and sounds and love and so on, is that you need the loops for
it. But we can of course train recurrent neural networks also. And I suspect that the new AI
architectures, which will end up being better than today's transformers will probably combine a few
additional ingredients like this, that the brain uses, that the transformers don't. Human brains
learn from often a lot fewer examples. We need a lot less training data than the big AI systems
of today. And, uh, you see these data centers going up using many, many millions of watts.
You know, your brain uses 20 watts. So clearly we have, we can get more ideas from the brain,
but we don't have to totally understand how the brain works. When the brain was optimized
by evolution, it was constrained to only develop a biological computer
that could self-assemble and that could do it with only the most,
using the most common atoms in the periodic table. You know, really weird constraints
that engineers just don't care about. Whereas there was no limit on, there was no need for
it to be simple and easy to understand. Whereas that's where we tend to be limited by engineers.
And what, what do you think is these missing ingredients? So you mentioned
recurrence. What do you think is required to get these models to do more reasoning?
I'm not gonna give you a glib answer because I think, I don't know, and nobody knows exactly. But
if you very crudely think of the history of AI as two stages. Stage one was gofi, good old fashioned
ai, where you have these symbolic logic-based systems. And then stage two was self-learning
neural networks that largely crushed the old stuff. This has made us think that the neural
networks are like the better cooler one, but if you look in the live living world of animals
around us, it's kind of been the other way around. Cats and dogs and eagles have often better vision
systems or all factor systems, et cetera, than we do. What's special about humans is not that we can
see better than an eagle, it's that we can also reason with symbols and communicate with human
language and mathematical language and programming languages. And somehow we can combine the old AI
and the new AI in a seamless way. And that's how I see the path to superhuman AI going as well.
People figure out how to take these powerful new systems and merge them with a lot of these
more symbolic techniques so that, in a way it's a bit more like how we humans do it
What's really fascinating with this is the analogies that the models are able
to take. So you train a model on code, it gets better at human language as well. And so it's
able to derive some higher abstraction, which allows it to do transfer learnings
between two entirely different domains which speaks to sort of, you know,
how important analogies are. Analogies both for the human brain, which seems to be the majority
of sort of what makes up how we reason. But equally for these models. What do you think are
some of the most interesting sort of analogies that you've come across when studying these
models? So how it's able to draw these conclusions based on derived insights from different domains?
Yeah, this is something we work a lot on in my AI research group. Because eight years ago we
switched from doing physics to doing machine learning. And we sit in this very office and,
and talk very much about these questions: How do these analogies get discovered? How
do machine learning systems generalize from one thing they've learned in one
domain to other things? And we published some recent papers about it, which I find
really cool. And it's all about discovering patterns, which neural networks are very,
very good at. You feed it massive amounts of data and then it starts to see patterns like wait a
minute you know. For example, you have a large language model read all the text on the internet,
and then it realizes that actually all these places, it makes more sense if it chooses to
represent them in a two dimensional space, a map, even if it's never seen a picture of a map.
And then we wrote a paper where we looked inside Llama 2, and there is a map sitting there.
Literally where Stockholm is here and Göteborg is there. And once it's realized that it can
represent things like this, it can now start answering questions that it never saw in the
training data. Like if you ask: is Göteborg west of Katmandu? You know, it probably never saw that
question, but it has a map. It represented it this way because to answer some other questions, right?
And now it can figure these things out. We see same things when translating. For example, if you
look at how it represents words, it puts them in a sort of high dimensional map, word embeddings.
And if you take, there was a nice paper recently where someone took the word embedding of English
words from reading a bunch of English text and word embeddings, and an Italian one from reading a
bunch of different Italian texts. And figured out how do you need to rotate and shift these things
so that they kind of match well?
And they got out a pretty good English Italian dictionary just from these matching up these
patterns. So I think these AI systems are learning a lot of these geometric
patterns and that's one of the key reasons that they're often able to generalize and
actually answer whole new questions that you have never, that they've never been trained on.
. That's, that seems to be the way that
which these models could also supersede current human knowledge, where they're able to draw these
analogies, from very different disciplines in a way which would've been infeasible for humans.
Maybe you're clever enough to sort of master all human knowledge, but it seems like that's quite
a difficult thing to do today. But these models can not only do that, but they can even fit it
into their short term memory soon with like the context windows. So what, what's your perspective
on that? In these model's ability to supersede the current human knowledge to go well beyond it?
Yeah, I think it's absolutely going to happen. No doubt about that. And I mean, if,
if you and I could just read all the Internet and everything written in all the languages somehow,
and keep this in our minds, I'm sorry I cannot, we would see a lot of patterns too. And when
I talk to colleagues of mine who are working on training these models, they tell me themselves,
they were pretty shocked. Like, oh my gosh, this thing can translate English to Chinese.
We never taught it to do that. Oh my gosh, it can code in Python. Like how did it figure that
out? And what I think we might be seeing a lot of in 2024 is patterns and connecting things,
not just in terms of the basic knowledge, but also in terms of using tools.
So right now these LLMs tend to output tokens, new word or pieces of words, but people are realizing
you can also give them the option to output commands, to a calculator that can do really good
math, or to a dictionary or to all sorts of other tools or to a database. And this is something we
humans of course, do routinely. If you're driving, you know, sending almost becomes like an extension
of your body. This can, this can start to bridge and overcome a lot of the intrinsic weaknesses
in large language models. Because we have all these old fashioned AI systems that can make very
powerful tools. So if the LLM learn to use them, then they can get the best of both worlds. This
is also very powerful for building robots, whether they be robots on wheels like self-driving cars or
more humanoid ones, where it can fundamentally be controlled to some level by a large language
model that then some of the tokens and outputs are just motor commands and things like that.
And what do you think is the right way of looking at these models? Because, you know,
initially they were language models, but now they're increasingly multimodal. Would
you say they're sort of a compression of all the world's knowledge? Are they
token predictors? Or what's the right way of viewing the current state of these models?
It's pretty diverse. I think it's almost easier to look at the endpoint of where this is going rather
than trying to classify exactly what happens right now. There's a huge commercial pressure to build
systems that are agents. traditional LLMs are more like an oracle. You ask it a question and it gives
you an answer. But there's so much commercial value to have agents which will actually do stuff,
take information and figure out what actions to take to accomplish some goal and then go
out and do it. And I think 2024 will probably also maybe be remembered as the year of the
agents when we start seeing a lot of more autonomous systems. First purely software
ones on the internet. And then gradually we'll get more and more physical agents too. This can
of course be great for many purposes, but this is also obviously something we have
to be careful with. Because if we have a lot of autonomous systems act in the world, you know,
it starts to feel less like just a new technology like electricity and more like a new species.
Do you think that we could radically accelerate the rate at which we discover
new science? And if we do that, what could that mean for the world?
It could be amazing. I mean, I really like the vision of your company to accelerate the growth
of knowledge and make it widely accessible to everybody. This is to me one of the coolest
things in the history of life on Earth that we've gone from being so disempowered, you know,
30 year life expectancy, knowing very little about what was going on, to developing science
over thousands of years, becoming ffirst more knowledgeable about what's actually happening.
And then through this knowledge, also developing technology that put us more in charge and let us
control our destiny. Sort of be the captain of our own ship. So I very much applaud this. I think if
you can have AI systems that can get very deep insights and very broad knowledge themselves,
clearly that can revolutionize education. You know, my job is not just out of a researcher, even
though that's what I spend most of my time on here in this office, but also very much as an educator.
And to me, the first thing I always have to do before I start teaching something is to make
sure I really have a deep understanding of it. Much deeper than what the students need to get
later. And then I need an understanding also of the students, where are they? That's also
something AI can get better at. Understanding what the people who are trying to learn,
actually know already and what they don't know and what their misconceptions are. And then
finally, I cannot tackle this question. What is the most helpful way for me, you know,
to convey this knowledge? Where do I start? What are the metaphors and analogies that are
gonna work? And how should I present this so that they keep being motivated and are curious
to learn more. I think AI can really help revolutionize education in the broad sense
of the word. I certainly love the idea of talking to some real expert in something
who's willing to give me some of their time and answer questions in a way that makes sense to
me. It's one of the great joys of working at this university. And if there are AI systems
that will play that role also and teach me things the way I will really get it, I would love that.
One of the assumptions with those sort of historical reasonings has
been that they are self-improving. The current state of these models is,
you know, you have to buy massive amounts of compute, you need to spend six months
training them. There's alot of small things that could go wrong when,
when you run these clusters. What's your intuition there? Because they're not really self-improving
now. So how could we sort of hit that escape velocity given how they're currently trained?
So I think it's a mistake to think that now over time now itself, it's not self-improving. Now
it's not self-improving and all of a sudden it is. And boom, singularity. It's a gradual transition.
Technology has always been self-improving. We always use today's technology to build
tomorrow's technology, which is why we've seen an exponential growth in productivity in technology
of most measures. But what we also see in this exponential growth, if you look at the world's GDP
over time or most measures of tech, the time it takes to get twice as good to keep shortening. So
it's actually growing faster than exponentially. And today, you have must have a lot of people
doing coding in your company right who are using autopilot of some form. I don't know
if it's making them twice as productive or 1.5 times or three times as productive, but
this is already an example of how AI is enabling AI development to go faster.
So it is a kind of recursive self-improvement, but there's still humans in the loop. Iit's just
that over time we get less and less humans in the loop. When we had farming before,
like almost all people in Sweden were in that loop, farming. Now it's like 1% in that loop.
Similarly with software development, there'll gradually be fewer and fewer. If you look at
a car factory today, there are way fewer people per car produced than there were 50
years ago. So I think that's how it's gonna go. We'll see that, uh, you need fewer and
fewer and fewer humans in the loop. At some point there might be no human in the loop,
and then things will take off even faster. But you're going to start noticing the approach.
Why do you have such a strong bias towards humans compared to the AI models?
Because I am a human, you know, I have a lovely little 1-year-old Leo. And when I
look into his eyes, you know, I feel he's my son. Of course,
I should feel more loyal to him than to some random machine, shouldn't I? And I
feel we humans have figured out collectively how to start building AI. And so I think we
have the right to have some influence over what future we build. I'm on Team Human here
not on Team Machine. I think we want to have the machines work for us, not the other way around.
I saw this awesome picture from the 2015 AI Safety Conference that you organized.
Can you tell us a bit more about who was there and what you talked about?
Oh yeah. That was quite fun. Kinda like approaching the 10th anniversary now.
Since I like to think big, I felt it was really important to start having a conversation with
all the leading players on how we can make AI get used for good things rather than for bad things.
And I felt the situation was totally dysfunctional in 2014 because on the one hand you had a small
group of people who were worrying about building smarter than human AI that we would lose control
over. And then on the other hand, there were other people actually driving the AI development in the
big companies and in academia who had never really talked with those people who were worried and felt
that those are just a bunch of weirdos, maybe they should ignore them because it could be
bad for funding. And so I had this vision that we should actually try to bring them together.
And, you know, one thing that Sweden is strangely good at is throwing good parties
from the Nobel Fest to the more informal ones. So I pulled out all the stops to try to entice
people to come to this conference. Put it not in Sweden, but in Puerto Rico
in January, I sent out a invitation with a picture of a guy digging his car out from one meter of
snow right next to the beach by the hotel and be like, where would you rather be in January of
2015? And I went first after the most high profile people I thought maybe I could actually persuade.
And then when you get them, you know, you can start building a Swedish snowball that rolls
down the hill and you get more. And in the end we got amazing people. We got Demis Hassabis, the
CEO of DeepMind, we got Elon Musk, we got really top professors from academia and also all the top
people who had been worrying, you know, Elliot Rutkowski, to Nick Bostrom and so many others.
And to make sure they didn't kill each other. We had a lot of wine and it was very pleasant. And I
was really happy with how this ended up. Everybody came together and signed this statement saying,
yep, the risks are real. Let's deal with them. Let's do research not just on how to make AI more
powerful, but also on how to make it safe and transparent and controllable. Elon Musk said,
okay, I'll give you 10 million to do a grants program to start getting a bunch of nerds
working on this. So we launched that and pretty quickly this field stopped being taboo to talk
about AI safety and the technical AI conferences would start having the nerd sessions basically
on these topics. So that also made me realize that even very few people can often make a big
difference. Many times things that need to happen don't happen just because of the bystander effect,
you know? And I think that's a message to anyone listening to this. If they have an
idea for a new startup or just some new social movement or anything, and they're like, ah,
this must be impossible because otherwise someone else would've done it. No, it might
very well be that nobody else actually tried very hard yet.
What were some of the things that people disagreed with the most at the conference?
I mean, there were big differences in the forecasts people had for how long
is it gonna take to get smarter than human AI? There were also big disagreements whether people
thought it would probably be fine or great, or whether it would probably suck. But everybody
pretty much agreed that, you know, it might happen and it might happen soon. So you know,
with that humility, it makes sense to take some precautions. Even the people who thought it was
very unlikely that humanity would go extinct, you know, these are people who still buy fire
insurance on their house. That doesn't mean they think their house is probably gonna burn down,
but just in case, you know, why not prepare a little bit, maybe have also put in a smoke
alarm and have a fire extinguisher handy. And, you know, given the enormous amounts of money
we're spending right now on training ever bigger models and making AI more powerful,
it's pretty reasonable, even for people who are sort of skeptical of the risks to say, well,
let's spend at least some substantial amount also on figuring out how to make these systems safe.
And one of the best boxes you have in your office is the bananas box. What do you think is some
of the most bananas ideas that could work that we are not paying enough attention to? For AI?
Generally
I think for AI in particular, if there is something really bananas that we're not
paying enough attention to, it's probably that we're thinking too small in terms
of architecture design. We are looking at the transformer and thinking about like minor tweaks,
but there could easily be completely different types of architectures. Even just look at the
history of computing, how many times we've had a quantum leap in architecture. First Alan Turing
and Charles Babbage and so on, they were like thinking about mechanical computers and there
was a pretty big shift to go from that to starting to do electronic ones like eniac and and so on.
And those computers, which is like when I was a teenager and first learned to code, you know,
that was again, a completely different paradigm of computation where you program everything in
and compile it into machine code than a neural network that just teaches itself.
Our brain, you know the solution that biology came up with is, again, very,
very different. And if you just take your step back and say, I'm gonna give you a big
blob of atoms, you know, what's the best way to arrange these to be really smart? You know,
it might be something we haven't thought about at all. And the neat thing is if we start getting
ever more powerful AI to the point where we can use AI to figure out how to make much better AI,
it will probably discover those really, really clever things. So my prediction is actually that
even now we're getting these evermore ginormous data centers the size of an airplane hangar where
you have to almost put a nuclear reaction next to it soon to power the whole thing. That's just
temporary. I suspect that we're overcompensating for a really poor software architecture with these
ridiculous amounts of hardware and training data. Once we get over that hump, we'll realize that
you could do it all with much less hardware, much less energy and much less training data.
I mean, it's quite absurd that we weren't using like mixture of experts and so on in
the prior design of some of these models and, and also that we weren't curating
the data sets sufficiently. What do you think are the most promising,
like new architectures, that you've seen that you think we should be exploring more?
So I mentioned tool use by LLMs. I think more generally what's very promising is
scaffolding. Where you think of the LLM as just one component in a bigger
architecture. Kahneman who sadly passed away around age 90 just very recently,
used to talk about System One and System Two in our human brains. System One, the fast thinking,
the intuitive thinking is a lot like neural networks where System Two is the more logic
based, symbolic reasoning we have which lets us speak Swedish and English and math and Python.
If you think about a future where the neural networks, the transformers say is System One,
you can imagine that it's just part of this bigger architecture which can enable clever, more clever
database structures, all sorts of tools, loops, various other techniques. I could totally see
this kind of neural network scaffolding around it being way more powerful than today's systems.
Do you think it's necessary to change the underlying model architecture or can this
be a heuristic on top? I mean, we already see that with sort of the chainof thought
and similar approaches where you sort of add reasoning on top of the current model.
So instead of stopping them as soon as they start outputting tokens,
you let them set up a plan and then recent sort of recurrently in that way.
I think you're already seeing more things like this. So the quiet star paper that just came out,
for example. A radical interpretation of it is that it's stupid to force the neural network
to output one token, to basically say everything that it thinks. That's not how you operate. When
you speak, sometimes you'll have several thoughts before you decide to say the next word, right?
So the quiet star architecture has more of that freedom and it performs way, way, way better. But
that's just a very small example of how messing a little bit with the architecture can make big
improvements. And I'm fairly confident we're gonna see huge improvements in the next year or two.
Max, you've outputted some really interesting
stuff in the research around symbolic regression. What's the latest there?
So the technical research we focus on in my group is very much about taking a black box
AI system that's doing something intelligent and automating the process of figuring out what it
works, how it works, so we can make, see how trustworthy it is and hopefully make it even
more trustworthy. So the simplest example of this is if you have a neural network,
which is just computing some function of some sort that it's learned somehow from data. The task of
figuring out what the formula actually is that it's learned called symbolic regression. If it's,
for the nerds who are listening to this, if it's a linear formula, it's just linear regression which
is super easy. But if it's some complicated formula, like some of the physics formulas
there on my, by my window, it's generally believed to be np hard. It could take longer
than the age of the universe just because there's exponentially many formulas that are of length.
And, but, but that, that one we managed to get state of the art performance on. We used a lot
of ideas from physics where we were able to discover automatically if this neural network
is actually modular and can be decomposed into smaller pieces of two different parts. And we
actually were able to rediscover many of the most famous physics formulas just from data.
So if you could go back in a time machine, we could have scooped Einstein and others on some,
some cool stuff. And we actually even managed to very recently discover a new physics result in
in climate chemistry about ozone, which we actually got published. So that was the
first time we used these tools to advance science a little bit. But more broadly,
what we wanna do is ultimately be able to take any black box AI system and figure
out what is, what algorithm is learned and what, what knowledge it's really learned.
Our last paper on this, we was one where we, we took about 60 different algorithms and
we trained a neural network to just learn to do these tasks. So now you have a black box,
but what did it do? And then we had an automated AI system which was able to figure out exactly
what it was doing and turn it into Python code so you can like, ah, and we were quite excited
about this and we're scaling this up now in a big way, see if we can make it work for,
for bigger systems. And the vision I have here is that if you have a system, an AI
system that's gonna affect people's lives in some way where you really want to have a high trust,
then it's a really good idea if you let the machine learning do the learning,
we don't have any better way than that, but then can distill out what it's learned into Python or
something else where you can actually prove that the code meets the specs that you have.
And I fundamentally believe that it's possible because we humans can do it. You know,
like when you were a little kid, if your dad threw you a tennis ball, you could catch it because your
brain had figured out how to compute parabolic trajectories. But when you got older you're like,
oh, this is a parabola, Y equals X squared you know formula. And this is how scientists
generally first intuitively figure out stuff, even though they don't know how their brain works, and
then they learn to distill out the knowledge in a way that you could, you could actually program
into a moon rocket, et cetera. And the fact that we've managed to make so much progress on this
already and the fact that this field is rapidly growing, I organize the biggest conference so
far here at MIT on this field called mechanistic interpretability, makes me pretty hopeful actually
that we don't have to be resigned to the idea that we'll never understand AI systems, the ones
that we really want to understand, I think we can use other AI systems to help us understand them.
Will the models derive those equations or will they have a much simpler heuristic?
If you take the example, I throw a ball at you and then you're not running the sort of
precise calculations, you just have a very simple heuristic given the wins,
the size of the ball and so on. What do you think these models will do? Will they have
that simple heuristic or will they actually incorporate the exact equation as well?
Our system actually does both. So it actually finds many formulas.
We put them in a plot where on one axis is how complicated they are and on the other axis is
how inaccurate they are. And it would find, like in this case, the simplest one that is just the
parabola where there is no air resistance whatsoever, but then it would find a more
complicated one, which is more precise. And this is a lot like how we humans do it also.
And sometimes all you want is a simple one, but in the big picture,
I think there's been too much pessimism on this question about whether we'll able be able to
build systems that we can actually trust and even prove things about. Because people have
made this mistake of assuming that all the work in interpreting and proving has to be done by people.
But AI systems are getting really good at that stuff now and they can help us. And you might
think: Oh, how am I ever gonna trust a system that was produced by an AI with a proof produced
by an AI if I don't understand if both the proof and the code is too long to read? That's okay.
Because it turns out that just like it's much harder to find a needle in a haystack than it
is to prove that it's a needle once you found it, it's much harder to find the correct code
and proof that it meets your spec than it is to verify that it works once you have it. So
all you have to do is actually understand your proof checker, which can be 300 lines
of Python and now you can fully trust some very powerful systems that have been made by an AI.
And you've had a lot of awesome folks at the university and there's this one story about
Douglas Engelbart running into Marvin Minsky and Marvin Minsky telling Engelbart about all of the
things he will make computers do, they will reason and they will be conscious and so on
to which Engelbart replied: You're gonna do all of that for computers. What are you gonna do for
humans? What are some of those stories? Did you have any interactions with Minsky or other ones
this sort of OG AI folks and how did you see their perception of AI change as the field advanced?
Most top AI thinkers and business leaders that I know don't have as much time to step
back and reflect as they probably wished they did. It's tough to run a company,
for example. And I get this as many of them, most of had this idea of: Well,
first you just need to get this thing to work and then I'm gonna figure out my strategy for how to
make sure this is good for society you know. And then many of them were really taken by surprise
that chat GPTs and stable diffusion and so on came decades before many expected. Like oh my God,
now what are we gonna do about this? What I would love to see ultimately happen to
make sure we get a good future is actually to give back some time to these people.
If you look at all other technologies that have the potential to cause harm, we have a solution
for how we do it with all of them, whether it be airplanes or medicines. We always have safety
standards. You know. If AstraZeneca comes up and says: We have a new miracle drug that's gonna cure
cancer and we're gonna start selling it in ICA tomorrow, Läkemedelsverket would be like: Where is
your clinical trial? Oh you couldn't be bothered making, you haven't had time to make one yet? Just
come back when you've done the clinical trial and then we will see if you meet the standards,
right? So that buys time for everybody involved. It's the responsibility. The companies now have
an incentive to figure out what the impact on society is gonna be. How many percent of people
are gonna get this side effect, that effect, They quantify everything in a very nerdy way,
and that way we can trust our biotech. That's why ultimately AstraZeneca has
a pretty good reputation. Same thing with airplanes, same thing with cars,
same thing with basically any tech that cause harm except for AI where here in the US there's
basically no regulation. If Sam Altman wants GPT-5 tomorrow, he can, right? So I think the sooner we
switch over to treating AI the way we treat all other powerful tech, the better. I think a very
common misconception is that somehow we have to choose between quickly reaping all sorts
of benefits of AI on one hand, and on the other hand avoiding going extinct. The fact is with
99% of the things that the people I talk with are excited about, which I think includes you with AI,
are things that are quite harmless. That have nothing to do with building smarter than human
AI that we don't know how to control. We can curate, we can help spread knowledge,
we can help make companies more efficient. We can do great progress in science and medicine,
et cetera, et cetera. So if we put safety standards in place that just end up slowing
down a little bit that that last percent the stuff that we might lose control over, then we
can really have this age of abundance for a number of years now where we can enjoy revolutions and
healthcare and education and so many other things without having to lose sleep that this is gonna
all blow up on us. And then if we can get to the point eventually where we can see that even more
powerful systems meet the safety standards, great. If it takes a little longer, fine,
we're not in any great rush. We can have life flourishing for billions of years if we get this
right. So there's no point in risking squandering everything just to get it one year sooner.
You're a big history nerd. What do you think is most analogous to this in history? Is there any
historical inventions that we can learn from that will behave quite similarly?
Yeah, so in 1942, Enrico Fermi built the world's first nuclear reactor in Chicago under a football
stadium. And when physicists found out about that, they totally freaked out. Why? Was it because they
thought this reactor was really dangerous? No, it was really small, low energy output. It was
because they realized that now we're only a few years away from the bomb. And three years later,
Hiroshima, Nagasaki happened right? There's a nice analogy there because around that time in 1951,
Alan Turing said that one day machines will become as smart as people and then very quickly they'll
become way smarter than people because we're biological computers and there's no reason
machines can't do much better. And then the default is we lose control over the machines.
But I'll give you a little canary in the coal mine warning so you know when you're close. The Turing
test, once machines become good enough at language and knowledge that they can
fool a lot of people into thinking that they are human, that's when you're close.
That's the Enrico for moment when you might have a few years. And last year,
Joshua Bengio even one of the most sighted AI researchers in the world argued that GPT-4 passes
the Turing test. You can squabble about whether it's passed the Turing test or whether we'll
pass it next year. But we're roughly there at the Enrico Fermi reactor now for AI where it's high
time to take it seriously. Big things are going to happen soon and let's get it right. Let's prepare.
So one final question. We're we meet here at your campus,
you know, two decades from from now. What do you think is the best case scenario?
The best case is we're still alive and we're happy and the humans are still in
charge of this planet. Except there's no more famine, no more wars, the climate is all good,
and we have basically managed to solve the biggest problems that have stumped
people throughout the ages. That's the win case that I'm going for.
And what do you think happens once we've solved
all problems? We've found treatments to all diseases, what does that world look like?
Well, Nick Bostrom just wrote a book on exactly that, the solved world as he calls it. I'm not
spending too much time thinking about that yet. I'd rather have the luxury of thinking
about that once we've actually gone a little closer to solving it now. Right now there's
so much exciting stuff to be done. Both on the nerd side, on figuring out how to make
systems the more trustworthy, and also on the societal side of just making sure that
our politicians put good safety standards in place. So that's where I'm spending my energy.
So let's get to some of the easier questions. What what do you believe is the meaning of life?
The easy questions. You know, something we've discovered
by finding all these fundamental equations of physics is that none of these equations have
any explicit mention of meaning put into them. So I think it's rather up to us conscious living
beings to create our own meaning, frankly. I think that consciousness and positive experiences is at
the heart of this because even beauty and love and passion and kindness and hope are also conscious
experiences after all. I don't think my desk here is having any of those experiences. And in other
words, I don't think that our universe gives meaning to us. We give meaning to our universe.
Browse More Related Video
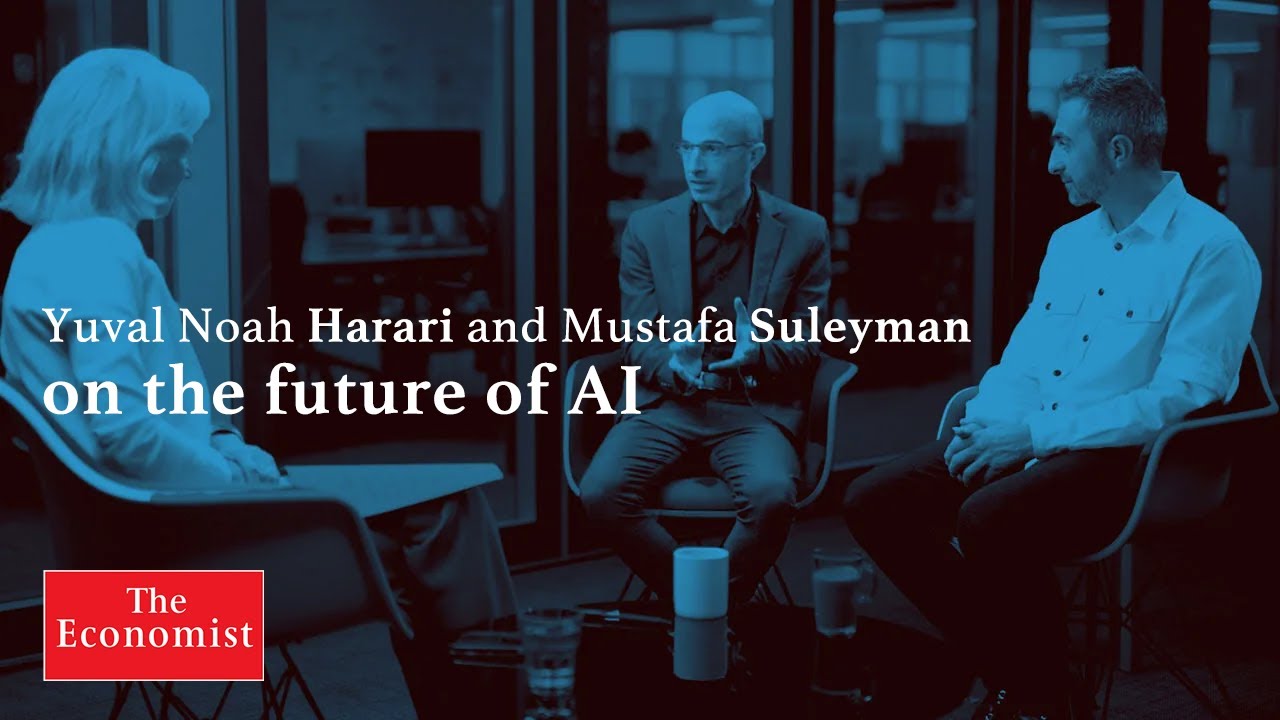
Mustafa Suleyman & Yuval Noah Harari -FULL DEBATE- What does the AI revolution mean for our future?

Algor-Ethics: Developing a Language for a Human-Centered AI | Padre Benanti | TEDxRoma
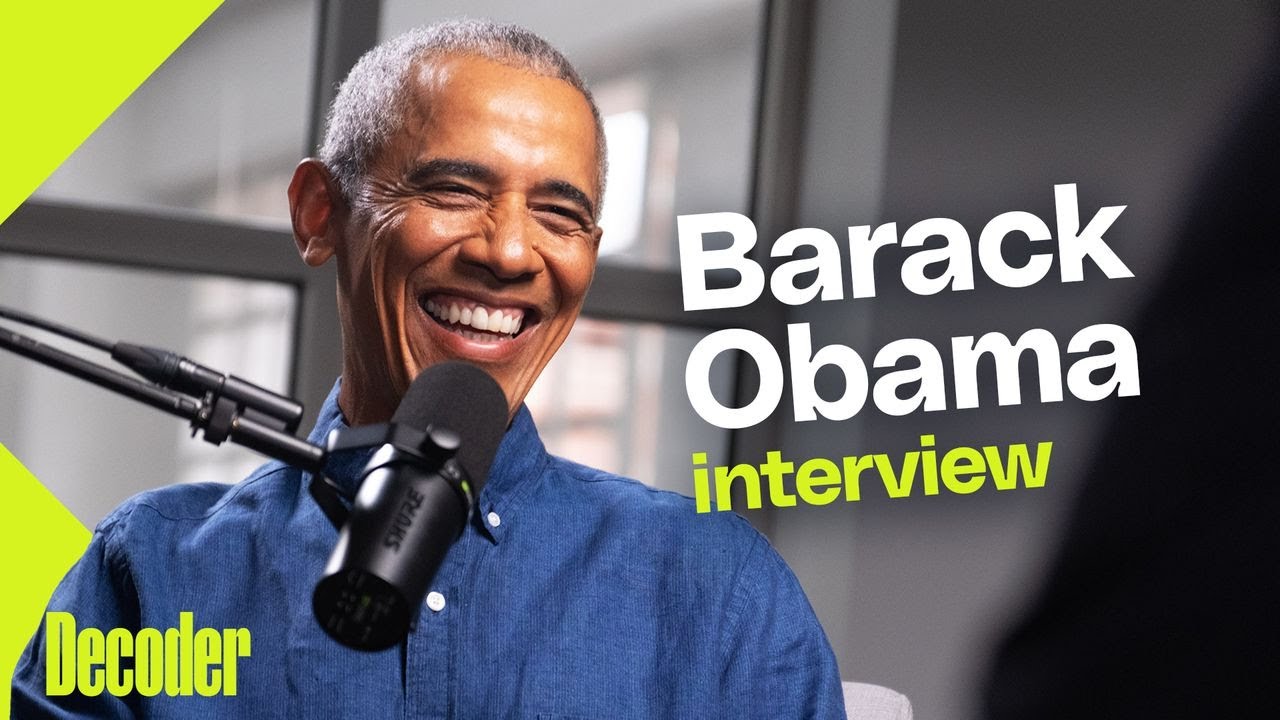
Obama on AI, free speech, and the future of the internet

Elon Musk's STUNNING Prediction | Sam Altman Attempts to Harness the Power of a Thousand Suns.
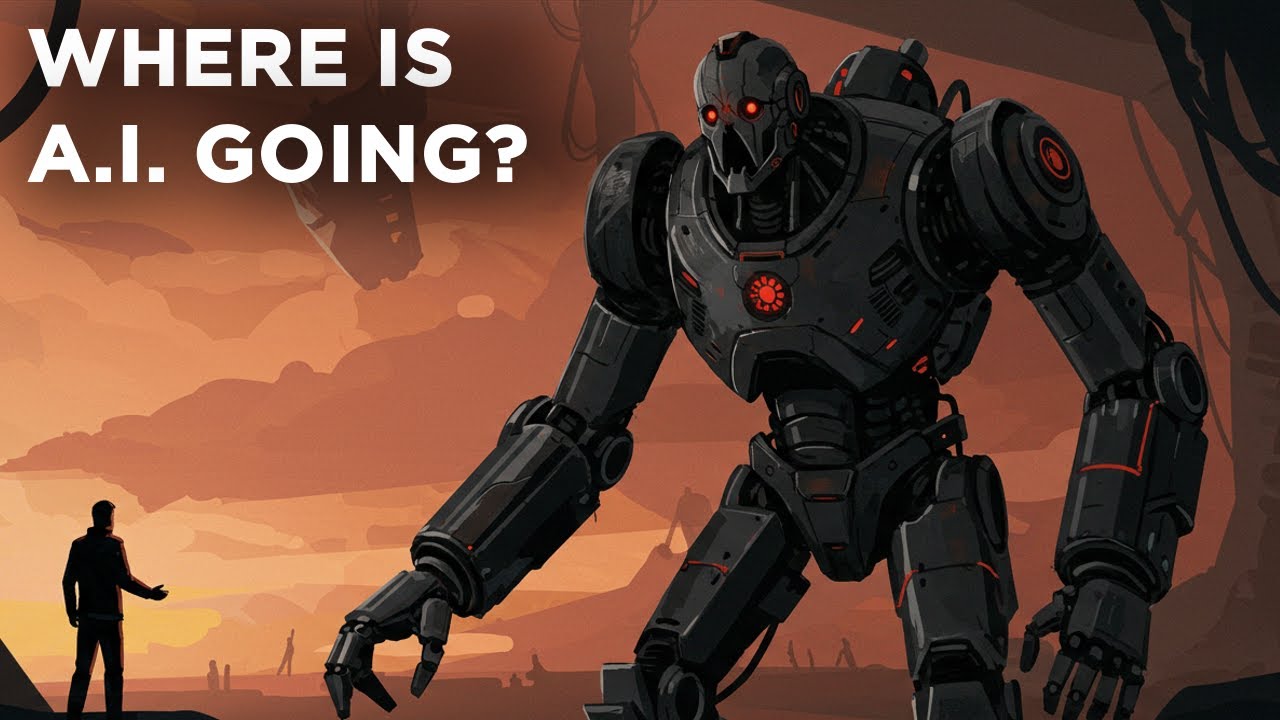
The Future of Artificial Intelligence (Animated)
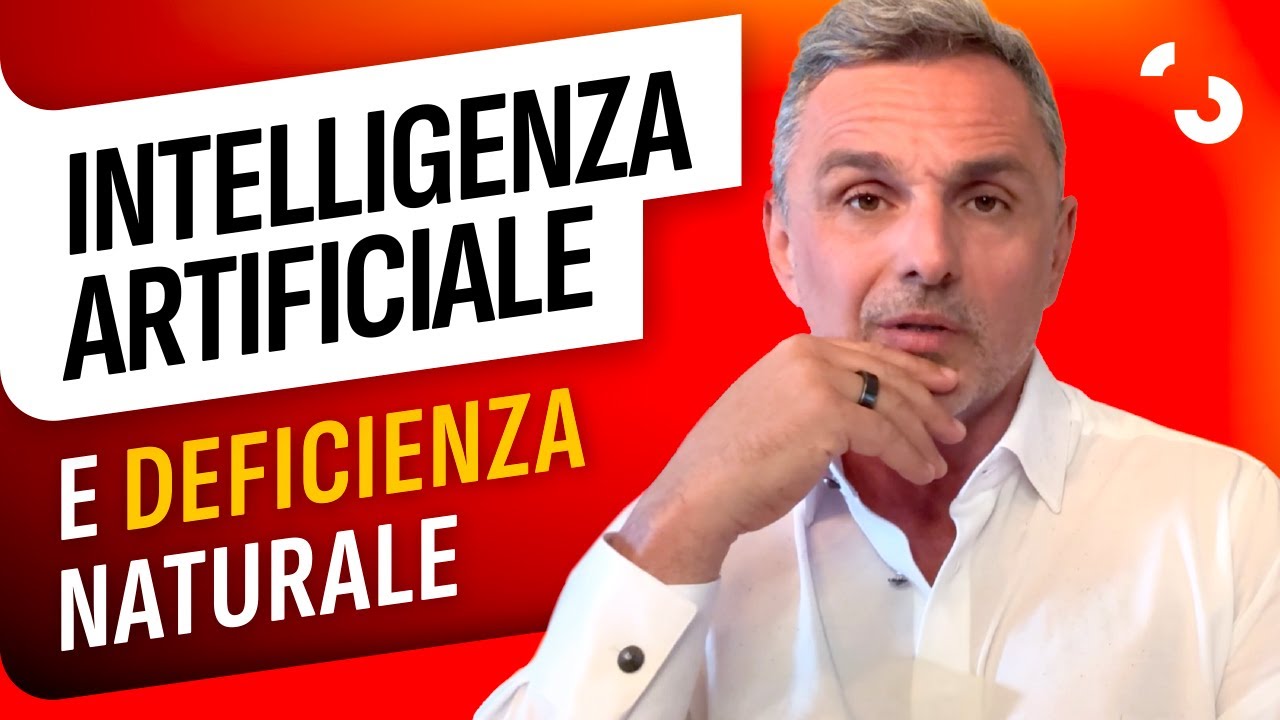
Intelligenza artificiale e deficienza naturale | Filippo Ongaro
5.0 / 5 (0 votes)