This Advanced Kind Of AI Could Be The Secret To AI Assistants
Summary
TLDRこのパネルディスカッションでは、多模態AIの未来について熱く語られました。多模態AIは、画像、ビデオ、テキストを処理できる機械学習モデルであり、人間の生活を高める可能性があるとされています。参加者は、多模様な産業でのAIの活用、ビデオデータの理解強化、そして倫理的・技術的な課題について討議しました。特に、ビデオの意味を理解し、人々の感情を捉える技術の進歩に注目しました。彼らは、AIが信頼を築く上で重要な役割を果たすことを強調し、垂直化とデータの豊富さ、そしてテクノロジーと人間の間の対話の信頼性について語りました。
Takeaways
- 🧠 マルチモーダルAIは、画像、ビデオ、テキストを処理できる機械学習モデルであり、さまざまな情報の形式を理解することが可能です。
- 👥 参加者はそれぞれの分野でマルチモーダルAIを活用する企業を立ち上げ、その技術を応用して革新的なサービスを提供しています。
- 🔍 Avoka AIは、音声AIを利用して最も先進的なレセプションを構築しており、特に家事サービス業に焦点を当てています。
- 🎥 12 Appsはビデオ理解のためのマルチモーダルAIを開発しており、ビデオ内の視覚要素、音声、テキストを解釈し、包括的なビデオ表現を提供しています。
- 📈 Lexi Millsはデジタル倫理とデジタルフォレンジクスに焦点を当てた財団を紹介し、AIツールを使って虐待事件のデータを掘り下げ、検証し、訴訟を進めています。
- 🛠️ マルチモーダルAIの分野では、技術的な課題だけでなく、倫理的な課題も存在しており、それらをどう克服するかが重要な議論となっています。
- 📱 ビデオや音声を活用したマルチモーダルAIは、顧客の感情や非言語的なコミュニケーションを理解し、より効果的なコミュニケーションを可能にします。
- 🌐 今後のマルチモーダルAIの分野で成功する企業は、特定の業界に深く根差ししてデータを収集し、信頼関係を築くことができる企業になるでしょう。
- 🔑 信頼性はAIの成功の鍵であり、企業は技術の信頼性を高めるだけでなく、人間とAIとの間の対話を信頼性のあるものにすることも重要です。
- 🚀 マルチモーダルAIは多くの分野に適用されており、ビデオ編集、スポーツ、医療、学習など、さまざまな垂直市場においてその価値が認められています。
- 🌟 今後のマルチモーダルAIの分野では、より大きなコンテキストモデルが登場し、長い会話の全体を保持し、より自然な対話を実現するでしょう。
Q & A
マルチモーダルAIとはどのような技術ですか?
-マルチモーダルAIは、画像、ビデオ、テキストなど、さまざまな情報の形式を処理できる機械学習モデルです。これにより、より豊かな情報の統合や理解が可能になります。
avoka AIはどのような企業ですか?
-avoka AIは、MITの出身者による共同創業者による企業で、音声AIを利用して最も先進のレセプションを構築しています。特に、電気工、水道工、エアコンなどの古い業界に焦点を当てています。
マルチモーダルAIが持つ最も魅力的な能力は何ですか?
-感情的な側面を組み込む能力が最も魅力的です。販売や説得に必要な非言語的な要素を理解することも重要です。
12 Appsはどのような企業ですか?
-12 Appsは、ビデオを理解するためのマルチモーダルAIを開発している企業です。ビデオ内の視覚要素、音声要素、テキスト要素を解釈し、ビデオの包括的な表現を作成します。
ビデオ理解における大きな課題は何ですか?
-ビデオの時間的次元、物体の動き、視覚と音声、テキストの整合性など、ビデオデータの取り扱いが非常に難しい点です。
デジタル倫理についてLexi Millsさんはどのように考えていますか?
-Lexi Millsさんはデジタル倫理に焦点を当てた財団活動を行い、AIツールを用いて虐待事件のデータを掘り下げ、証拠を集めることにより、以前は曖昧とされていた事件を検証しています。
avoka AIではどのようにして販売を改善していますか?
-avoka AIでは、AIを活用して販売における感情的な側面や人間のnatureを理解し、顧客とのコミュニケーションを改善しています。
12 Labsのビデオ理解技術はどのように使われていますか?
-12 Labsの技術は、例えばYouTubeのビデオから特定の瞬間を見つけるための検索や、ビデオ編集、新しいTV番組や映画のトレーラーの作成、セキュリティー監視など様々な場面で使われています。
感情分析は過去数年でどのように進化しましたか?
-感情分析はテキスト分析の精度が向上した一方で、より重要なのは音声そのものに基づくモデルの開発です。エネルギーの高低などから感情的な状態を推測することができるようになりました。
AIが人間の感情を理解することの重要性とは?
-AIが人間の感情を理解することは、特に販売や顧客サービスにおいて非常に重要です。顧客の関心や要望を読み取り、適切な対話の進行形を決定するのに役立ちます。
マルチモーダルAIが広く採用されるにつれて、企業が成功するためにはどのような要素が必要ですか?
-企業が成功するためには、特定の業界への深い垂直化、高品質のデータの蓄積、信頼性の高め、そしてテクノロジーが人間の能力を補助するというポジショニングが重要です。
Outlines
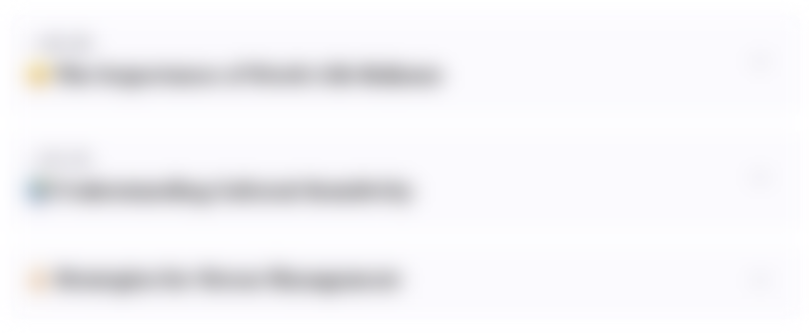
This section is available to paid users only. Please upgrade to access this part.
Upgrade NowMindmap
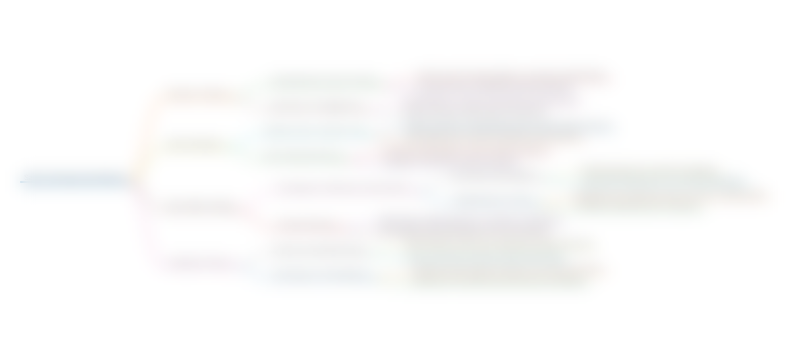
This section is available to paid users only. Please upgrade to access this part.
Upgrade NowKeywords
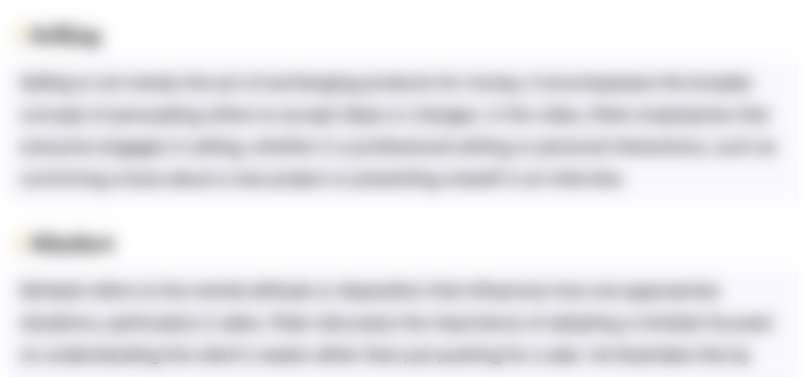
This section is available to paid users only. Please upgrade to access this part.
Upgrade NowHighlights
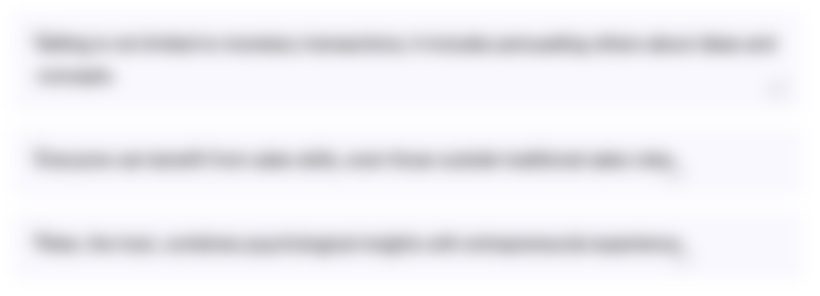
This section is available to paid users only. Please upgrade to access this part.
Upgrade NowTranscripts
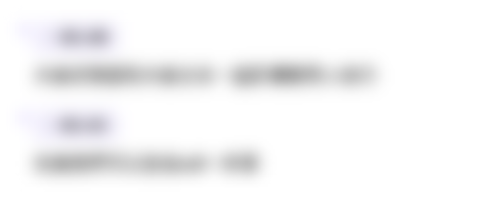
This section is available to paid users only. Please upgrade to access this part.
Upgrade Now5.0 / 5 (0 votes)