Weekly Sync Up - LLM Research for Poultry
Summary
TLDRThe meeting focuses on utilizing a dataset with sales and price data from regions like Jawa Timur and Denpasar. Challenges arise with data aggregation at different levels (unit vs. city) and the need to handle missing data. Interpolation is considered, and there’s an emphasis on enriching the dataset by integrating additional sources, like the Ministry of Agriculture’s data. The team discusses the next steps, including refining data aggregation methods, handling missing values, and combining external data sources to improve model accuracy for predictive analytics.
Takeaways
- 😀 The team will communicate through a shared platform, with information stored on DevOps for easier tracking of features and activities.
- 😀 Important information, including designs and datasets, will be made available for reference by team members.
- 😀 The dataset consists of association prices, recommended prices for farmers, and must be compared with actual sales data from various regions in Indonesia.
- 😀 Sales data includes details like the number of animals sold, weight, and unit of measurement, which are used to determine actual selling prices.
- 😀 Prices and sales data must be updated regularly and assigned to appropriate dates for effective analysis and forecasting.
- 😀 The suggested price data can be distributed via WhatsApp to farmers or sellers for easier implementation and tracking.
- 😀 The dataset includes reasons for price variation, such as empty cages or sick animals, which can influence pricing decisions.
- 😀 The real selling price is based on the recommended price, weight of the animals, and factors like local market conditions or special events.
- 😀 The team needs to decide whether to aggregate prices by city or by individual farms, depending on data availability and the level of detail required.
- 😀 There are gaps in the dataset that need to be addressed, such as missing data for certain locations. Interpolation is used to estimate missing values for analysis.
- 😀 The model development might require combining multiple data sources to overcome the issue of insufficient data for effective training, with potential use of data from the Ministry of Agriculture.
Q & A
What is the main focus of the communication discussed in the transcript?
-The main focus is on managing and updating a dataset related to livestock sales across various regions like Jawa Timur and Denpasar, and the coordination between team members to ensure proper data entry and aggregation for modeling.
How is the dataset organized, and what information does it contain?
-The dataset includes sales data for livestock such as the number of animals sold, their weight (tonnage), the price at which they were sold, and the reasons for variations in price, including factors like animal health and market conditions.
What role does data aggregation play in this process, and what issues have arisen?
-Data aggregation is critical to ensure accurate analysis. Issues have arisen in aggregating data from various cities or units, particularly when prices differ across locations, leading to challenges in creating a cohesive time series dataset.
What is the suggested approach to handling missing or incomplete data in the dataset?
-The suggested approach is to use interpolation to fill in missing values, although there are concerns about its effectiveness, especially when data gaps are significant.
What kind of data does the team need to focus on for the predictive modeling?
-The team needs to focus on aggregating data either by specific units or cities, ensuring that the data is comprehensive enough for accurate predictive modeling, especially considering the price variations and missing values.
Why is the price association data important in this context?
-The price association data is important because it suggests recommended prices for livestock, which can help standardize pricing and guide the sales process, considering regional variations and market conditions.
What concerns were raised about the small size of some datasets?
-The small size of certain datasets, especially when aggregating by unit or city, limits the ability to generate a robust time series model. The team expressed concerns that the model might not perform well due to the limited amount of data.
How does the team plan to deal with different price ranges across various regions?
-The team is considering whether to focus on price ranges within specific cities or units, using methods like calculating the average price or defining a price range to deal with varying prices across different locations.
What external data sources are being considered to augment the internal dataset?
-The team is considering integrating external data from the Ministry of Agriculture, which could provide more comprehensive coverage of price data across various regions, especially in areas where internal data is sparse.
What is the next step for the team regarding the dataset and modeling process?
-The next step involves continuing to explore additional data sources, refining the data aggregation approach, and beginning model development. The team will also consider using smaller datasets for testing and validation.
Outlines
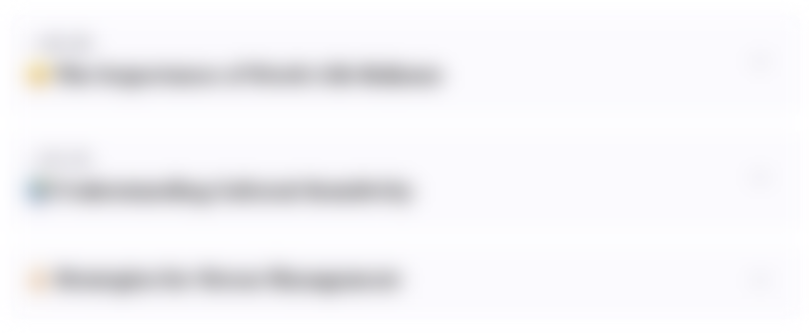
This section is available to paid users only. Please upgrade to access this part.
Upgrade NowMindmap
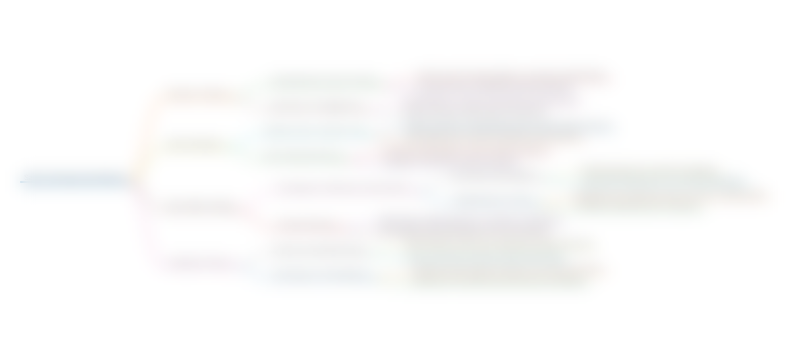
This section is available to paid users only. Please upgrade to access this part.
Upgrade NowKeywords
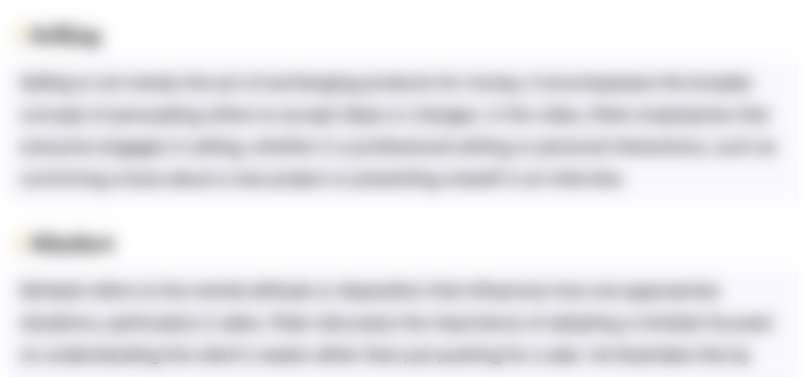
This section is available to paid users only. Please upgrade to access this part.
Upgrade NowHighlights
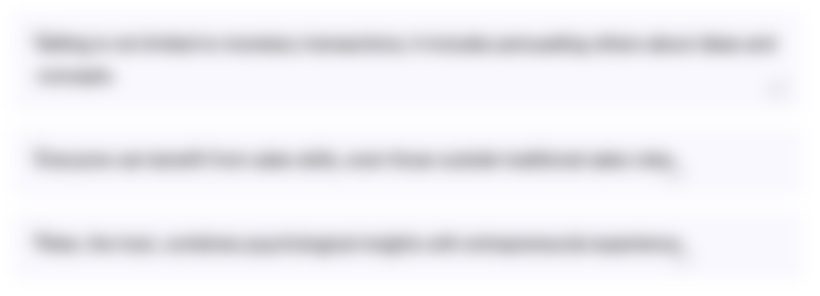
This section is available to paid users only. Please upgrade to access this part.
Upgrade NowTranscripts
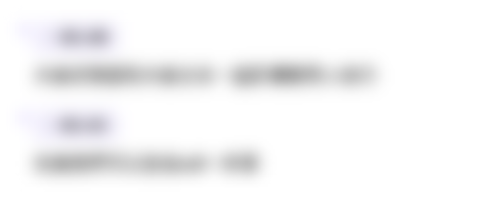
This section is available to paid users only. Please upgrade to access this part.
Upgrade NowBrowse More Related Video
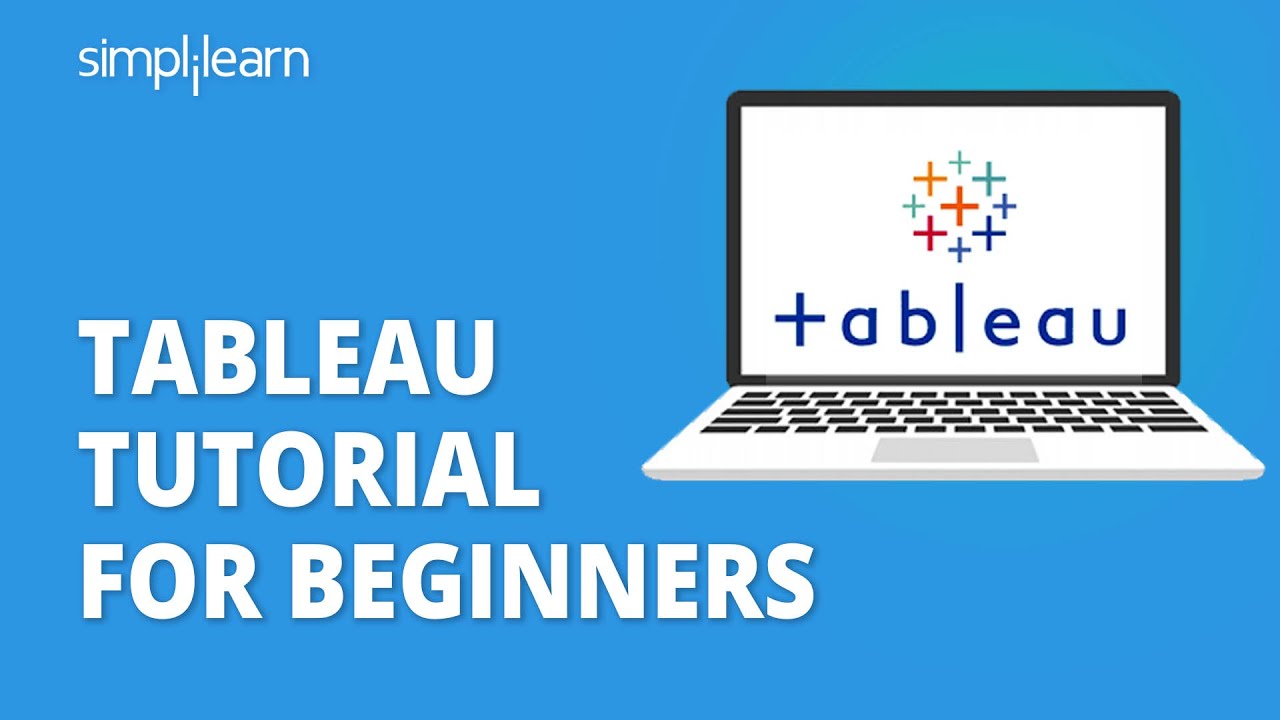
Tableau Tutorial for Beginners in 20 Minutes | Complete Tableau Training for Beginners | Simplilearn
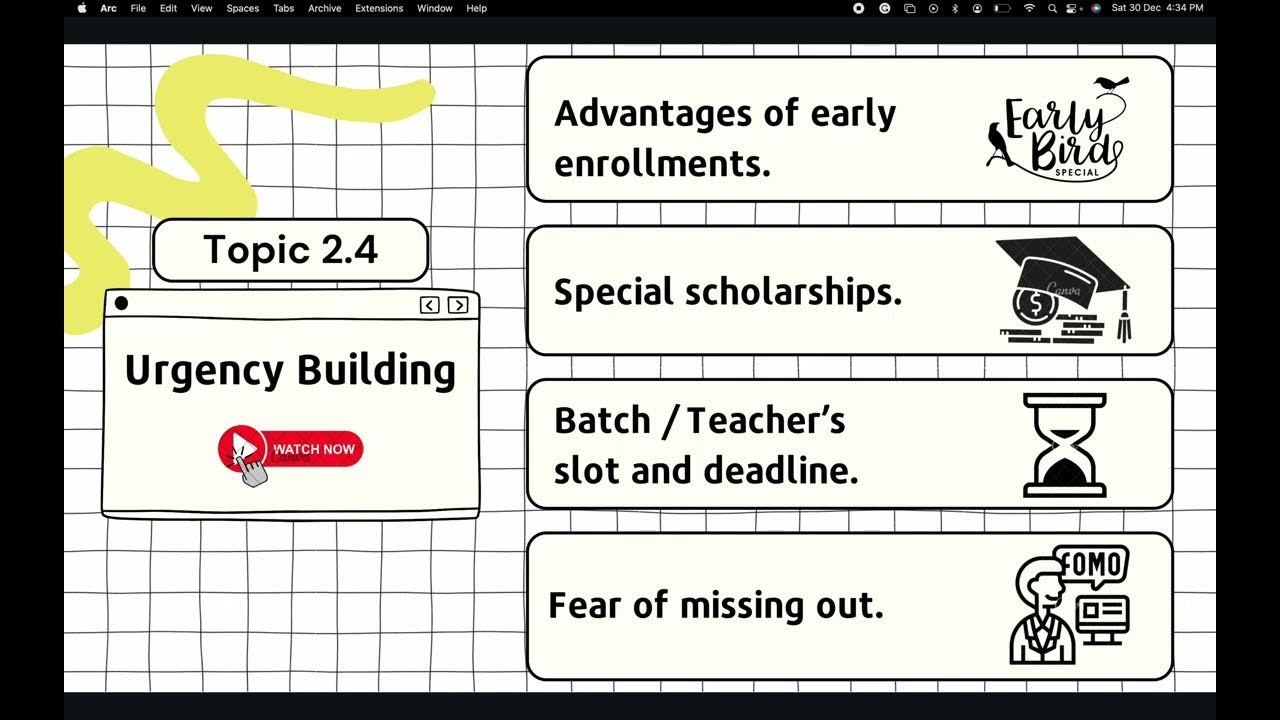
Urgency Building- Call

Case Study on Regression Part I

Python Module 6 (Part 1) Using Python Libraries Like Pandas for Displaying Data
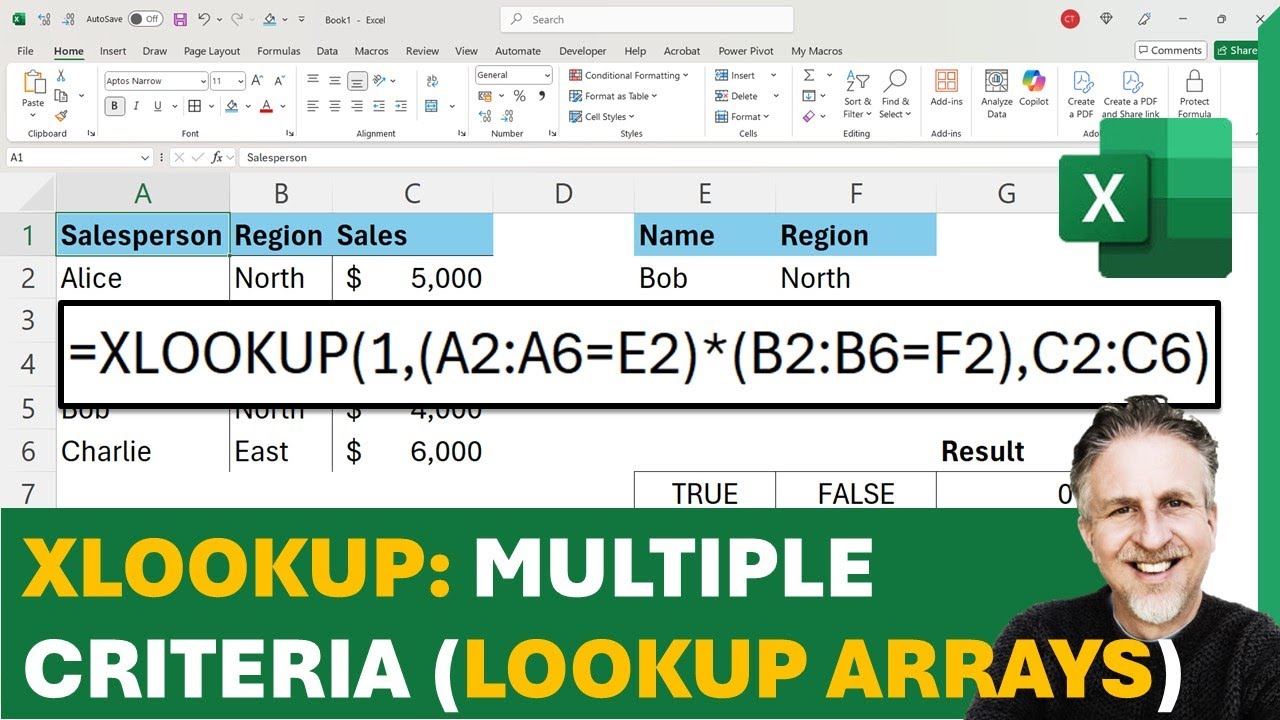
Excel XLOOKUP with Multiple Criteria - Multiple Lookup Arrays (Multiple Columns)
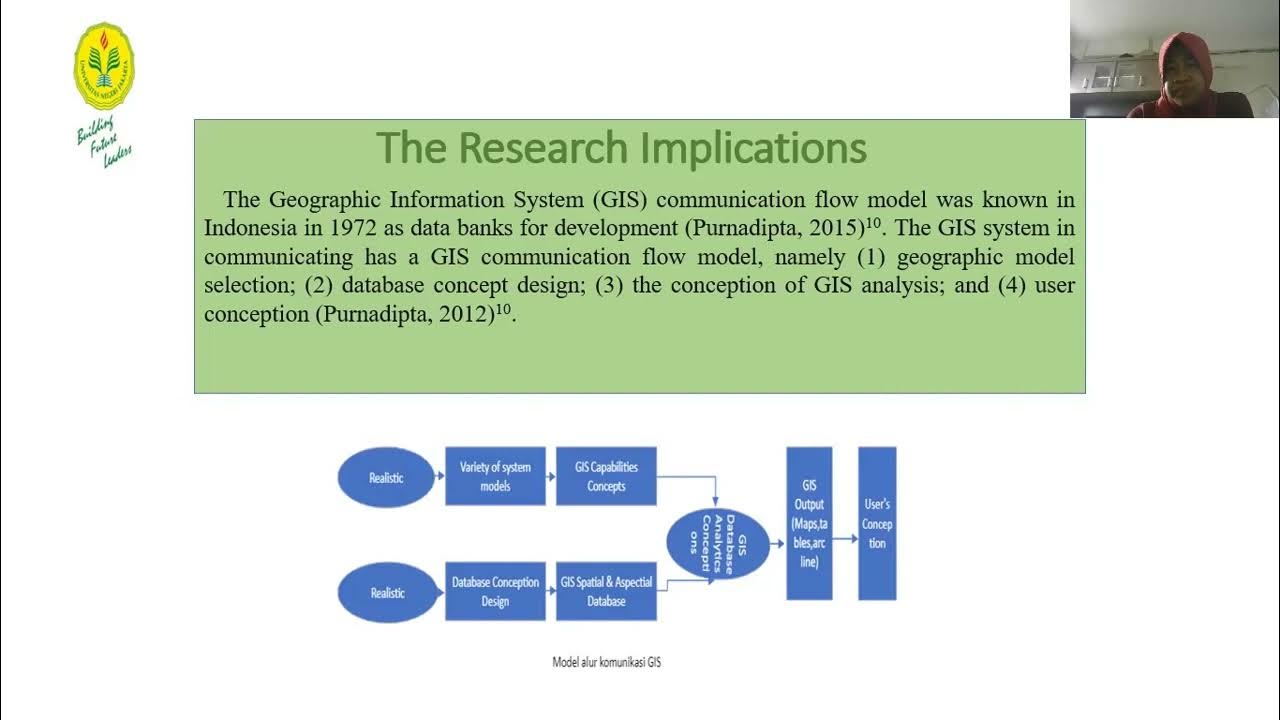
ABS 29 Science Diena
5.0 / 5 (0 votes)