DeepSeek R1 vs OpenAI o3-mini vs o1 pro vs Gemini Flash 2.0 Thinking | Lex Fridman Podcast
Summary
TLDRThis conversation dives deep into the evolution of AI reasoning models, particularly focusing on OpenAI's GPT-3, 01, Gemini, and other advanced models. It highlights the differences in model training, such as reinforcement learning, instruction tuning, and reasoning-heavy techniques. The discussion also examines how these models handle open-ended philosophical queries, offering a comparison of their reasoning capabilities, response quality, and performance. From philosophical insights to technical advancements in training, inference, and cost reduction, the conversation explores the future of AI reasoning and its potential to achieve more sophisticated intelligence.
Takeaways
- 😀 OpenAI's 01 Pro provides rich, philosophical insights but can be inconsistent across tasks compared to other models like 03 Mini.
- 😀 03 Mini is fast and efficient, but it often delivers more generic answers, especially for complex philosophical questions.
- 😀 Gemini Flash 2.0 from Google focuses on integrating reasoning with traditional models but lacks the depth of reinforcement learning used by other models like R1.
- 😀 DeepSE's R1 model uses explicit reasoning chains and reinforcement learning to produce more detailed and structured answers compared to other models.
- 😀 Chain of Thought (CoT) reasoning is now a default method in language models, allowing for step-by-step verification of answers, particularly in math and logic tasks.
- 😀 The **Monte Carlo Search** method in some models uses parallel samples to improve the accuracy of the response by selecting the best one from multiple outputs.
- 😀 Cost efficiency is improving rapidly in AI models; training and inference costs have decreased by up to 1200X, making advanced models more accessible.
- 😀 The training techniques used in models like 01 and Gemini Flash include reinforcement learning (RL), instruction tuning, and large-scale reasoning, which enable them to tackle open-ended questions.
- 😀 There is a tradeoff between model expressiveness and specialization, with models like 01 being more flexible in handling diverse tasks compared to specialized models like Gemini Flash.
- 😀 The future of AI reasoning models lies in leveraging parallel processing and advanced search techniques to enhance their ability to solve complex problems efficiently.
- 😀 OpenAI's models (01 Pro and 03 Mini) are part of a broader trend toward more efficient and powerful AI systems that will transform industries by combining more complex reasoning and improved computational methods.
Q & A
What are the different flavors of reasoning models discussed in the transcript?
-The transcript discusses various flavors of reasoning models, including OpenAI's 01, 03 mini, Gemini, and the Deep Seek models. These models differ in their training processes, with some focusing more on reinforcement learning (RL), while others like Gemini Flash are based on a more traditional training stack augmented with reasoning.
How does the reasoning training process work for these models?
-The reasoning models go through a large-scale reasoning training with reinforcement learning (RL) followed by post-training techniques such as heavily filtered instruction tuning and reward models. These models are then further refined with additional training to make them more human-preference aligned and capable of handling diverse tasks.
What is the primary difference between Gemini Flash and OpenAI's models like 01?
-Gemini Flash, particularly its 2.0 version, focuses on more traditional training techniques with reasoning added, whereas OpenAI’s 01 model undergoes intensive RL training to elicit reasoning capabilities. Gemini Flash is considered less expressive and more math-heavy, while OpenAI's models like 01 are designed for flexibility and can handle a broader range of tasks.
What does the term 'self-domesticated apes' refer to in the context of human nature?
-The term 'self-domesticated apes' refers to the idea that humans, through self-domestication, have developed unique cognitive and social abilities that set them apart from other species. This concept is explored in the context of understanding human nature and our capacity for advanced reasoning and communication.
What was the insight provided by Deep Seek's Gemini Flash 2.0 thinking model about humans?
-Gemini Flash 2.0 suggested that humans are not just social animals but 'self-domesticated apes.' It proposed that this self-domestication is key to understanding human cognitive and social abilities, emphasizing traits like adaptability, social dependence, and plasticity.
How does OpenAI's 01 Pro model approach philosophical questions like 'Give one truly novel insight about humans'?
-OpenAI’s 01 Pro consistently provided brilliant, thought-provoking responses to philosophical questions. It excelled at delivering insights that made the user pause and reflect, combining wit, clarity, and depth in its answers. 01 Pro stands out for generating responses that are both intellectually sharp and creatively phrased.
Why was 03 Mini considered less effective for answering open philosophical questions?
-03 Mini, while performing well in other tasks, was considered less effective for philosophical questions because it often provided generic responses. Despite its speed and efficiency, it lacked the depth and novelty that models like 01 Pro were able to generate in such contexts.
What role does Chain of Thought play in reasoning models, and how is it different in models like 01 Pro and Deep Seek?
-Chain of Thought refers to the process where a reasoning model generates a series of logical steps to solve a problem. Models like Deep Seek R1 and OpenAI’s 01 Pro demonstrate the power of Chain of Thought by showing the full deliberative process, which allows users to observe how the model arrives at its conclusions. This transparency in reasoning is valuable for understanding and trusting the model's outputs.
How do search techniques and parallel sampling contribute to the performance of reasoning models like OpenAI’s 01 Pro?
-Search techniques and parallel sampling involve running multiple chains of thought simultaneously, which enhances the model's ability to generate accurate responses. By sampling many possible solutions and selecting the best one, models like 01 Pro can improve their reliability and produce more accurate results, especially in complex tasks.
What is the significance of the cost reduction in inference for models like GPT-3 and the implications for the future of AI?
-The significant reduction in inference costs, from $60-$70 per million tokens for GPT-3 to mere cents for newer models, is a crucial factor in the scalability of AI technologies. This cost reduction will make advanced reasoning models more accessible and enable the development of more sophisticated systems, driving further innovation in artificial intelligence.
Outlines
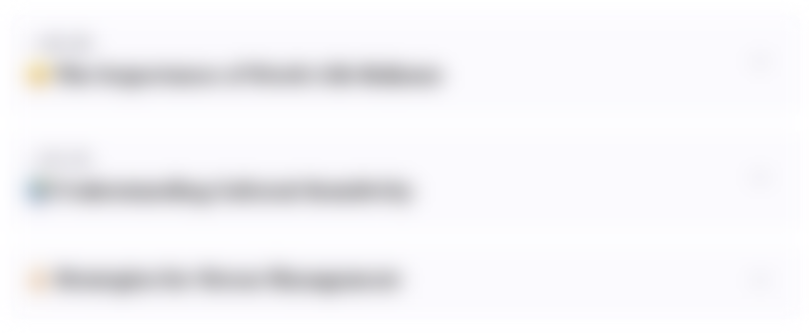
This section is available to paid users only. Please upgrade to access this part.
Upgrade NowMindmap
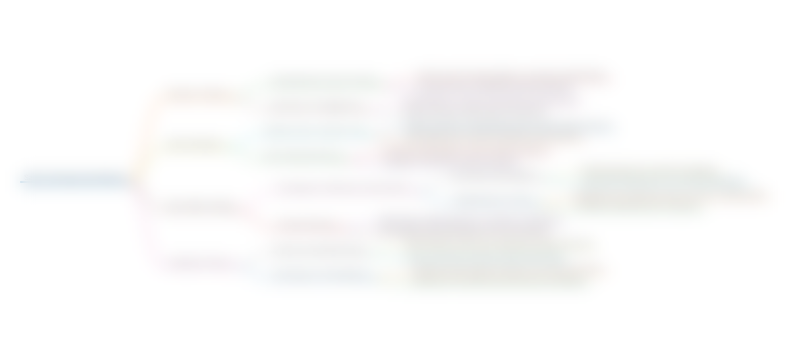
This section is available to paid users only. Please upgrade to access this part.
Upgrade NowKeywords
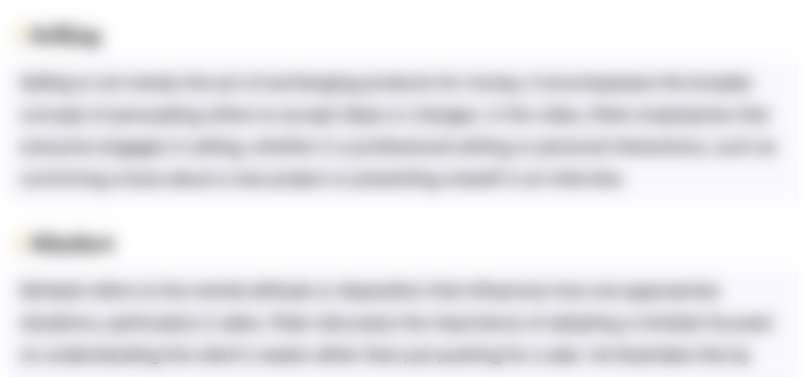
This section is available to paid users only. Please upgrade to access this part.
Upgrade NowHighlights
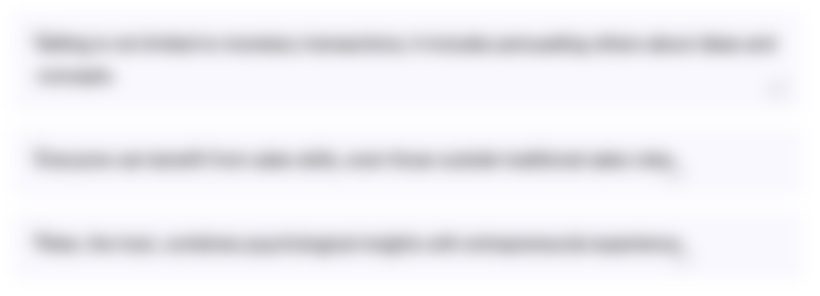
This section is available to paid users only. Please upgrade to access this part.
Upgrade NowTranscripts
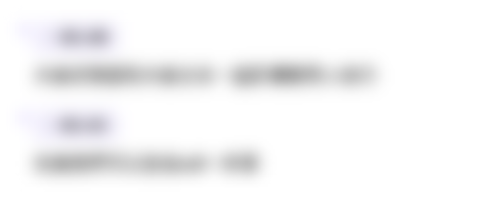
This section is available to paid users only. Please upgrade to access this part.
Upgrade NowBrowse More Related Video
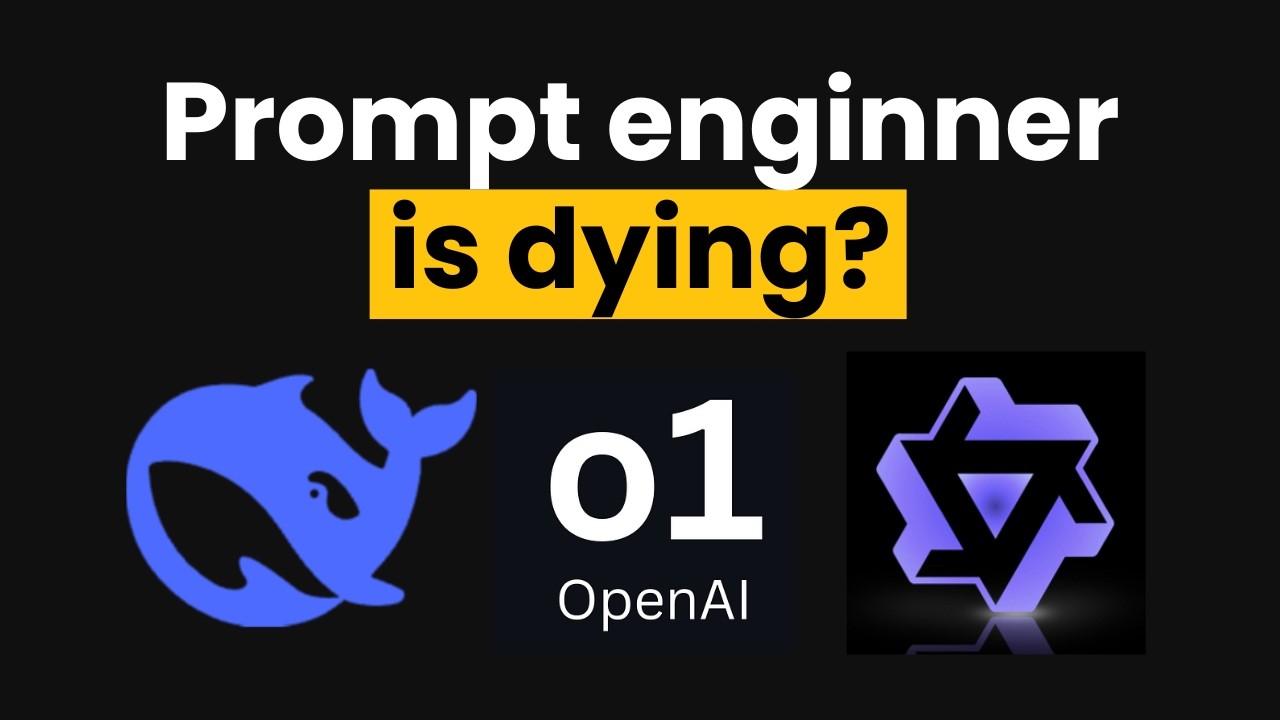
Deepseek R1 - The Era of Reasoning models
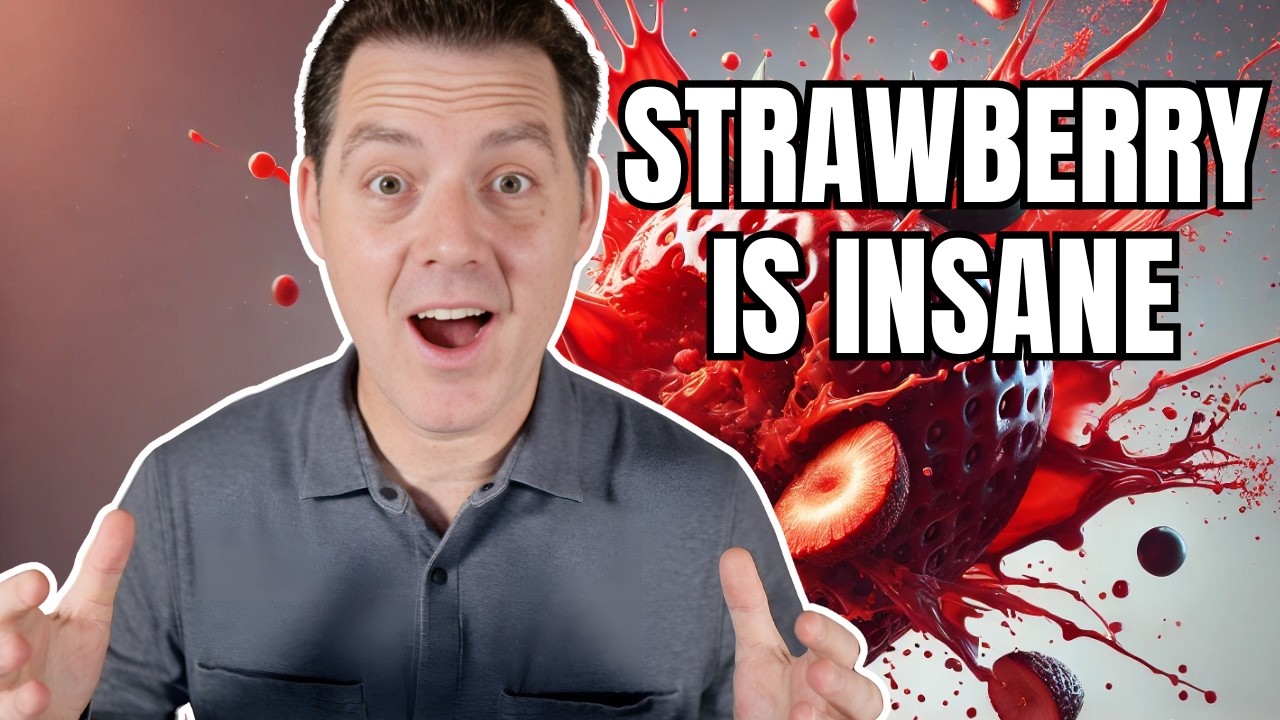
OpenAI Releases GPT Strawberry 🍓 Intelligence Explosion!
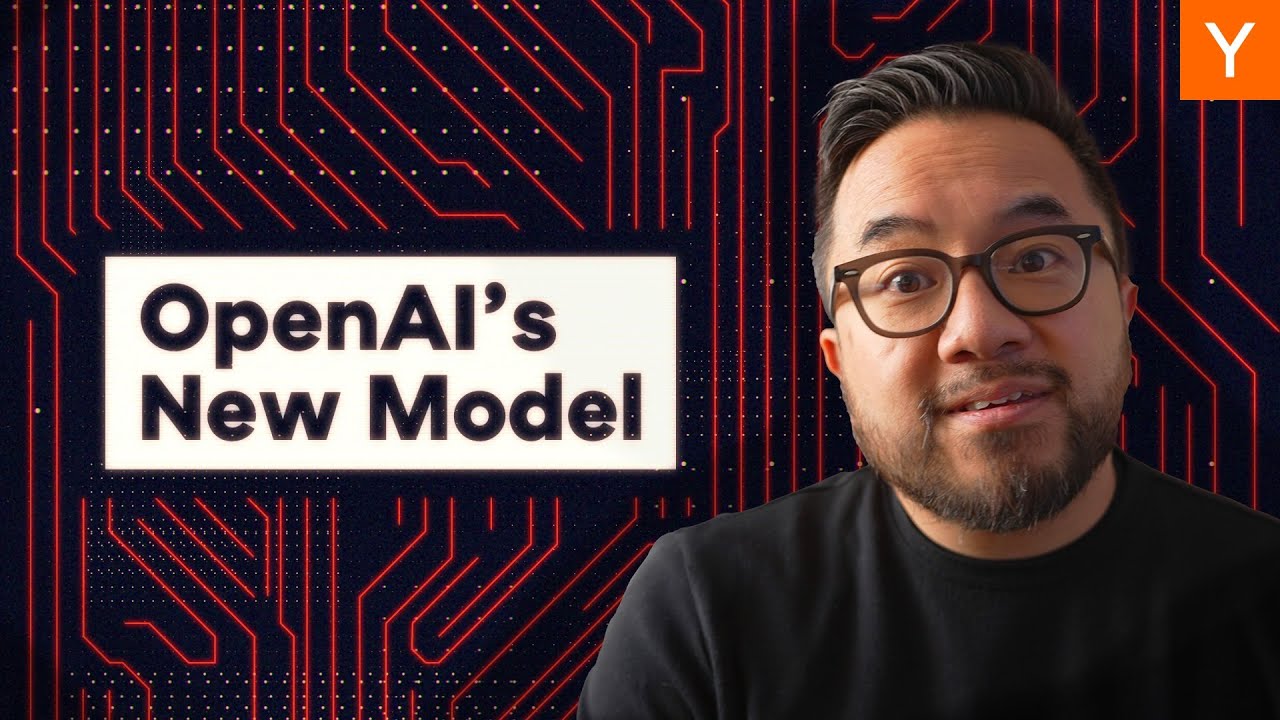
Why OpenAI's o1 Is A Huge Deal | YC Decoded
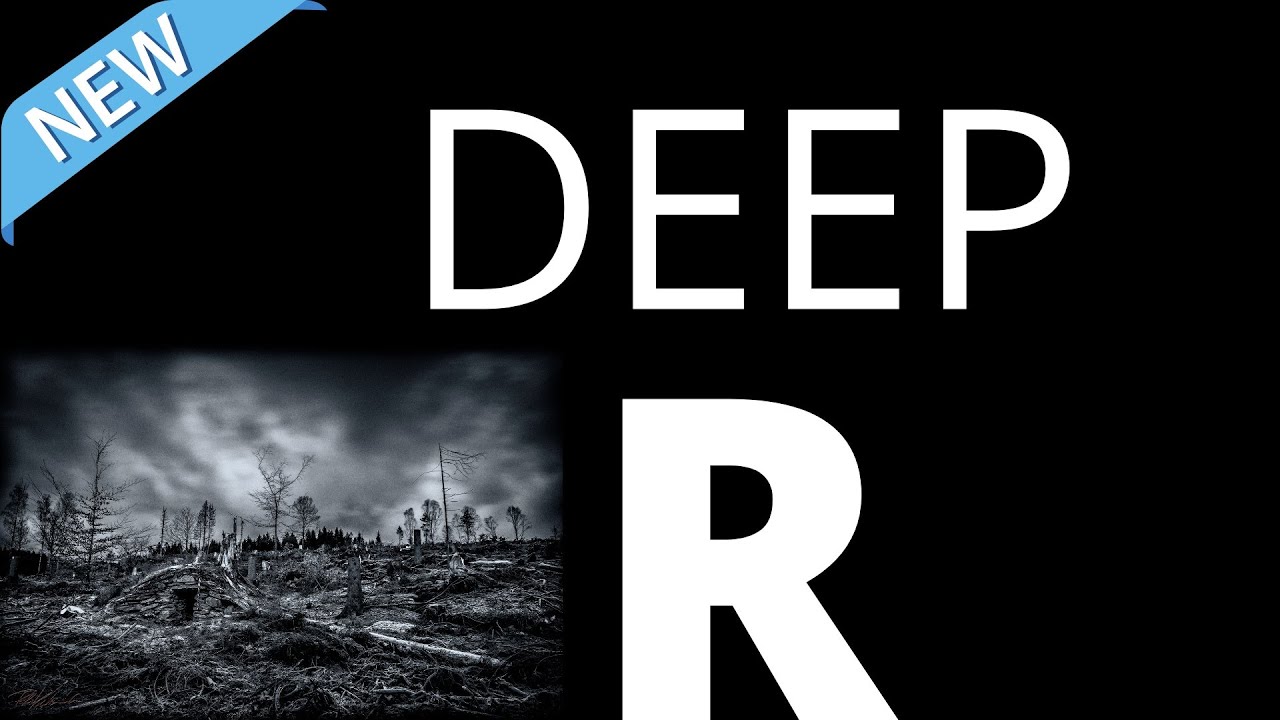
AI DISASTER: Product DEMO by OpenAI - Deep Research
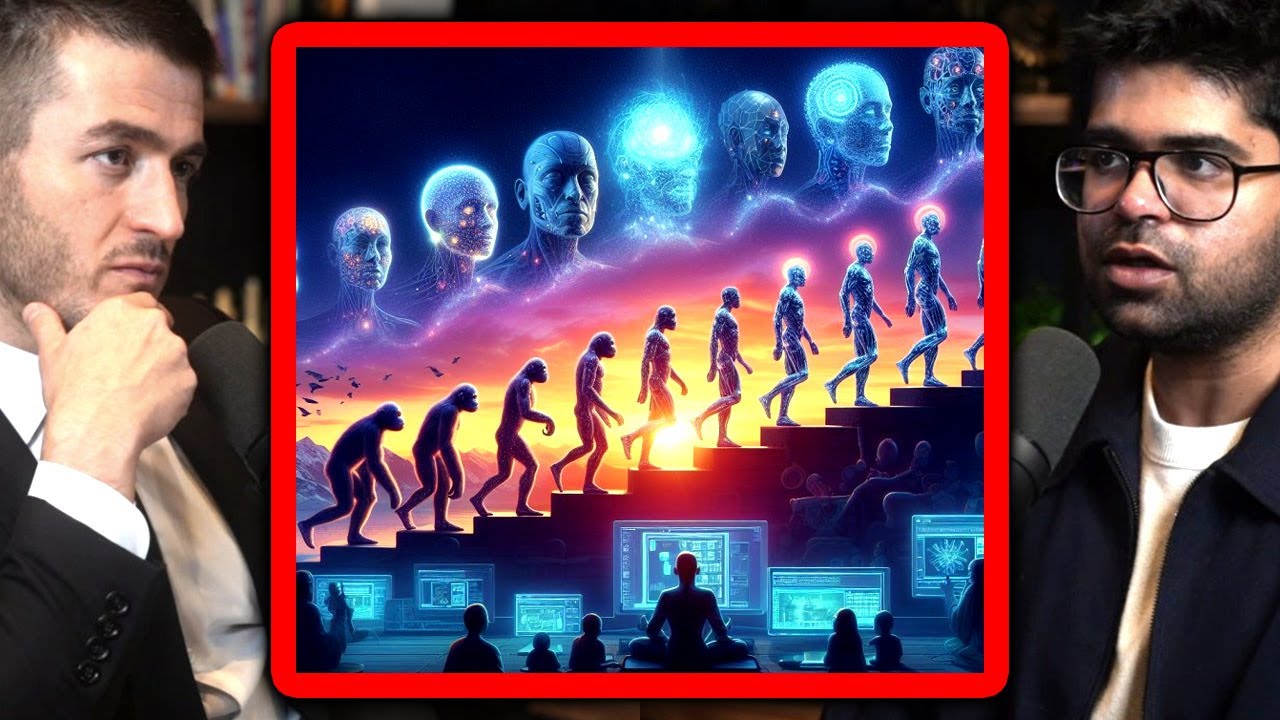
Recent breakthroughs in AI: A brief overview | Aravind Srinivas and Lex Fridman
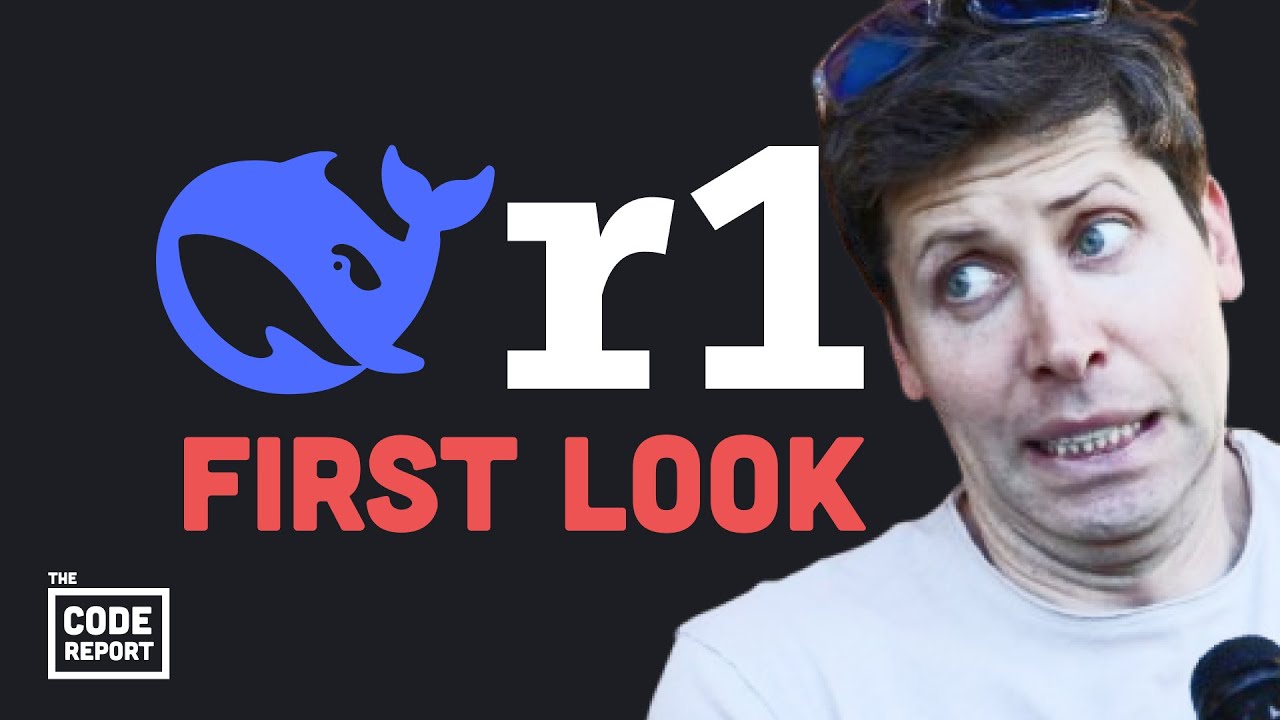
This free Chinese AI just crushed OpenAI's $200 o1 model...
5.0 / 5 (0 votes)