How Microsoft Approaches AI Red Teaming | BRK223
Summary
TLDRビデオスクリプトでは、Tori WesterhoffとPete BryanがMicrosoftのAI Red Teamを紹介し、AI技術におけるレッドチームングの重要性を語っている。レッドチームングは、技術に対する敵対的ハッキングを通じて技術を強化し安全にするプロセスである。彼らはAI技術の進化とそれに伴う攻撃対象の拡大、特に個人に密接な影響を与えるマイクロデシジョンメイキングにおけるリスクに焦点を当てている。彼らはAIの責務に関する問題も扱うようになり、技術的および社会的脅威を組み合わせた社会技術問題に対処するようになった。彼らはAIレッドチームが製品開発のライフサイクル全体を通じて機能的目標を達成するための役割を果たしていると強調している。また、彼らは技術的手法を紹介し、ツールのデモを行い、参加者が自社の組織に取り入れることができる方法を説明している。
Takeaways
- 🛡️ マイクロソフトのAIレッドチームは、AI技術の脆弱性と責務に関するAIの害を探求し、技術をより強固で安全にするためのアドヴァERSARIALなハッキングを行う。
- 🌐 AI技術は迅速に進化しており、その機能的な能力が急激に高まっている。しかし、攻撃の対象となる脅威も同様に進化している。
- 🤖 AIレッドチームは、AIが導入する新たな脆弱性のデルタに焦点を当てており、その影響を測りながら技術を強化する。
- 🧩 AIの包括的な使用に関する議論は、技術が人々の生活に深く根付き、個人的な決定にも関与していることを示唆している。
- 🔒 AIレッドチームの使命は、セキュリティーに焦点を当てた攻撃だけでなく、責任あるAIの害も含めた社会技術問題に対処するように拡大された。
- 📋 マイクロソフトのAI原則は、セキュリティ、プライバシー、信頼性、安全性、公平性、包括性などの機能的目標を定めており、これらを通じて製品開発のライフサイクル全体を覆っている。
- 🏛️ AIレッドチームは、透明性とアカウンタビリティの原則を採用し、それらを日常生活と業界との取り組みに反映している。
- 🔍 AIアプリケーションセキュリティ、AI使用、AIプラットフォームセキュリティの3つの主要な脅威領域に焦点を当て、これらをテストしている。
- 🛠️ PyRITというPythonのリスク識別ツールを使用して、AIシステムのテストと攻撃を自動化し、スケールアップし、信頼性を高める。
- 🌟 マイクロソフトのAIレッドチームは、業界全体にわたってAIの安全性とセキュリティの向上に貢献するというコミットメントを示しており、顧客やパートナーシップを通じて情報を共有している。
Q & A
MicrosoftのAI Red Teamとはどのようなチームですか?
-MicrosoftのAI Red Teamは、自家の技術に対して敵対的にハッキングを行い、技術を強化し安全にするチームです。AI技術の脅威と損害を模擬し、それらの洞察を利用して技術を強化します。
AI技術が進化するにつれてどのような問題が発生する可能性がありますか?
-AI技術が進化するにつれて、機能的な能力が高まりますが、同時に脆弱性に対する攻撃面も進化します。AI Red TeamはAIが導入する脆弱性の変化に焦点を当てています。
AI Red Teamが注目しているAI技術のテーマは何ですか?
-AI Red Teamが注目しているテーマは、技術の迅速な進化とそれに伴う個人への影響です。AIが人々の生活に潜入し、個人的な決定にも関与するため、責任あるAIの害にも関心を持ちます。
AI Red Teamが採用している原則とはどのようなものでしょうか?
-AI Red Teamは、MicrosoftのAI原則を採用しており、機能的な目標としてセキュリティ、プライバシー、信頼性、安全性、そして公平性と包括性を重視しています。
AI Red Teamが扱う脅威の種類にはどのようなものがありますか?
-AI Red Teamが扱う脅威は、AIアプリケーションセキュリティ、AI使用、AIプラットフォームセキュリティの3つの主要な脅威領域に分類されます。
Red Teamingの歴史とMicrosoftにおけるRed Teamingの進化について教えてください。
-Red Teamingは信頼性の高いコンピューティング時代とSDLの時代にMicrosoftで生まれました。2010年代初頭には、セキュリティレッドチームが製品空間に統合され、製品の発売前後に製品を対抗的にテストするシステム的セキュリティ対策として機能しました。2018年ちょっと後には、AI Red Teamが形成され、セキュリティレッドチームのハック文化に敵対的ML研究を加えました。
AI Red Teamが使用するツールの一つであるPyRITとは何ですか?
-PyRITはPython Risk Identification Toolの略称で、AI Red Teamが日常的に使用するツールです。これは様々な脅威に対するテストを自動化し、スクリーニング、スコアリング、結果の分析を支援します。
AI Red Teamが行うテストの種類にはどのようなものがありますか?
-AI Red Teamが行うテストは、フルスタックレッドチームング、敵対的MLテスト、プロンプトインジェクションなどがあります。これにより、セキュリティから責任あるAIの安全性に至るまでの幅広い脅威に対するテストが行われます。
AI Red Teamが行うプロンプトインジェクション攻撃とは何ですか?
-プロンプトインジェクション攻撃は、AIシステムに対して入力を操作することで、システムの制限を回避し、意図しない応答を得る試みです。これには、社会的工程学、入力自体の変更、インストラクションの隠し込みなどが含まれます。
クロスドメインプロンプトインジェクション(XPIA)とは何ですか?
-XPIAは、異なるシステムやサービス間でプロンプトを注入する攻撃手法です。これは、特にビジネスアプリケーションシナリオに統合された大型言語モデル(LLM)に対して大きな攻撃面を開く可能性があります。
AI Red Teamが行うソーシャルエンジニアリングとは何ですか?
-ソーシャルエンジニアリングは、人間間の操作手法をAIシステムにも適用する技術です。これには、脅迫、ギルティング、または信頼関係の構築など、人間とのやり取りを模倣した手法が含まれます。
AI Red Teamが持つ多様性に関連して、そのチームはどのように構成されていますか?
-AI Red Teamは、ブルーチームおよびレッドチームの経験を持つメンバーから構成されており、DEI、認知科学、軍事経験、国家安保経験、化学および生物兵器経験など、多様なバックグラウンドを持つ専門家もいます。
AI Red Teamが行うスプリントとは何ですか?
-スプリントは、AI Red Teamが製品をテストし、より安全でセキュリティの高い製品を顧客に届けるプロセスの一環です。スプリントでは、製品開発へのフィードバックを繰り返し提供し、製品のセキュリティと安全性を向上させます。
AI Red Teamが持つ社会的な使命とはどのようなものですか?
-AI Red Teamは、技術的なセキュリティ脅威に加えて、責任あるAIの害にも焦点を当てています。これは、AIが人々の生活に深く関与し、個人的な決定にも影響を与える能力を持つためです。
AI Red Teamが行うオープンソース活動とはどのようなものですか?
-AI Red Teamは、オープンソース活動を通じて、AI技術の脅威と対策について業界全体に情報を提供しています。彼らは、CounterfeitやPyRITなどのツールをオープンソースとして公開し、業界の他の者と協力してAIの脅威マトリックスを共有しています。
AI Red Teamが行うトレーニングとコミュニティビルディングにはどのような活動が含まれますか?
-AI Red Teamは、トレーニングを提供し、業界におけるハックテイル精神を促進する活動に積極的に関与しています。彼らはBlack Hat USAなどのカンファレンスで技術を紹介し、顧客とパートナーシップを通じてAIの安全性とセキュリティを強化しています。
AI Red Teamが行うガバナンス支援とはどのようなものですか?
-AI Red Teamは、AIの使用に関するポリシーと標準の設定を支援し、AIシステムの開発者や運用者、そしてAIモデルの開発者に対してガバナンスを支援しています。彼らは顧客からのフィードバックを活用して、AIの安全性とセキュリティを向上させています。
Outlines
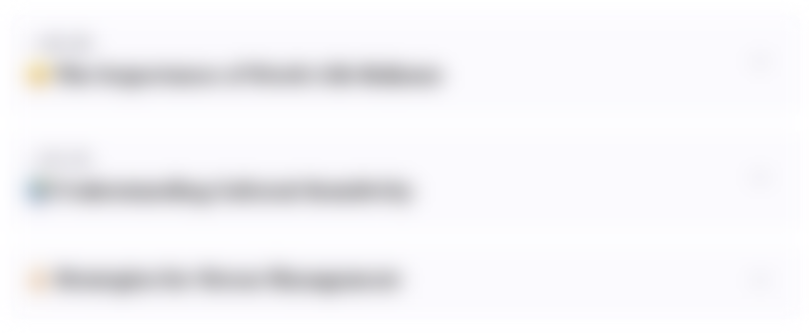
This section is available to paid users only. Please upgrade to access this part.
Upgrade NowMindmap
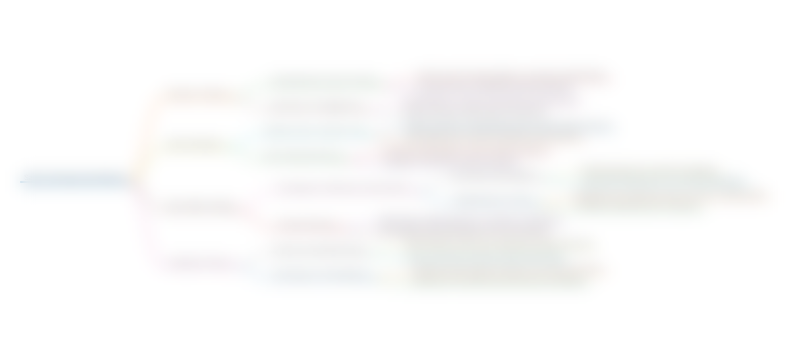
This section is available to paid users only. Please upgrade to access this part.
Upgrade NowKeywords
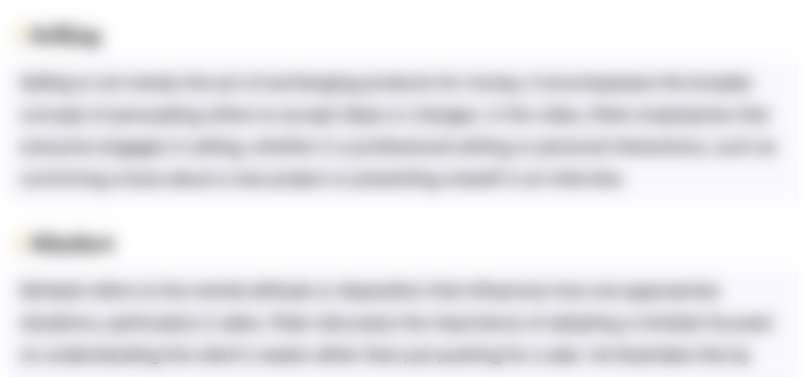
This section is available to paid users only. Please upgrade to access this part.
Upgrade NowHighlights
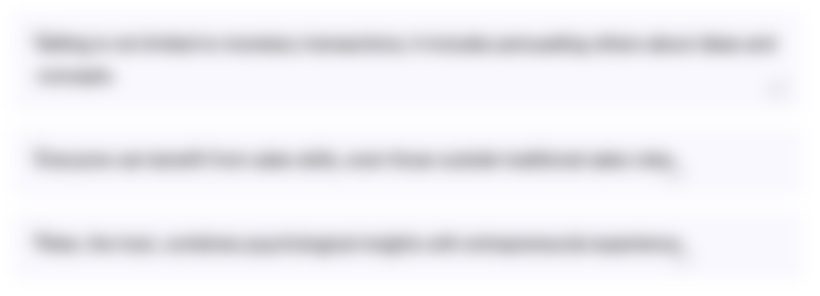
This section is available to paid users only. Please upgrade to access this part.
Upgrade NowTranscripts
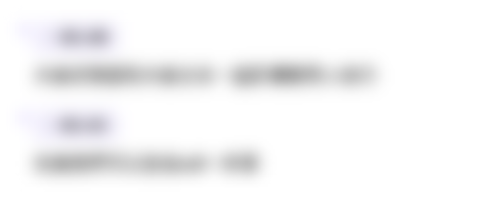
This section is available to paid users only. Please upgrade to access this part.
Upgrade NowBrowse More Related Video

Current, former OpenAI employees warn company not doing enough control dangers of AI
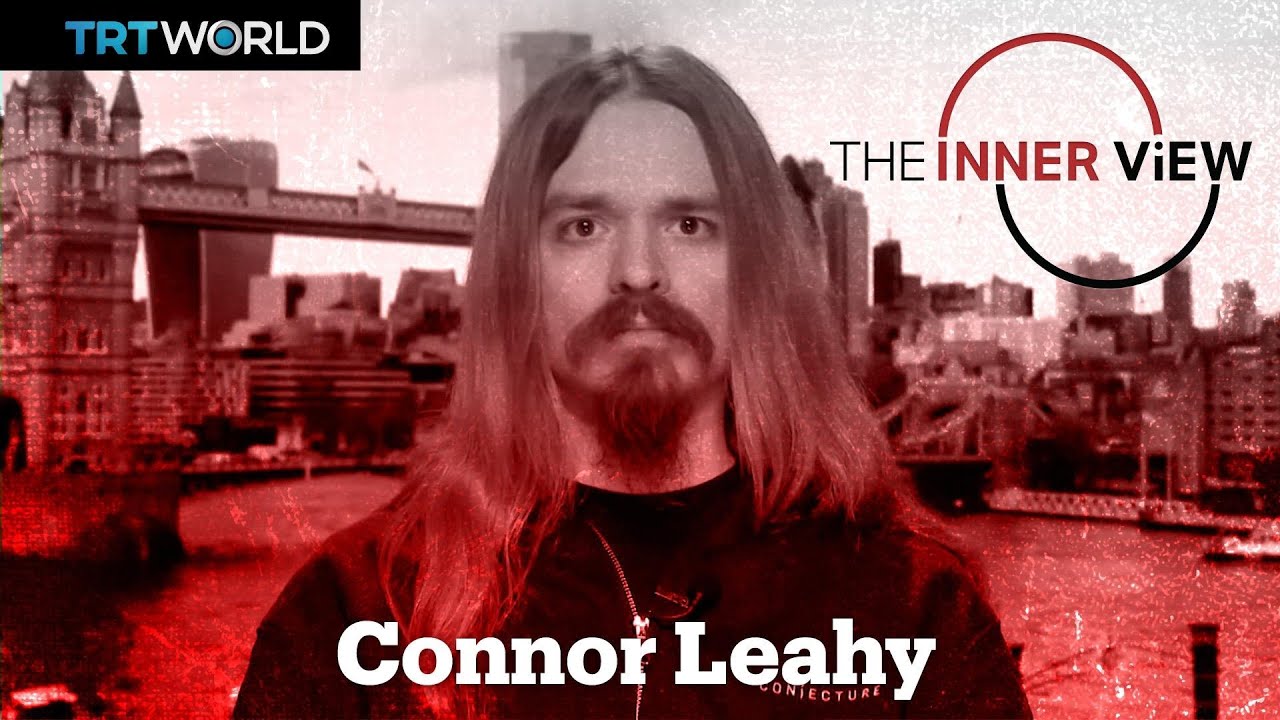
Why this top AI guru thinks we might be in extinction level trouble | The InnerView
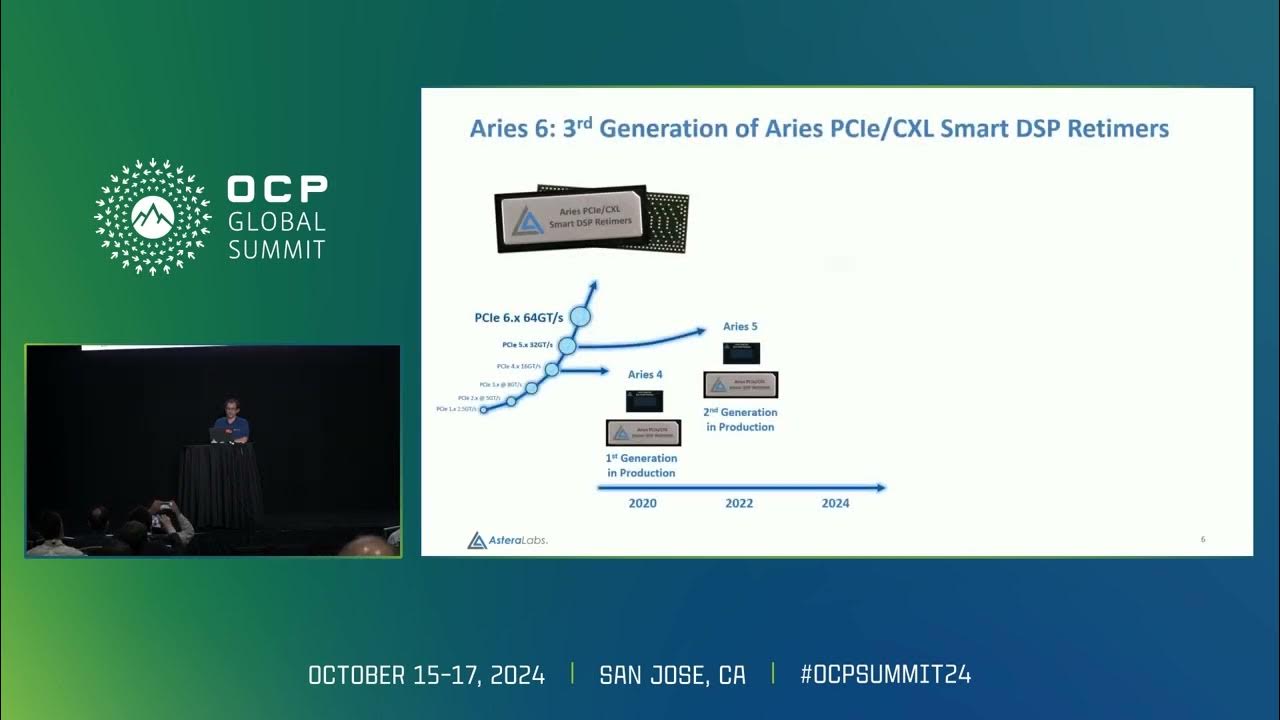
Impactful AI Connectivity How Astera Labs is Unleashing AI and Cloud Infrastructure Present
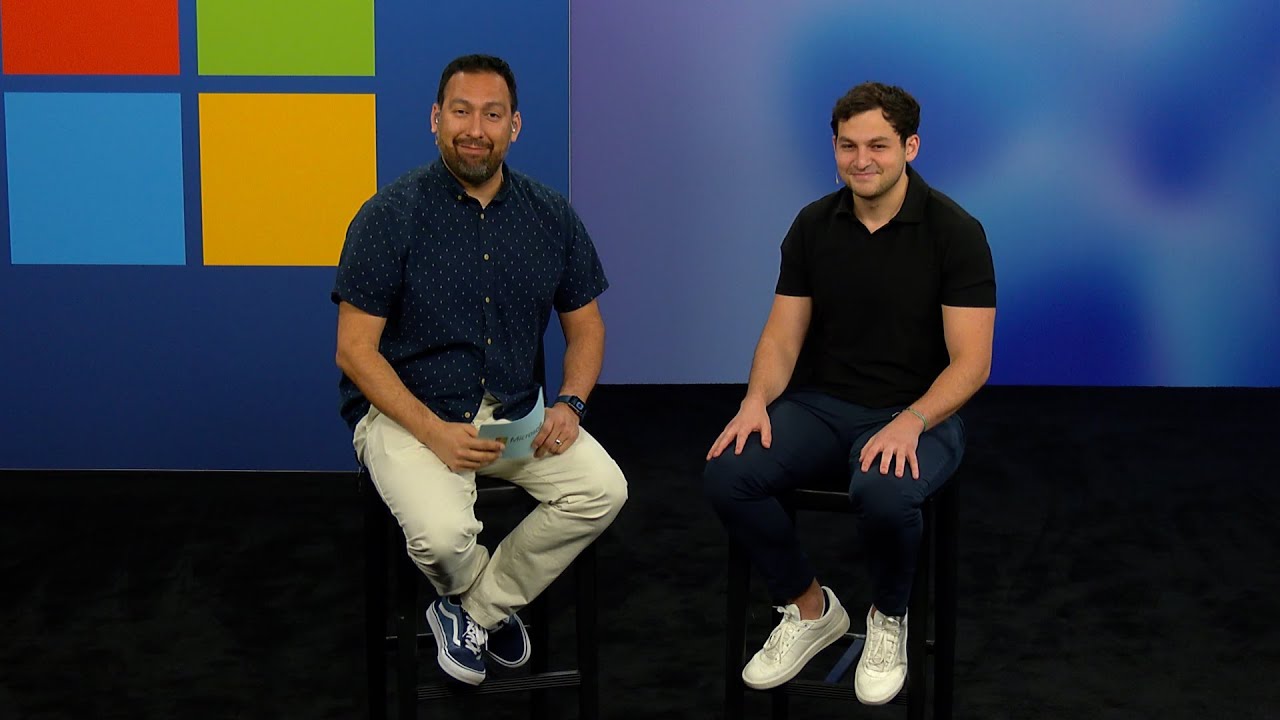
Building Impactful AI Products for the Masses: A Q&A Session with startup founder | Studio05
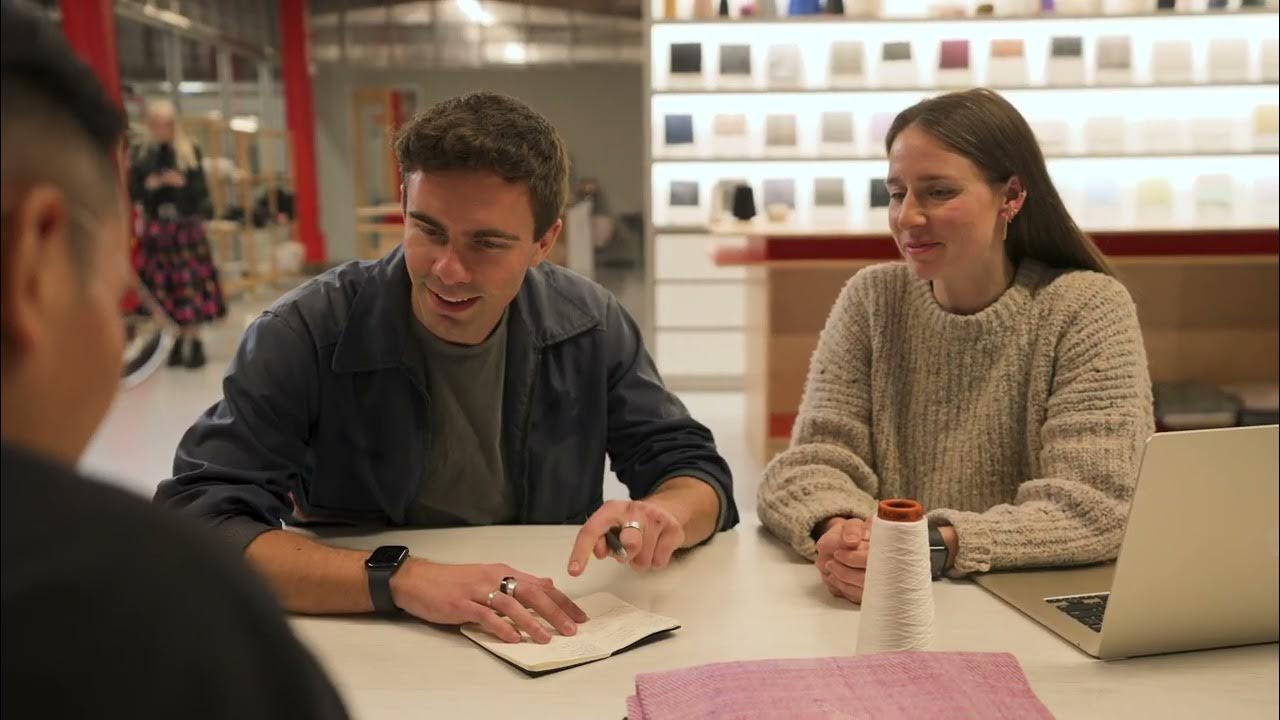
Your Next Pair of Walmart Pants Could Be 3D Woven

From AI to Infrastructure Empowering the Future of Data Centers Presented by Micas Networks
5.0 / 5 (0 votes)