Nvidia CEO Jensen Huang talks blowout quarter, AI, inferencing, ongoing demand, and more
Summary
TLDRYahoo財経のマーケットドミネーションで、テックエディターであるダン・ハウリーと共に、Nvidiaの創業者CEOジェンセン・ウォンが登場。Nvidiaは、強力な財務第1四半期のデータセンター収益が前年比4127%増加し、AI投資の勢いが続いていることを示した。ブラックウェルという次世代チップも今年発売予定で、その収益も今年中に大きくなると予想されている。さらに、10対1のフォワード株式分割と配当アップも発表された。ブラックウェルはトレンドパラメーターAIモデル向けに設計され、データセンターの技術革新に貢献する。AIファクトリーとして構築されたNvidiaのプラットフォームは、複雑なシステムで、高ボリュームでの製造が難しく、供給不足が続きそうだ。自動車業界もデータセンターの大きな分野となり、テスラをはじめとする自走車の分野でAI技術が重要な役割を果たしている。
Takeaways
- 🚀 Nvidiaは、予想をはるかに超える強力な財務第1四半期を報告し、データセンターの収益が前年比で4,127%増加しました。
- 📈 AIの支出の勢いは続いており、Nvidiaはまた楽観的な販売予測を発表しました。
- 🔄 Nvidiaは10対1のフォワード株式分割と配当金の引き上げを発表しました。
- 💡 BlackwellはNvidiaの次世代チップで、今年に出荷され、今年にはBlackwellの収益が大幅に寄与すると予想されています。
- 🌟 BlackwellはトレンドパラメータAIモデル向けに設計されており、データの処理量がモデルサイズの倍に増加するごとに4倍増加していることを考慮して設計されています。
- 🛠️ Blackwellは生成的なAIに特化しており、情報の生成に必要とされる非常に複雑でパフォーマンスの高い技術に対応しています。
- 🔄 推論は従来は単純でしたが、生成的なAIの登場により、推論は非常に複雑化しました。Nvidiaのアーキテクチャの多様性により、人々は新しいAIを継続的に革新し、創造することができます。
- 📊 Nvidiaは推論市場において優位性を持っており、世界中のデータセンターとウェブ上で行われている推論の大部分がNvidiaで行われています。
- 🤖 自動運転車市場では、Teslaが最も進んでおり、すべての自動車メーカーがデータセンター内でAIを利用してさまざまな機能を強化しています。
- 🌐 NvidiaはAIファクトリーと呼ばれる非常に複雑なシステムを構築し、それをパートナーがさまざまなデータセンターに取り込める形で分割しています。
- 📈 クラウドプロバイダーからの収益は現在、データセンター収益の40%前後ですが、他の業界がAI分野に参入することで、両者とも成長が見込まれています。
Q & A
Nvidiaはどのくらいの成長を遂げていますか?
-Nvidiaは強力な財務第1四半期でアナリストの予想を大幅に上回りました。データセンターの収益は年々4,127%増加しました。
ブラックウェルという次の世代のチップはどのようなものか、そして今年の収益にどのような影響を与えると予想されますか?
-ブラックウェルはAIモデルのトレンドパラメーターを扱うための設計で、今年にも出荷されると予想されており、ホッパーよりも高価な製品です。そのため、今年の収益は大幅に増加すると予想されています。
ブラックウェルチップはどのような特徴を持っていますか?
-ブラックウェルはトレンドパラメーターAIモデル用に設計されており、非常に高速な推論を行います。また、データセンターへの適応性にも優れており、空気冷却、液体冷却、x86または新しく設計されたGraceブラックウェルスーパーチップに対応しています。
Nvidiaはどのようにして推論市場での競争優位を維持する予定ですか?
-Nvidiaは推論が非常に複雑な問題であること、そして多様なモデルを使用するソフトウェアスタックの複雑性から、競争優位を維持する戦略を立てています。ブラックウェルの登場もその一環です。
供給不足はどのように対処する予定ですか?
-ブラックウェルとホッパーのチップは非常に高い需要があり、供給が不足しているとのことです。NvidiaはAIファクトリーを構築し、それをパートナーに提供することで対処する予定です。
クラウドプロバイダーと他の業界はどのようにNvidiaのデータセンター収益に寄与していますか?
-クラウドプロバイダーはデータセンター収益の40%程度を占めていますが、他の業界もAIを活用し始めており、将来的には双方が成長すると予想されています。
NvidiaはどのようにしてAIファクトリーを構築していますか?
-NvidiaはCPU、GPU、複雑なメモリを組み合わせ、MVリンクやInfiniBandスイッチ、Ethernetスイッチなどを使ってAIファクトリーを構築しています。これらは非常に複雑なスパインと呼ばれるmvyリンクで接続され、ソフトウェアによって管理されています。
自動車業界においてNvidiaのデータセンターはどのような役割を果たしていますか?
-自動車業界はデータセンターの中で最も大きな企業分野となっています。Nvidiaは自走車のトレーニングに必要な膨大なビデオデータを処理するAI技術を提供しています。
ブラックウェルはどのような種類のデータセンターに適応できますか?
-ブラックウェルは空気冷却、液体冷却、x86、Graceブラックウェルスーパーチップ、InfiniBandデータセンター、Ethernetデータセンターなど、多様なデータセンターに適応できます。
Nvidiaは今後どのような市場ニーズに対応する予定ですか?
-Nvidiaは今後もAIの多様な分野における需要に対応し、特に物理世界を理解し、ビデオから学ぶ新しいタイプのAI技術に焦点を当てています。
NvidiaはどのようにしてAI技術を進化させていく予定ですか?
-Nvidiaはビデオから学ぶことによって物理世界を理解するAI技術を開発し、多様な業界でその能力を拡大する予定です。また、ビデオを用いたトレーニング能力も強化していく予定です。
Outlines
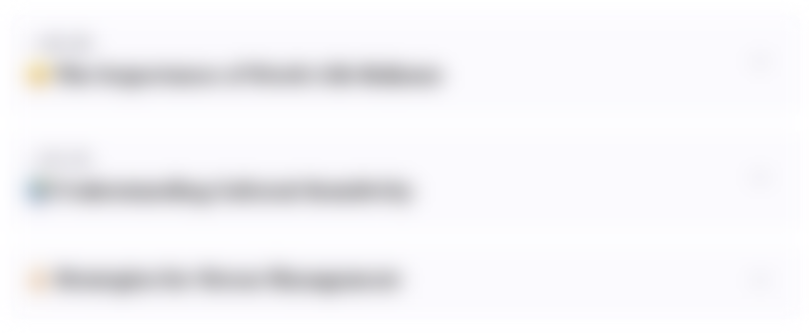
This section is available to paid users only. Please upgrade to access this part.
Upgrade NowMindmap
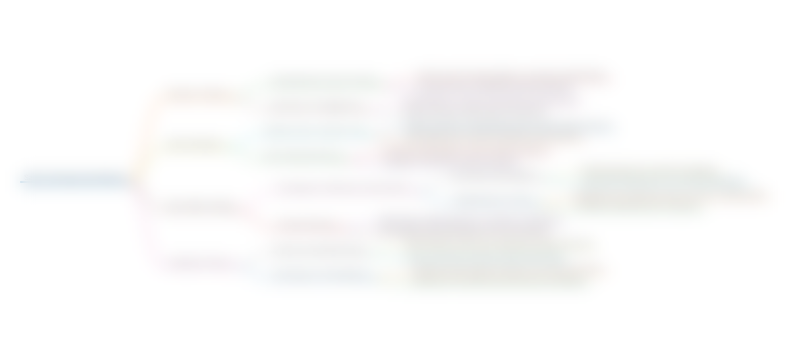
This section is available to paid users only. Please upgrade to access this part.
Upgrade NowKeywords
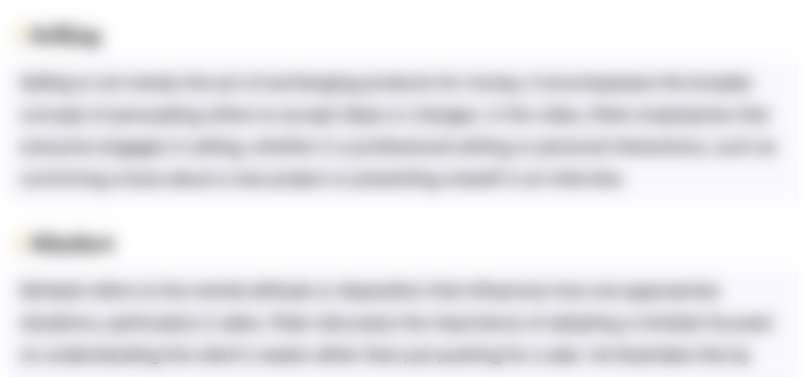
This section is available to paid users only. Please upgrade to access this part.
Upgrade NowHighlights
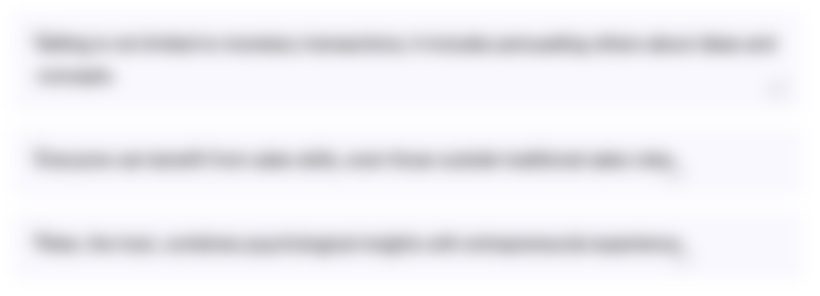
This section is available to paid users only. Please upgrade to access this part.
Upgrade NowTranscripts
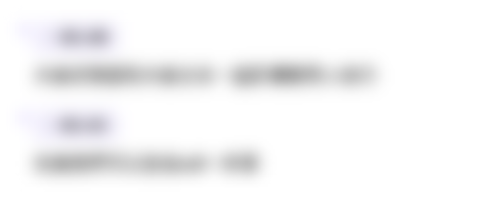
This section is available to paid users only. Please upgrade to access this part.
Upgrade NowBrowse More Related Video
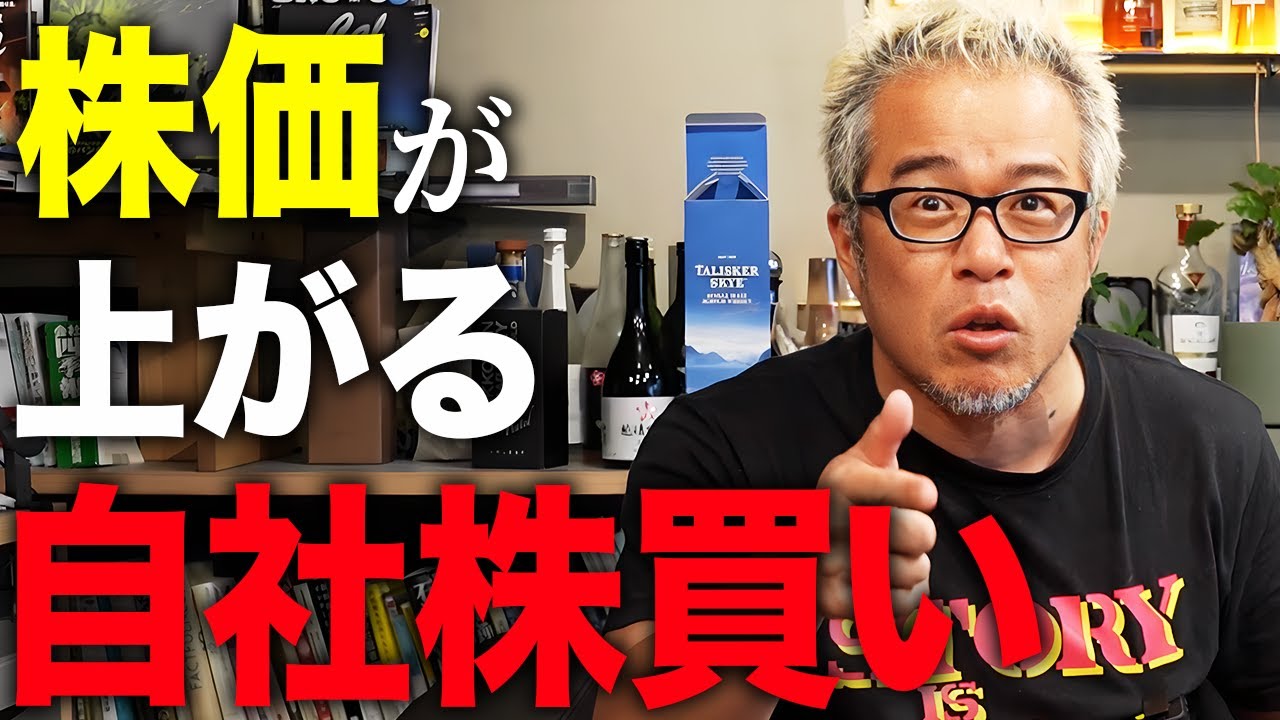
自社株買いが株価を上げる理由を解説します。
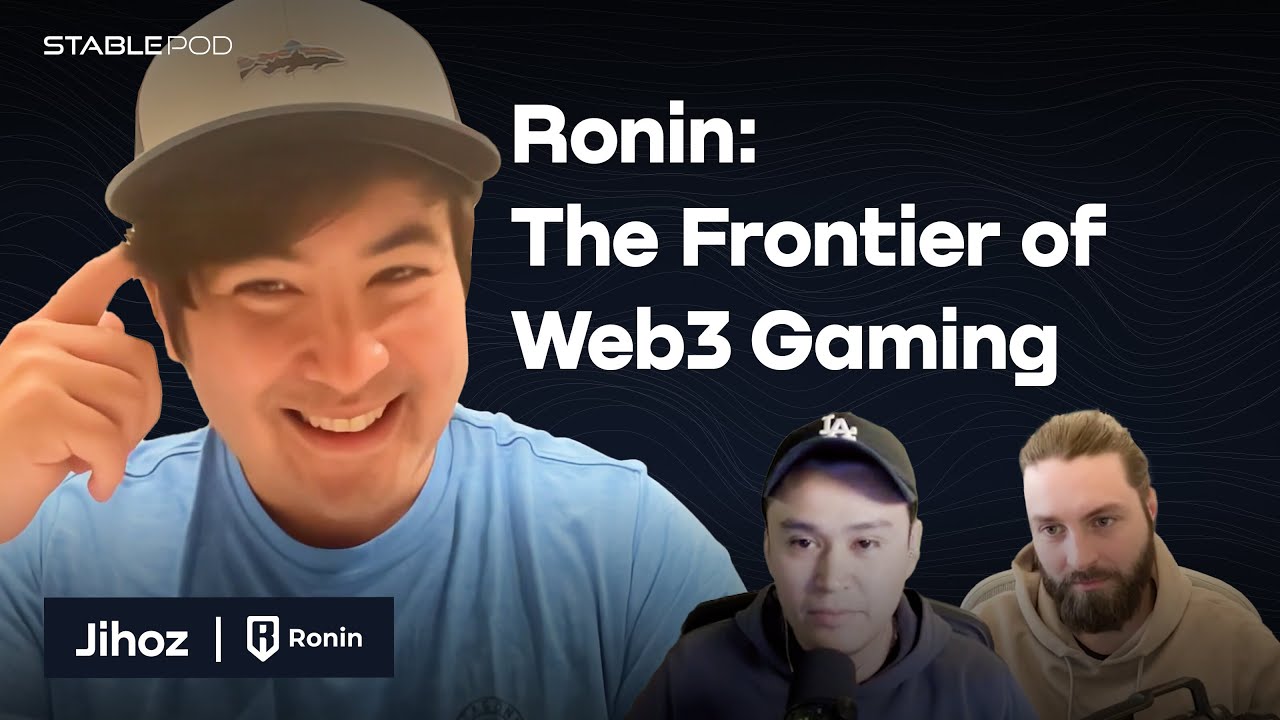
Ronin: The Frontier of Web3 Gaming w/ Jihoz (Ronin)
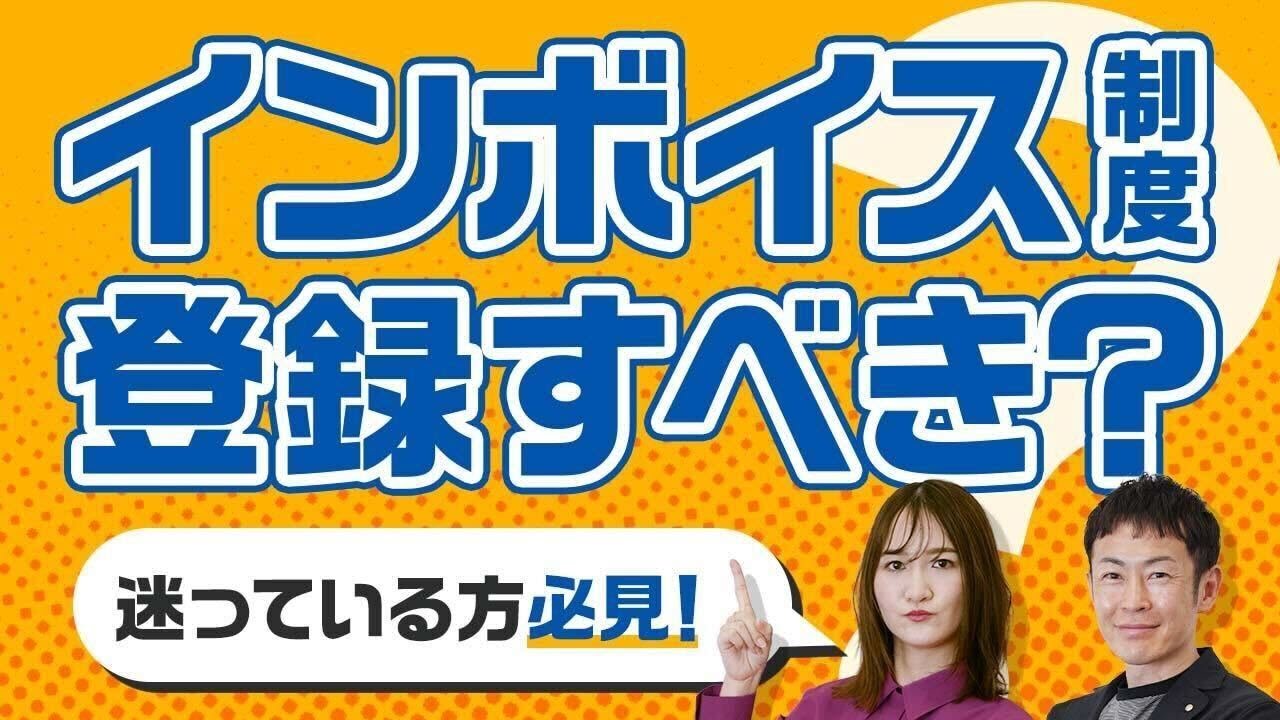
【インボイス制度】免税事業者は課税事業者になるべき?判断基準やメリット・デメリットを解説!

Sam Altman talks with MIT President about AI (May 2024)

Jim Cramer breaks down Nvidia earnings
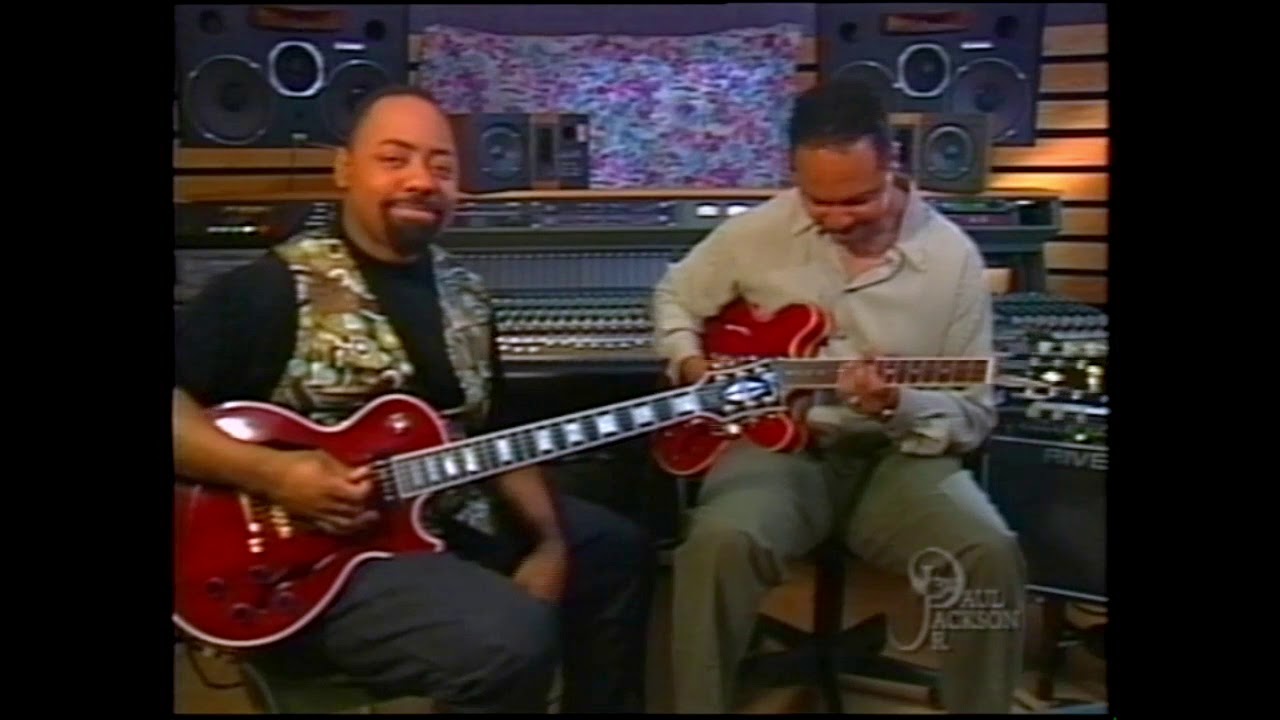
The Science Of Rhythm Guitar, Chapter 8-The Concept Of Space, Part 2(featuring Ray Parker Jr.)
5.0 / 5 (0 votes)