Open Source "Thinking" Models Are Catching Up To OpenAI o1 Already...
Summary
TLDRThe video explores the latest developments in AI reasoning models, focusing on a range of breakthroughs by companies like Deep Seek, Quinn, and Lava. It highlights the shift from traditional layer-stacking to test-time compute (TTC) for scaling AI, which improves reasoning accuracy by using step-by-step logic. The video covers the competition between AI labs, showcasing Deep Seek's R1 Light and Quinn's 32B models, and the emergence of new methodologies like the Journey learning paradigm. Additionally, the narrator reflects on the challenges and potential of these models in tackling complex reasoning tasks, such as the Riemann Hypothesis.
Takeaways
- 😀 OpenAI's GPT-4 and other AI models have struggled with reasoning tasks, as shown in a test comparing their accuracy on simple date difference calculations.
- 😀 Test-time compute (TTC) is emerging as a key method to scale AI reasoning, focusing on inference time and generating multiple reasoning steps before arriving at an answer.
- 😀 DeepSeek's R1 model, despite being a lightweight and early preview, shows potential in reasoning transparency, although it doesn't always outperform OpenAI's models in accuracy.
- 😀 DeepSeek's R1, when given a reasoning problem, struggled with basic tasks like calculating the number of days between two dates, unlike OpenAI's GPT-4 which handled it correctly every time.
- 😀 Despite its potential, DeepSeek R1 suffers from a strict context window limit that reduces its ability to handle large inputs, such as PDFs, affecting its summarization quality.
- 😀 DeepSeek's release of its scaling law for reasoning models aligns with OpenAI's previous predictions, further validating the importance of improving reasoning chains in AI.
- 😀 Models like DeepSeek R1 and Quinn 32B are showing promising performance, with Quinn 32B outperforming other models like GPT-4 in certain benchmarks, even when quantized.
- 😀 The introduction of a Chain of Thought (CoT) method, as seen in Lava Chain of Thought, brings systematic reasoning to vision-language tasks, improving model performance on complex reasoning questions involving images or diagrams.
- 😀 Marco1, another reasoning model, uses Monte Carlo Tree Search (MCTS) for test-time compute, providing a strong documented approach for complex search and inference tasks.
- 😀 Research on replicating OpenAI’s reasoning abilities, particularly through a project called 01 Journey, introduces a novel training paradigm (Journey Learning), focused on synthesizing long reasoning processes and integrating trial-and-error corrections, advancing the field of AI reasoning.
Q & A
What is the key difference between OpenAI's reasoning model (01) and DeepSeek's R1 light preview model?
-The key difference lies in the transparency of their thought processes. DeepSeek's R1 model provides a more transparent real-time reasoning process, while OpenAI's 01 model is more focused on implicit reasoning. However, DeepSeek's model is still in an early preview stage and may not perform as well as OpenAI's 01 in certain tasks.
What does TTC (Test Time Compute) mean in the context of scaling AI models?
-Test Time Compute refers to using additional inference time compute during the model's operation to generate more tokens that help in the reasoning process. This method enhances model performance by allowing the model to 'think' step-by-step, rather than relying on intuition alone.
Why did the script mention 'implicit reasoning' and how does it affect AI model performance?
-Implicit reasoning refers to the intuitive, fast, and less systematic thinking used by models like GPT-4, which may rely heavily on memorization and intuition. This can result in less reliable performance for complex or out-of-distribution tasks, whereas explicit step-by-step reasoning helps improve accuracy and reliability.
How did the reasoning performance of OpenAI's 01 preview compare to DeepSeek's R1 in solving simple date-related math problems?
-In solving a basic date-related problem, OpenAI's 01 preview got the correct answer 1 out of 5 times, while DeepSeek's R1 light preview didn't get it right at all. This illustrates some of the limitations of the newer model despite its transparency.
What are some reasons behind OpenAI's 01 model underperforming in certain tasks, according to the script?
-The underperformance of OpenAI's 01 model may be due to its reliance on implicit reasoning, which is faster but less reliable for complex tasks. This contrasts with more structured reasoning models that perform better when forced to think step-by-step.
What is the significance of DeepSeek's model being able to publish weights for local use?
-DeepSeek's ability to publish weights for local use is a significant development because it allows users to run the model independently, unlike OpenAI's 01, which doesn't offer this option. This marks a major shift in accessibility and transparency in AI research.
What is the proposed advantage of Chain of Thought reasoning in Lava Chain of Thought models?
-The Chain of Thought reasoning in Lava Chain of Thought models is designed to break down the answer generation process into structured stages, such as summarization, visual interpretation, logical reasoning, and conclusion generation. This systematic approach helps improve performance, particularly on vision-related tasks with complex diagrams or images.
What is the challenge in using AI models for complex reasoning tasks, and how are researchers addressing this challenge?
-One challenge in using AI for complex reasoning tasks is the difficulty of models in reasoning step-by-step, especially when encountering out-of-distribution data. Researchers are addressing this by developing models that generate intermediate reasoning steps and combining search techniques like trial and error correction and backtracking to improve accuracy.
What did the author mention about the performance of the Quill 32b model compared to larger models?
-The Quill 32b model, despite having fewer parameters than larger models like Llama 40b or GPT-4, outperformed them in certain benchmarks. This demonstrates that a smaller, well-tuned model can sometimes outperform larger models depending on the task.
How does the concept of 'out-of-distribution' data relate to AI reasoning models like OpenAI's 01?
-'Out-of-distribution' data refers to situations where AI models encounter tasks or problems they haven't been trained on. Models like OpenAI's 01 are more likely to succeed in reasoning tasks with explicit step-by-step processes, even in these situations, because they rely on reasoning rather than intuition alone.
Outlines
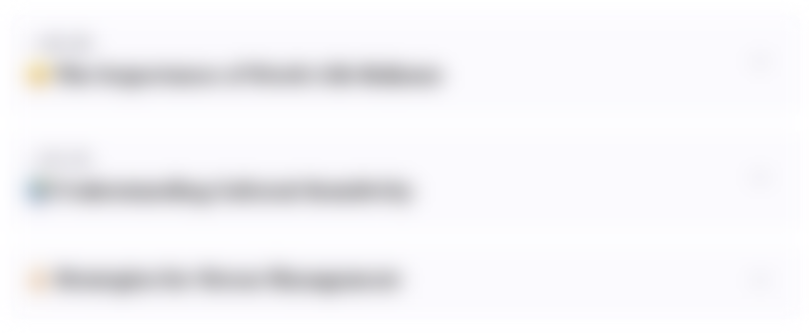
This section is available to paid users only. Please upgrade to access this part.
Upgrade NowMindmap
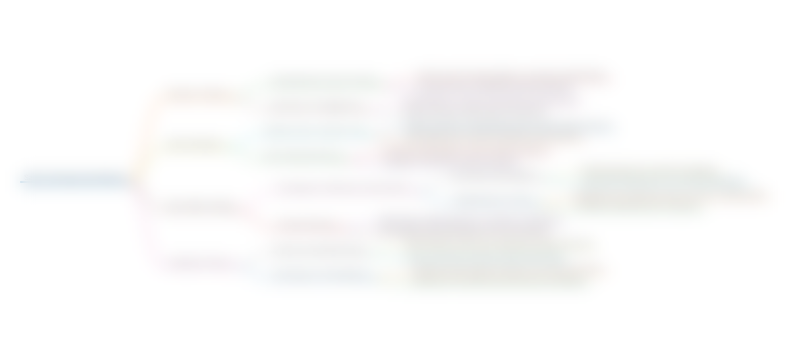
This section is available to paid users only. Please upgrade to access this part.
Upgrade NowKeywords
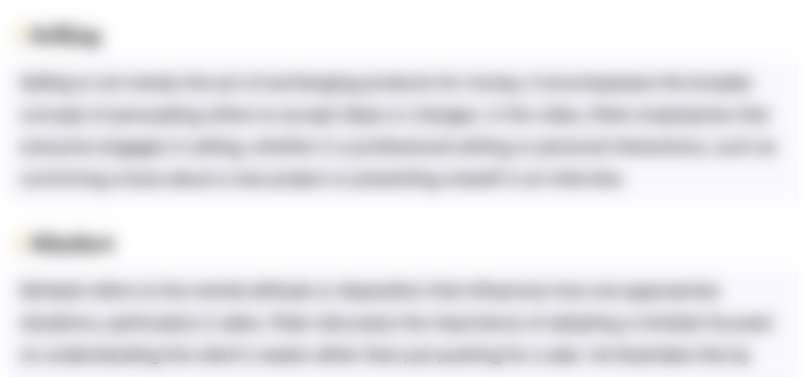
This section is available to paid users only. Please upgrade to access this part.
Upgrade NowHighlights
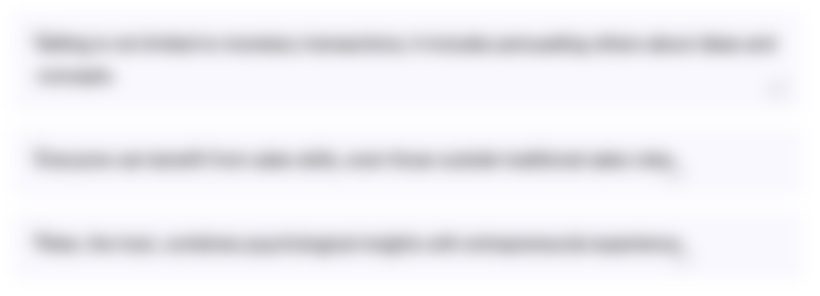
This section is available to paid users only. Please upgrade to access this part.
Upgrade NowTranscripts
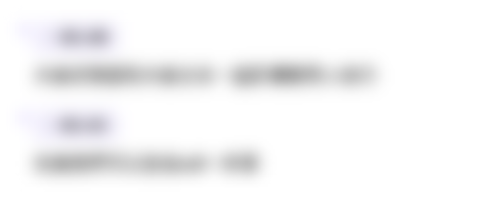
This section is available to paid users only. Please upgrade to access this part.
Upgrade NowBrowse More Related Video
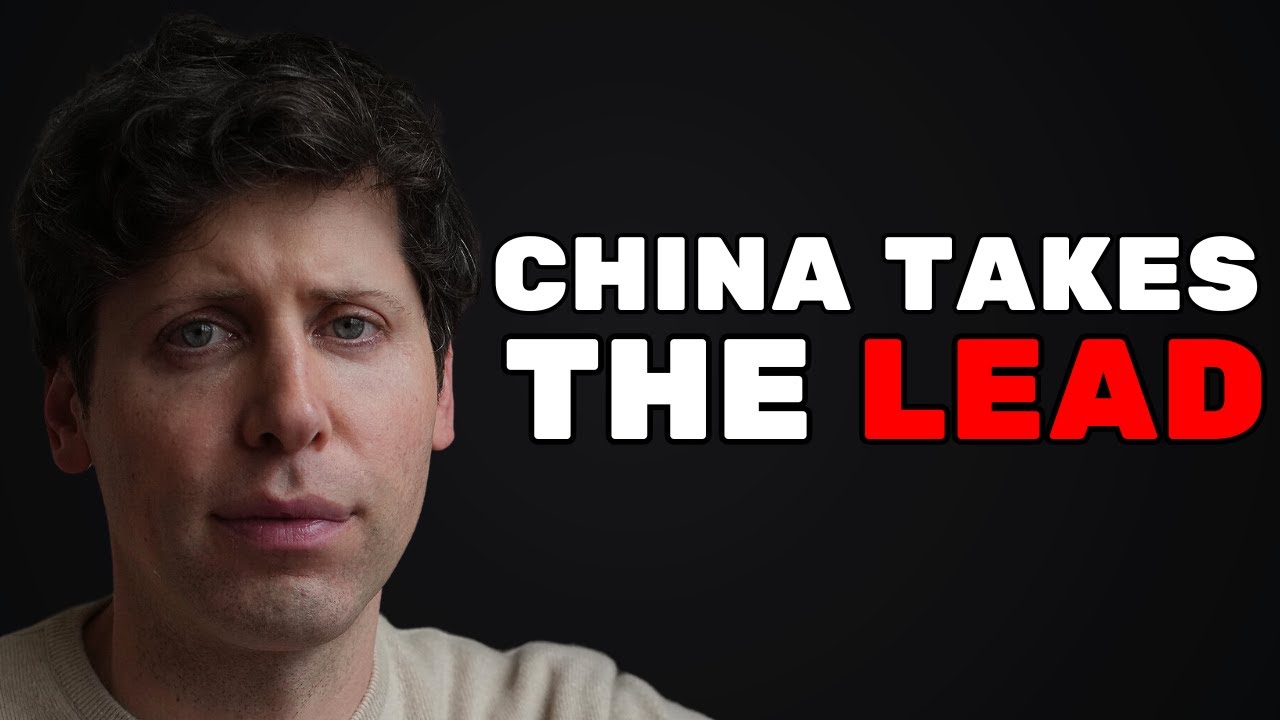
China's 'DeepSeek R1' DEFEATS OpenAI's o1! AI Art Turing Test, Figure 02 Update
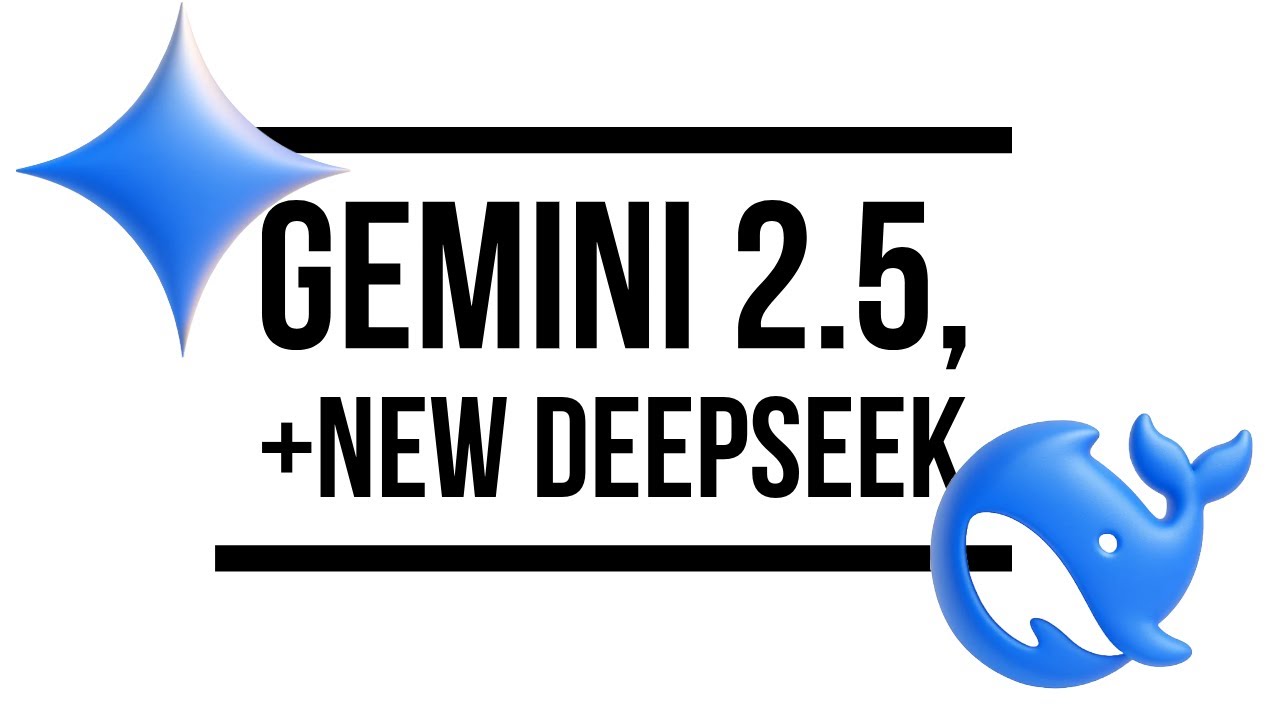
Did AI Just Get Commoditized? Gemini 2.5, New DeepSeek V3, & Microsoft vs OpenAI
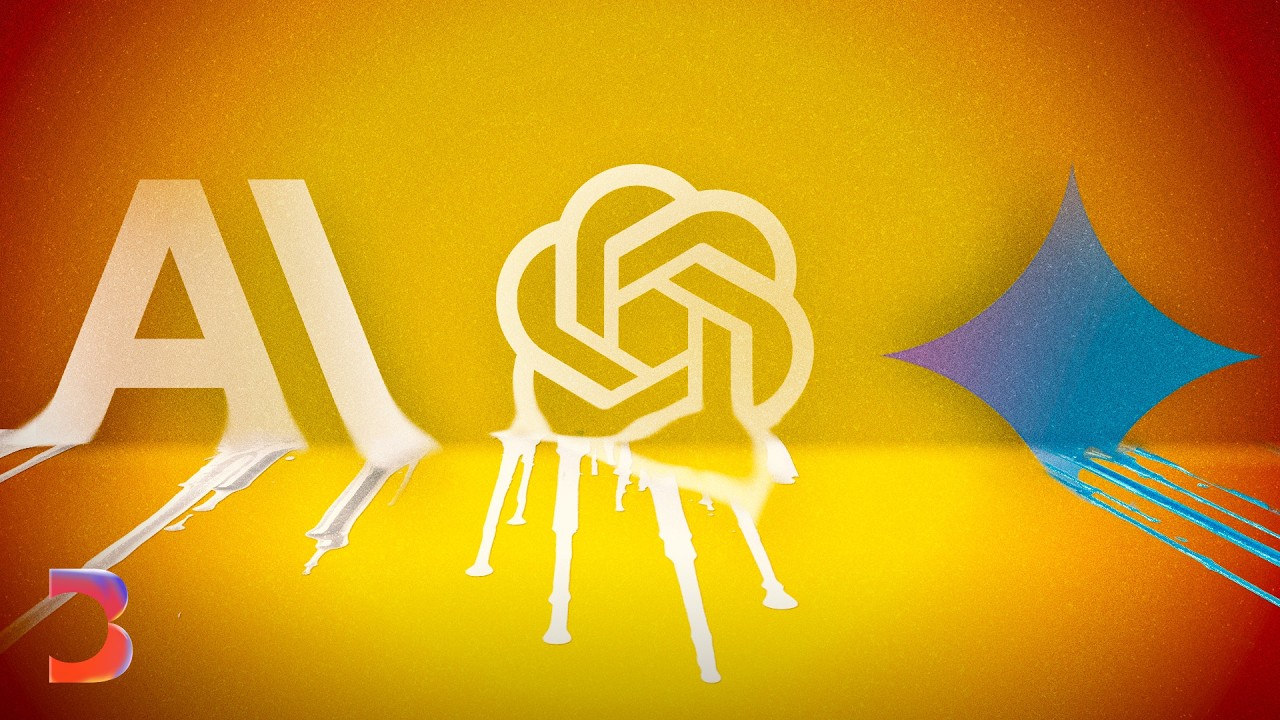
How AI Got a Reality Check

AI Czar David Sacks Explains the DeepSeek Freak Out
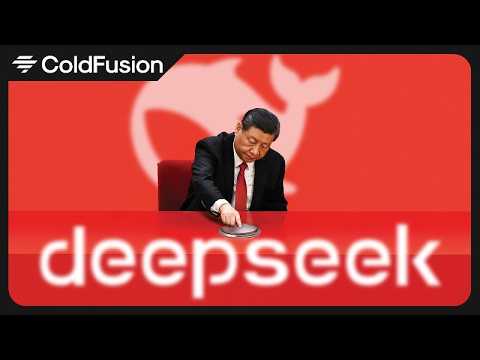
China’s DeepSeek - A Balanced Overview
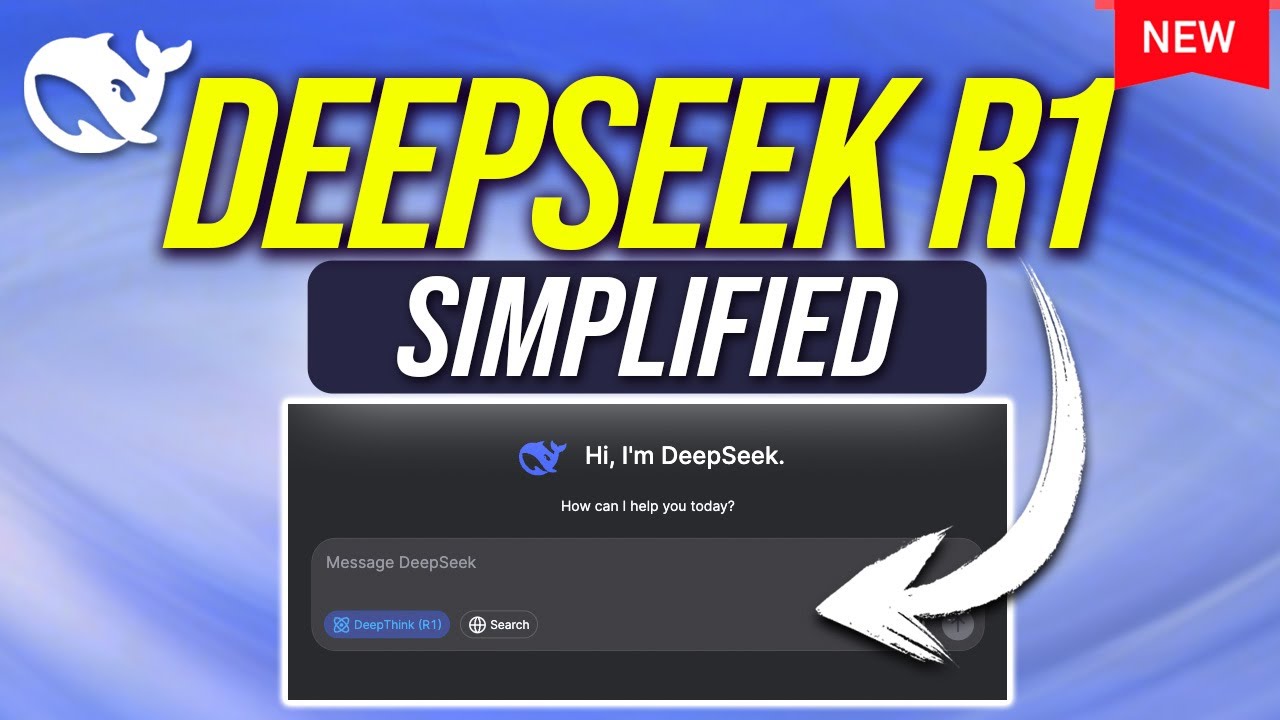
DeepSeek R1 Explained to a 10 Year Old
5.0 / 5 (0 votes)