How OpenAI Trained SORA & What CTO is NOT Saying...
Summary
TLDRAI-driven video generation models like OpenAI's Sora and Apple's Vision Pro are revolutionizing how we interact with both digital and physical environments. By combining large-scale AI models, spatial computing, and photorealistic simulations, these technologies enable real-time, voice-driven manipulation of environments. The integration of platforms like Unreal Engine and vast datasets from companies like Microsoft and Google further enhances realism. As the line between digital and physical spaces blurs, we are entering an era where immersive, AI-powered simulations are reshaping our perception of reality, making the idea of living in a simulation feel increasingly plausible.
Takeaways
- 😀 AI is creating hyper-realistic visuals that are indistinguishable from reality, enabling new possibilities in video generation.
- 😀 OpenAI’s Sora model uses space-time patches, similar to tokens in text models, to represent video data, allowing for dynamic simulations of environments.
- 😀 Sora, trained on massive datasets, can simulate aspects of people, animals, and environments in 3D space without explicit knowledge of physics.
- 😀 The integration of AI models with Apple’s Vision Pro and spatial computing allows users to interact with and modify their real-time environment using voice commands.
- 😀 Sora's simulation abilities suggest that large-scale video models could enable highly capable simulators of both physical and digital worlds.
- 😀 Sora’s training likely involved large datasets from platforms like YouTube, gaming footage, and possibly even surveillance footage, raising concerns about data sources.
- 😀 The future of AI video models could lead to the ability to modify real environments in real-time, blending physical and virtual spaces seamlessly.
- 😀 Advances in AI video generation require massive computational resources, which are only available to a few companies like OpenAI and Google.
- 😀 Environmental concerns are significant, as generating AI images and videos consumes vast amounts of electricity, with video generation being especially power-hungry.
- 😀 The combination of large datasets, powerful cloud services like Microsoft’s, and Unreal Engine’s photorealistic capabilities could fuel further advancements in AI video generation.
Q & A
What is Sora, and how does it contribute to AI-generated video content?
-Sora is an AI video generation model that uses space-time patches, similar to tokens in text-based models, to simulate highly realistic environments, people, and animals. By learning from massive datasets, Sora can generate lifelike video content and understand complex 3D physics and spaces at scale.
What are space-time patches, and how do they function in Sora's video generation process?
-Space-time patches are essentially visual tokens that represent video and image latent codes. These patches allow Sora to process and generate dynamic, realistic videos by breaking down video data into manageable components, similar to how text tokens function in language models.
Why is the concept of 'scale' important for Sora's video simulation capabilities?
-Scale refers to the massive amount of data and computational power required to train models like Sora. As the model is exposed to more data, it begins to simulate real-world dynamics, such as movement and physics, with higher fidelity. This scale is key to unlocking the model's potential to create photorealistic video simulations.
What role does Apple Vision Pro play in the development of AI-driven video environments?
-Apple Vision Pro, with its spatial computing and AI vision, is designed to overlay digital content onto the real world. This combination will allow users to interact with and modify their real environment in real-time using voice commands, creating an immersive experience where digital and physical worlds seamlessly blend.
How might Unreal Engine contribute to the training of AI models like Sora?
-Unreal Engine, known for its photorealistic rendering capabilities, could serve as a valuable tool for training AI models like Sora. By generating synthetic datasets of highly realistic environments, Unreal Engine can provide AI models with a vast range of visual data, improving their ability to simulate complex 3D spaces and interactions.
What is the environmental impact of AI video generation, and why is it a concern?
-AI video generation requires significant computational resources, which can lead to a high environmental cost. Text-to-image models already consume as much energy as charging a smartphone, and video generation is even more energy-intensive. This raises concerns about the sustainability of large-scale AI models and their environmental footprint.
How do public and private datasets contribute to the training of AI models like Sora?
-Public datasets, such as Kinetics 600 and YouTube's vast library of videos, provide open access to large amounts of video content for training AI models. Private datasets, like those from Microsoft and Shutterstock, offer additional valuable resources, including game recordings, video calls, and even surveillance footage, which can be used to enhance the model's capabilities.
What implications does the increasing data traffic from video content have on AI model training?
-Video data accounts for over half of global internet traffic, making it an essential resource for AI models that focus on visual content. With platforms like YouTube processing massive amounts of video data, this influx of content can significantly aid in training AI models to generate more accurate, high-fidelity video simulations.
What could be the future of AI video generation with platforms like Google's Gemini and OpenAI's Sora?
-With the advances in AI, particularly through platforms like Google's Gemini, which boasts a 10 million token limit, and OpenAI's Sora, the future of AI video generation looks incredibly promising. These technologies could enable the creation of interactive, dynamic environments and real-time video content that responds to user input, blurring the lines between digital and physical worlds.
What are the potential applications of AI-driven video and AR technologies in everyday life?
-AI-driven video and AR technologies could revolutionize how we interact with our environment. With devices like the Apple Vision Pro, users could modify their surroundings in real-time, creating dynamic virtual environments or altering physical spaces. Additionally, AI avatars could enhance communication, transforming how we interact remotely or socially.
Outlines
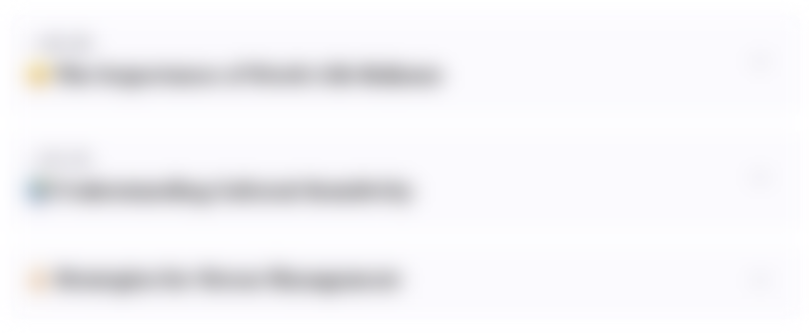
This section is available to paid users only. Please upgrade to access this part.
Upgrade NowMindmap
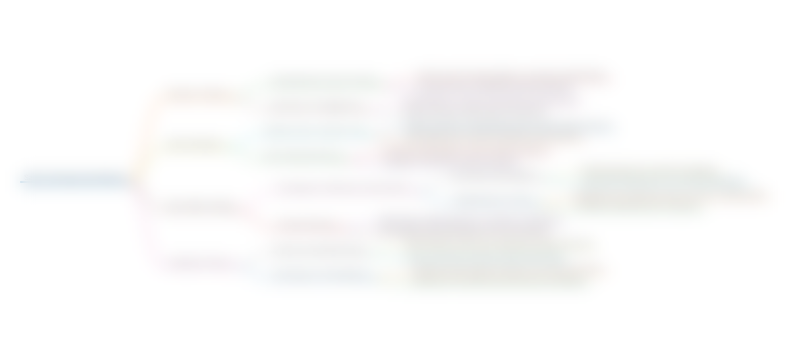
This section is available to paid users only. Please upgrade to access this part.
Upgrade NowKeywords
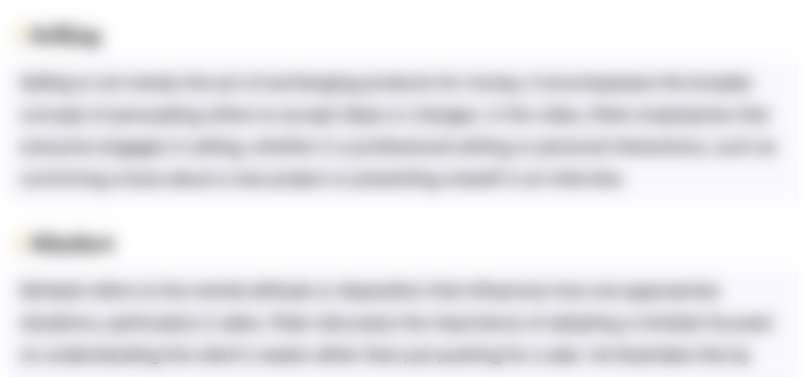
This section is available to paid users only. Please upgrade to access this part.
Upgrade NowHighlights
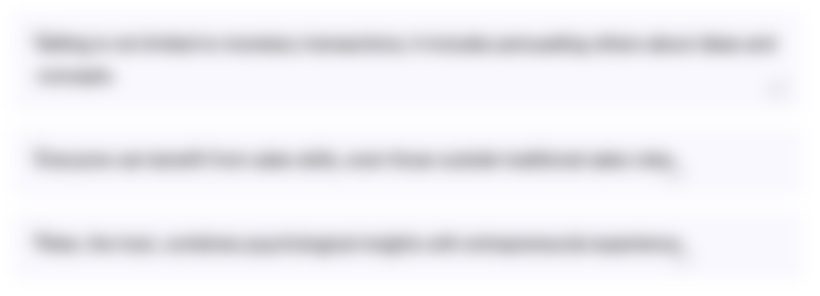
This section is available to paid users only. Please upgrade to access this part.
Upgrade NowTranscripts
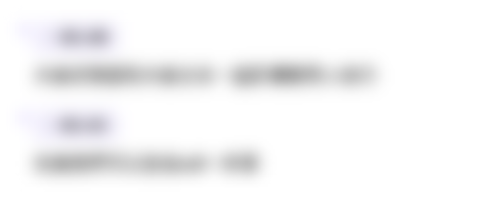
This section is available to paid users only. Please upgrade to access this part.
Upgrade Now5.0 / 5 (0 votes)