Decision and Classification Trees, Clearly Explained!!!
Summary
TLDRIn this StatQuest video, Josh Darmer introduces decision and classification trees, explaining their structure and functionality. He details how to build a classification tree using raw data, demonstrating the process of choosing the best questions to split data based on impurity measures like Gini impurity. Through an example involving preferences for the movie 'Cool as Ice,' he illustrates how to analyze data and make predictions. The video emphasizes the importance of avoiding overfitting and highlights techniques like pruning and cross-validation to ensure reliable predictions. With clear explanations, it serves as a helpful resource for understanding decision trees.
Takeaways
- 😀 Decision trees help classify data by making decisions based on true or false statements.
- 😀 Classification trees categorize data, while regression trees predict numeric values.
- 😀 Mixing numeric and categorical data in a decision tree is acceptable.
- 😀 The top of a decision tree is called the root node, with internal nodes and leaf nodes below it.
- 😀 A decision tree can become impure if its leaves contain mixtures of categories.
- 😀 Gini impurity is a common method for quantifying the impurity of leaves in a decision tree.
- 😀 The lower the Gini impurity, the better the feature predicts the target variable.
- 😀 When using numeric data, thresholds are calculated to create splits in the tree.
- 😀 Overfitting can occur if too few data points are used to make predictions, and this can be mitigated through pruning or setting limits on leaf size.
- 😀 Cross-validation is used to determine the best parameters for building a decision tree.
Q & A
What are decision trees and classification trees?
-Decision trees are a model used for decision-making, where a series of questions lead to a conclusion. Classification trees are a type of decision tree that categorizes data into discrete classes based on certain features.
What is the difference between a classification tree and a regression tree?
-A classification tree predicts categorical outcomes, while a regression tree predicts numeric values. This distinction is important depending on the type of data being analyzed.
How do you build a classification tree from raw data?
-To build a classification tree, start by determining which feature to split on first (e.g., 'loves popcorn' or 'loves soda'). You analyze how well each feature predicts the target variable and select the one with the highest predictive power.
What is Gini impurity, and why is it used?
-Gini impurity is a measure used to quantify the impurity of a leaf in a classification tree. It helps determine how well a particular split separates the classes within a dataset.
What does it mean when a leaf is described as 'impure'?
-An impure leaf contains a mix of different classes (e.g., some individuals love 'Cool as Ice' and some do not), making it difficult to predict the outcome accurately.
What role does pruning play in decision trees?
-Pruning is a technique used to reduce the size of a decision tree by removing branches that provide little predictive power, which helps prevent overfitting to the training data.
What is the significance of cross-validation in building decision trees?
-Cross-validation is used to evaluate the performance of the decision tree by testing it on different subsets of data. It helps determine the best model configuration and prevents overfitting.
How do you decide which feature to place at the root of the tree?
-You analyze the Gini impurity values for each feature and select the one that results in the lowest impurity after the first split, indicating it provides the most information about the target variable.
What are leaf nodes in a decision tree?
-Leaf nodes are the terminal points of a decision tree where no further splits occur. They represent the final classification outcome based on the data that reaches them.
Why is it important to consider the number of people in each leaf?
-The number of people in each leaf affects the confidence in predictions made by the tree. Fewer people in a leaf may lead to unreliable classifications, hence limits can be set on how many samples must be in a leaf.
Outlines
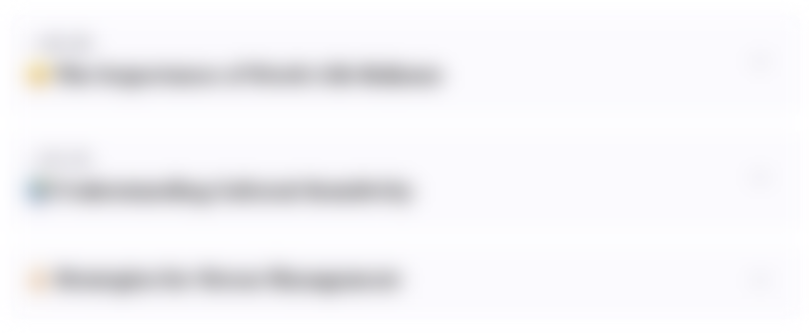
This section is available to paid users only. Please upgrade to access this part.
Upgrade NowMindmap
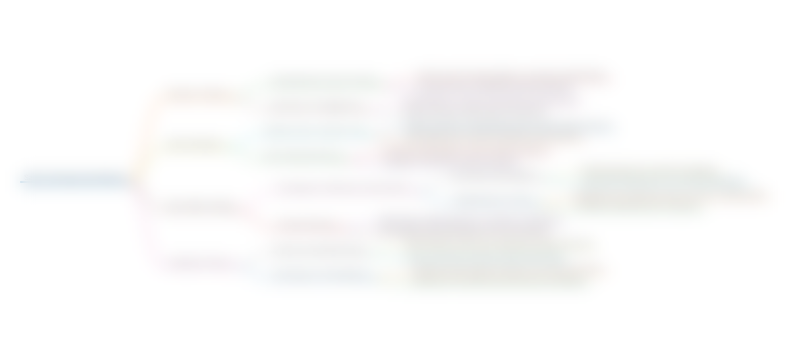
This section is available to paid users only. Please upgrade to access this part.
Upgrade NowKeywords
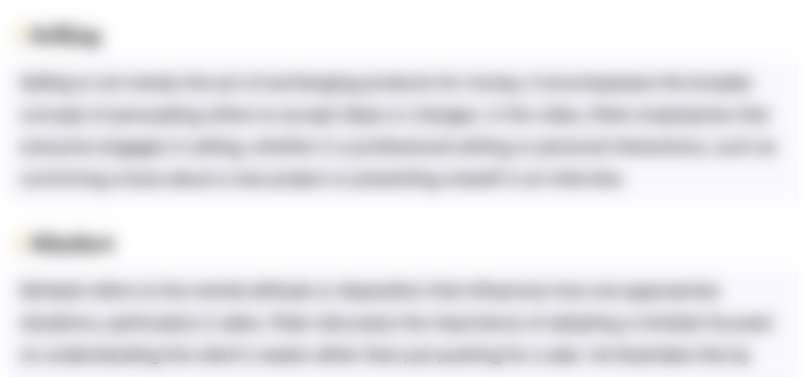
This section is available to paid users only. Please upgrade to access this part.
Upgrade NowHighlights
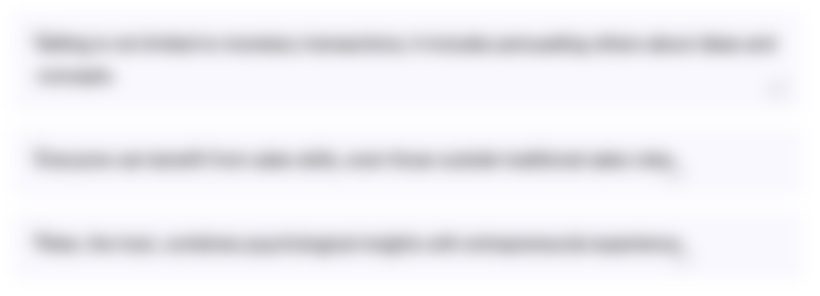
This section is available to paid users only. Please upgrade to access this part.
Upgrade NowTranscripts
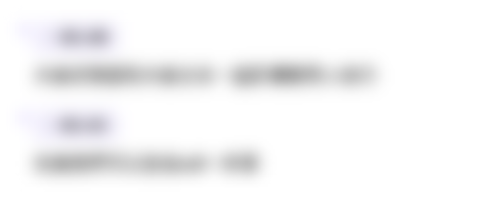
This section is available to paid users only. Please upgrade to access this part.
Upgrade NowBrowse More Related Video
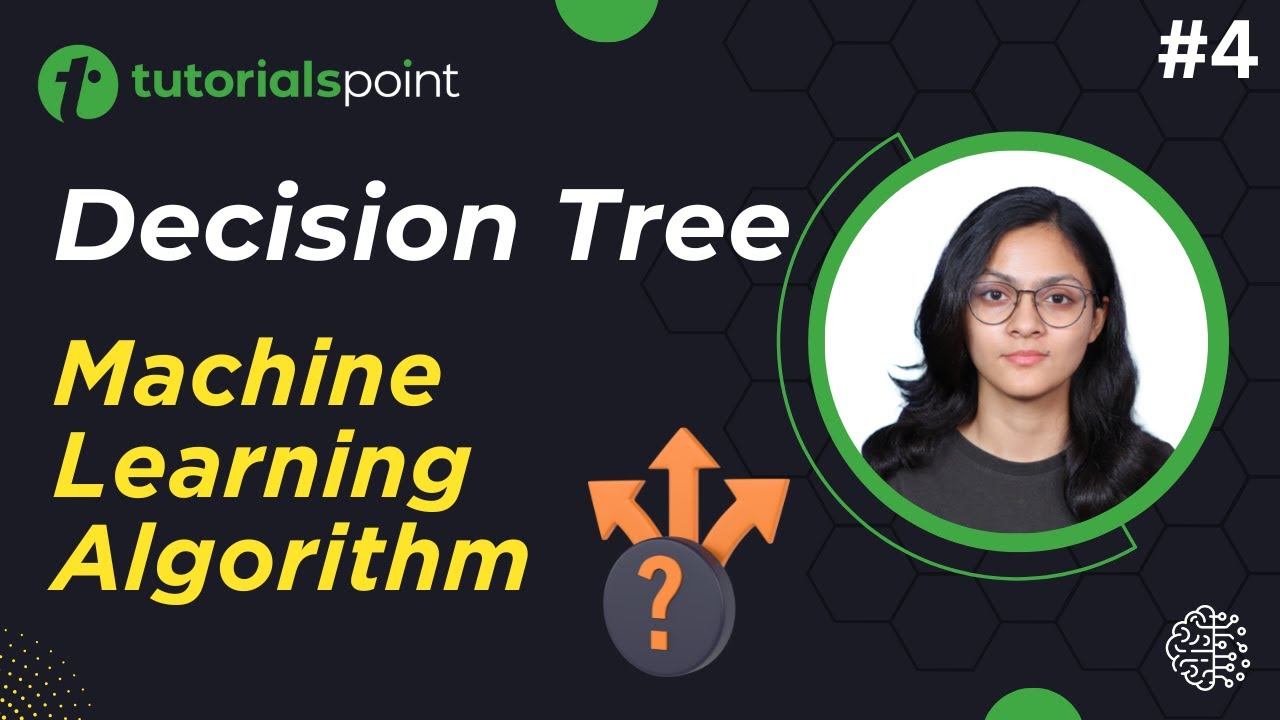
Decision Tree Algorithm | Decision Tree in Machine Learning | Tutorialspoint

Pemikiran dan Prediksi Analitik Data (Data Analytic Thinking and Prediction)
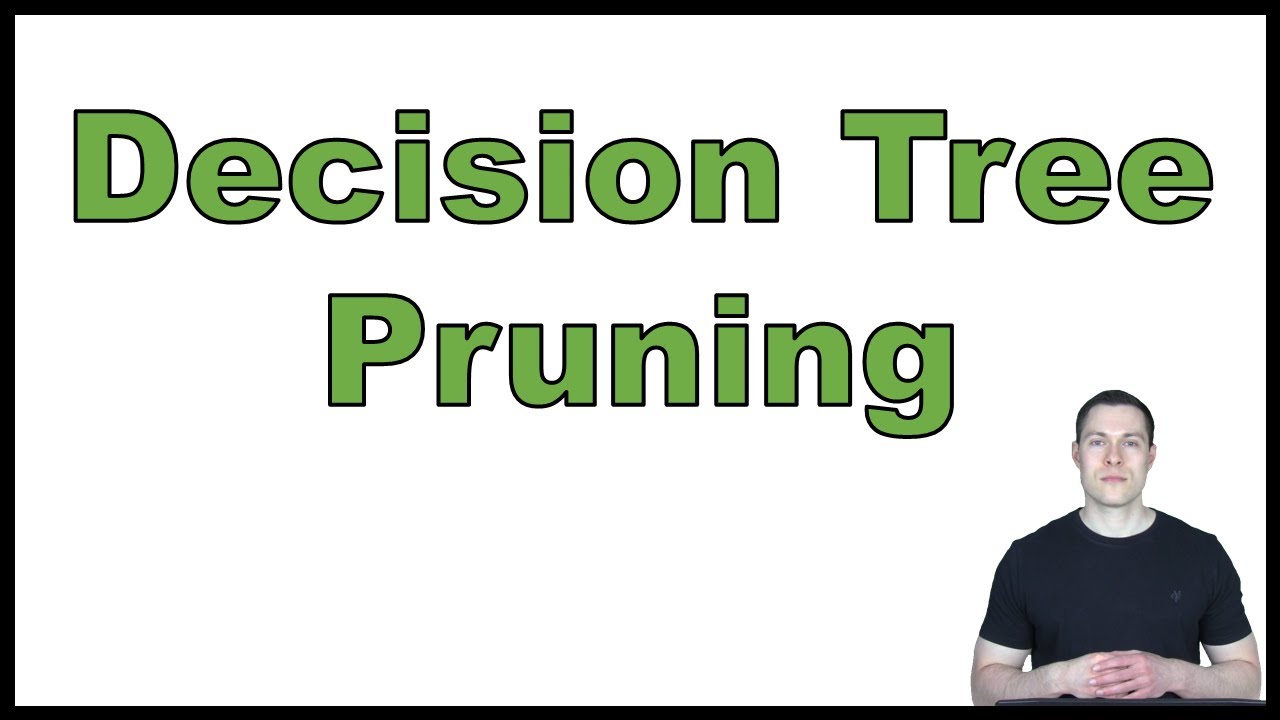
Decision Tree Pruning explained (Pre-Pruning and Post-Pruning)
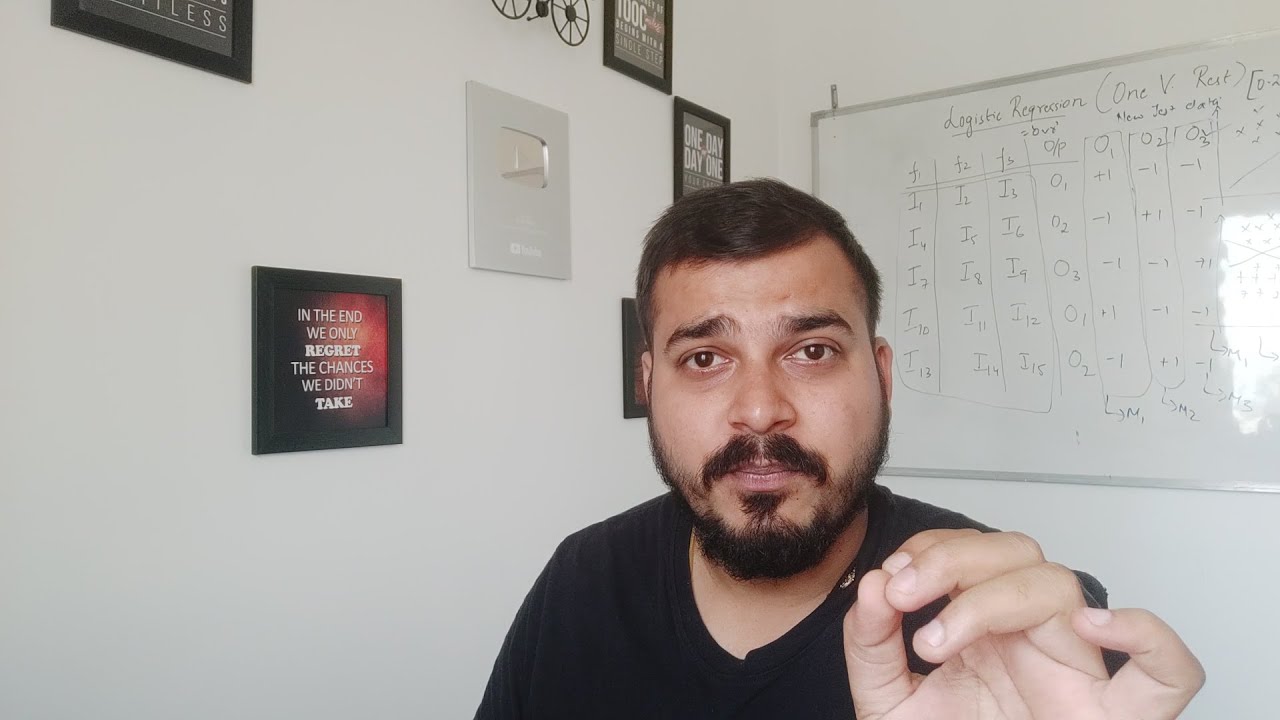
Interview Questions On Decision Tree- Data Science
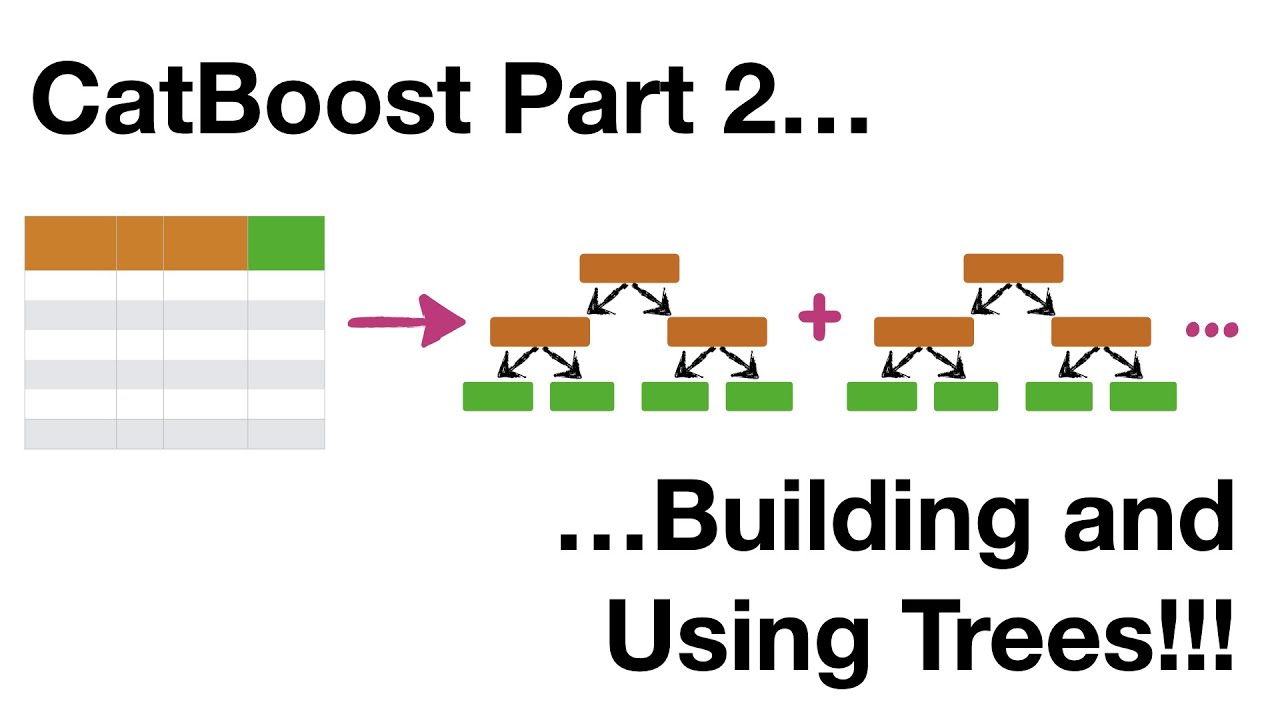
CatBoost Part 2: Building and Using Trees
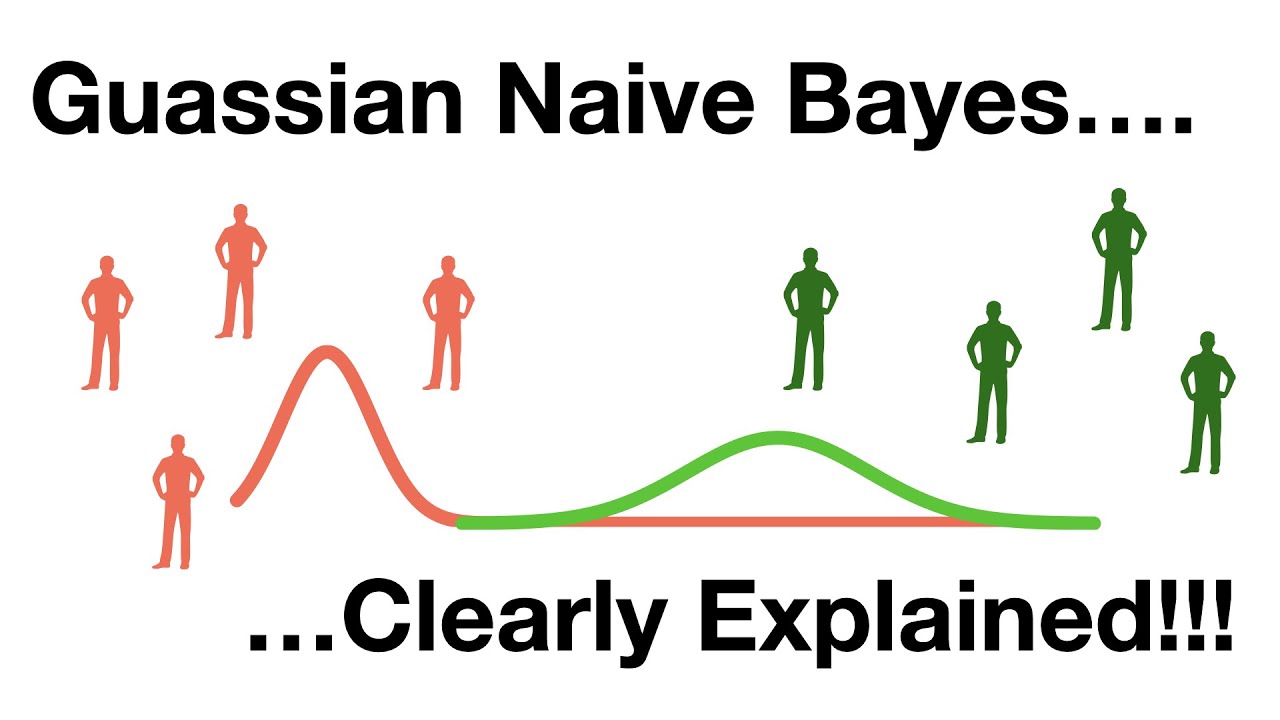
Gaussian Naive Bayes, Clearly Explained!!!
5.0 / 5 (0 votes)