Andrew Ng Explores The Rise Of AI Agents And Agentic Reasoning | BUILD 2024 Keynote
Summary
TLDRIn this talk, the speaker explores the rise of generative and agentic AI workflows, highlighting how these technologies enable faster prototyping and innovation. By leveraging multimodal models (combining text, image, and video data), businesses can now extract more value from unstructured data. The speaker emphasizes the growing importance of data engineering and the increasing capability of AI to handle complex tasks across various industries. Through practical examples, the speaker illustrates how AI is reshaping workflows in fields such as healthcare, legal tech, and visual media, making this an exciting time for developers and businesses alike.
Takeaways
- 😀 Agentic AI is transforming workflows by enabling systems that can autonomously perform tasks, reducing the need for manual intervention.
- 😀 Visual AI applications are becoming more accessible, with examples like analyzing airborne moments in videos and identifying specific features like luggage straps.
- 😀 Agentic orchestration layers, like Langchain, are simplifying the process for developers to create sophisticated agentic AI applications.
- 😀 Token generation speed is a critical factor in agentic AI workflows, and efforts are being made to improve both software and hardware to accelerate this process.
- 😀 Large language models (LLMs) are evolving from answering human queries to supporting tool use, broadening their capabilities for agentic workflows.
- 😀 The rising importance of data engineering, especially for unstructured data like text, images, and video, is essential for organizing and extracting value from large datasets.
- 😀 Image and video processing is experiencing a revolution similar to text processing, offering new opportunities for businesses to leverage visual data in applications.
- 😀 Visual AI tools have made it easier to process and index large amounts of visual data, unlocking new possibilities for categorization and search.
- 😀 As the AI stack becomes more agentic, there is a focus on reducing the complexity and cost of building applications, enabling faster development cycles.
- 😀 The rise of unstructured data is driving the need for new AI technologies that can efficiently manage and deploy visual and text data in real-world applications.
- 😀 The growing ease of building AI applications in both visual and non-visual fields creates new opportunities for innovation, especially in industries that rely on complex data sets.
Q & A
What is generative AI and how is it compared to electricity in the video?
-Generative AI is a type of artificial intelligence capable of creating new content, such as text, images, or videos, based on patterns learned from data. In the video, it is compared to electricity because, like electricity revolutionized industries in the past, generative AI is expected to have a similarly transformative impact across a wide range of sectors.
What is the AI stack, and why is it important?
-The AI stack consists of layers that support AI development, including infrastructure (semiconductors, cloud), foundation models (large language models), and applications. It is important because businesses need to build on top of this stack to create valuable AI applications, and this stack is evolving to make building applications faster and easier.
How has the speed of AI model development improved?
-AI model development has sped up significantly, with prototyping and experimentation now possible within days instead of months. This acceleration is due to advances in generative AI tools, which allow developers to iterate quickly and innovate more efficiently.
What are agentic AI workflows and why are they important?
-Agentic AI workflows refer to systems where AI can perform tasks iteratively, learning from feedback and refining its outputs. They are important because they enable AI to solve more complex problems by continuously improving and adapting, which is crucial for applications in fields like legal processing, healthcare, and visual AI.
What are the four major design patterns for agentic workflows?
-The four major design patterns for agentic workflows are: 1) Reflection (AI reviews and improves its own outputs), 2) Tool Use (AI calls external tools to enhance its capabilities), 3) Planning (AI plans and sequences actions), and 4) Multi-Agent Collaboration (multiple agents collaborate to complete a task efficiently).
What is multimodal AI, and how is it changing the landscape of AI development?
-Multimodal AI refers to AI models that can process and integrate multiple types of data, such as text, images, and videos. This capability is expanding AI’s potential, allowing for more sophisticated applications like generating captions for images or performing tasks that require both visual and linguistic reasoning.
Why is data engineering becoming more important in AI, especially with unstructured data?
-Data engineering is becoming more important because AI is increasingly focused on unstructured data, such as text, images, and video, rather than just structured data (like numbers in tables). Managing, processing, and organizing this unstructured data efficiently is crucial for deriving value from it in AI applications.
What are the four key AI trends that Andrew identifies as worth paying attention to?
-The four key AI trends Andrew identifies are: 1) Improvements in token generation speed, 2) Large language models being optimized for tool use in agentic workflows, 3) The rising importance of data engineering for managing unstructured data, and 4) The ongoing image processing revolution, which is expected to create new opportunities for businesses.
How is image processing in AI expected to evolve, and why is it important?
-Image processing in AI is still in an early phase but is advancing rapidly. As it improves, businesses will be able to extract more value from visual data, which has traditionally been harder to analyze than text. This evolution will open up new possibilities for industries that rely on visual information, such as healthcare, entertainment, and security.
What makes this a great time to be a builder in the field of AI?
-It’s a great time to be a builder in AI because generative AI is allowing rapid experimentation, and agentic workflows are making more complex applications feasible. The ability to develop, test, and iterate quickly is accelerating innovation, opening up many new opportunities to create valuable AI applications that were previously impossible.
Outlines
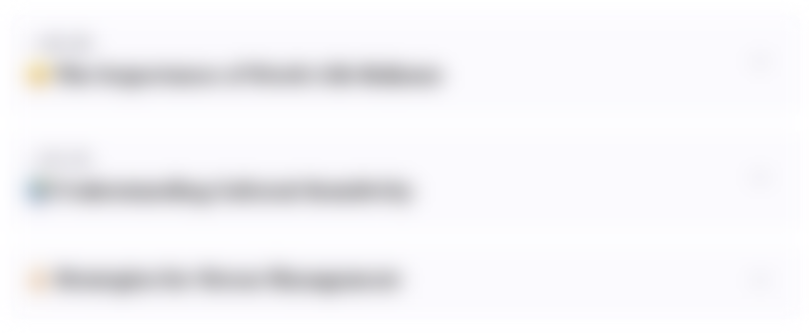
This section is available to paid users only. Please upgrade to access this part.
Upgrade NowMindmap
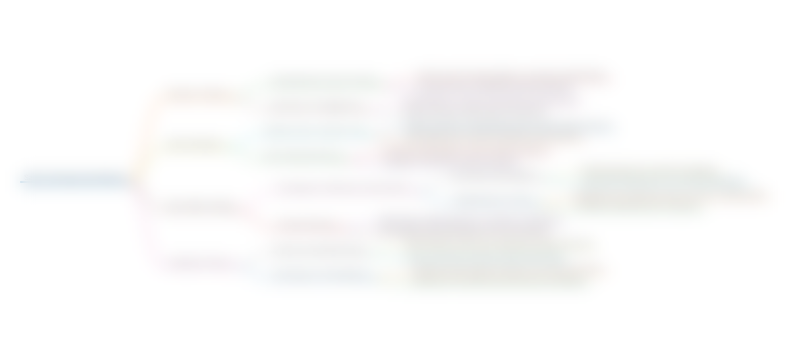
This section is available to paid users only. Please upgrade to access this part.
Upgrade NowKeywords
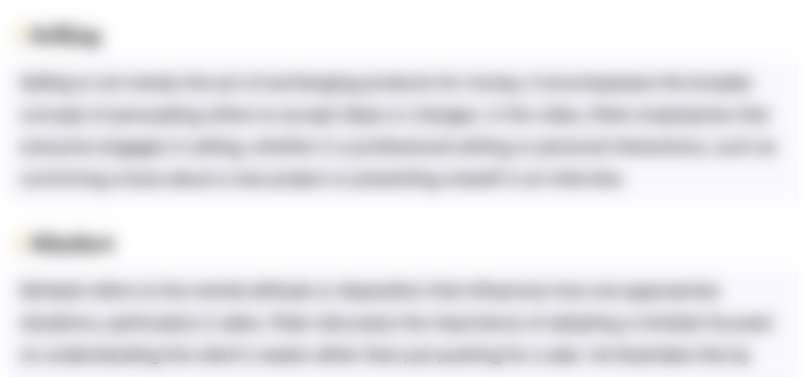
This section is available to paid users only. Please upgrade to access this part.
Upgrade NowHighlights
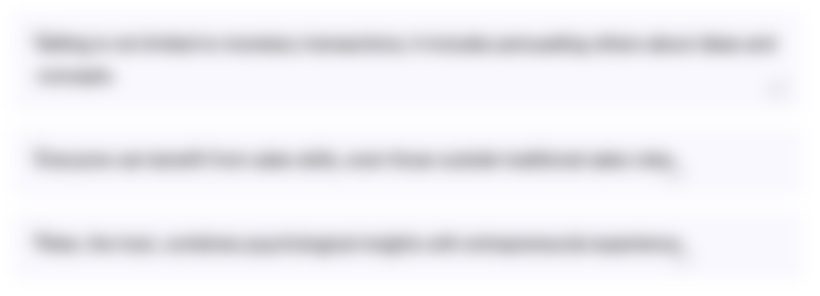
This section is available to paid users only. Please upgrade to access this part.
Upgrade NowTranscripts
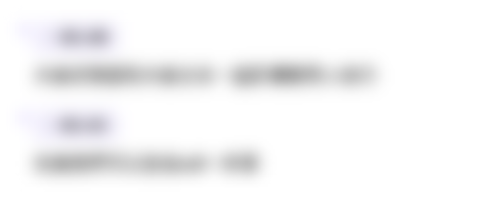
This section is available to paid users only. Please upgrade to access this part.
Upgrade NowBrowse More Related Video
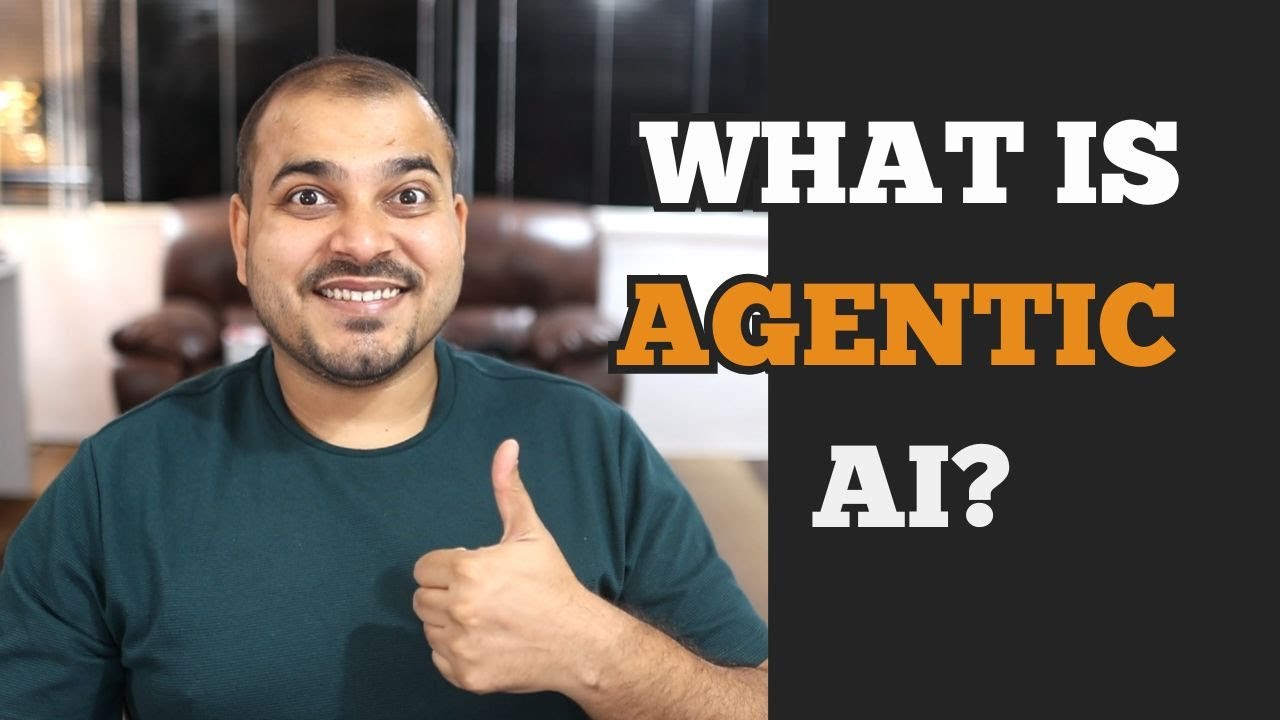
What is Agentic AI? Important For GEN AI In 2025
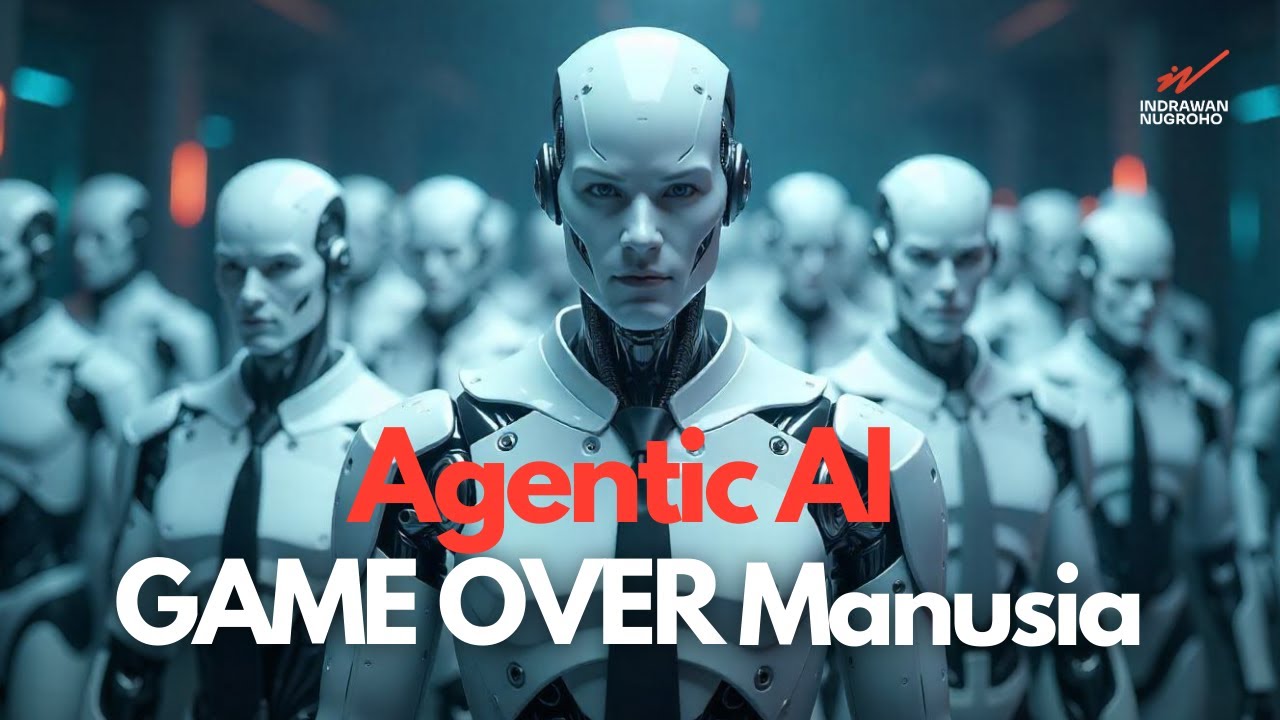
Era Agentic AI Dimulai. Solusi atau Bencana Industri?
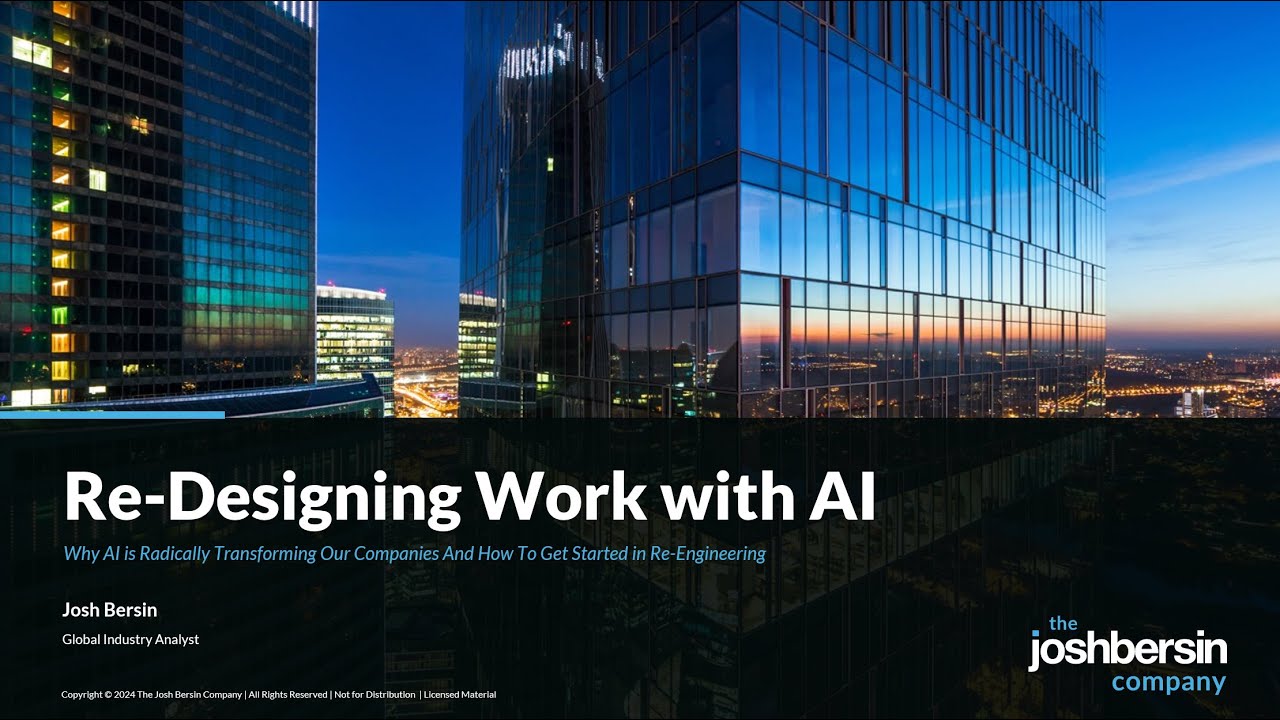
How To Redesign Your Work And Your Business With AI
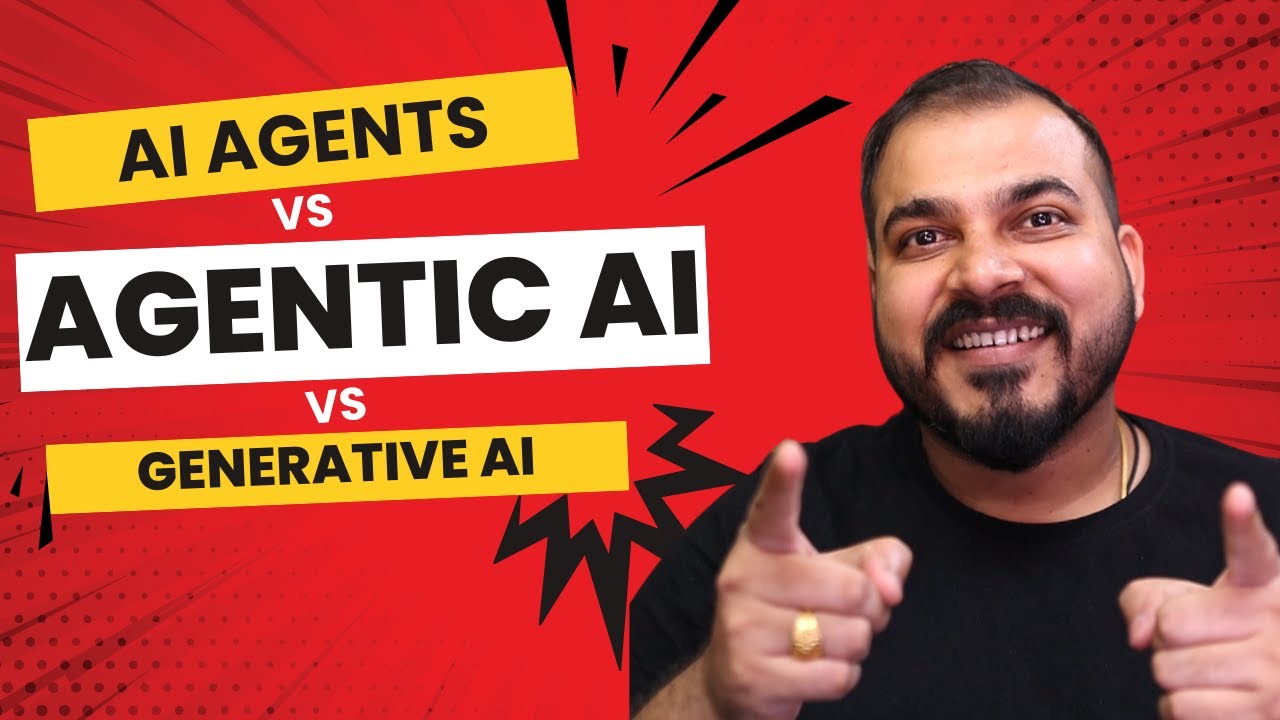
Generative AI Vs Agentic AI Vs AI Agents
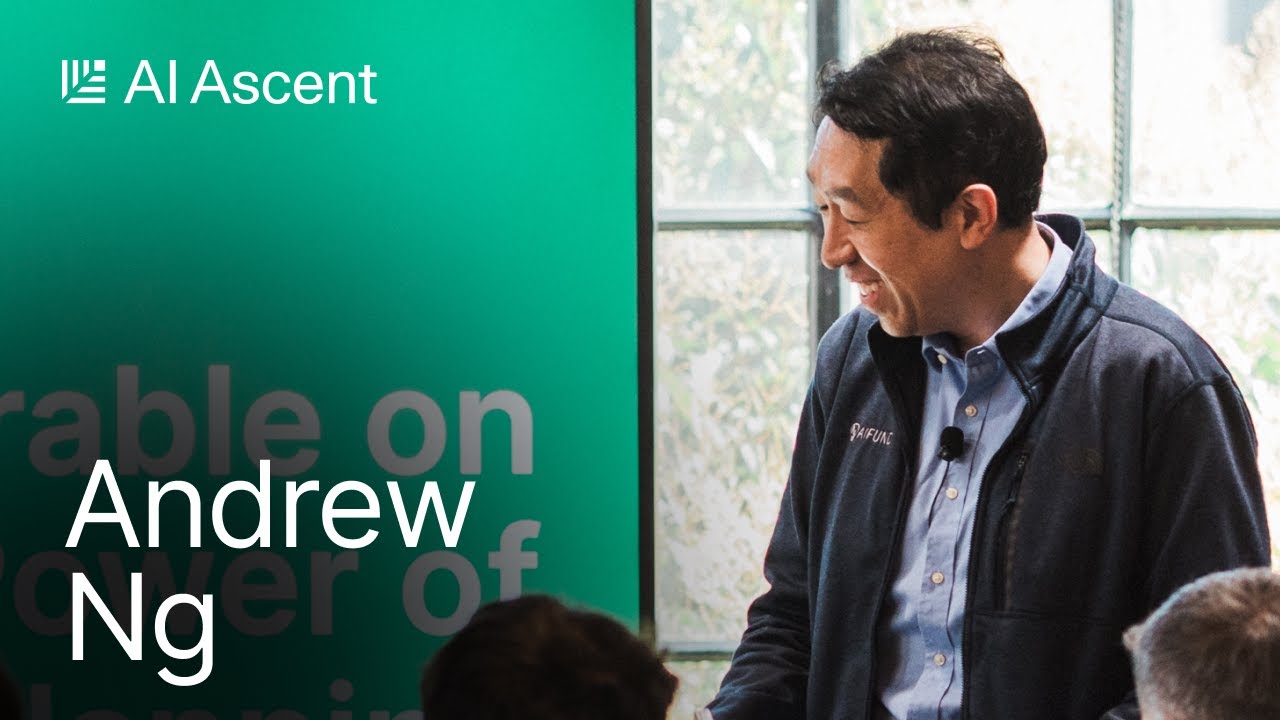
What's next for AI agentic workflows ft. Andrew Ng of AI Fund
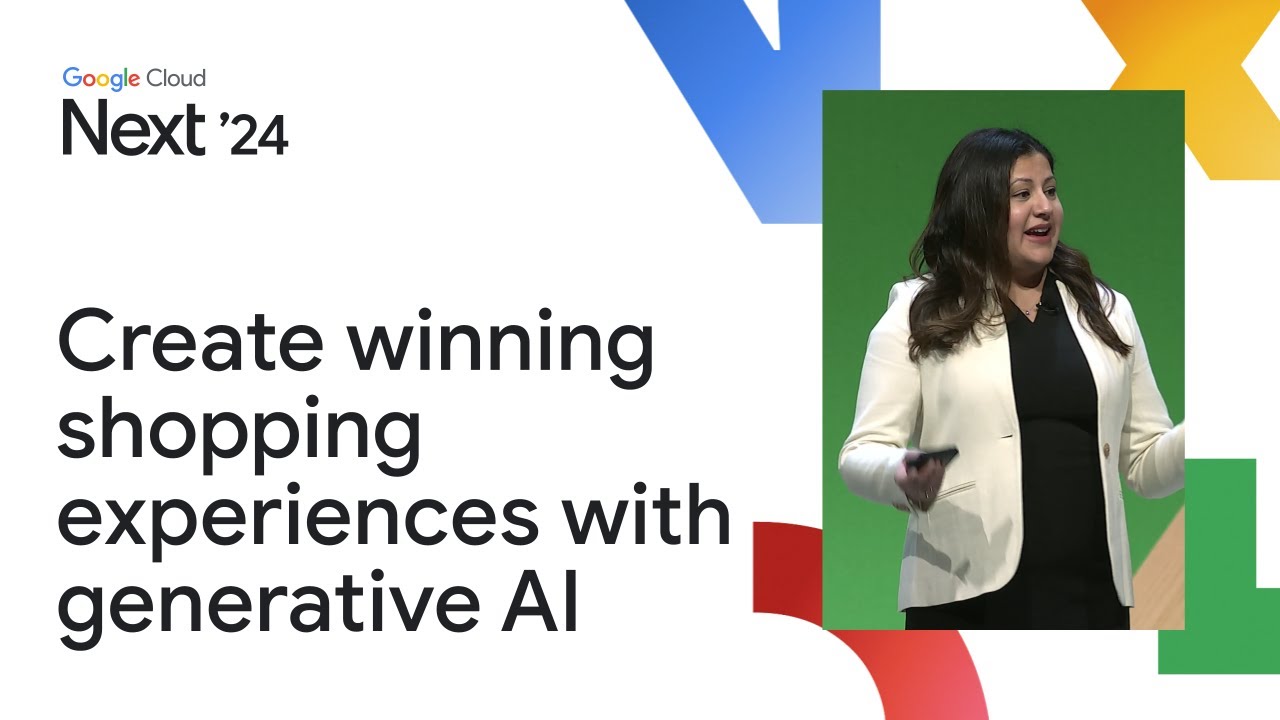
Create winning shopping experiences with generative AI
5.0 / 5 (0 votes)