How will AI transform precision medicine? – Ava Amini
Summary
TLDRThe speaker from Microsoft Research shares a vision for AI-driven precision health, emphasizing the uniqueness of human biology and the need for personalized medicine. They highlight the limitations of current cancer treatments and propose leveraging AI to understand the complexity of biological data, collaborating with the Broad Institute for precision oncology. The presentation introduces 'evoi', a generative AI for designing novel protein therapies, showcasing its ability to create proteins with new functions, aiming to revolutionize treatment by personalizing discovery and experimental design.
Takeaways
- 🤖 The speaker emphasizes the potential of AI in revolutionizing biological discovery and precision health by leveraging the unique genetic makeup of individuals.
- 🧬 Human health is described as both robust and delicate, with a call for personalized medicine that takes into account the nuances of each person's biology.
- 🔍 Current medical practices are criticized for not fully utilizing the rich and complex biological data available, often treating patients based on population averages rather than individual differences.
- 💡 The speaker introduces a new vision for precision medicine using AI, aimed at understanding disease mechanisms and designing new treatments tailored to individual patients.
- 🧬 The script highlights the opportunity to 'unlock the language of biology' by using AI to interpret the vast amount of data stored within each person's biological makeup.
- 🧬 The speaker shares a concrete example of how AI can be applied to cancer treatment, noting the current limitations in targeted therapies and the need for more personalized approaches.
- 📈 The potential of AI is underscored by the ability to analyze nearly 50 million data points from a single tumor biopsy, offering a rich dataset for developing new AI models.
- 🤝 Collaboration with the Broad Institute of MIT and Harvard is mentioned to develop AI systems for precision oncology, integrating molecular measurements into AI training for personalized treatment recommendations.
- 🛠️ The speaker introduces 'evoi', a generative AI model developed by Microsoft Research, designed to create new protein sequences with potential therapeutic applications.
- 🔬 The script discusses the importance of reasoning across different scales and types of data to fully understand and utilize the language of biology for developing new treatments.
- 🔁 The concept of a 'data and learning flywheel' is presented as a method to continuously improve AI models by integrating new data from lab experiments, aiming to close the loop on precision medicine.
Q & A
What is the main vision being discussed in the script?
-The main vision discussed is building AI systems to enable and accelerate new biological discoveries towards a vision of Precision Health, aiming to personalize medicine and better understand the mechanisms of disease.
Why is it important to consider individual differences in medicine?
-Individual differences are important because each person has a unique genetic makeup and history of experiences. Current medicine often does not account for these nuances, leading to less effective treatments that are not personalized.
What is the current state of targeted therapies for cancer patients?
-Fewer than 40% of cancer patients have access to targeted therapies that are specific to changes in their cells. Of those, less than 5% respond effectively to the current treatments, indicating a need for improvement.
How does the speaker define the problem with the current approach to treating cancer?
-The speaker defines the problem as treating cancer in an ad hoc, reductionist way where the individual's richness and complexity are lost, often resulting in treatments that work on average but not personalized.
What is the scale of biological data that can be generated from a single tumor biopsy?
-From a single tumor biopsy, nearly 50 million individual data points can be generated, considering various levels of biological resolution such as DNA mutations, transcriptome, proteins, and cell interactions.
What is the opportunity presented by this scale of biological data for AI researchers?
-The opportunity is to develop new AI systems that can process and analyze this vast amount of data to unlock new biological insights, design new treatments, and improve personalized medicine.
What is the role of AI in the vision of precision oncology discussed in the script?
-AI is central to the vision of precision oncology, where it is used to analyze molecular measurements from patients, train AI systems to produce personalized treatment recommendations, and design new molecular therapies.
What is the generative AI model 'evoi' mentioned in the script, and what does it aim to do?
-Evoi is a new generative AI model developed to design new molecular therapies, such as proteins, by learning from large-scale evolutionary data sets. It aims to produce proteins with new functions that have never been seen before in nature.
How does the AI model 'evoi' utilize the functional part of a protein to design new proteins?
-Evoi uses the functional part of a protein as a prompt, learning from its training data to design a new protein sequence around that part, creating a brand new protein that can be linked to a specific biological function.
What is the ultimate goal of developing these AI systems in the context of precision medicine?
-The ultimate goal is to close the loop on precision medicine by generating a data and learning flywheel that can personalize discovery, experimental design, and ultimately lead to more effective, tailored treatments for patients.
Outlines
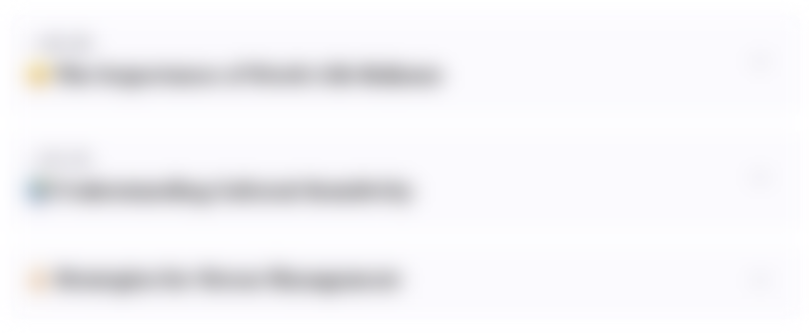
This section is available to paid users only. Please upgrade to access this part.
Upgrade NowMindmap
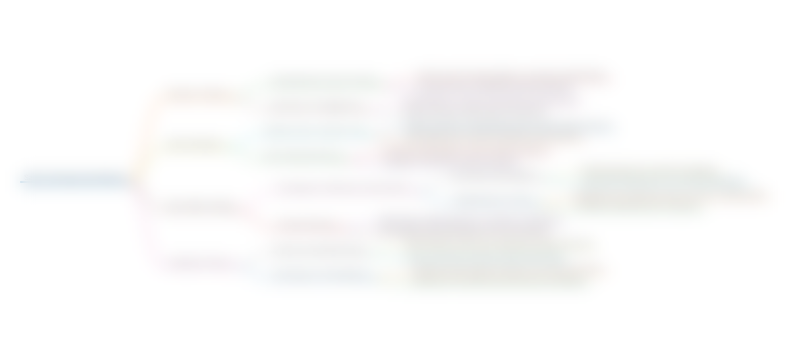
This section is available to paid users only. Please upgrade to access this part.
Upgrade NowKeywords
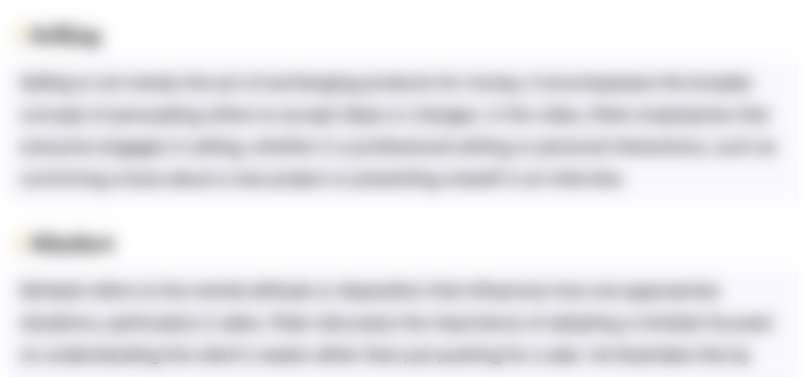
This section is available to paid users only. Please upgrade to access this part.
Upgrade NowHighlights
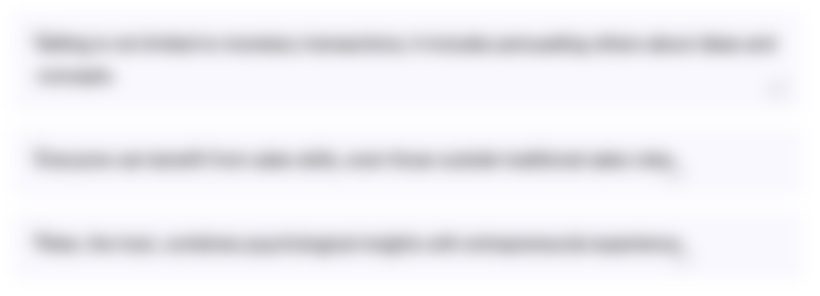
This section is available to paid users only. Please upgrade to access this part.
Upgrade NowTranscripts
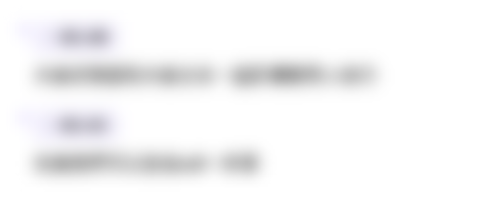
This section is available to paid users only. Please upgrade to access this part.
Upgrade NowBrowse More Related Video

How AI is pushing medical robotics toward autonomy

How omics and bioinformatics are used in the Precision Medicine Lab
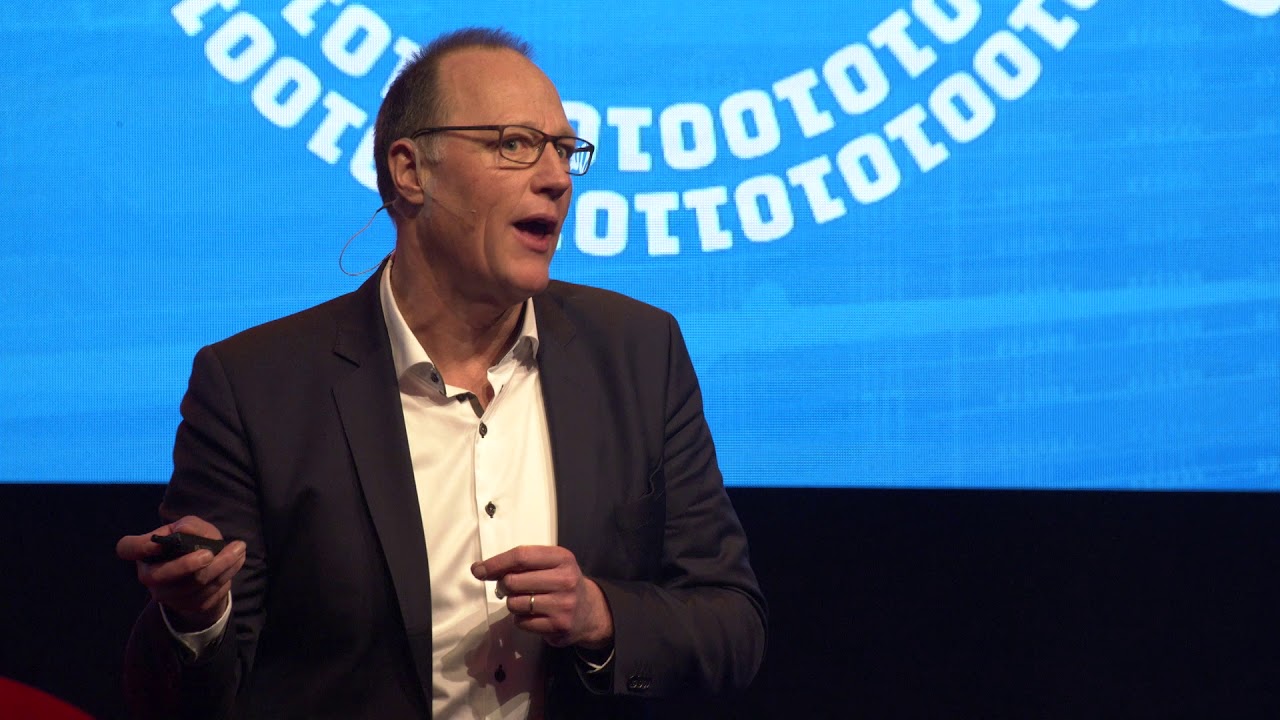
Prediction Machines: How artificial intelligence can help us | Frans Feldberg | TEDxAlkmaar
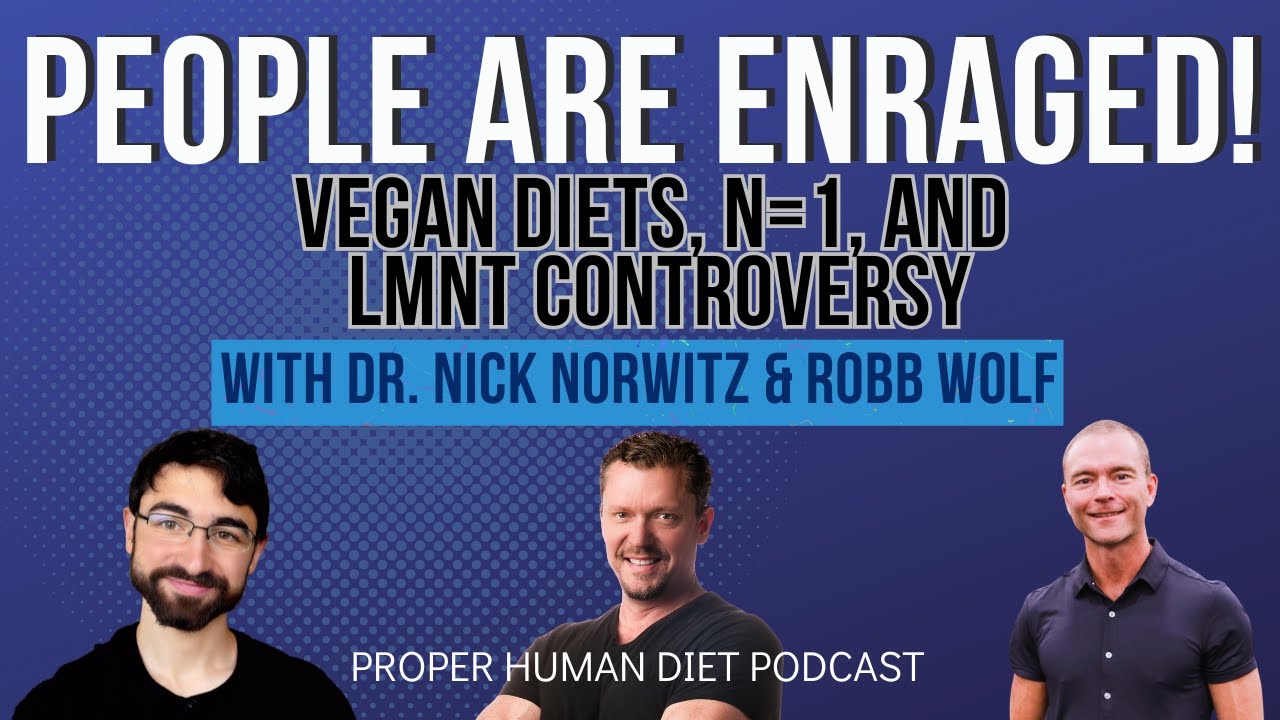
The LMNT Scandal Explained & Nick Norwitz Goes VEGAN with Robb Wolf
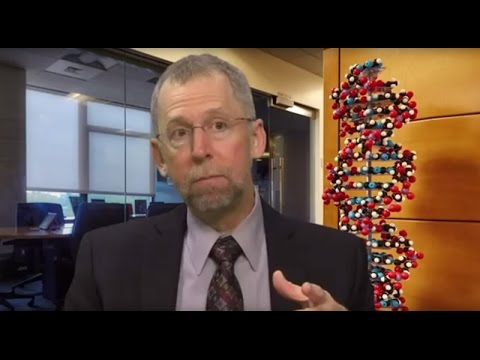
The Impact of the Human Genome Project, 25 Years from its Launch
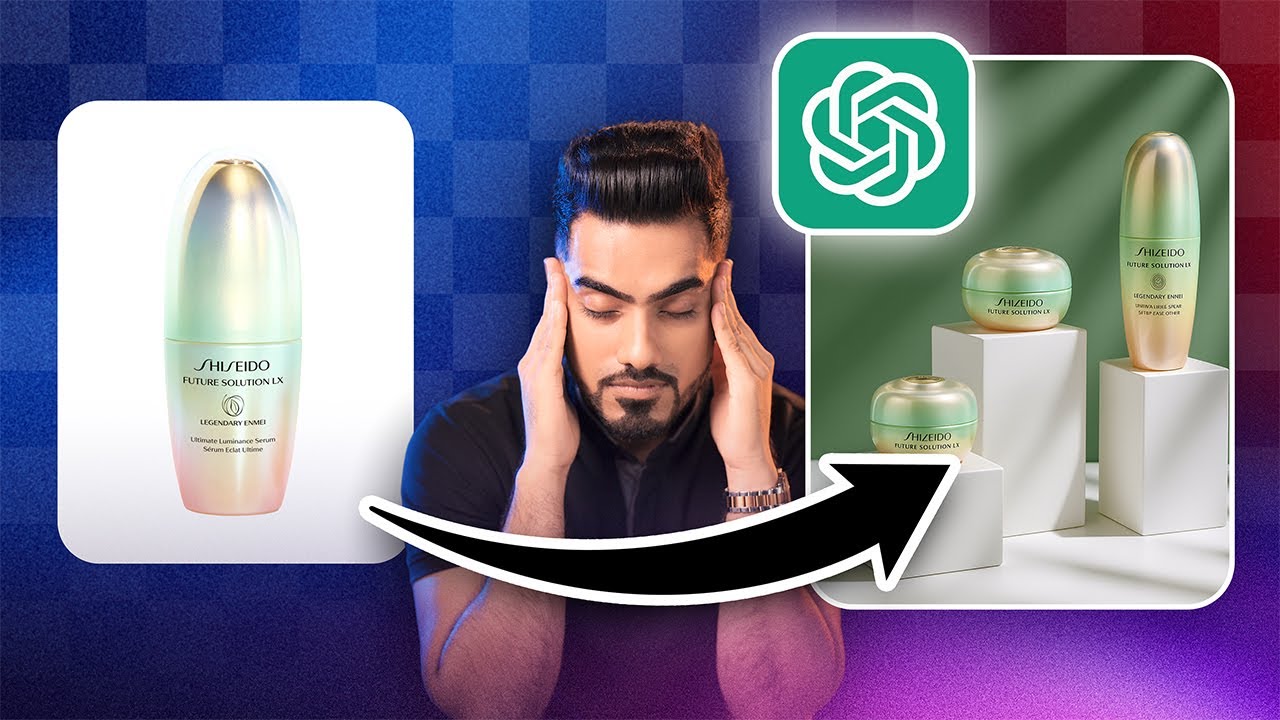
Why Learn Photoshop When ChatGPT Does Everything?
5.0 / 5 (0 votes)