So... What Is The Right Way To Train AGI?
Summary
TLDRThis video delves into the critical importance of data quality in machine learning, emphasizing that successful AI models rely more on well-curated data than sheer volume. It highlights a paper that reveals how complex behaviors can emerge from simple systems, demonstrating that training models on diverse, intricate data leads to improved reasoning abilities. The research shows that models excel when predicting immediate outcomes rather than distant ones, suggesting a focus on complexity without chaos. This approach not only enhances AI development but also resonates with theories of human cognition, offering insights into the emergence of intelligence.
Takeaways
- 😀 Data quality is crucial for AI success; it's more important than the quantity of data.
- 😀 Recent research indicates that smaller, curated datasets can outperform larger ones.
- 😀 Models require time to capture patterns effectively; simply adding data can overwhelm them.
- 😀 Instruction tuning helps AI learn more effectively by using dialogue formats and synthetic data.
- 😀 Over-reliance on synthetic data can lead to a lack of complexity and richness in AI outputs.
- 😀 AI-generated content often lacks nuance, making it recognizable compared to human writing.
- 😀 The emergence of intelligence may occur in systems operating at the edge of chaos.
- 😀 Complex and chaotic data patterns contribute to better performance in logical reasoning tasks.
- 😀 Attention mechanisms in models allow for the integration of historical data, enhancing predictions.
- 😀 Findings may inform both AI development and our understanding of human cognition and intelligence.
Q & A
What is the primary factor determining the success of predictions in statistics and machine learning?
-The quality of the data used is the primary factor determining the success of predictions.
What recent trend has been observed regarding data curation for AI models?
-Researchers have become more selective in how they curate data, focusing on the effectiveness of smaller data sets over larger ones.
What does the term 'model collapse' refer to in the context of AI training?
-Model collapse refers to a situation where a model's performance stagnates due to being overwhelmed by massive, poorly curated data sets.
How does the concept of 'heavy tail distribution' apply to human-written data?
-Human-written data contains a heavy tail distribution of unusual and unique words that provide richness and complexity, which synthetic data often lacks.
What is the significance of instruction tuning in training AI models?
-Instruction tuning helps train large language models to perform better in dialogue formats, enhancing their capabilities as AI chatbots.
How did the paper 'Intelligence at the Edge of Chaos' contribute to understanding AI behavior?
-The paper suggests that intelligence can emerge from simple systems exhibiting complex behaviors, which helps in understanding how AI can produce logical behaviors.
What are the four classes of behaviors identified in the study of Elementary Cellular Automata?
-The four classes are: class one (homogeneous state), class two (periodic structures), class three (chaotic patterns), and class four (complex structures).
What relationship was found between the complexity of training rules and AI model performance?
-The study found that models trained on rules generating complex patterns performed significantly better on harder downstream tasks.
Why did models trained to predict the next move in chess outperform those predicting multiple steps ahead?
-Models trained to predict the next move learned more efficient, non-trivial solutions, likely due to focusing on immediate predictions rather than distant ones.
What implication does the research suggest regarding the generation of synthetic data for training AI?
-The research suggests that generating synthetic data with sufficient complexity can enhance the learning capabilities of AI, leading to more transferable reasoning.
Outlines
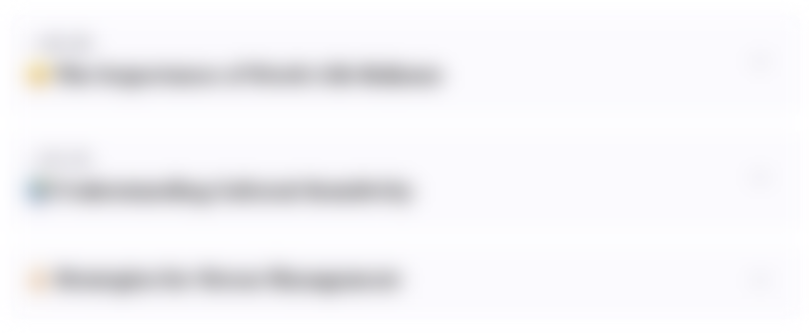
This section is available to paid users only. Please upgrade to access this part.
Upgrade NowMindmap
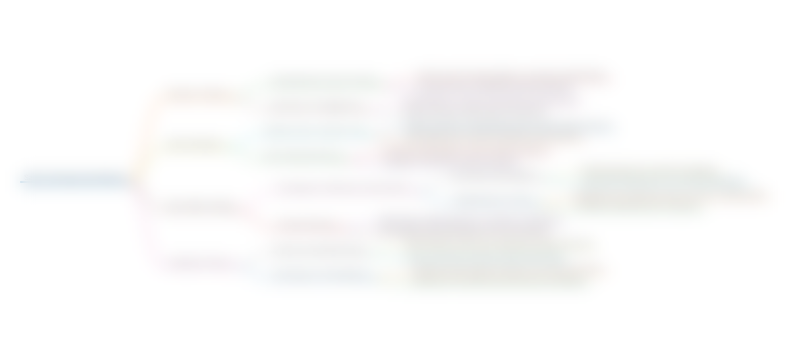
This section is available to paid users only. Please upgrade to access this part.
Upgrade NowKeywords
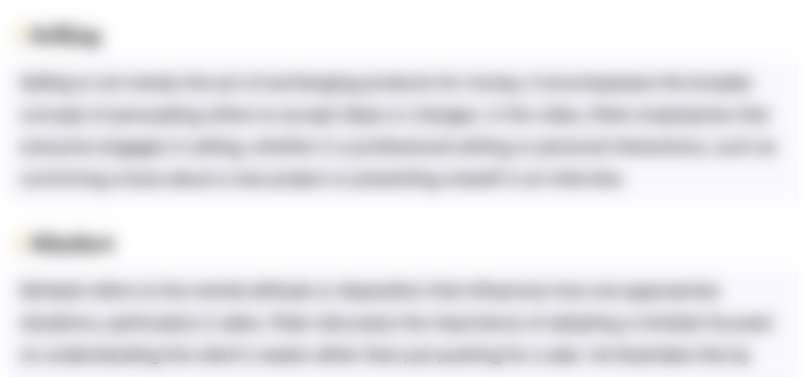
This section is available to paid users only. Please upgrade to access this part.
Upgrade NowHighlights
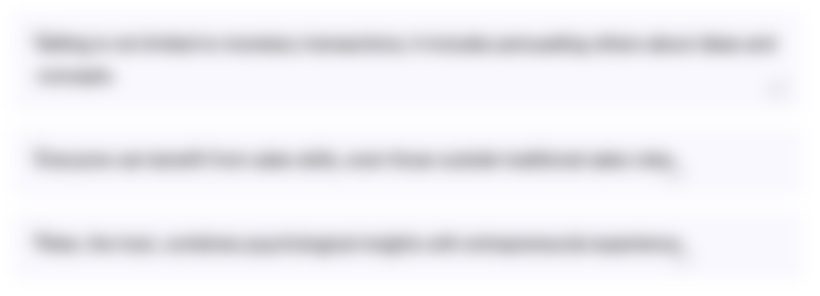
This section is available to paid users only. Please upgrade to access this part.
Upgrade NowTranscripts
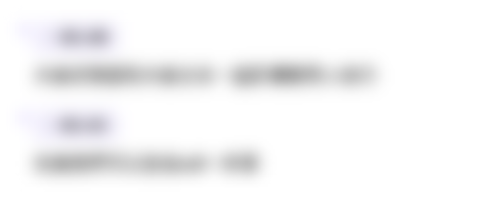
This section is available to paid users only. Please upgrade to access this part.
Upgrade NowBrowse More Related Video
5.0 / 5 (0 votes)