GLM Part 2: Numeric General Linear Models: An Alternative to Regression
Summary
TLDRIn this engaging lecture, the speaker explores the use of Generalized Linear Models (GLMs) with numeric predictors, emphasizing their application in predicting outcomes like car prices based on mileage. Through a series of analytical steps—including plotting distributions, creating scatterplots, and evaluating diagnostic metrics—audience members learn to assess relationships between variables effectively. The speaker shares a personal story about leveraging statistical models to negotiate a better car deal, illustrating the practical benefits of statistical analysis in everyday decision-making. The session concludes with key learning objectives, reinforcing the importance of understanding and interpreting statistical data.
Takeaways
- 😀 GLMs are useful for predicting outcomes based on numeric predictors, such as predicting car value from miles driven.
- 😀 The analysis involves plotting the univariate distribution of both the predictor and outcome to understand their characteristics.
- 😀 A scatterplot is essential for visualizing the relationship between numeric predictors and outcomes, revealing patterns like the inverse relationship between mileage and car cost.
- 😀 The mathematical definition of a GLM is expressed as: cost = intercept + slope × miles + e.
- 😀 Checking assumptions like normality, linearity, and homoscedasticity is crucial for validating the model's accuracy and reliability.
- 😀 Key estimates to interpret in GLMs include the slope (indicating value depreciation per mile driven) and the intercept (cost of a new car).
- 😀 A strong correlation coefficient (e.g., -0.814) indicates a significant relationship between the predictor and outcome variables.
- 😀 The concept of residuals is important; the model aims for normally distributed residuals rather than normality of the original data.
- 😀 Statistical analysis can lead to better financial decisions, as demonstrated by the speaker's experience in negotiating car prices using statistical models.
- 😀 The primary learning objectives include understanding GLMs, visualizing relationships, assessing assumptions, and interpreting key statistical estimates.
Q & A
What is the primary purpose of using Generalized Linear Models (GLMs) with numeric predictors?
-GLMs are used to predict outcomes based on numeric predictors, allowing for analysis of relationships between variables, such as predicting car prices from mileage.
Why is it important to visualize the univariate distribution of the outcome variable?
-Visualizing the univariate distribution helps to understand the spread and range of the outcome variable, which is essential for identifying potential issues in modeling.
What does a scatterplot reveal about the relationship between mileage and car cost?
-A scatterplot shows that as mileage increases, car cost generally decreases, indicating a negative relationship between the two variables.
What is the significance of the slope in a GLM?
-The slope indicates how much the outcome variable (e.g., car cost) is expected to change for each unit increase in the predictor (e.g., mileage), quantifying the strength of the relationship.
What do we assess in the diagnostics of a GLM?
-In the diagnostics, we assess normality, linearity, and homoscedasticity of residuals to ensure that the assumptions of the model are met and to validate the model's fit.
How is the intercept interpreted in a GLM?
-The intercept represents the expected value of the outcome variable when the predictor is zero, which, in the context of car prices, would indicate the cost of a brand-new car.
What does a correlation coefficient of -0.814 indicate?
-A correlation coefficient of -0.814 indicates a strong negative relationship between mileage and car cost, suggesting that as mileage increases, car cost tends to decrease significantly.
How can statistics help in everyday decision-making, such as buying a car?
-Statistics can provide insights and predictions based on data, enabling informed decisions, such as negotiating a better price for a car based on its predicted value.
What steps should be taken after building a GLM to evaluate its effectiveness?
-After building a GLM, one should visualize the relationship, check diagnostic plots for assumptions, interpret the estimates, and compare the model's predictions with actual outcomes.
What are some key estimates to focus on when analyzing a GLM?
-Key estimates include the slope, intercept, correlation coefficient, standardized slopes, and R-squared, all of which provide insights into the strength and nature of the relationships in the model.
Outlines
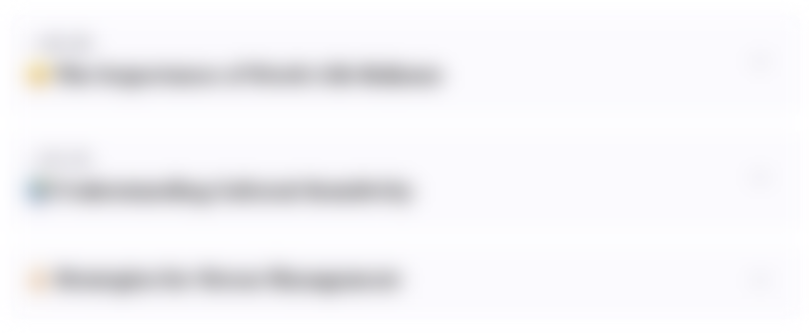
This section is available to paid users only. Please upgrade to access this part.
Upgrade NowMindmap
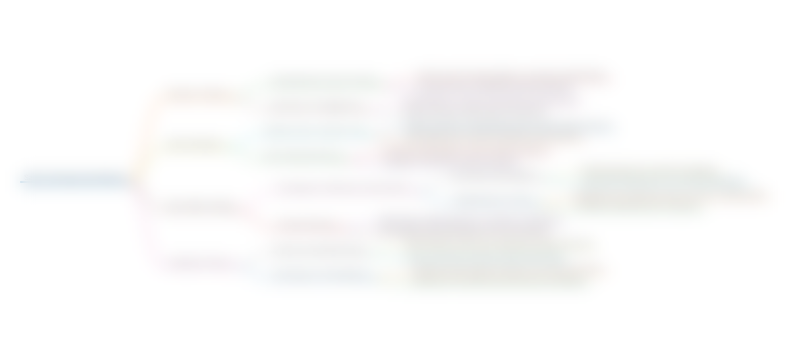
This section is available to paid users only. Please upgrade to access this part.
Upgrade NowKeywords
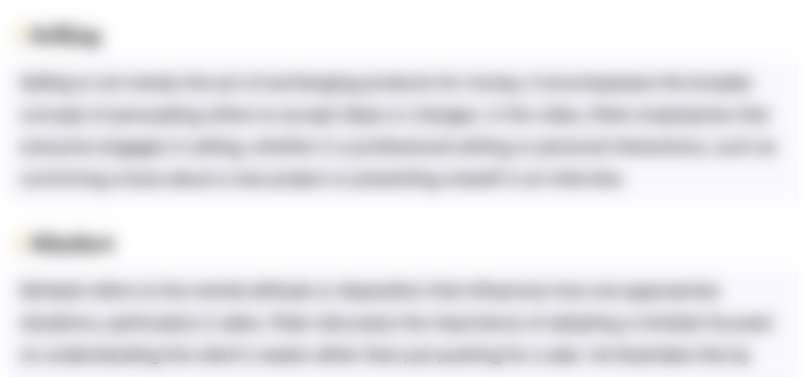
This section is available to paid users only. Please upgrade to access this part.
Upgrade NowHighlights
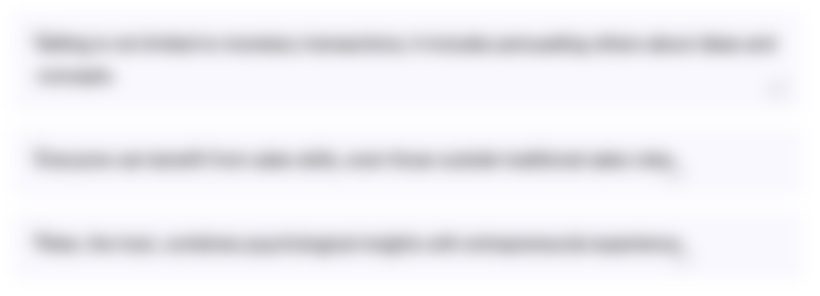
This section is available to paid users only. Please upgrade to access this part.
Upgrade NowTranscripts
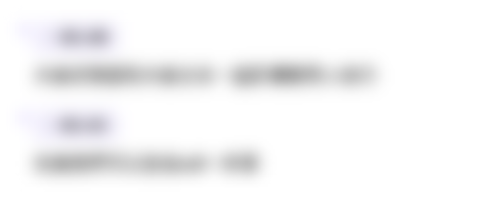
This section is available to paid users only. Please upgrade to access this part.
Upgrade NowBrowse More Related Video
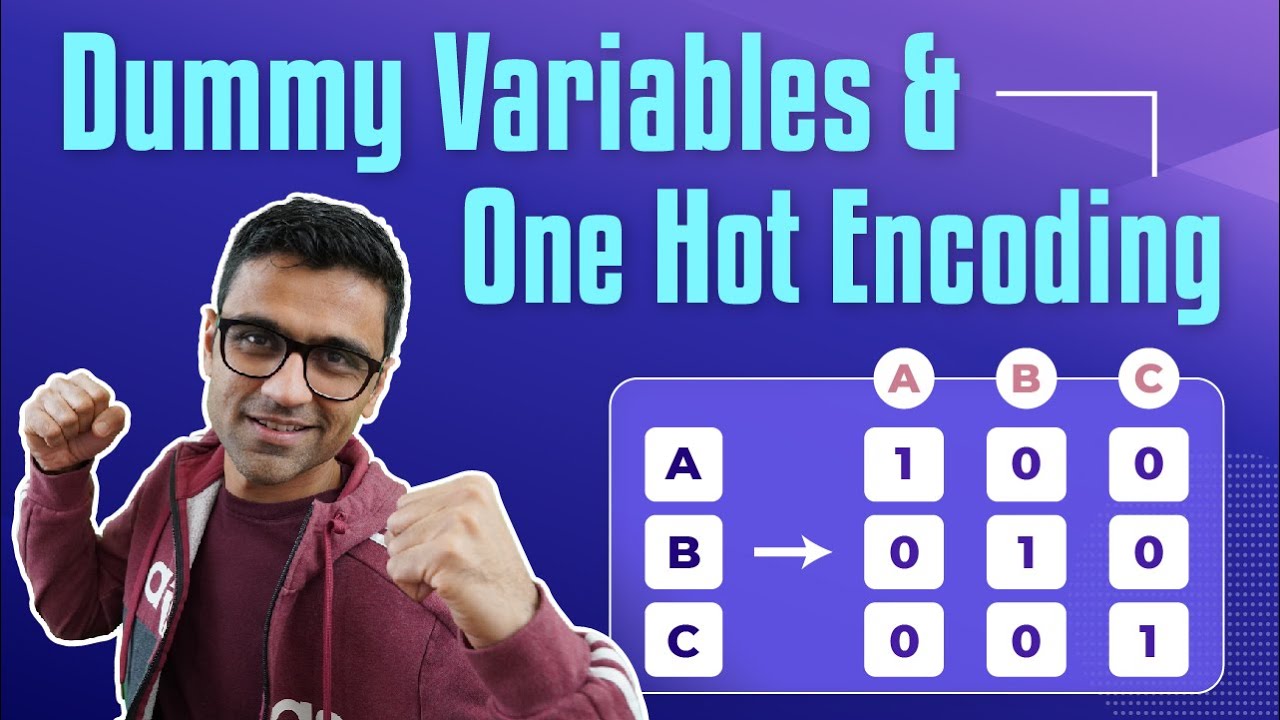
Machine Learning Tutorial Python - 6: Dummy Variables & One Hot Encoding
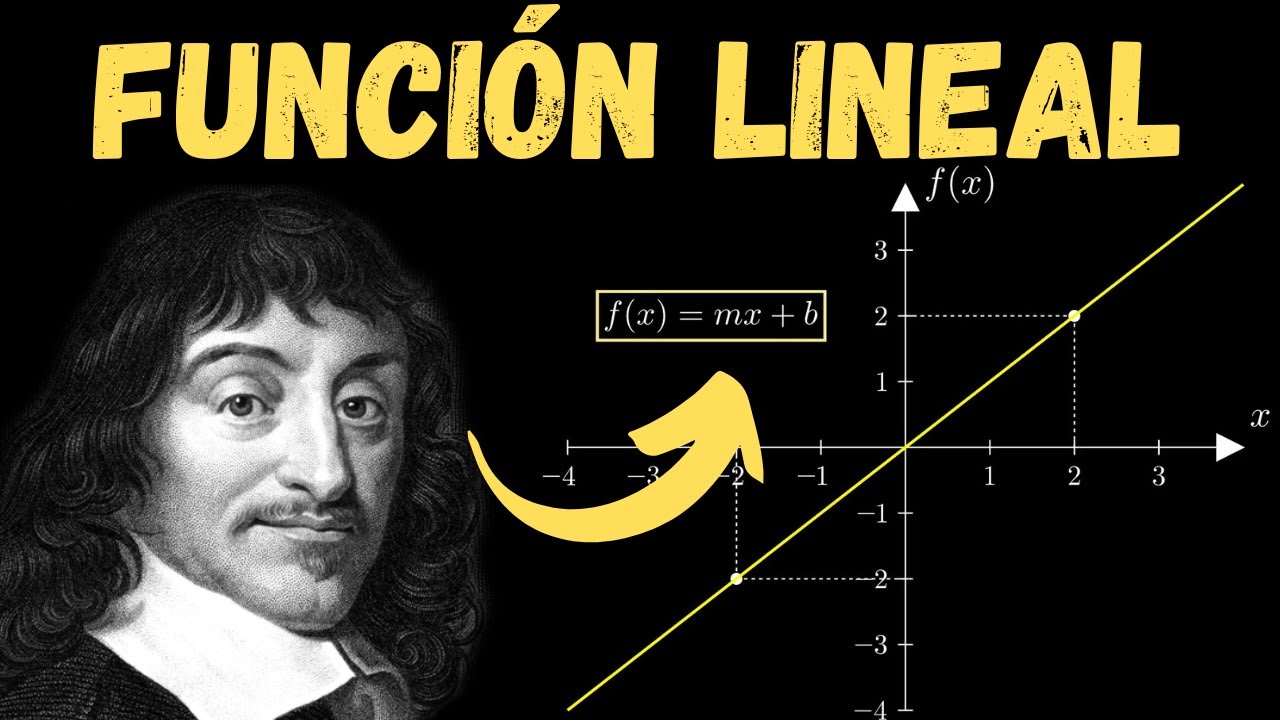
¿Qué es una FUNCIÓN LINEAL? ▶ GRÁFICA, PENDIENTE e INTERCEPTO en 20 MINUTOS ⌚🚀

Core Learning Algorithms A - TensorFlow 2.0 Course

Applications of Regression

Lecture 04 : Project Selection Models
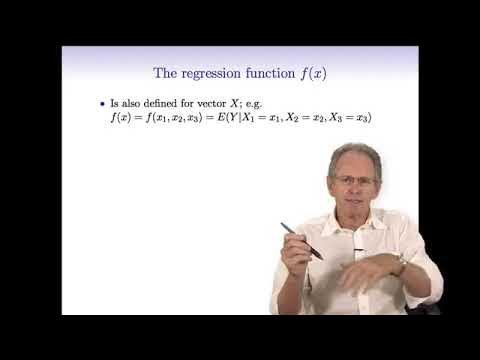
Statistical Learning: 2.1 Introduction to Regression Models
5.0 / 5 (0 votes)