No Priors Ep.61 | OpenAI's Sora Leaders Aditya Ramesh, Tim Brooks and Bill Peebles
Summary
TLDR「No Priors」というポッドキャストのエピソードで、OpenAI SoraチームのAdia、Tim、Billが登場し、新しい生成型ビデオモデルSoraについて語っています。Soraはテキストプロンプトを受け取り、高解像度で視覚的に整合性のある1分間のクリップを生成できます。彼らは、このような大規模なビデオモデルがワールドシミュレータになる可能性と、スケーラブルなTransformersアーキテクチャをビデオ分野に適用することで、人工知能(AGI)への道を模索しています。また、Soraがどのようにして創造的なコンテンツの制作を容易にし、エンターテイメントや教育、コミュニケーションの新しいパラダイムをもたらす可能性があるかについて語ります。さらに、ビデオモデルの安全性に関する議論も行われ、偽造や誤情報のリスクを最小限に抑えるための取り組みが紹介されています。
Takeaways
- 🚀 Soraは、テキストプロンプトから高解像度で視覚的に一貫性のある、最大1分間のビデオクリップを生成できる新世代のビデオモデルです。
- 🤖 Soraの開発チームは、人工知能(AGI)への道に重要な役割を果たすと信じており、複雑な環境や世界を神経ネットワークの重みの中でモデル化できると見ています。
- 🌐 Soraは、将来的には人や動物、オブジェクトなどを含むワールドシミュレータとして機能し、人間がそれらと相互作用できる可能性があります。
- 🎨 Soraはアーティストやクリエイターにアクセスを提供し、フィードバックを得ることで、ツールとして最も有用になる方法や安全に導入する方法を学んでいます。
- 📈 Soraの能力は、コンピュートパワーやデータの追加によって向上し、より良いシミュレーションや長期的なビデオ生成が可能になる見込みです。
- 🧩 SoraはTransformerアーキテクチャを用いてスケーラブルで、ビデオデータの複雑な関係を学習することができます。
- 📚 Soraは、SpaceTime patchesという概念を導入し、ビデオを3D立方体として扱うことで、言語モデルのように様々なタイプの視覚データを扱えるようになりました。
- 🔍 Soraは、物理世界の理解を深めるために、ビデオから3D情報を自ら学習し、オブジェクトの永続性や詳細な物体相互作用を改善する予定です。
- 🌟 Soraのリリースは、ビデオモデルにおけるGPT-1のような瞬間であり、今後のバージョンでは創造性やAGIへの貢献がさらに期待されます。
- 🤔 Soraの開発チームは、偽造動画や誤情報のリスクなどの安全性問題に注意を払いながら、テクノロジーの利点を最大限に活かす方法を模索しています。
- ✨ Soraの進化は、人間の世界モデルよりも高精度な予測が可能になる一方で、人間の知能とは異なる知能の形を探求する可能性を示しています。
Q & A
Soraという新感覚の生成ビデオモデルはどのような特徴を持っていますか?
-Soraは、テキストプロンプトを受け取り、高解像度で視覚的に整合性のある、最大1分間のクリップを生成する新しい斉次変換子アーキテクチャを応用した生成ビデオモデルです。それは非常に複雑な環境や世界をニューラルネットワークの重みだけでモデル化できる可能性を示しています。
SoraがAGI(人工的一般知能)への道に沿っていると信じている理由は何ですか?
-Soraは複雑な環境をモデル化する能力を持ち、人々が相互作用し、考え方、さらには動物や様々な物体をモデル化する方法を学ぶ必要があるため、AGIへの道に沿っているとされています。
Soraを一般に利用可能にするためのロードマップやタイムラインはありますか?
-現時点では、製品の即時計画やタイムラインはありませんが、アーティストやレッドチームにアクセスを提供し、フィードバックを得ることで、Soraが世界に与える影響や人々にどのように役立つかについて考えています。
Soraの導入によって、安全に関する懸念は何ですか?
-Soraの導入によって、偽造動画やスプーンのリスク、誤情報の拡散など、新たな安全問題が懸念されます。また、企業やソーシャルメディア企業、ユーザーの責任分担についても検討する必要があります。
Soraの将来的な進化について、どのような期待を持っていますか?
-Soraはより複雑で長期的な物理的相互作用をより正確に捉えることができるようになると期待されています。また、Soraは3D情報を学び、人間の世界をより深く理解するため、より知能的で総合的なAIモデルに貢献すると見ています。
Soraが持つ創造性とビジュアルの魅力について、どのように考えられていますか?
-Soraは言語理解を通じてユーザーが生成内容を方向付けることができる能力を持っていますが、特にアディアの美学が深く埋め込まれているわけではありません。将来的には、個人のセンスに合わせてカスタマイズすることができると期待されています。
Soraのトークン化について説明してください。
-Soraでは、SpaceTimeパッチという概念を使ってデータを表します。これは、画像やビデオのデータがどのように存在するかにかかわらず、それを表すことができます。これにより、Soraは720pビデオだけでなく、縦ビデオやワイドスクリーンビデオ、1:1から2:1までのアスペクト比の映像を生成できます。
Soraのアーキテクチャの進化について教えてください。
-Soraのアーキテクチャは、画像ジェネレーターからビデオジェネレーターへと拡張するのではなく、1分間のHDビデオを生成するための問いを出発点として、スケーラブルで単純な方法でデータを分解することができるモデルを目指して進化しました。
Soraが今後のAI研究の道筋に与える影響について説明してください。
-Soraは、ビデオデータから学び、3Dや物体の永続性などの概念を理解し、人間の世界をより深く知ることができます。これにより、AIモデルがより知能的で総合的になることが期待されており、ビデオジェネレーション以外にも幅広い影響を与える可能性があります。
Soraの将来のアップデートで期待されることとは何ですか?
-Soraの今後のアップデートでは、より複雑で長期的な物理的相互作用の正確性を向上させることに加えて、個人の美学やスタイルをよりよく理解し、カスタマイズすることができるようになると期待されています。
Soraの導入に伴い、社会的な責任や安全性に関する問題についてどのように考えていますか?
-Soraの導入に伴い、誤情報のリスクや偽造メディアの拡散などの問題に対処する必要があります。また、Soraを展開する企業は、技術の責任を負い、社会メディア企業やユーザーにも責任を分担してもらう必要があります。
Outlines
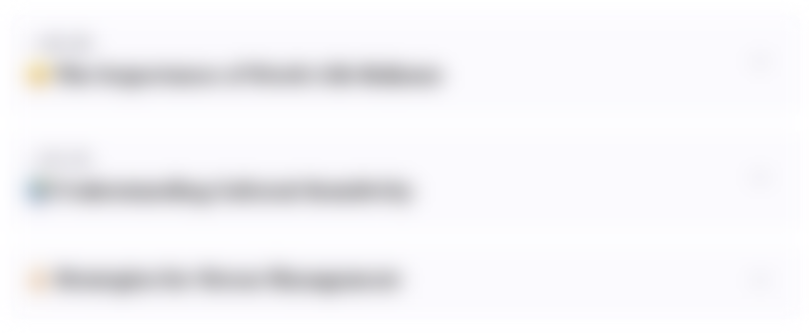
This section is available to paid users only. Please upgrade to access this part.
Upgrade NowMindmap
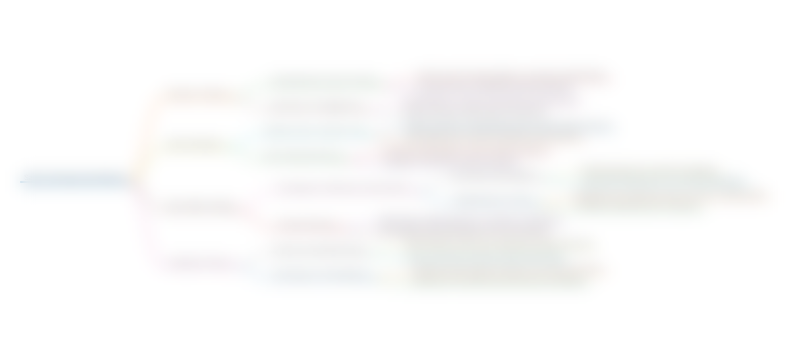
This section is available to paid users only. Please upgrade to access this part.
Upgrade NowKeywords
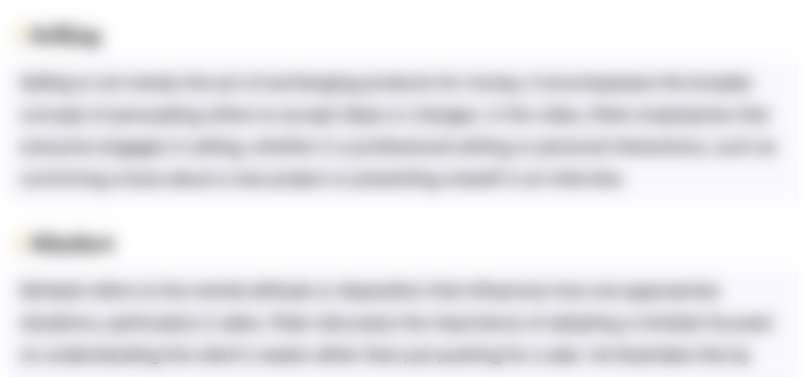
This section is available to paid users only. Please upgrade to access this part.
Upgrade NowHighlights
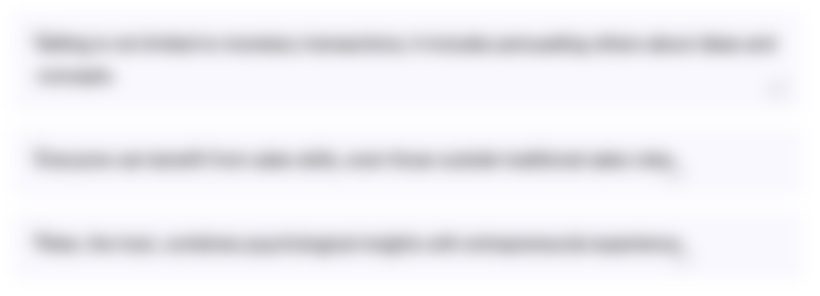
This section is available to paid users only. Please upgrade to access this part.
Upgrade NowTranscripts
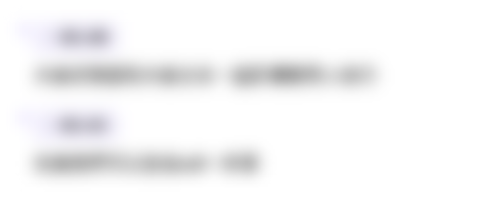
This section is available to paid users only. Please upgrade to access this part.
Upgrade NowBrowse More Related Video
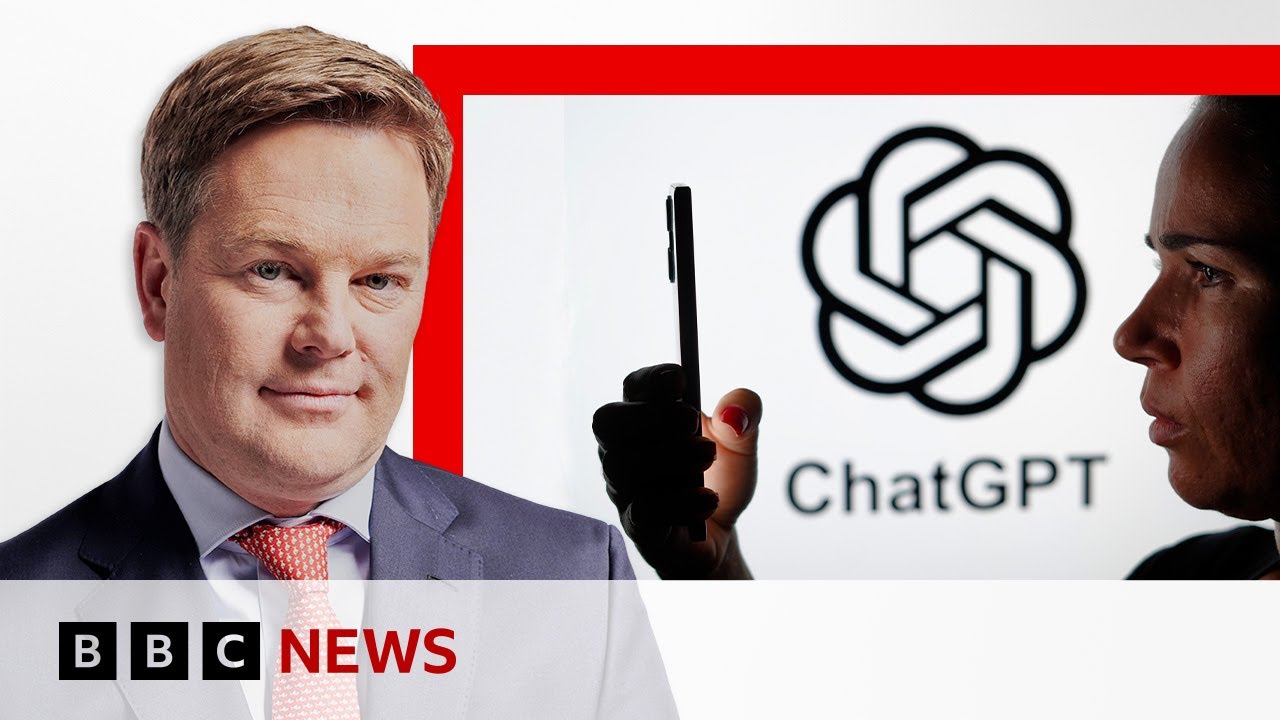
How good is the latest version of ChatGPT? | BBC News
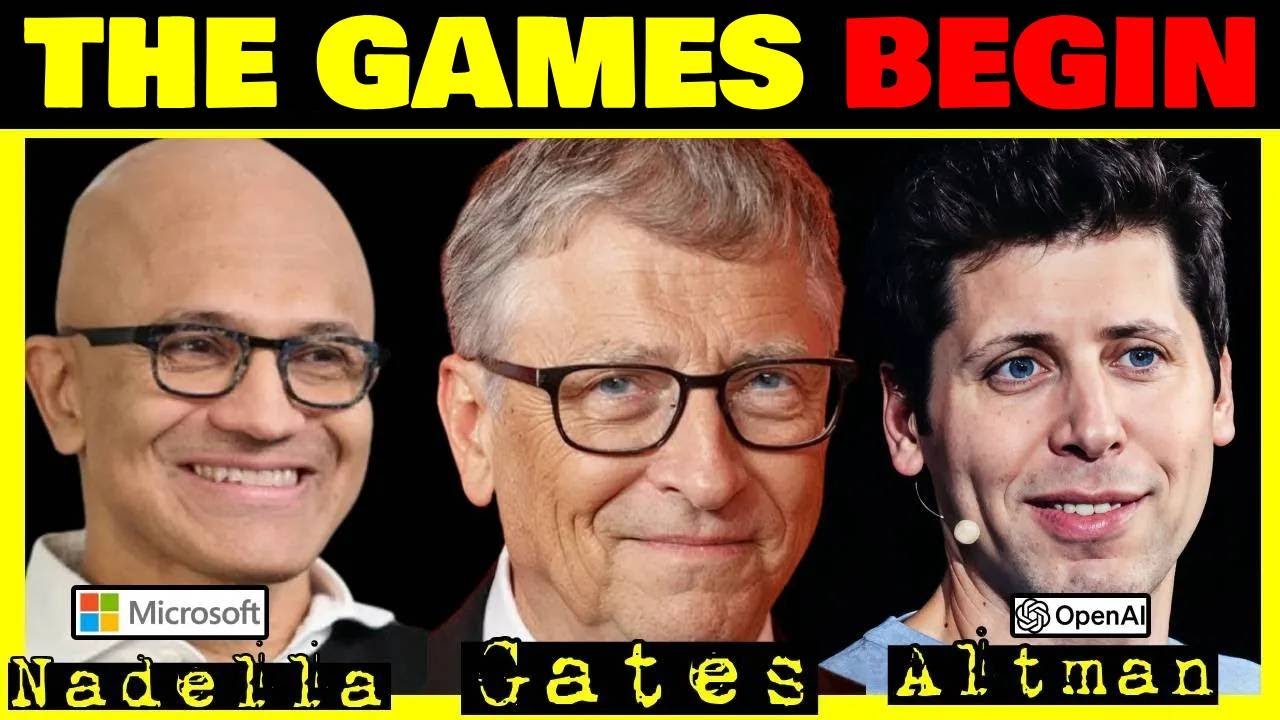
Microsoft's new "Embodied AI" SHOCKS the Entire Industry! | Microsoft's Robots, Gaussian Splat & EMO

The TRUTH About James Jani Sucess
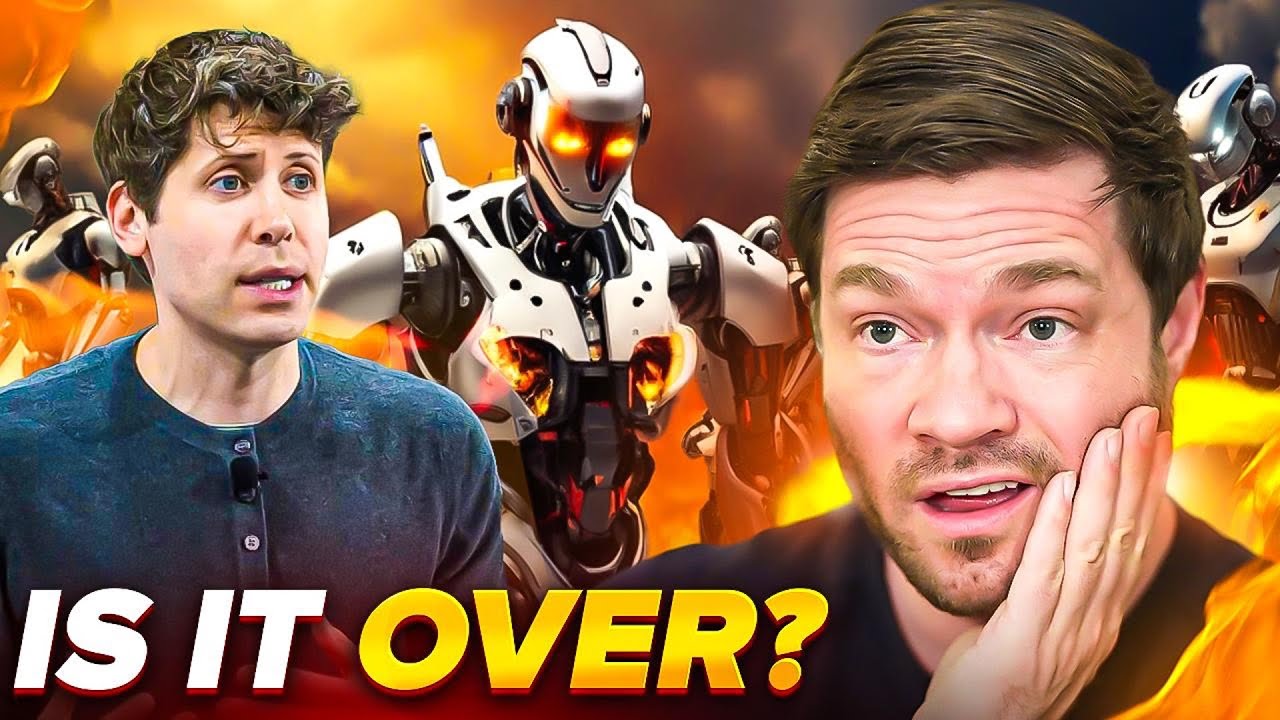
AI News: AGI In 2 Years, Meta’s LEAK, AI Sarcasm Detector & More
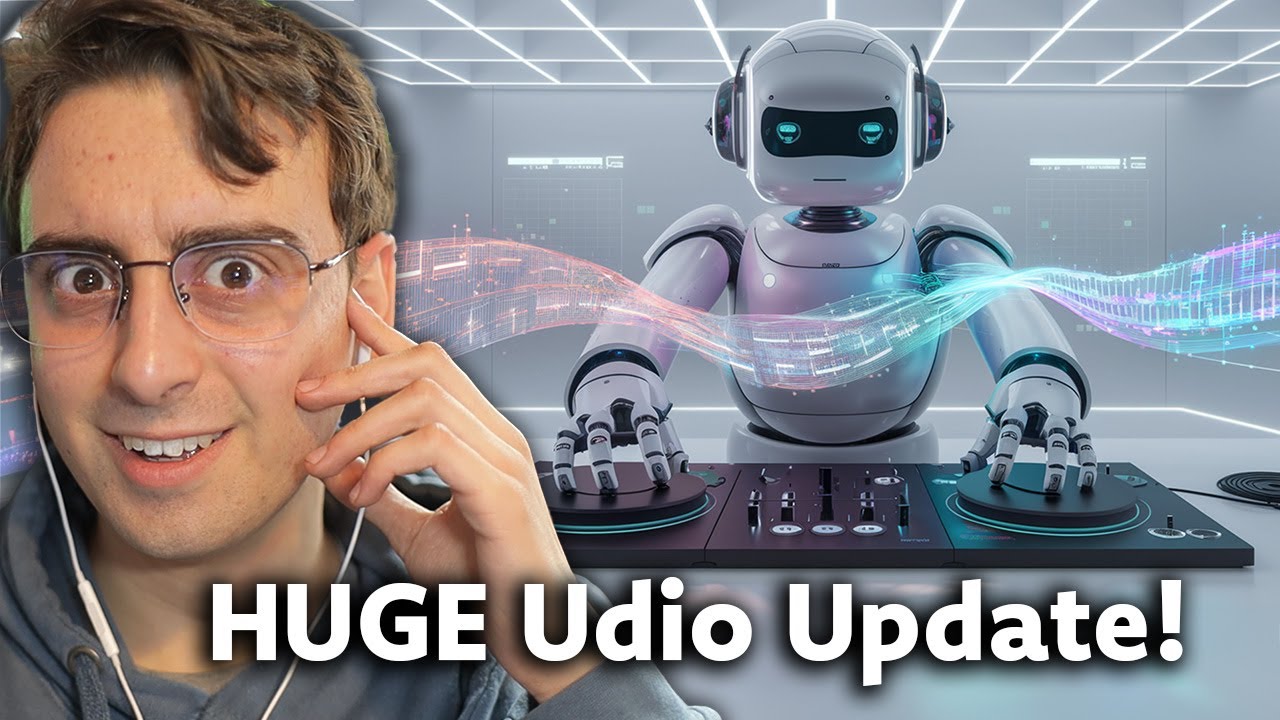
Audio Upload on Udio AI Music is INCREDIBLE!
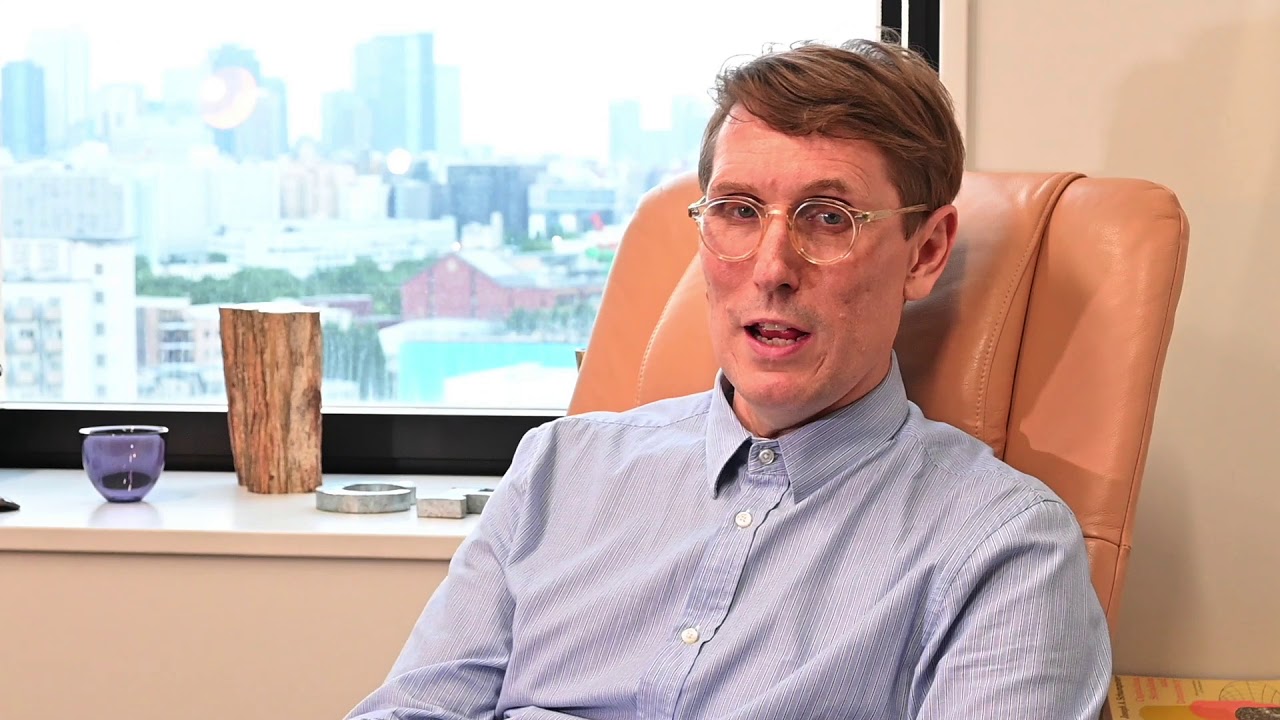
i2B W6 B
5.0 / 5 (0 votes)