Linear mixed effects models
Summary
TLDRThis video lecture delves into linear mixed effects models, an advanced extension of linear regression, ideal for analyzing complex data structures with nested subgroups. It clarifies the distinction between fixed and random effects, providing guidance on selecting and modeling these effects based on research questions. Emphasizing the importance of model diagnostics, the lecture illustrates how to assess residuals and ensure assumptions are met. Additionally, the concept of partial pooling is introduced, demonstrating its utility in enhancing parameter estimates, particularly for small sample sizes. Overall, the lecture underscores the power and complexity of mixed effects models in statistical analysis.
Takeaways
- 😀 Mixed effects models extend typical linear models like regression, accommodating complex sampling designs.
- 🔍 Choosing a mixed effects model involves understanding fixed vs. random effects, which can be subjective based on the data structure.
- 🌊 Fixed effects are parameters of interest, while random effects account for variability within nested subgroups.
- 🏞️ Random effects can be modeled with varying intercepts and slopes, providing flexibility in analysis.
- 📈 Model diagnostics are crucial; assess residuals to ensure no patterns or trends indicate model misfit.
- 📊 Centering and standardizing independent variables enhance model convergence and facilitate comparison of effects.
- ⚖️ Random effects should ideally have at least five levels to provide reliable estimates of their distributions.
- 🚫 Be cautious of multicollinearity among independent variables, as it complicates interpretation and model fitting.
- 🔄 Mixed effects models can improve parameter estimates through partial pooling, especially for small sample sizes.
- 🛠️ Understanding data, study design, and model assumptions is vital for the effective application of mixed effects models.
Q & A
What are linear mixed effects models and why are they used?
-Linear mixed effects models are an extension of typical linear models, such as linear regression, used to analyze data with complicated sampling designs. They account for both fixed and random effects, making them suitable for data that includes nested subgroups.
What distinguishes fixed effects from random effects in mixed models?
-Fixed effects are parameters of interest that are consistent across observations, while random effects account for variations between groups or levels in the data that are not the main focus of the study. The decision to treat a factor as fixed or random depends on the research question and data structure.
How can you determine whether to treat a factor as fixed or random?
-There are no strict rules for classifying factors as fixed or random. However, a common guideline is to consider a factor as random if you are controlling for non-independence created by a nested data structure and are not interested in the factor itself.
What is the role of random effects in mixed models?
-Random effects help model the variability within groups and account for repeated measures. They are typically drawn from a broader population distribution, which allows for better estimation of the overall model.
What is the difference between nested and crossed random effects?
-Nested random effects occur when one level of a random effect exists within another unique level of a higher random effect, while crossed random effects allow all combinations of levels to occur together. This distinction affects how the data is structured and analyzed.
Why is it important to check the assumptions of mixed effects models?
-Checking assumptions ensures that the model adequately describes the data. This includes verifying the distribution of residuals and the equality of variance among groups. If assumptions are violated, the results may not be valid.
What diagnostic tools can be used after fitting a mixed effects model?
-Common diagnostics include plotting residuals versus fitted values to check for trends or patterns, and assessing the normal distribution of residuals within each group. This helps identify potential issues with model fit.
How does centering and standardizing independent variables improve model fitting?
-Centering and standardizing independent variables can enhance model convergence by addressing issues with large value ranges. It also allows for easier comparison of effect sizes among independent variables by measuring them in standard deviations.
What information is typically included in the output of a mixed effects model?
-The output generally includes model specification and fit, random effects estimates, fixed effects coefficients and their standard errors, and correlation between fixed effects. However, p-values are often not reported due to difficulties in estimating degrees of freedom.
What is partial pooling or shrinkage in the context of mixed effects models?
-Partial pooling or shrinkage refers to the process of using information from the overall population distribution to inform estimates for individual groups, especially those with small sample sizes. This approach helps improve the accuracy of parameter estimates.
Outlines
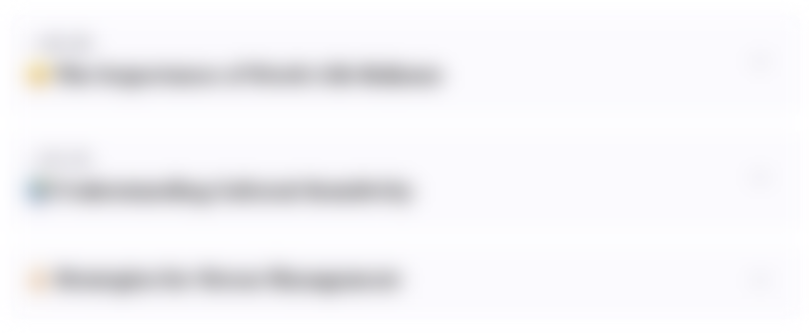
This section is available to paid users only. Please upgrade to access this part.
Upgrade NowMindmap
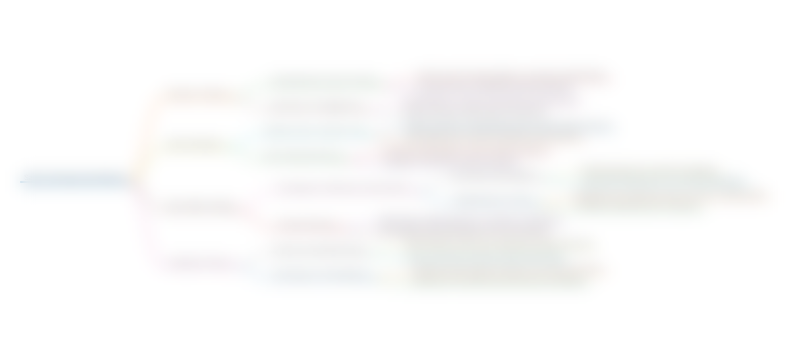
This section is available to paid users only. Please upgrade to access this part.
Upgrade NowKeywords
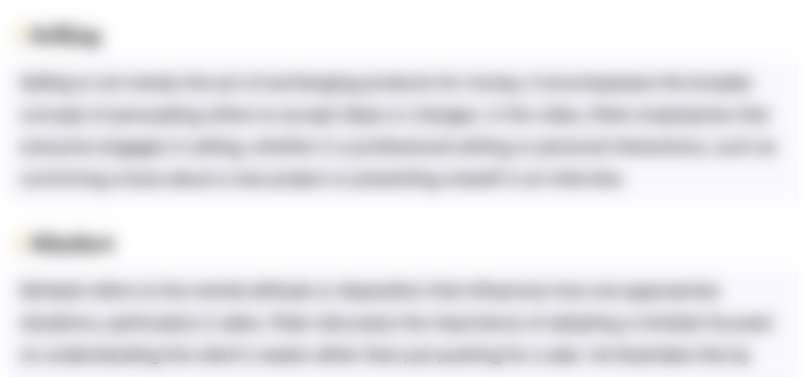
This section is available to paid users only. Please upgrade to access this part.
Upgrade NowHighlights
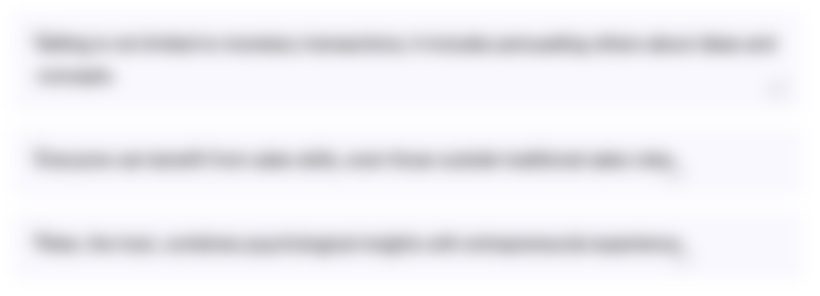
This section is available to paid users only. Please upgrade to access this part.
Upgrade NowTranscripts
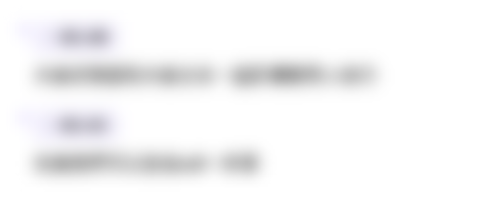
This section is available to paid users only. Please upgrade to access this part.
Upgrade NowBrowse More Related Video
5.0 / 5 (0 votes)