Energy, not compute, will be the #1 bottleneck to AI progress – Mark Zuckerberg
Summary
TLDRThe video script discusses the challenges and future prospects of GPU production and its impact on AI development. It highlights the recent supply constraints that have limited the availability of GPUs, even for companies with sufficient funds. The speaker anticipates a shift towards significant investment in building out GPU infrastructure, but raises concerns about potential energy constraints. They compare the energy consumption of a hypothetical gigawatt-scale training cluster to that of a nuclear power plant, emphasizing the regulatory and logistical hurdles in establishing such facilities. The summary also touches on the exponential growth of data centers and the need for substantial capital investment to keep pace with technological advancements. The speaker concludes by acknowledging the uncertainty in predicting the trajectory of AI scaling and the potential for encountering various bottlenecks along the way.
Takeaways
- 💰 **Supply Constraints**: There have been recent issues with GPU production, leading to supply constraints even for companies with sufficient funds.
- 🚀 **Investment in Infrastructure**: Companies are now considering significant investments to expand their GPU infrastructure.
- ⏳ **Energy Limitations**: Energy constraints may become a limiting factor before financial investment does, due to the extensive energy requirements for large-scale AI model training.
- ⚡ **Gigawatt Scale**: A single training cluster at the gigawatt scale is comparable to a nuclear power plant's output, highlighting the energy-intensive nature of advanced AI training.
- 🏭 **Regulatory Hurdles**: Building new power plants and transmission lines for such facilities is heavily regulated and can take many years to permit.
- 💡 **Long-Term Projects**: Establishing massive facilities to support AI training is a long-term endeavor due to the time required for regulatory approval and construction.
- 📈 **Exponential Growth**: There's uncertainty about how long the exponential growth in AI capabilities will continue, affecting investment decisions.
- 🏗️ **Data Center Scale**: Many data centers are in the range of 50 to 150 megawatts, and companies are building the largest clusters possible within these constraints.
- 🔌 **Future Expansion**: The construction of data centers at scales of 300 megawatts, 500 megawatts, or even a gigawatt is not yet a reality but is anticipated in the future.
- 🌐 **Global Impact**: The potential for truly groundbreaking AI advancements is significant, warranting substantial investments in infrastructure.
- 🔮 **Uncertain Future**: Industry experts cannot definitively predict the continuation of current scaling rates for AI, and there may be unforeseen bottlenecks ahead.
Q & A
What has been the issue with GPU production in recent years?
-There have been supply constraints in GPU production, which even affected companies with sufficient funds to purchase GPUs. They couldn't acquire as many as they wanted due to limited availability.
Why are companies now considering investing heavily in building out GPU clusters?
-As the supply constraints are easing, companies are seeing an opportunity to invest in building larger GPU clusters to capitalize on the potential for advancements in AI and machine learning.
What is the capital question companies are facing?
-Companies are questioning at what point further investment in GPU clusters stops being financially worthwhile due to diminishing returns.
What energy constraints are mentioned as a potential bottleneck for large-scale GPU cluster development?
-The energy required to power large clusters could become a bottleneck, as building and permitting new power plants and transmission lines is a heavily regulated and time-consuming process.
How does the speaker put the energy consumption of a gigawatt training cluster into perspective?
-A gigawatt training cluster's energy consumption is comparable to that of a significant nuclear power plant, which is solely dedicated to training AI models.
What is the typical size of data centers in terms of energy consumption?
-Many data centers operate on the order of 50 to 100 megawatts, with larger ones reaching up to 150 megawatts.
Why is building a data center with a capacity of 300 megawatts or a gigawatt considered challenging?
-Apart from the technical and financial challenges, building such large data centers involves significant regulatory hurdles and long lead times due to energy permitting and infrastructure development.
What is the speaker's view on the future of building gigawatt-scale data centers?
-The speaker believes it will eventually happen, but it is not an immediate prospect and will take considerable time to plan and execute.
How does the speaker assess the risk of investing heavily in AI infrastructure?
-The speaker sees value in investing tens or hundreds of billions in infrastructure, assuming exponential growth in AI continues, but acknowledges the uncertainty and potential bottlenecks in scaling.
What historical pattern does the speaker refer to regarding exponential growth?
-The speaker refers to the historical pattern where exponential growth in a field often hits bottlenecks at certain points, which may be overcome quickly if there is significant focus and investment.
What does the speaker imply about the relationship between capital investment and AI model improvement?
-The speaker implies that simply investing more capital does not guarantee a sudden leap in AI model capabilities; there are various bottlenecks that need to be addressed along the way.
What is the main challenge in planning for exponential growth in AI?
-The main challenge is predicting how long the exponential growth will continue, as it is difficult to plan around such growth, especially when considering the long-term infrastructure projects required.
Outlines
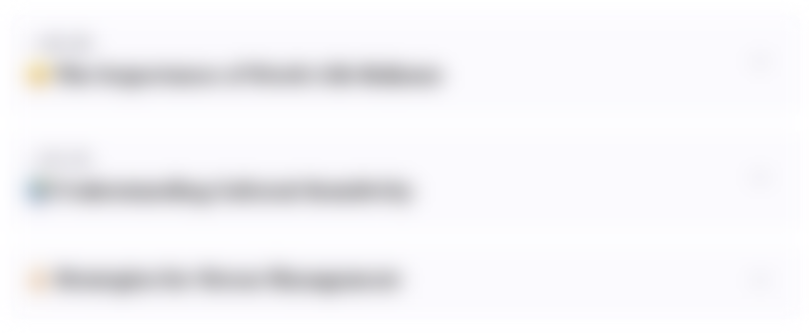
This section is available to paid users only. Please upgrade to access this part.
Upgrade NowMindmap
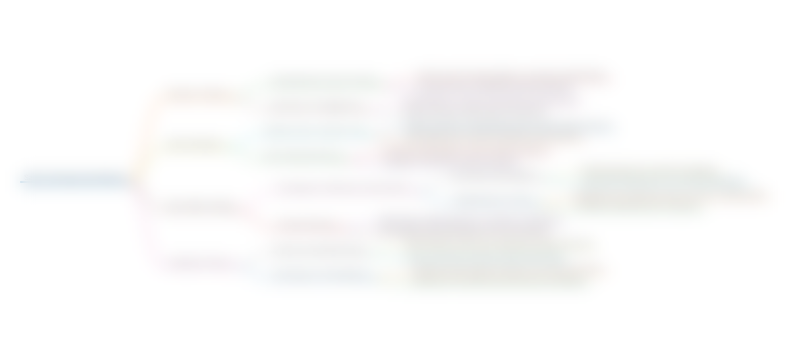
This section is available to paid users only. Please upgrade to access this part.
Upgrade NowKeywords
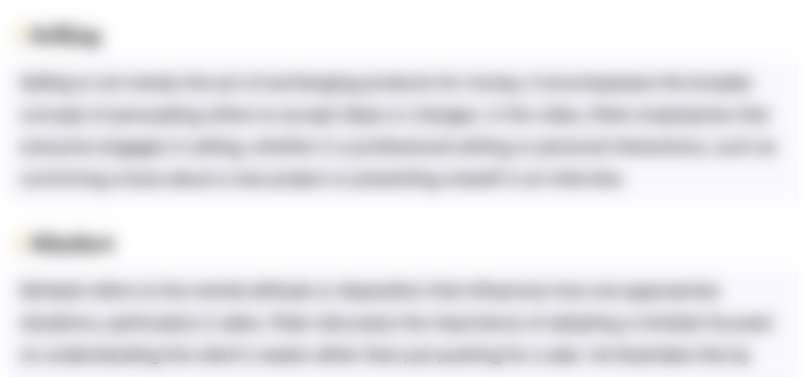
This section is available to paid users only. Please upgrade to access this part.
Upgrade NowHighlights
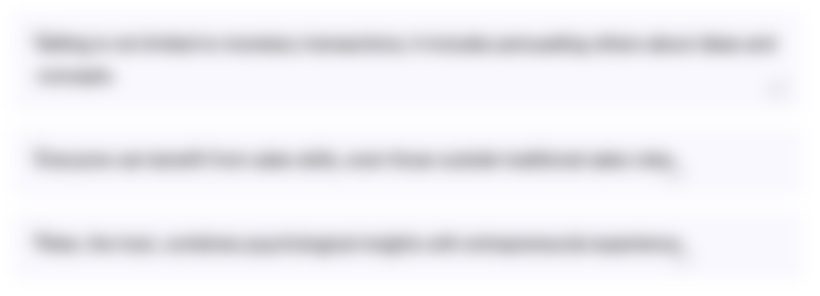
This section is available to paid users only. Please upgrade to access this part.
Upgrade NowTranscripts
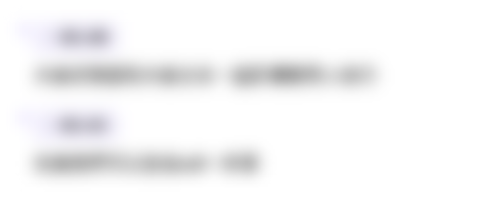
This section is available to paid users only. Please upgrade to access this part.
Upgrade NowBrowse More Related Video
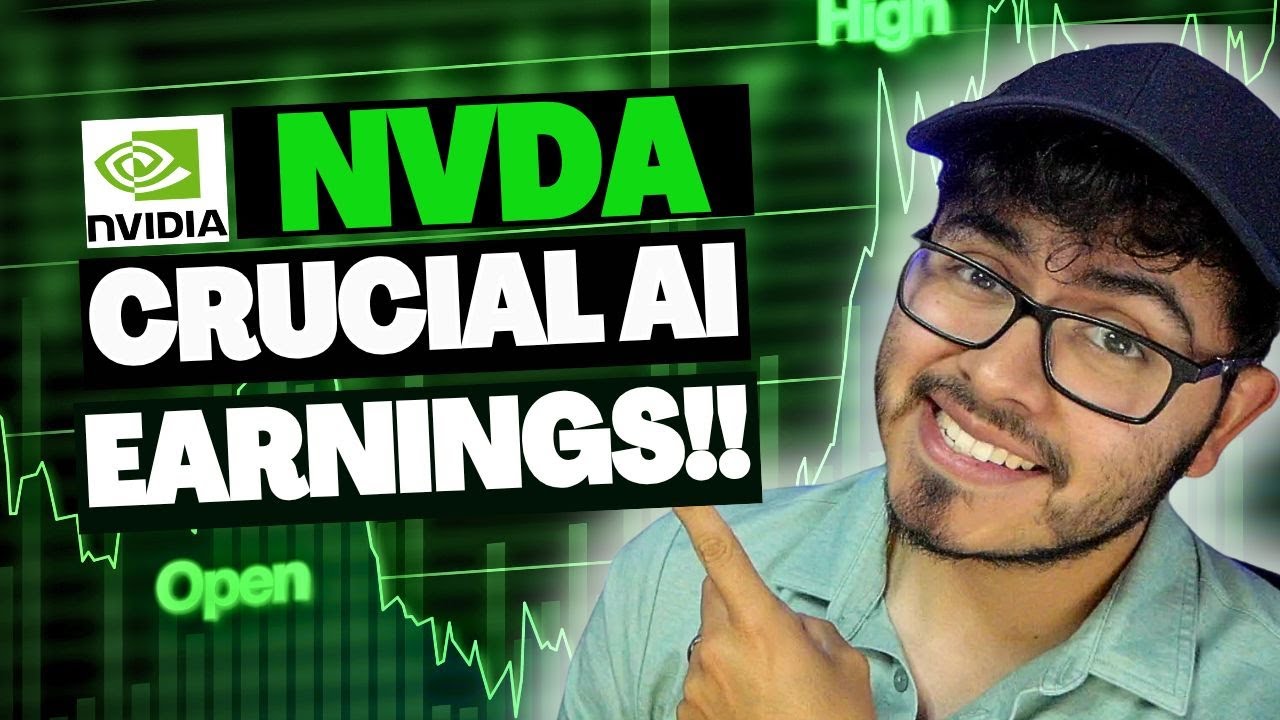
Nvidia Stock CRUCIAL AI EARNINGS You Need to Know! NVDA Stock
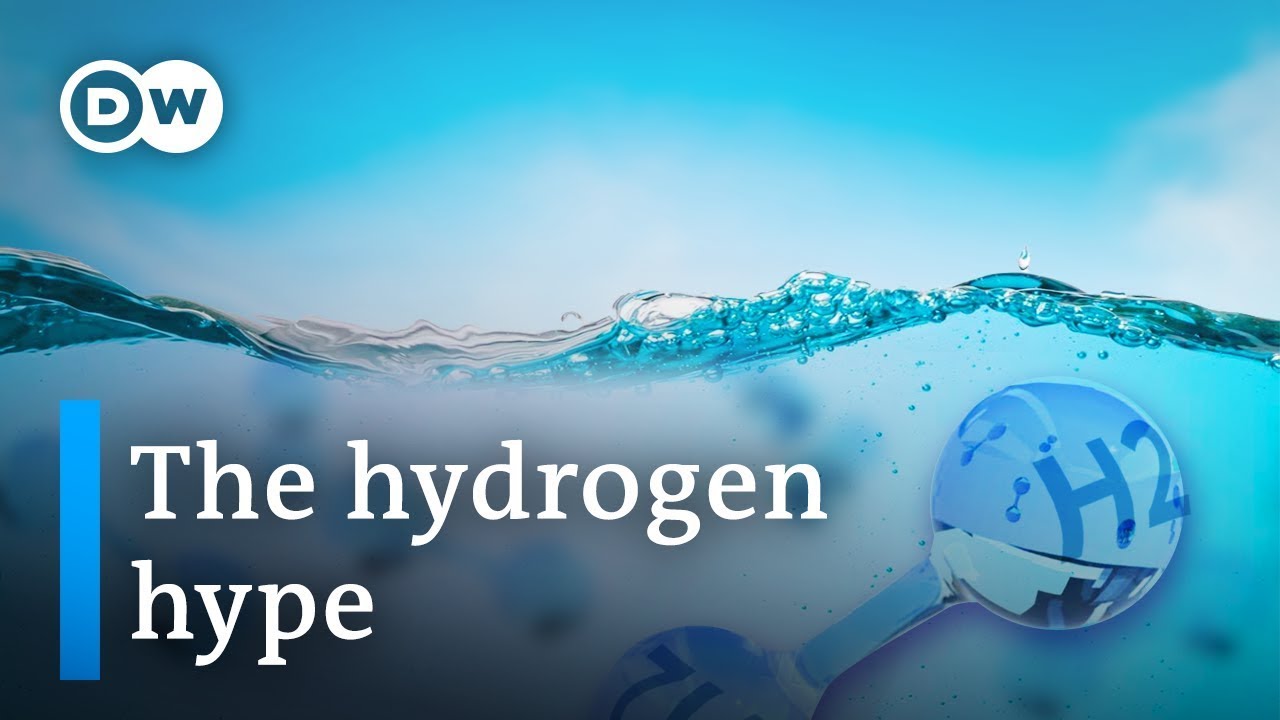
The truth about hydrogen

トロッコ問題+自動運転|功利主義?義務論?見れば分かります!【未来予測 シンギュラリティ】人工知能 AI

Brussels my love? Get ready, get set for a major year of elections around the world
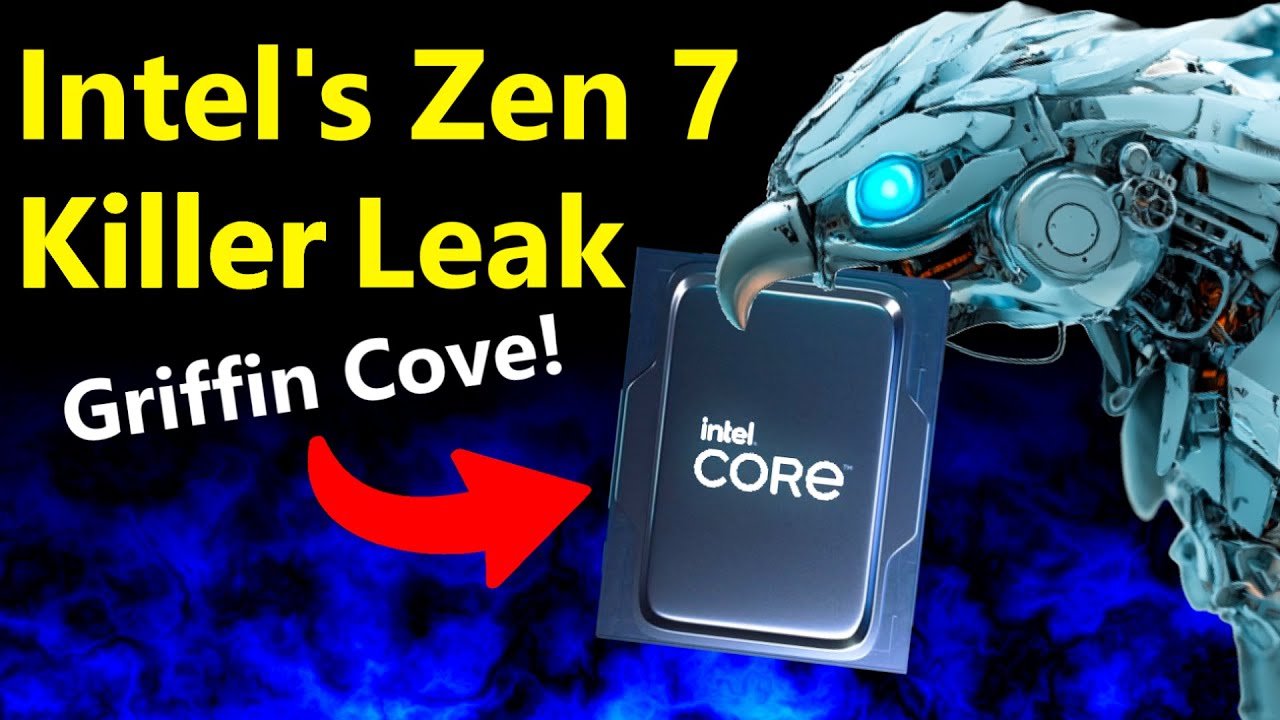
Intel Griffin Cove Leak: Killing AMD Zen 7 without E-Cores! (+ ARC Battlemage Update)

Will AI Steal Your Job?How AI Will Transform Daily Life
5.0 / 5 (0 votes)