Forecast Accuracy & Time Series Regression | SCMT 3623
Summary
TLDRThis lesson focuses on assessing forecast accuracy, emphasizing two key dimensions: magnitude and bias. A good forecast closely matches actual demand, minimizing forecast error. The lesson explains how to calculate forecast error, including the mean absolute deviation (MAD) and mean absolute percentage error (MAPE), which measure error magnitude. It also discusses forecast bias, evaluated using the mean error. By comparing over- and under-predictions, learners can choose reliable forecasting methods. Understanding both magnitude and bias helps improve forecast quality and select the best forecasting technique for accurate demand prediction.
Takeaways
- 🔍 A good forecast is one that closely matches actual demand, minimizing the deviation between predicted and actual demand.
- 📊 Forecast accuracy is measured through the forecast error, defined as the difference between actual demand and predicted demand for a specific period.
- 🧮 The last period demand forecasting method is used to generate in-sample forecasts based on past demand.
- 📉 Mean absolute deviation (MAD) measures the average magnitude of forecast errors, indicating how far forecasts are from actual demand.
- ⚖️ A lower mean absolute deviation suggests better forecast quality, while higher values suggest worse accuracy.
- ⚖️ Forecast bias occurs when predictions systematically overestimate or underestimate demand, which can be measured by the mean error.
- 🔁 The mean error helps determine whether forecasts consistently over-predict or under-predict demand.
- 📊 Various metrics are used to evaluate forecast accuracy, such as mean absolute percentage error (MAPE), mean squared error (MSE), and root mean squared error (RMSE).
- 📏 Bias in forecasts is addressed by assessing both under-prediction and over-prediction, comparing these patterns to calculate overall error.
- 🎯 To ensure reliable forecasts, both the magnitude of forecast errors and bias need to be measured and compared across different forecasting techniques.
Q & A
What defines a 'good' forecast according to the lesson?
-A good forecast is one that is very close to actual demand, meaning the deviation between predicted and actual demand is low. Additionally, the forecast should be unbiased, meaning there is no systematic over or under prediction of demand.
What is forecast error, and how is it calculated?
-Forecast error is the difference between actual or realized demand (yₜ) and the predicted demand (ŷₜ) for the same period. It shows how far off the forecast was from the actual demand.
How can we visually assess forecast accuracy in the provided data example?
-In the example, the actual demand is shown by the orange line, while the forecast is represented by the blue line. The difference between the two lines for each period represents the forecast error.
What does the lesson say about the importance of forecast bias?
-Forecast bias refers to whether predictions systematically overestimate or underestimate demand. The goal is to avoid a consistent pattern of over or under prediction. The mean error is used to measure this bias.
What are the two key dimensions of forecast accuracy discussed in the lesson?
-The two key dimensions are the magnitude of forecast errors (how far off the forecasts are on average) and the bias in forecasts (whether the forecasts consistently over or under predict demand).
What metric is used to assess the average magnitude of forecast errors?
-The mean absolute deviation (MAD) is used to assess the average magnitude of forecast errors. It tells us how far off the forecasts are, on average, from the actual demand.
What does a higher mean absolute deviation indicate?
-A higher mean absolute deviation indicates that the forecasts are less accurate, meaning the average forecast error is larger and the quality of the forecast is worse.
How is the bias in forecasts measured, and what does it show?
-Bias is measured using the mean error. This value compares the total over-prediction against the total under-prediction. If the mean error is close to zero, the forecast has little to no bias.
What other metrics are used to assess the magnitude of forecast errors besides MAD?
-Other metrics include the mean absolute percentage error (MAPE), mean squared error (MSE), and root mean squared error (RMSE). Each provides different perspectives on the size of the forecast errors.
Why is it important to assess both the magnitude and bias of forecasts?
-Assessing both the magnitude and bias is important because it helps determine the overall quality of the forecast. It allows comparison between forecasting methods and helps in selecting the most reliable technique for specific situations.
Outlines
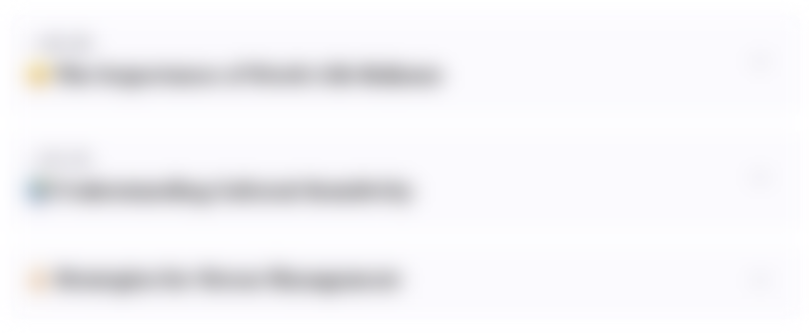
This section is available to paid users only. Please upgrade to access this part.
Upgrade NowMindmap
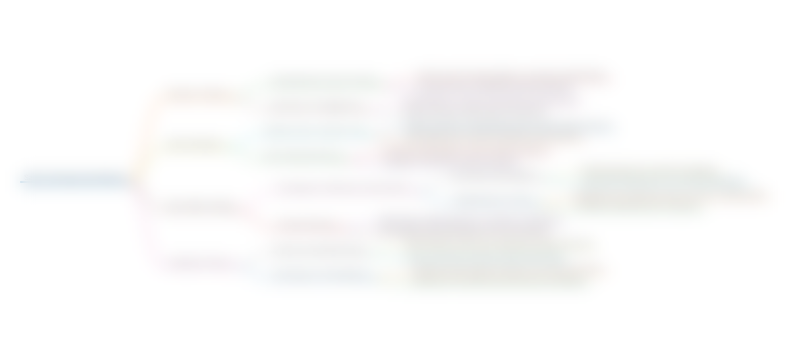
This section is available to paid users only. Please upgrade to access this part.
Upgrade NowKeywords
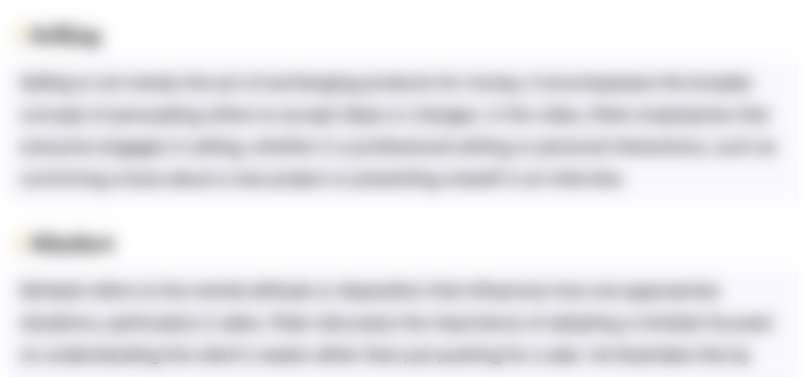
This section is available to paid users only. Please upgrade to access this part.
Upgrade NowHighlights
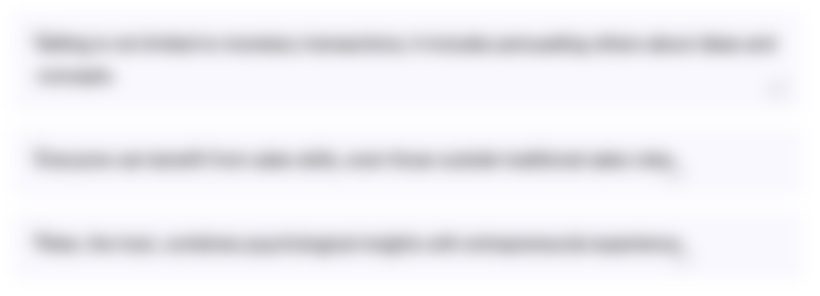
This section is available to paid users only. Please upgrade to access this part.
Upgrade NowTranscripts
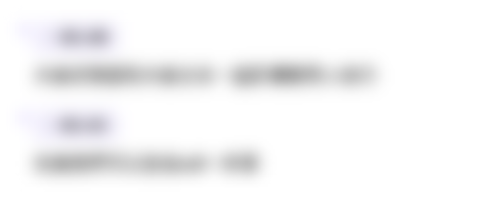
This section is available to paid users only. Please upgrade to access this part.
Upgrade NowBrowse More Related Video

Operations & Supply Chain Management: Forecasting & Demand Planning
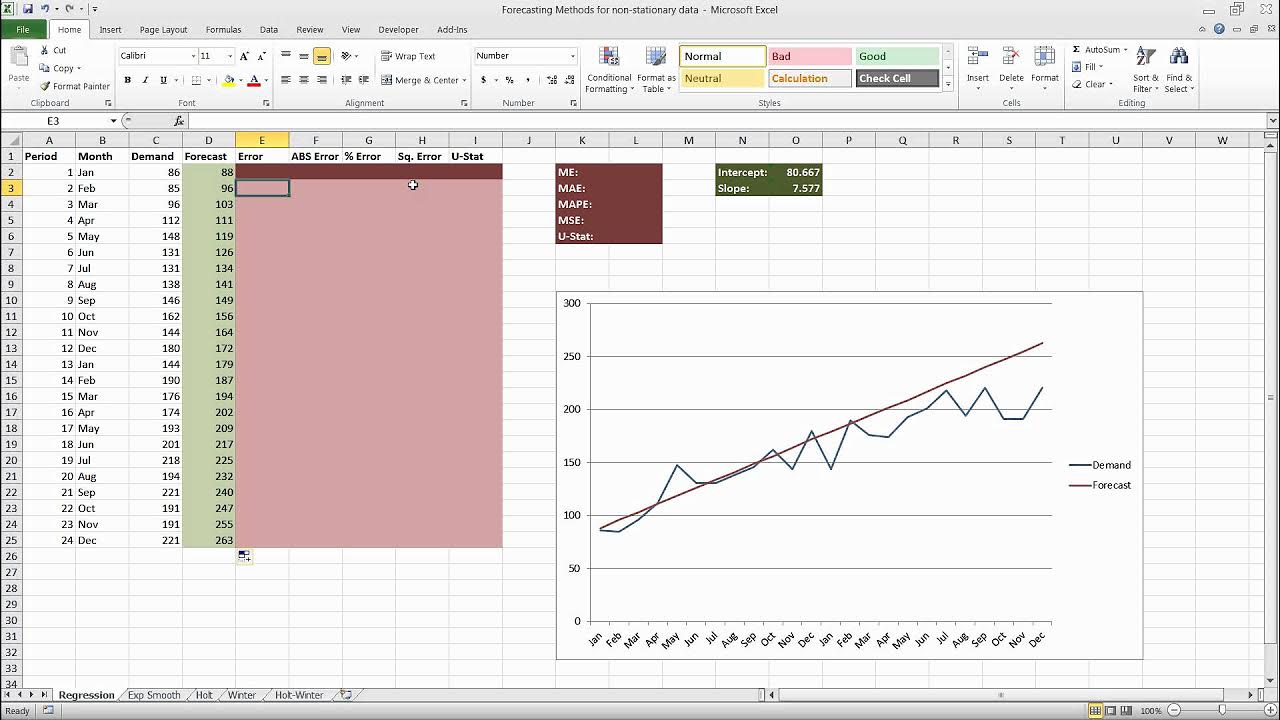
Forecasting in Excel Using Simple Linear Regression
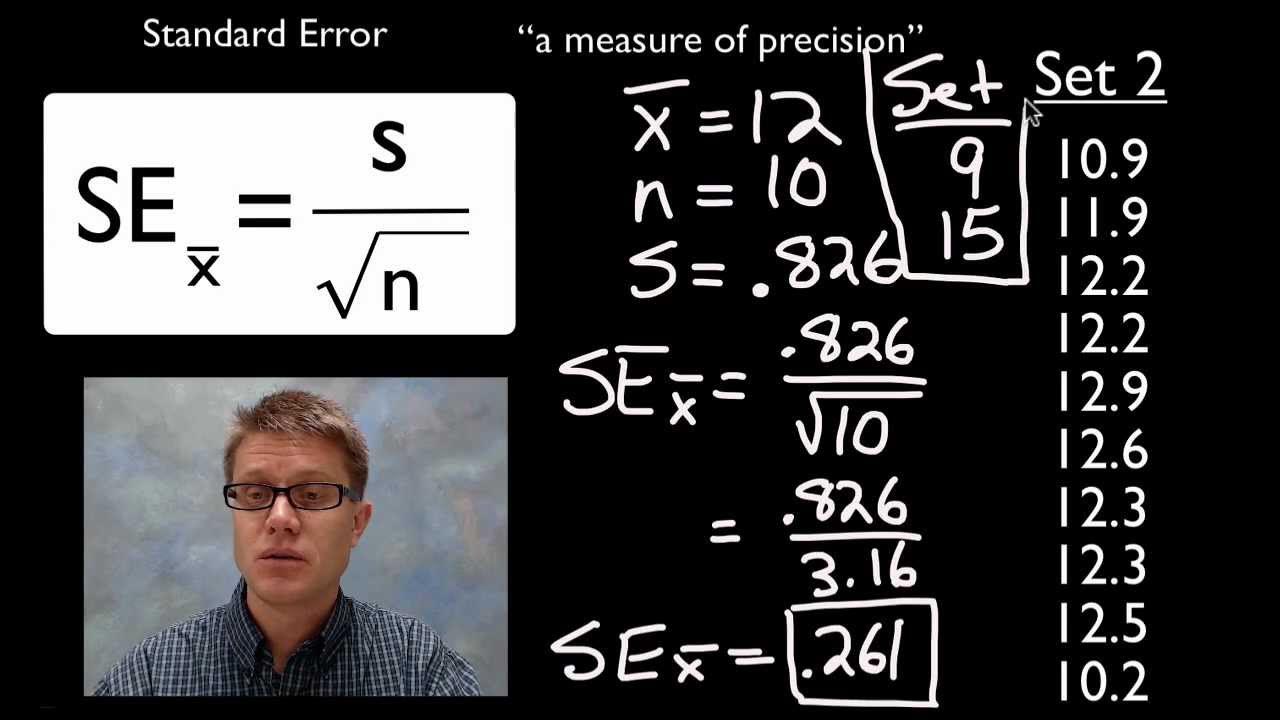
Standard Error
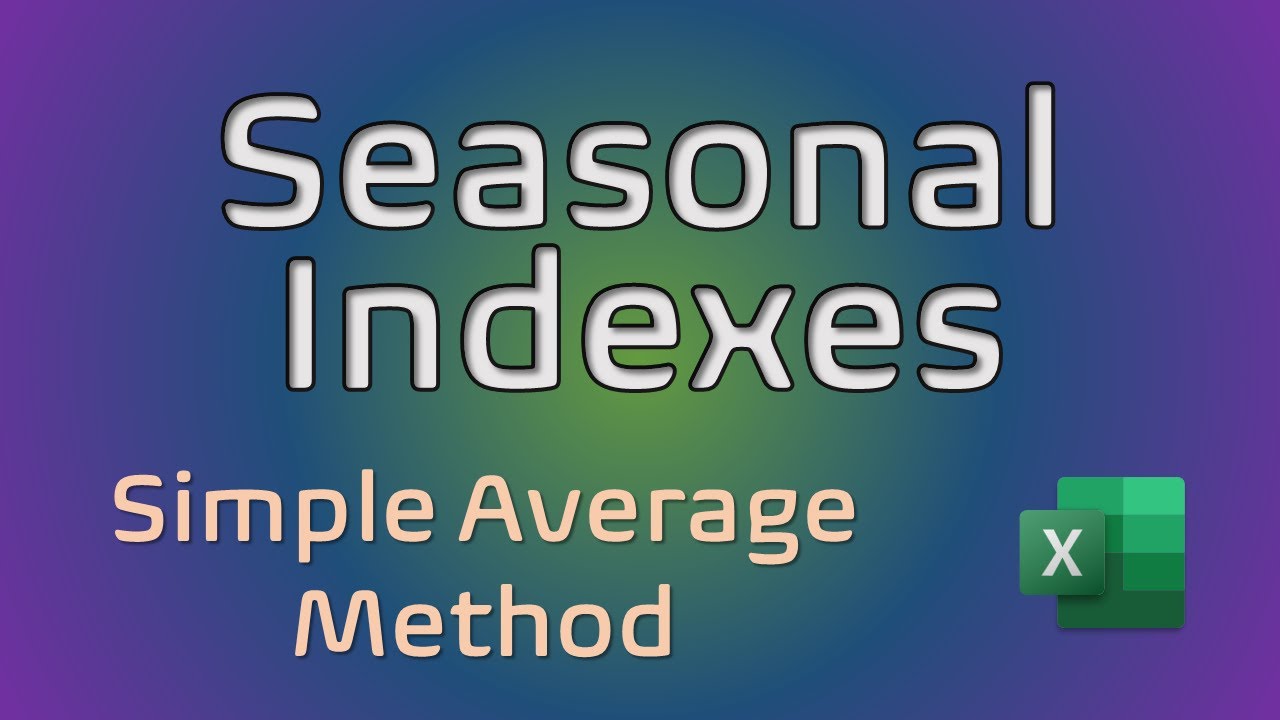
Calculating Seasonal Indexes (without trend) in Excel | Forecasting

Calculating Power and the Probability of a Type II Error (A One-Tailed Example)

Video Materi METROLOGI INDUSTRI Error dalam Pengukuran
5.0 / 5 (0 votes)