Hypothesis Testing: One Sided vs Two Sided Alternative | Statistics Tutorial #14 |MarinStatsLectures
Summary
TLDRThis transcript explains the difference between one-sided and two-sided hypothesis tests. It discusses a scenario where a mean BMI from 2008 is compared to a sample from 2018 to determine if there's an increase. In a one-sided test, the alternative hypothesis focuses on the mean being greater than the 2008 value, while the two-sided test explores whether the mean is different. The transcript emphasizes that the p-value alone shouldn't determine conclusions and suggests considering the overall study design, effect size, and other factors in decision-making.
Takeaways
- 🔍 The difference between one-sided and two-sided hypothesis tests is the focus on the direction of the deviation from the null hypothesis.
- 📊 In a one-sided test, we only look at deviations in one direction (e.g., whether the mean in 2018 is greater than in 2008).
- 📉 In a two-sided test, we examine deviations in both directions, considering the possibility that the mean could be either greater or less than the hypothesized value.
- 📐 For the one-sided test, the test statistic (Z-score) was 2.08, leading to a p-value of 1.9% when using the Z-distribution and 2.4% with the t-distribution.
- ⚖️ The two-sided test considers the probability of being 2.08 standard errors away in either direction, giving a p-value of 4.8%.
- 🔗 The p-value in a two-sided test is roughly double that of a one-sided test, but the practical difference isn't huge for small p-values.
- 📉 P-values are useful, but should not be the sole decision factor in research; they provide one piece of evidence.
- 📚 It’s essential to look beyond p-values, considering factors like effect size, confidence intervals, study design, and power.
- 🚫 Avoid treating alpha (0.05) as a strict cutoff, and instead focus on the broader context of the data and research question.
- 📖 The American Statistical Association’s statement on p-values emphasizes that a single number should not dictate conclusions in scientific research.
Q & A
What is the null hypothesis in the given example?
-The null hypothesis in the example is that the mean BMI in 2018 has not changed from the mean BMI in 2008, which is 25.3.
What are the differences between a one-sided and a two-sided alternative hypothesis?
-In a one-sided alternative hypothesis, we are testing if the mean has changed in one direction (greater or less). In the example, it checks if the mean BMI in 2018 is greater than in 2008. In a two-sided alternative hypothesis, we test if the mean has changed in either direction (greater or less) from the 2008 mean.
What is the test statistic in the given example?
-The test statistic in the example is 2.08, which represents how many standard errors the sample mean is from the hypothesized population mean.
How is the p-value for a one-sided test interpreted in the example?
-For the one-sided test, the p-value represents the probability of getting an estimate 2.08 standard errors or more above the hypothesized mean if the null hypothesis is true. This p-value comes out to be 1.9% when using the Z-distribution and 2.4% with the t-distribution.
How does the two-sided p-value differ from the one-sided p-value in the example?
-The two-sided p-value is double the one-sided p-value because it considers deviations on both sides of the hypothesized mean. In the example, the two-sided p-value is 4.8%.
Why might the two-sided test be considered more conservative or fair?
-The two-sided test is considered more conservative because it accounts for deviations in both directions from the null hypothesis, not just in one direction. This approach is viewed as a fairer test of whether there is a significant difference from the hypothesized value.
Why is it important not to focus solely on the alpha value of 5%?
-It is important not to focus solely on the 5% alpha value because it is not a 'magical cutoff.' Both a p-value of 3% and 6% are considered similarly significant, and decisions should not hinge entirely on whether the p-value crosses the 5% threshold.
What does the speaker suggest about relying solely on the p-value for decision-making?
-The speaker suggests that relying solely on the p-value to make decisions is insufficient. Other factors, such as the effect size, confidence intervals, sample size, study design, and power, should also be considered when interpreting results.
What other aspects should be considered alongside the p-value when evaluating a study's findings?
-In addition to the p-value, factors like the effect size, confidence intervals, sample size, study design, margin of error, and statistical power should be taken into account when evaluating study findings.
What is the role of the American Statistical Association’s statement on p-values according to the speaker?
-The American Statistical Association’s statement on p-values emphasizes that p-values are just one tool among many in making research decisions and that they should not be used as the sole criterion for determining the validity of results.
Outlines
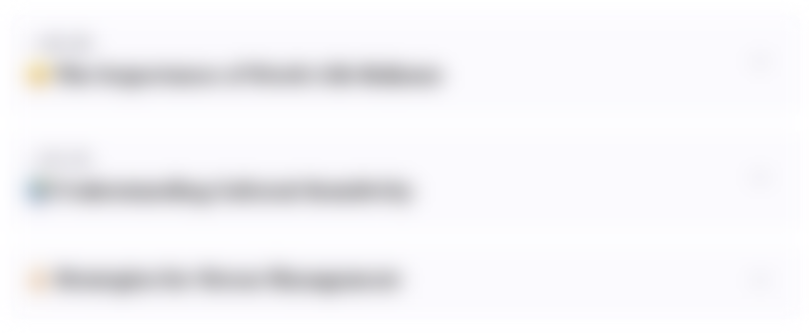
This section is available to paid users only. Please upgrade to access this part.
Upgrade NowMindmap
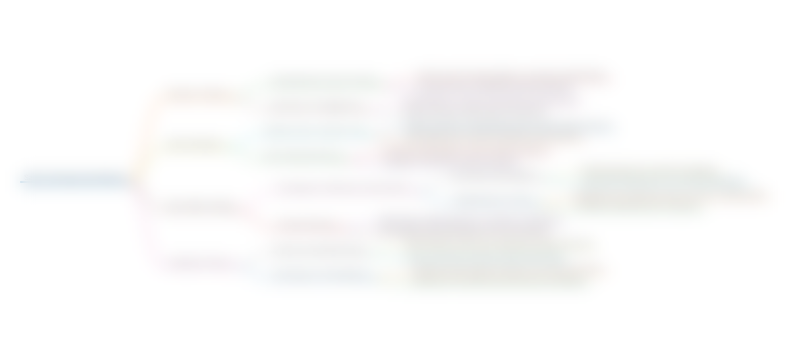
This section is available to paid users only. Please upgrade to access this part.
Upgrade NowKeywords
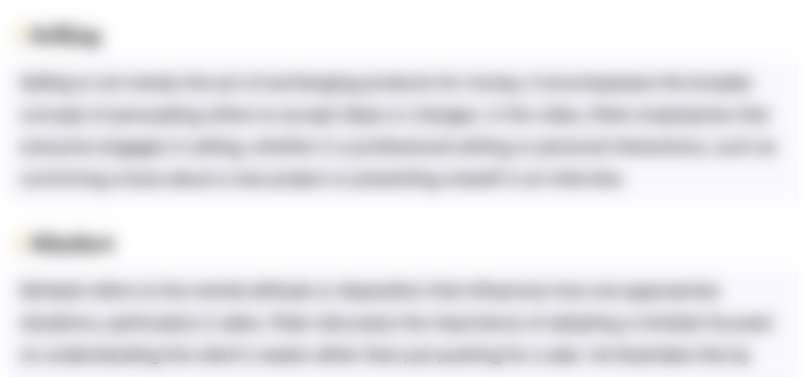
This section is available to paid users only. Please upgrade to access this part.
Upgrade NowHighlights
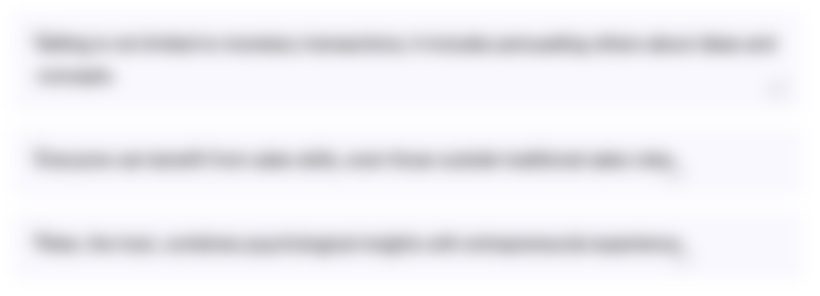
This section is available to paid users only. Please upgrade to access this part.
Upgrade NowTranscripts
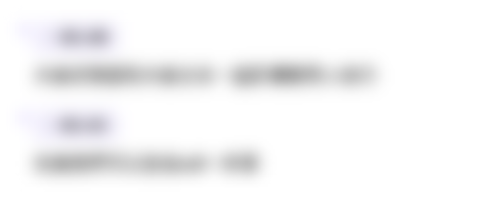
This section is available to paid users only. Please upgrade to access this part.
Upgrade NowBrowse More Related Video
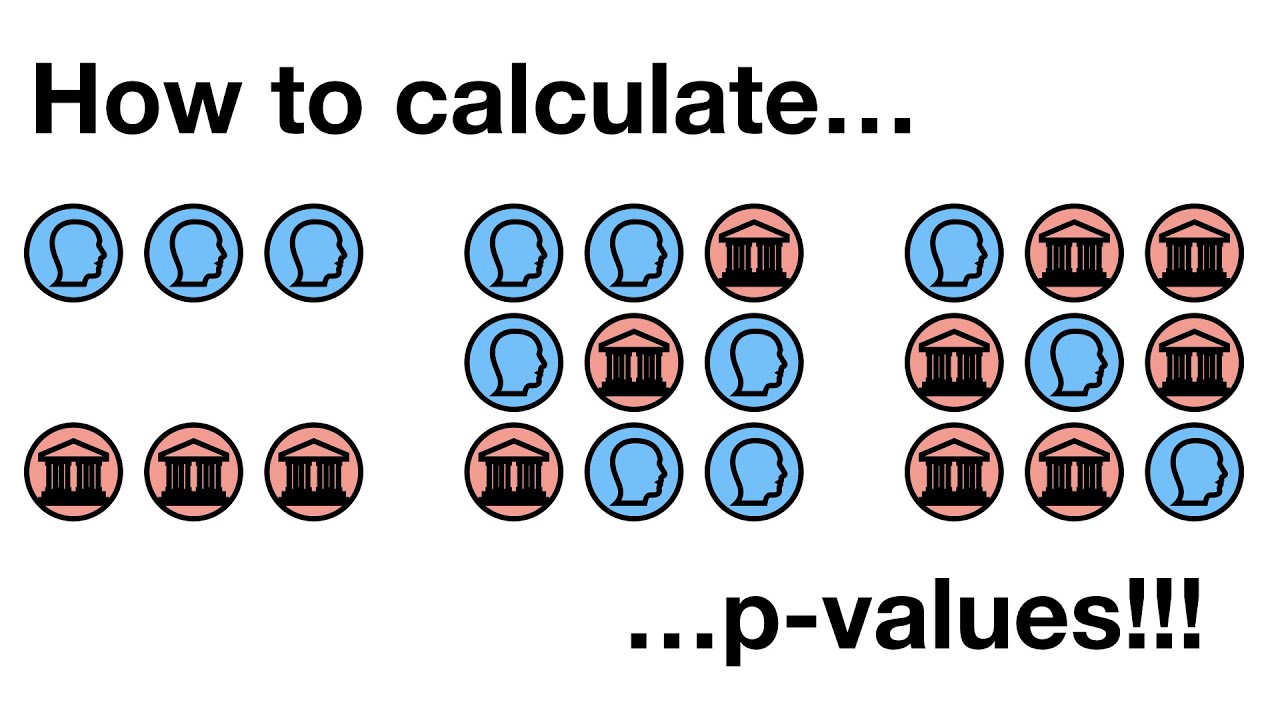
How to calculate p-values
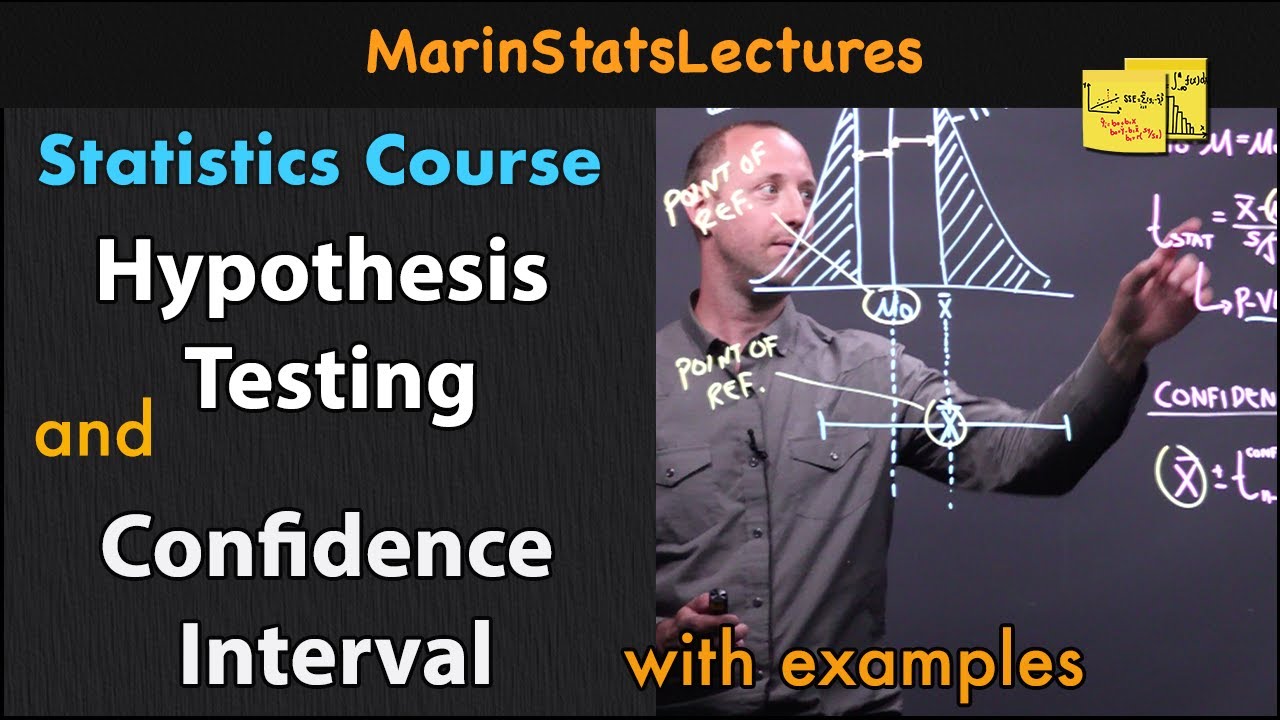
Hypothesis Test vs. Confidence Interval | Statistics Tutorial #15 | MarinStatsLectures

Understanding the Discrete Fourier Transform and the FFT

What is the difference between perspective and bias?
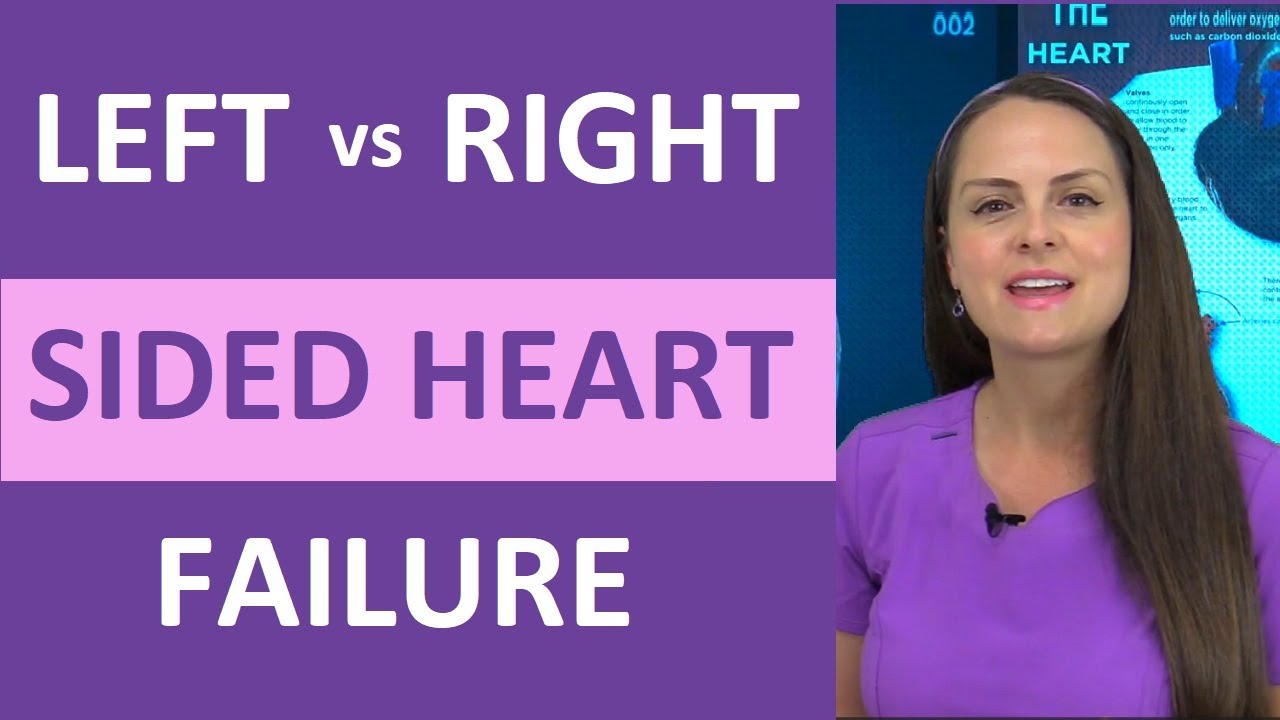
Left-Sided Heart Failure vs Right-Sided Heart Failure Pathophysiology Nursing NCLEX Review
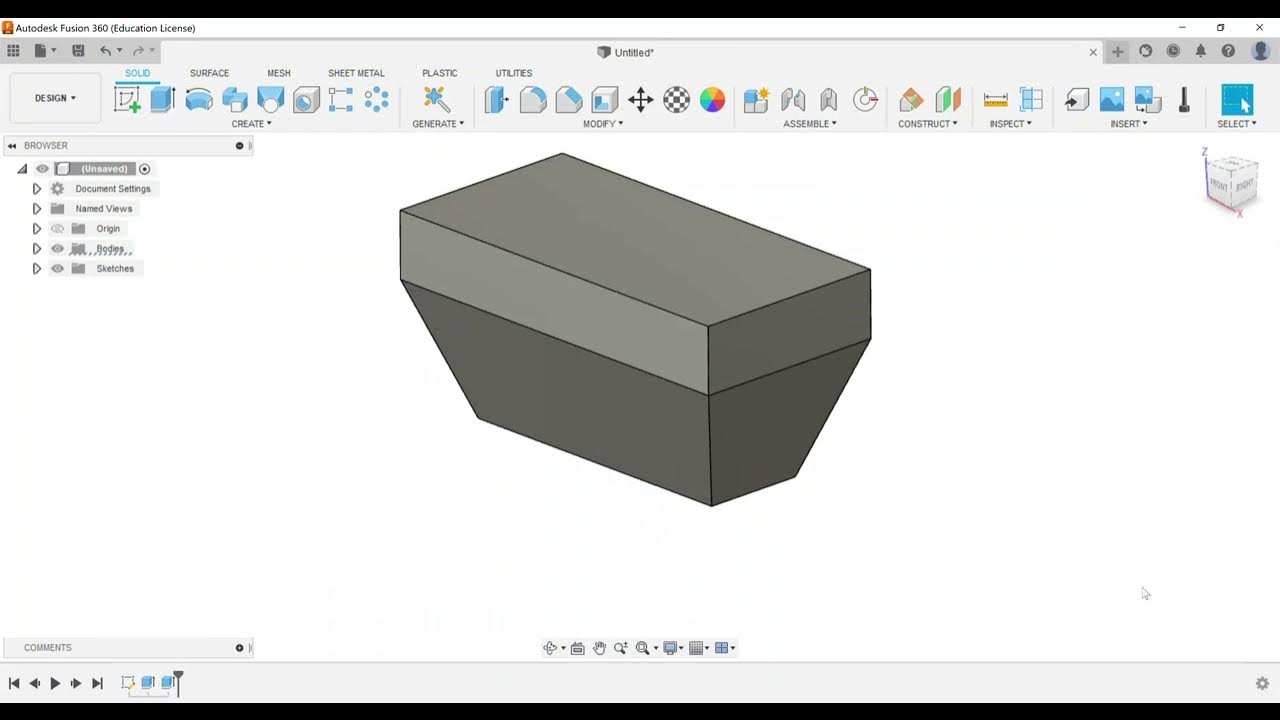
Part Modeling - Create Extrude Features
5.0 / 5 (0 votes)