Increasing AI Tool Adoption by Front-Line Workers
Summary
TLDRThe webinar, 'Increasing AI Tool Adoption by Front-Line Workers,' discusses the challenges of implementing AI in organizations due to resistance from end users. Experts Kate Kellogg, Mark Sendak, and Suresh Balu share research findings and tactics for successful AI integration, emphasizing the need to address user concerns about workflow and autonomy. They highlight the predictive, laborious, and prescriptive nature of AI and provide examples from various industries, including healthcare, to illustrate how these tactics can be applied effectively.
Takeaways
- π― AI implementations often fall short due to end user resistance, as they perceive few benefits and increased workload.
- π Research by Kate Kellogg, Mark Sendak, and Suresh Balu found that addressing end users' concerns about workflow and autonomy can lead to AI tool adoption.
- π₯ Involving end users from the beginning is crucial for successful AI implementation, as they have unique insights and needs.
- π‘ AI solutions should not only make regular work faster and easier (the sundae) but also offer new capabilities (the cherry on top).
- π To increase end user benefits, AI solutions must predict outcomes valuable to the user and reduce the labor involved in their tasks.
- π οΈ Developers need to influence top managers to change the reward system to align with AI outcomes and user adoption.
- π AI's laborious nature requires reducing the burden on end users, automating data input and pre-staging interfaces to meet their needs.
- π Integrating AI tools into existing workflows requires careful planning to ensure minimal disruption and seamless use.
- π Protecting end users' autonomy is key when implementing prescriptive AI, avoiding interference with core tasks they value.
- π Measuring the impact of AI tactics involves assessing clinical outcomes, process efficiency, cost-effectiveness, and addressing health disparities.
Q & A
What is the main challenge faced by organizations in implementing AI tools?
-The main challenge is that end users often resist adopting AI tools, especially those guiding decision-making, as they see few benefits for themselves and the new tools may add to their workload and reduce their autonomy.
How can developers ensure that AI tools are more readily adopted by end users?
-Developers can ensure adoption by addressing end users' concerns about workflow and autonomy, reconciling conflicting stakeholder interests, and increasing the benefits for the end users.
What are the three ways AI is different from other technologies?
-AI is different because it is predictive, laborious, and prescriptive, which can result in few benefits, additional labor, and decreased autonomy for employees.
What are the six tactics identified for successfully implementing AI solutions?
-The six tactics are not explicitly mentioned in the transcript, but they revolve around increasing end user benefits, reducing labor, and protecting autonomy while addressing workflow and stakeholder concerns.
How can organizations measure the success of AI implementations?
-Success can be measured by increased revenue, reduced costs, improved product and service quality, and most importantly, by the adoption rate and the positive impact on the end users' workflow and autonomy.
What role do front-line workers play in the adoption of AI solutions?
-Front-line workers play a crucial role as they are the end users who interact directly with the AI tools. Their acceptance and proper use of these tools determine the success of AI implementations in achieving organizational goals.
How can AI solutions result in increased revenue and reduced costs for organizations?
-By improving product and service quality, AI solutions can lead to higher customer satisfaction and loyalty, resulting in increased revenue. Additionally, they can help optimize processes, reduce inefficiencies, and minimize waste, leading to reduced costs.
What is the significance of the 'ice cream sundae' analogy mentioned in the transcript?
-The 'ice cream sundae' analogy is used to illustrate the two-fold approach to AI solutions: the base (the sundae) represents the core functionality that makes regular work faster and easier, while the cherry on top represents the additional, innovative capabilities that AI can bring to a job.
What are some examples of industries where the six tactics for successful AI implementation can be applied?
-The transcript mentions examples from HR recruitment, sales, and fashion buying. These tactics are generalizable across industries, suggesting they could also be applied in healthcare, finance, marketing, and more.
How can developers address the issue of end users' resistance to AI tools?
-Developers can address resistance by involving end users from the beginning, understanding their needs and concerns, and designing AI tools that align with their workflow, provide clear benefits, and do not infringe on their autonomy.
What is the role of rewards and incentives in the adoption of AI tools?
-Rewards and incentives play a significant role as they can motivate end users to adopt AI tools. By tying rewards to outcomes that AI tools can improve, organizations can encourage end users to engage with and utilize the AI solutions effectively.
Outlines
π Introduction to AI Tool Adoption Webinar
The webinar, titled 'Increasing AI Tool Adoption by Front-Line Workers,' is moderated by Elizabeth Heichler from MIT Sloan Management Review. It addresses the common issue of AI implementations not meeting their goals due to end-user resistance. The discussion focuses on research by Kate Kellogg, Mark Sendak, and Suresh Balu, which identifies strategies for successful AI adoption. The webinar introduces the concept that employees are resistant to solutions that do not improve their work experience, highlighting the predictive, laborious, and prescriptive nature of AI and its impact on employees.
π Six Tactics for Successful AI Implementation
Kate Kellogg presents six tactics for successful AI implementation based on research conducted at Duke over five years. These tactics are applicable across industries and are illustrated with examples from different sectors. The tactics include increasing benefits for end users, addressing their concerns about workflow and autonomy, and reconciling conflicting stakeholder interests. The importance of involving end users from the beginning and changing the reward system to align with the new AI tools is emphasized.
π§ Case Study: AI in HR Recruitment
A detailed case study is presented about an AI solution for HR recruiters, specifically sourcers, who identify and attract candidates with technical skills. The AI tool aims to predict which candidates are likely to accept job offers. However, the true end users are the sourcers, who have different needs from the interviewers. The developers address the sourcers' concerns by introducing a pre-interview assessment and changing the reward system to include successful candidate acceptance of offers.
π€ Challenges in AI Adoption
The discussion highlights the challenges in AI adoption, such as the need for end users to bring their own requirements, which complicates development, and the difficulty developers face in influencing the reward system. It is stressed that involving end users from the start and understanding their needs is crucial for successful AI implementation. The webinar also touches on the labor-intensive nature of AI and the need to reduce the workload for end users.
π οΈ Example: AI for Salespeople
An example of an AI solution for salespeople is provided, illustrating how the AI tool was developed to reduce labor for end users. The organization involved sold manufacturers' products to wholesalers and retail chains. The AI tool was designed to help salespeople with white space analysis, identifying opportunities for sales based on clients' existing purchases and potential needs. The developers automated data inputting and pre-staged the interface to meet the salespeople's needs.
π Protecting Autonomy with AI
The importance of protecting end users' autonomy when implementing AI solutions is discussed. An example from the fashion industry is used to illustrate how AI can assist fashion buyers without infringing on their core creative tasks. The AI developers avoided interfering with the buyers' decision-making process and instead focused on helping with less enjoyable tasks. The example emphasizes the need to add the six tactics to the toolkit for successful AI adoption.
π‘ Real-World AI Application: Healthcare
Mark Sendak shares examples of AI application within the healthcare industry, specifically at Duke Health. He discusses the process of identifying projects for innovation and the importance of aligning senior strategic priorities with the needs of front-line workers. The discussion includes the challenges faced by primary care physicians in managing chronic diseases and the potential of AI to assist in preventing disease progression.
π©Ί Healthcare AI: Primary Care Physicians
The webinar delves into the specifics of how AI can support primary care physicians in managing kidney disease. It highlights the burden on physicians due to the progression of chronic diseases and the feedback they receive from specialists. An AI solution is presented that allows kidney specialists to send recommendations directly to primary care doctors, helping them manage kidney disease more effectively.
π₯ Integration of AI in Healthcare
The discussion continues with strategies for integrating AI in healthcare settings, focusing on reducing labor for end users and ensuring the AI tools are effectively used. The importance of aligning incentives for primary care doctors and involving downstream specialists in the development and validation of AI systems is emphasized. The webinar also touches on the challenges faced by emergency department doctors in managing various conditions.
π§ Understanding AI Decision Support
The webinar addresses the importance of front-line users understanding the nature of AI decision support. It emphasizes that AI provides predictions with a certain level of confidence, not definitive answers. The need for explainability in AI outputs is discussed, with the level of understanding required varying depending on the domain. In healthcare, a higher level of explainability is necessary due to the critical nature of the decisions being made.
π Measuring the Impact of AI
The panelists discuss how to measure the impact of AI technologies, emphasizing the need for adoption and continuous use for AI tools to generate value. They share their experiences from Duke Health, where they measure the effectiveness of AI tools by looking at clinical outcomes, process and adoption measures, cost and economics, and addressing health disparities. The importance of reducing the burden on clinicians to ensure adoption is highlighted.
π Monitoring Model Drift and Feedback
The importance of monitoring model drift and incorporating front-line user feedback is discussed. The panelists explain that model drift can occur due to changes in technology infrastructure or process and workflow shifts. They share examples from Duke Health, where they proactively monitor changes and update their models accordingly. The need for end users to understand the AI tools and provide feedback for continuous improvement is emphasized.
π Training and Educating Users on AI
The webinar addresses the importance of training and educating users on AI, especially in understanding that AI provides predictions with confidence levels rather than definitive answers. The panelists discuss the different levels of understanding required by different stakeholders and the need for AI explainability. They share experiences from Duke Health, where they ensure clinicians understand the algorithms used in AI tools and provide extensive documentation for different types of stakeholders.
π Final Thoughts on AI Adoption
Kate Kellogg concludes the webinar with final thoughts on AI adoption. She emphasizes the importance of the work being done in AI and the need for continuous learning, iteration, and support from developers, C-suite leaders, researchers, and funders. She encourages the audience to remember the front-line workers who will bring AI solutions into the real world and to focus on their needs and experiences.
Mindmap
Keywords
π‘AI Tool Adoption
π‘Predictive AI
π‘Labor Intensive
π‘Prescriptive AI
π‘End User Concerns
π‘Workflow Integration
π‘Autonomy
π‘Incentives Alignment
π‘Data Science
π‘End User Benefits
Highlights
AI implementations are often falling short due to resistance from end users who see few benefits for themselves and increased workload.
Research by Kate Kellogg, Mark Sendak, and Suresh Balu found that addressing end users' concerns about workflow and autonomy can lead to successful AI adoption.
AI solutions can result in increased revenue, reduced cost, and improved quality for organizations, but may also lead to decreased autonomy for employees.
Six tactics have been identified for successfully implementing AI solutions, which are generalizable across industries.
AI is different from other technologies as it is predictive, laborious, and prescriptive.
To increase benefits for end users, developers should focus on improving outcomes and changing the reward system.
Reducing labor for end users involves automating data input and pre-staging the interface to meet their needs.
Protecting end users' autonomy involves avoiding infringement on their core tasks and seeking their input in solution design.
AI solutions should be designed to make regular work faster and easier, with the ability to do cool things as a 'cherry on top'.
End users' involvement from day one is crucial for the success of AI implementation.
Developers need to upwardly influence top managers to change the reward system for end users.
AI solutions should be integrated into clinical workflows to generate value and ensure continuous use.
Measuring the impact of AI solutions involves assessing clinical outcomes, process and adoption measures, cost and economics, and addressing health disparities.
Model drift is important to monitor, as it can indicate changes in technology infrastructure or process and workflow shifts.
Training and educating front-line users on the nature of AI decision support output is crucial for effective tool adoption.
Transcripts
- Hello, and welcome to our webinar,
"Increasing AI Tool Adoption by Front-Line Workers."
I'm Elizabeth Heichler, editorial director
at MIT Sloan Management Review,
and I will be your moderator.
Now to today's topic.
Many AI implementations are falling short of their goals
to help organizations improve product and service quality,
reduce costs, and increase revenues.
The reason is that end users often resist adopting AI tools,
especially those that guide decision-making
because they see few benefits for themselves,
and the new tools often add to their workload
while taking away some of their autonomy.
However, research by Kate Kellogg,
Mark Sendak, and Suresh Balu
has found that when developers ensure that end users'
concerns about workflow and autonomy are addressed,
conflicting stakeholder interests can be reconciled
and AI tools more readily adopted.
Kate is the David J. McGrath Jr.
Professor of Management and Innovation
here at the MIT Sloan School.
Mark and Suresh are at the Duke Institute
for Health Innovation, where Mark
is the population health and data science lead,
and Suresh is program director,
along with his role as associate dean
for innovation and partnership
for the Duke University School of Medicine.
Welcome Kate, Mark, and Suresh.
- Thanks, Elizabeth.
All right, everyone.
Let's get this webinar started.
Here is our punchline.
Employees don't like solutions that make their lives worse.
For organizations, AI solutions can result
in increased revenue, reduced cost, and improved quality,
but there's three ways that AI is different
from other technologies, and we'll talk about these.
It's predictive, it's laborious, and it's prescriptive.
So that means that for employees,
it can result in few benefits, additional labor,
and decreased autonomy.
We've been doing research at Duke over the last five years,
and we've identified six tactics
for successfully implementing solutions.
We've compared implementations that are successful
versus those that are failed.
And these six tactics are generalizable across industries.
So I'm gonna start by presenting three examples
of how you would use these tactics
in these other industries.
And then I'm gonna hand it off to Mark
who's gonna present examples from Duke Health.
We'll finish with Q&A.
So here are the six tactics, and let's take one at a time.
First, because AI is predictive,
you need to increase benefits for end users.
I'm gonna use an example of an AI solution
for HR recruiters, and in particular for sourcers.
So sourcers for this organization located
and attracted candidates.
They identified candidates
with difficult-to-find technical skills,
engaged with them to speak with them about the company,
and then handed them off to HR interviewers
to be interviewed.
And the actions taken upstream by these sourcers
had large consequences for the downstream interviewers
because the interviewers then need to screen them
for mutual fit.
And then the HR closers were the ones that offered the jobs.
So the interviewers really wanted the sourcers
to find candidates who are more likely to accept offers
once they were offered.
And so they came to the AI developers and they said,
give us a solution that will help the sourcers predict
which candidates will be accepting the offers.
The problem for that was that the interviewers
were not the true end users for the tool.
It was actually the sourcers who were gonna need
to use the tool, and they had different needs.
They wanted to hit their quotas by finding all candidates
with the desired skills, regardless of how likely
they were to accept the offer.
So the developers were smart and they said,
all right, so let's see what we can do to address
the pain points of the sourcers.
And what they found out was for the sourcers,
a big problem was that they would hand off these candidates,
and they would just sit there in the system for two weeks
before the interviewers could interview them
because the interviewers didn't have enough bandwidth.
So the developers said, okay,
why don't we do something different?
We're gonna introduce
a pre-interview assessment part of this,
where now, candidates are gonna get handed off,
and they're gonna have to do an assessment
of their coding skills
before they move to the interview stage.
And so, you may be sitting there thinking,
well, wait a minute, that's not data science.
Exactly.
And I had a developer just give me a great analogy for this.
And that is that for any AI solution,
it's like an ice cream sundae where the data science
is a cherry on top.
So if you think about your own life and all the things
you're trying to get done,
what you really wanna do is be able to get your regular work
done faster and more easily.
And that's the sundae.
And then that's great if you can also then do
these really cool things and do your job differently,
that's the cherry on top.
But you need to first give them the sundae.
They also were smart because they rewarded the outcomes
the solution was designed to improve.
And so historically, the sourcers had been rewarded
just for the number of candidates they passed on
who fit the desired skills.
And they changed the reward system.
So now, they would also be rewarded
for the number of candidates who accepted offers.
So this sounds so easy as you guys sit back there
in your offices and you think, of course I know how
to think about the needs of end users.
But what we found is that it's easier said than done
for two reasons.
The first is that these end users always
bring their own requirements,
and that complicates development.
And interestingly, it's not usually the developers
that have trouble with that.
It's usually the people who come to the developers
asking for the solutions.
So the HR interviewers in this case,
who say, "Oh yeah, we know the sourcers
are the true end users, but let's not get them involved yet.
Let's get a little further along before we bother them
and get them involved."
But you need to get them involved from day one.
And then the second reason it's hard is that developers
don't control the reward system.
So they need to upwardly influence top managers
in order to get the reward system changed,
and that happened in this case also,
but for AI implementation to be successful,
you are gonna need to increase end user benefits
in these two ways.
The next thing about AI is it's very laborious,
and you need to reduce the labor for the end users.
I'm gonna use an example of an AI solution
for sales people.
The sales people in this organization
sold manufacturers product in large quantities
to wholesalers and retail store chains.
And they spent a lot of their time
building personal relationships with customers
in order to identify new opportunities.
So they were very busy, the salespeople,
generating leads, making calls, responding to emails
in order to hit their sales target.
So they're busy people anyway,
and now, you're asking them to do all this labor.
It's not completely new that you've asked for feedback
for end users.
So for an example, in this organization,
they had a CRM system for sales.
And whenever they did a new iteration of development,
they would ask for feedback from the end users.
But what's different about AI is it requires feedback
in all of these different steps,
and all of you out there who are implementing AI,
know that it takes a long time to do.
And it's very laborious to do all of these steps.
And so you need to figure out how to reduce the labor
for the end users that are involved in doing these steps.
So in this organization,
they enlisted the head of process improvement,
who was the one who wanted the tool in the first place,
by the way.
The salespeople in the beginning,
really not very interested in this tool.
And they got the head of process improvement to perform
a lot of this data work originally.
And they also initially built the model
so it required a smaller amount of training data in order
to show proof of concept to the salespeople
and show them what the promise of this tool was.
They also reduced integration work for the end users
and they did this by automating the data inputting required
to use the tool as much as possible.
And they also pre-staged the interface
to meet the salespeople's needs.
So just to give you an example of that,
the sales people in this organization
did what's called white space analysis
where for any given client,
they would look at what are they already buying
versus what's the full product line
that they could be buying based on their industry
and based on particular demographics.
So the AI solution developers made it easier for them
to do that white space analysis, again,
giving them that whole ice cream sundae.
Again, sorry, easier said than done for two reasons.
First of all, end users are great at the data work
because they're the ones that know the ins and outs
of the data.
They know the idiosyncrasies, the best outcome measures.
So of course the developers would love
to have their input as much as possible.
And then when it comes to integration work,
they're also the best.
They know the daily workflow.
They know where this solution should best fit in.
And the developers know that if they enlist their help,
then these end users will also serve as super users
to persuade their peers to use the solution.
So the answer is not to completely avoid
asking end users for help.
It's just to use the labor
of the end users very judiciously.
Finally, because AI is prescriptive,
you need to protect the autonomy of end users.
And here, I'm going to use an example for a solution
for fashion buyers, from my colleague,
Melissa Valentine at Stanford.
And let me just start by saying,
it's pretty funny, but I'm gonna give you guys
a lesson on fashion.
If I have any friends on this webinar,
they're getting a really big kick out of this right now,
but it's a great example.
So let's see how I do.
Fashion buyers selected and ordered which clothing
would be sold to gain maximum profit.
So they traveled the world, looking at the fashion runways,
what are the best pieces?
Then they combined their knowledge of fashion
with their business sense to decide
what mix of brands to buy.
And that's what you would then see
in the spring season, online or when you're in stores
is what those fashion buyers decided to buy.
So top managers had always used a detailed planning process
to better help control the fashion buyer decision-making,
but AI was just a whole different level here,
where now, it was gonna use historical data
that incorporated the intuition of the fashion buyers
that they'd been using for years
to provide highly specific recommendations
and allow managers to track whether the buyers
were following them.
And so perhaps not surprisingly, the buyers said,
"Wait a minute.
We don't love the idea of introducing this solution
that's gonna allow people outside of fashion buying,
who know nothing about fashion,
to be shaping and critiquing our decision making.
So the AI developers were smart.
They avoided infringing on the end user core tasks.
So in fashion buying, what the fashion buyers loved to do
was the creative task of knowing fashion
and knowing what that assortment could be.
That's why they went into this job in the first place.
What they didn't like the scutwork,
was now figuring out which vendors
are gonna be the ones to supply
those different fashion items.
And so what the developers did was they developed a solution
that allowed the buyers to dictate the assortment,
but helped them allocate sizes across vendors
and do the scutwork piece that they didn't like to do.
They also asked the end users to help evaluate the solution.
So the end users initially said,
"Thank you very much for your fancy AI solution,
but I like my current planning process.
I understand my technology.
I'm not gonna look dumb in front of people.
I really know the ins and outs. I'm not interested."
And so the developer said, "Okay, that that's fine.
Do you mind helping us design an A/B test
to evaluate the performance of your current system
versus this new system?"
And they did that.
So then the fashion buyers would see,
wow, this new system is actually pretty good.
So great. Let's protect autonomy.
Easier set than done. Here's why.
First of all, intervening around core tasks
promises to yield greater gain.
So if you think about it, fashion buying is high up
in the value chain.
If you intervene there with an AI solution,
you are gonna affect everything downstream
in terms of revenues and gross margins.
If you intervene just around the vendor piece,
it's a lot less impact.
But what we found is intervening around a circumscribed set
of tasks with the solution that actually gets used
is a lot more effective than building
this perfect AI solution that intervenes
in exactly where you want to, but then doesn't get used.
The second thing that's hard
is when you do involve these end users,
especially if they're not so interested in this solution
to begin with, they are gonna select
the most challenging tests for you to do
to prove your solution.
So that's not so easy, but again,
we have found that it's necessary to protect autonomy
if you want this to be successful.
So we suggest that you add these six tactics
to your toolkit.
Why?
Because success doesn't arise from big data
and sparkling technologies.
Instead, it depends on these end users on the ground.
So here's stark reality.
If you wanna be successful,
you need to increase values for those who are working
on the front lines in order for AI to function
in the real world.
So with that, I'm going to go ahead and hand this off
to my colleague, Mark Sendak,
who's gonna take you through the Duke examples.
- Thank you, Kate, for walking us through the six tactics.
Hi, everybody. My name is Mark Sendak
and I'm speaking to you
from the Duke Institute for Health Innovation
where I work as a physician intrapreneur.
And I'm gonna take the six tactics that Kate
just walked us through,
and I'm gonna ground them in examples within healthcare,
a very kind of professional services-oriented industries.
So at Duke Health, we have two structured ways
of identifying projects for innovations.
On the left-hand side, this is our annual request
for applications where we align senior strategic priorities
from our leadership with the needs of front-line workers.
We've done about 100 projects through that process.
And then on the right-hand side,
we have more of an innovation "Shark Tank" competition
where we help commercialize IP and inventions
built at the university.
So I'm gonna go through the same six tactics
in the same buckets.
This will likely sound familiar.
You just heard about the great use cases
that Kate brought up from non-health care industry.
And I'm gonna tell you what this looks like
within a health system.
So to kick things off, I wanna help orient things
around patient experiences and provider experiences.
So we're gonna kick things off with a patient.
This could be a family member.
This could be yourself
if you have experience with a chronic illness,
where typically, there's some trajectory that starts
on the left-hand side with a healthy adult.
They may develop an early-stage chronic disease.
This could be diabetes, hypertension, kidney disease.
Gradually over time, that that condition can worsen.
The kidney function can worsen.
Liver function can worsen.
And ultimately, for some patients,
the organ function gets so bad that we need to start looking
at a way to replace that organ.
And so what that looks like at a place like Duke Health
is that that individual patient is gonna end up interacting
with our system differently.
So they may start by seeing a primary care physician,
but as a kidney disease, liver disease,
whatever organ it is, as that deteriorates,
they need to see additional specialists.
And ultimately, on the right-hand side,
you see that organ transplantation
is very high-cost, multidisciplinary team,
where you have very specialized surgeons
and immunotherapies.
So it really goes from upstream to downstream,
and more complex in the number of people involved.
So a lot of the problems that we work on,
and that we build AI or ML solutions for
is to prevent some downstream progression
and prevent the bad outcomes from happening.
But like Kate mentioned with the interviewers
and the sourcers in the HR process,
if we're trying to prevent downstream progression,
that often requires that there's somebody earlier
in the process,
which is really often the primary care doctor,
that is intervening earlier.
And so this may mean that the primary care doc
has to do more to manage the chronic diseases,
to really make sure that these conditions don't worsen.
So we all here may have heard
how burdened healthcare workers are.
So I'm gonna start with the example
of a primary care physician.
Primary care docs really do have to manage everything.
So we'll start off by talking about kidney disease,
where the kidney disease may progress.
A patient may have to go see a kidney specialist,
and the kidney specialist may tell the primary care doc,
"You could have done more to prevent
the kidney disease progression."
Same for patients with diabetes.
There's gonna be endocrinologists
who see really late stage diabetes.
Maybe they see kidney disease.
You can lose your vision,
retinal complications from diabetes
so you're gonna see endocrinologists
who tell the primary care docs,
"You could have done more earlier to manage this condition.
You could have done more and earlier
to manage heart disease, to manage liver disease,
to manage lung disease."
The list goes on.
So at the end of the day, you get these upstream workers
who are really burdened by all of these requests
from the downstream specialists, telling them,
"Hey, we could have done more earlier
and prevented these downstream complications."
So when we're building AI,
this really does change the tactics.
And so this first tactic is identifying
who your end user is, and addressing their problems.
So let's go back to our primary care doc.
So we're gonna use the example of kidney disease,
where there's nephrologists who are specialists
in caring for kidney disease patients.
And they're telling the primary care docs,
"You could have done more earlier to manage
this kidney disease."
But we have to take a step back and think about,
okay, what are the primary care doctors' pain points?
First off, they have limited time to address
all of the conditions.
So what we did with the AI is we helped a specialist,
a kidney specialist send recommendations directly
to the PCP to help them manage that kidney disease,
to help relieve some of the burden
of having to think about that specific condition.
Another problem for PCPs is that they go
from one 15-minute visit to the next 15-minute visit.
And that's day after day after day.
I'm sure folks in the audience have experienced this.
When you walk into a doctor's appointment,
you often have to reiterate the same thing multiple times
because there's not a lot of opportunity
to prepare for these visits.
And so what we did with the AI is that the specialist
sends the recommendation immediately before the visit.
That way, the information is teed up for the PCP
to be able to act on it.
And then lastly, it's the fact that,
like I mentioned before,
PCPs go in and out of visits all day
and they may not have the opportunity
to really follow-up with patients
and make sure that that patient with kidney disease,
where they recommended a change in the medication dose
or recommended that they see a dietician
or a kidney specialist, they may not actually
know whether that happens.
So what we did with the AI is we also complimented
the tool with care managers
who would actually follow-up on patients.
The second tactic, building off of Kate's examples,
is to align the outcomes and share in the reward
with your true end users.
Which in our case, is the primary care doctor.
The reality is if you are able to prevent progression
of kidney disease, you actually end up saving
a health insurer or a payer a lot of money.
So to put this in dollar estimates,
a dialysis crash start that happens in the hospital
can cost upward of $80,000.
And you've gotta imagine
if you have these kidney specialists telling the PCPs,
"This could have been avoided if we acted sooner,"
ultimately, we want to align the incentives
so the PCP can help also receive some of the shared savings
from avoiding these bad outcomes for kidney disease.
So we actually did work with our health system leader
to help align incentives for the downstream
and upstream users.
The next bucket of tactics relates to the labor involved
in building and validating AI systems.
So let's go back to our primary care doc example.
We have all of these downstream specialists telling
the primary care doc, "Hey, you could have managed
this chronic disease earlier
and prevented these bad outcomes."
Unfortunately in healthcare though,
those primary care docs aren't the only generalists
who get that kind of feedback all the time.
Another setting where this happens is emergency departments.
Probably another setting that many folks
in this audience are familiar with.
You show up, and the ED doc has to manage
whatever comes their way.
So for example, you have a certain type
of heart attack, an NSTEMI.
That is lower risk and patients don't have to go
to the ICU when they have this type of heart attack.
They have a better chance of doing well in the hospital
and returning home.
But what happens is that the ED docs,
in the time pressure that they face
going from one patient to the next,
they're just trying to make sure that patients
get the care they need.
So they send a lot of these patients
to the intensive care unit.
So you have intensive care unit docs going down to the ED
and saying, "Hey, if you identified this disease sooner
and you recognize that this was a low risk condition,
you actually don't need to send these patients to the ICU.
That's a really high cost resource,
and we can use that bed for somebody else."
Something similar happens for sepsis.
You have the hospital specialists coming down
to the ED saying, "Hey, if you had identified
and started treating sepsis sooner,
we could have avoided the bad outcomes for our patients
in the hospital."
The ED docs are even going upstream from their setting,
trying to say, "Okay, can we change EMS routing?"
So EMS, if you're able to route patients
to the other hospital, you can make it more efficient.
When there's patients backing up in the waiting room,
and you're waiting hours to see folks,
people are going to the ED docs saying,
"Hey, can you improve telemedicine within your setting?
That way, we can see people
in the waiting room more quickly.
And then the last example that we'll build off of,
it relates to blood clots that go to your lungs
called pulmonary embolisms.
And once again, this is trying to tell the ED docs,
"Hey, these low risk patients,
they don't need to come to the hospital.
You could actually send them back home."
So these ED docs similar to the primary care docs,
they really feel like they carry the weight of the world.
And I know what this looks like personally,
my wife is a pediatrician and a primary care doc.
And literally day after day,
you're only able to manage a very small portion
of the things that you encounter.
So when we're building technologies to try to improve
how we manage these conditions,
we have to be thinking
about how do we deploy the Mack Trucks to remove
some of that labor that's falling on these upstream users
who are inundated with requests to do their jobs better.
So tactic three is how do we reduce the labor
on those upstream end users to build out the datasets
for AI model development?
So what we did in this case
is we actually asked the downstream specialists
who care for pulmonary embolism
to adjudicate the cases,
make sure that we were defining our outcome accurately.
Outcome definition is probably one
of the most important things that you do
when building an AI model.
These downstream specialists, they did all the QA,
quality assurance, quality control
for the inputs of the model,
making sure that the data that was being fed to the model
to learn from was all valid.
And then the third thing was we often run our models
in what we call a silent mode.
And this is when we run it prospectively
without actually exposing the end user to the algorithm,
just to make sure that it works in a production setting.
And our beta testers during the silent mode
were the specialists.
And they took on that labor.
So really, we do everything
and try to minimize anything
that we ask these inundated upstream workers to take on
as we develop the AI solutions.
The next tactic is when you actually go to integrate,
how do you minimize burden for use of the AI tool?
So going back to our pulmonary embolism use case,
we have the ED docs who carry the weight of the world,
and they're being told, "Hey, these low risk patients
with pulmonary embolisms, you can actually send them home."
The challenge here is that the ED docs
are reluctant to send patients home
unless if they know that the patient
is gonna get the care they receive outside of the hospital.
So what did we do?
We built the AI solution in a way
where patients who are identified
as having this low risk pulmonary embolism,
there's a notification sent to a care manager
who schedules the appointment, in clinic, three to five days
after that emergency department encounter.
So literally, it's the notification sent
to schedule the appointment,
and then the ED doc is told,
"Hey, this is a low risk patient.
We've already coordinated things to make sure
that the patient can be safely seen at home,
and this patient can be safely discharged."
So the first two use cases,
I talked about healthcare examples,
which is a big part of my life,
working at the Duke Institute for Health Innovation.
The last two tactics, I'm gonna ground in experience
from a different part of my life as the father
of two young girls.
So these are characters I've gotten to know really well
over the last five, six years.
You can probably imagine with two daughters,
how important "Frozen" is to our family.
So on the right-hand side, you have Elsa.
So Elsa is really kind of one of the main drivers
of the "Frozen" series, and hence the name "Frozen."
It comes from the fact that Elsa has magical powers
to freeze anything.
She can turn anything to ice.
And so she has these magical powers.
She is seen as an outcast in her community
because of these magical powers.
But at her core, she identifies with these powers
and with what it gives her the ability to do.
So she has this tension of having magical powers,
not being really warmly accepted by her community,
and she's a princess.
So she's the oldest daughter in a royal family.
And she comes to terms with the fact
that she really is not eager to take on a leadership role
within her community and within her kingdom.
Thankfully, she has this amazing younger sister, Anna.
Anna has grown up in Elsa's shadow in some ways,
and looks up to her older sister.
She is fascinated with the magical powers
of her older sister, but she understands
that her older sister needs protection
and that she's seen as an outcast.
And the things that her older sister identifies with
are really seen as kind of scary or intimidating by others
within the kingdom.
And Anna is eager to take on a leadership role
in her kingdom and kind of emerges in the series
as the heir to the throne.
So we have Elsa and Annas throughout our own organizations.
And I'll try to map these to the org structure
that Kate went through,
where there are many examples of front-line workers
with magical powers that we are trying to further equip
with tools that we're significantly investing in.
These tools can be intimidating.
They can make folks feel like they have the ability
to do things that they wouldn't be able to do as leaders
in the organization.
And those front-line workers need protection
from managers and organizational leaders
that more fit the character of Anna.
And so this is kind of one of the most tense scenes
in the first "Frozen" movie,
where Elsa ends up leaving the kingdom.
She seeks solace in a large ice kind of castle
that she builds herself.
And many of the villagers, they go out
to try to encourage her to come back
and stop using her power.
And for Elsa, this is really kind of scary.
And she has to kind of fight back with the villagers
to keep her core tasks and her ability to continue
using the powers that she has.
And her sister ends up intervening,
and helping kind of mediate the need for Elsa
to be able to really embrace and use her special powers.
The last tactic is more modeled off of the sequel
in the series, "Frozen II,"
where Elsa and Anna develop
kind of a common framework and model,
defining under which conditions can the magical powers
most advance the interests of the kingdom.
How should the magical powers be used versus not used?
Where should Elsa focus her efforts,
and what should she be prioritizing?
What are the parameters
by which she should be using her powers?
And the second movie kind of really brings
together the two sisters
where you have this highly equipped,
talented front-line out there in the community
using magical powers and a leader in the kingdom
who is protecting and making sure that her sister's powers
are respected within the community.
So I hope that this helps map these different types of roles
and these different types of tactics
to all of our organizations.
And we're gonna kind of review the six tactics.
We bucket them into these predictive AI,
laborious AI, prescriptive AI.
And I hope the examples that we've given you
from other non-healthcare industries, fashion,
hiring, grounded in healthcare examples,
as well as other types of characters that folks on the call
may be familiar with.
So I'm gonna stop sharing my screen
and we're gonna be going to a Q&A.
- Great, thank you so much.
Great presentation.
Glad to have you all back with us.
We'd like to remind our audience
that we welcome your questions.
We've had some come in during the presentation,
but we would love to have more.
So you can submit those using the questions module
on the GoToWebinar control panel.
So just to kick things off.
And I'll address this to maybe whoever wants to grab this.
Are there important differences in how you apply the tactics
for AI solutions that are built internally
versus those that are purchased from an external vendor?
I dunno if you wanna grab that, Mark maybe?
- Yeah, so I'll kick off, and then Suresh,
if there's anything you wanna add.
So I'll talk through one of our examples.
We talked about kidney disease.
And that was an example where we used an AI tool
that was built externally.
And so even within the examples
we've talked about, there's both,
where some of the algorithms we curate our own data for
and then we build those internally.
Other times, there's well-validated external studies
or products on the market that will then integrate.
I would say one piece that is different
when you build the
tool internally, there's a lot more of the labor required
to validate the inputs and validate the outputs.
So we have to really bring together
the clinical specialists,
agree on what the relevant information is,
what's the right way to define the outcome,
adjudicate those outcomes.
Oftentimes, we have multiple comparisons,
so there's like three variations of how we could define it.
Whereas when we bring in an externally developed solution,
a lot of that expert consensus is already built.
And typically, it's a specialist or an expert
within our own setting who's kind of like handing us
a publication saying,
"Hey, this is well-accepted in our profession
as kind of the gold standard for what other sites
are doing in terms of risk prediction.
Can we use this?
So that's the major difference,
is the amount of end user labor going into the validation
of the inputs and outputs.
But the other tactics are relatively consistent
in how we do the workflow integration and everything else.
- Well, and just a follow up on that question.
Is there a difference in the extent to which users along
the process want better understanding
of how the tool is working?
You probably have a bit more visibility into your own.
How does that play out with externally purchased tools?
- So you wanna go, Suresh?
- Yeah, I think that when we start with an external tool,
that is that information asymmetry.
So we need to certainly address that right
from the beginning in terms
of is it the right set of outcome?
What are the limitations?
I think those things have to be captured
in a structured form so that this is incorporated
into the education of that specific tool
that's being developed and validated.
And then, presented both to the end user
and all the other users as well,
other stakeholders will touch upon that tool.
Otherwise, it becomes difficult to engage
and drive adoption and see the returns.
Mark, anything else?
- Yeah, one thing to build off Suresh's comment
is one of the ways that we address the information asymmetry
is by doing the silent trials.
So whether it's internal or external,
every clinician in our setting,
before they use a tool
for their own patient care prospectively,
they want to know, how does this work for my patients?
And so we anticipate that,
and it doesn't matter whether we built the tool
or the tool was built elsewhere.
Before we actually go to folks
and try to get them ready for a rollout,
we try to give them the information saying,
"Hey, by the way, we've already done the analysis.
This is how it works in this setting.
- Right. Okay, great.
Thank you, Mark.
Now, here's a very general advice question,
which I'm gonna kick to Kate
since you worked with a lot of practitioners.
This audience member is a business manager working
with a team of data scientists and engineers.
What advice do you have for a non-technical person
collaborating with AI/ML experts
so that we are all speaking the same language?
And that's a very broad question,
but I think you probably understand the sentiment
that it's coming from and any thoughts you have.
- And so, first of all, this is what's known as a cold call,
guys in business school,
and now, I'm on the receiving end.
So I can see how this feels from the other side.
So is the questioner asking,
they are the person with
the non-technical background?
- Yeah, they are a business manager working with that team
and looking for-
- Okay, so I think that the easiest thing I would say
is this is where boundaries, banners, and brokers
are really important.
And in organizations, product managers often play this role
between sort of like top managers and domain experts
on the one hand, and then the AI developers
on the other hand.
So I guess I would say of course, as a manager,
get yourself up to speed as much as you can.
Learn as much about the technical as you can.
But the reality is that you're not gonna be able to learn
everything you need to know to be really effective
in the detail development.
And that's where people like product managers are helpful.
- Right. Okay, great.
Thank you.
And then another question I'll toss this out
to whoever wants to grab it,
which is how do you measure the impact of these tactics?
How do you measure adoption and contribution
of AI technology?
- So this is interesting because I was recently asked
by someone who's at a research site,
"Tell me what is a good adoption rate?"
And so that just sparked me reaching out
to my networks and asking.
And so what I found interesting in this
is that sometimes, companies can really measure
this very closely at the end user level.
Like they can tell is the end user hovering
over the solution?
How much are they engaging?
They have all these very detailed measurements.
And then at a place like Duke,
they may be measuring this by looking at the impact
more than the very detailed use.
So maybe Suresh or Mark,
you guys could give a quick answer.
Like, how do you measure impact in your setting?
- Yeah.
- I think of- - I'll go.
Go ahead, Mark.
- I think of the phrase like competing for eyeballs.
And I would say that that is a more typical lens
to look at things when you're trying to present
something to somebody, and you're competing for real estate
where they're looking.
And so there's a lot of implementations
that are firing popups or notifications.
We generally try to avoid that type of implementation.
So we more try to structure our products
where somebody is responsible for using a tool
and acting on things that they are presented.
And there should be very little distraction
from their time spent using that tool.
So that's why we typically go to the next step,
and say how do we measure effectiveness of the tool?
Because it is built-in that there's labor involved
in the tool use, and that labor is typically dedicated
for use of the tool.
- Right. Okay.
Well, and then keeping on this theme of impact,
and maybe rolling up to organizational politics a bit,
and Suresh, I think with given your role there,
this might be a good one for you.
Given the enormous investments
that your organization is making,
how do you demonstrate value and demonstrate impact
to organizational leaders to keep these projects going,
keep this work going?
- I will share what we've been doing here,
and how we've be in demonstrating that
in a much more practical way of doing things.
We are an innovation team.
So we have a very clear guiding principles
by which we take on projects.
Every single project, or an idea, or a concept
that we touch upon, certainly the very first aspect is,
a solution is built to show value.
It's built to integrate, integrate in the sense
that most of these AI tools do not generate value
if it does not get integrated into the clinical workflow.
So adoption and continuous use is an important aspect of it.
That's why I've been going through the six items
as to how to really engage and drive adoption
is an important aspect that we discussed today.
And then certainly, we look at ability to scale as well,
doing all this stuff in a responsible fashion.
So when we take on a project right at the outset,
we clearly define the outcomes that we are going after,
along with the right set of measures.
Specific milestones and the risk
and the risk mitigation steps.
And our evaluation pieces fall into four,
typically four different categories.
One is the clinical outcomes that we are looking for.
The second is certainly the process and adoption measures,
efficiency measures, those type of attributes
on a project-specific basis.
Third is cost and economics aspects
of it certainly figure in.
And the fourth one certainly falls into the equity
and how do we really address the health disparities as well?
These are the four different categories that we look at,
but certainly, right through the whole AI adoption piece,
we look at can we reduce the burden or the workload
on the clinicians who are using those set of tools?
Otherwise, adoption just goes away.
So these are the four basic categories that we look at,
and we demonstrate on a project-by-project basis.
Thank you.
- Okay, great. Thank you.
Now I have another question that I think
we can look at from both maybe generally,
and I might ask Kate to give us
her thoughts and then also a little bit of what's Duke doing
in this area.
And that is how important is it to monitor model drift
in these kinds of implementations?
And what are, I think we'd be interested to hear,
from Duke about if you have any procedures
by which front-line users are giving feedback to the team.
But also, I'm interested, Kate, in just your overall sense
of how important that is to do
and maybe if you think front-line users
have a role to play?
- So I guess what I'll say is that we have absolutely seen
that a solution gets put out there by developers,
and you really have no idea who is gonna grab hold
of that solution and wanna use it,
and maybe not understand what they're using it for.
So that when I think of model drift,
like one thing is, is it even the people
that you designed this for who are the ones
that end up using it?
And if not, you need to find out
who is using it, and what are they using it for?
And do they really understand the tool well enough
to be using it accurately.
And then the second thing on the model drift,
when it is being used by the users,
is what can you do to continue to iterate
as you learn, as you see what the end users
are actually doing?
How can you feed that back into the solution?
And I think there's a big myth in AI
that it's always a learning model.
And I've just seen this again and again.
It's not always a learning model.
In fact, in many cases right now,
it is not automatically a learning model,
what you see out there in the wild.
And so what it really is is people using it
and then development team seeing what they're doing and,
and then improving the tool as a result.
So that's what I've seen.
I don't know, Mark and Suresh,
if you wanna say how you work with model drift at Duke?
- So I wanna go off of Kate,
where I almost view drift,
you can imagine, like on one hand,
you have the drift of the technology infrastructure
that the AI is plugged into.
On the other hand, you can have drift in the process
and people and workflows
that are putting the tool into practice.
So when it comes to the technology,
and we see changes all the time.
There's new ways to measure labs.
There's new medications that are given.
There's new monitors that are purchased.
Like our small innovation team doesn't control
the supply chain of Duke Health.
So we have to be very proactive about monitoring changes
in data inputs and representation and updating
on, typically, a bi-annual basis,
the way that we map our model to the data input sources.
On the other hand, just to give some examples of process
and workflow shift.
One example is where you're seeing successful adoption
in one setting.
And going to Kate's point, somebody starts trying to use
the tool for an adjacent use case
where it may or may not be appropriate.
Another example is a lot of our tools,
one of the follow-up actions is some communication
between different types of specialists.
And there's instances where we launch a tool
with one expectation around communication,
but then that deteriorates over time.
And instead of calling people,
people are just sending asynchronous texts.
And so we have to monitor, okay,
are the downstream actions still being conducted
with the same rigor?
And how do we continue to train people,
continue to communicate why the structure's important?
All of those things.
- Got it. Thank you.
And let's see, I'm not sure if this next question
is relevant to you guys or not,
but I will toss it out there.
You may have opinions, if not experience.
"Can you comment on the pros and cons
of using synthetic data for prototyping
and accelerating model development?"
- Okay, guys, so I'm a qualitative researcher.
No way am I touching this one.
I'd go right to my Duke tech people.
- Do you wanna start Suresh, or do you want me to?
- Please.
- Okay, so I would say where we use synthetic data
or de-identified data,
'cause you can say there's a spectrum of the proprietary
or confidential nature of data.
So whenever you're getting to the point of needing
to validate something for operational use,
you have to be using
the identified proprietary, confidential data.
But you can gradually progress across that spectrum.
So environments in which we are very comfortable
using synthetic or completely
de-identified data is training.
So when we're bringing new people into the organization,
trying to help teach them our process, our workflows,
how to work with data, synthetic data can be perfectly fine.
The other is just testing of the technology.
So when we take tools that we've built internally,
and we're trying to validate them in new environments,
we can install them in a new environment,
and then run it on synthetic or test data.
That is it's kind of like a canned problem set
where we know the inputs, we know the outputs,
we're just making sure that it functions.
But I would say that the closer you get to needing
to validate the clinical utility,
the more you need to start
getting into confidential proprietary datasets.
- Got it. Okay. Thank you.
- (indistinct) Use for synthetic data is development
of workforce, in terms of training and educating,
we have found significant value add in those
because that's an important aspect of that.
- Great, and that was a perfect transition
because the next question that I had teed up
was in fact about training and educating users.
So here it is.
We've heard that it's important for front-line users
to understand the nature of AI decision support output.
That it's a prediction with a certain level of confidence,
not a so-called right answer,
which people tend to, maybe who don't know a lot about AI,
think it's like spitting out the right answer.
How important do you think that kind of understanding
is for the sort of decision support tools
that we have been talking about in this webinar?
So maybe I'll, that that's more broadly.
I'd like to, maybe Kate can answer that.
And I can.
- Yeah. Why don't I start?
And then I'll hand it off to you guys for specific examples
of what you do at Duke around training.
So I think this falls into the bigger category
of AI explainability, and what is it that the end user
really needs to understand.
And oftentimes, what happens with AI outputs
is there's a mismatch between what the AI is saying
and what the user knows from their own experience.
And in fact, that's why we're building these solutions
in the first place.
But that can make it very difficult for the end user
to trust the AI recommendation.
So one thing I would say is, I think it does depend
on what domain you're in.
So in healthcare, it's different than, for example,
I have a project with some colleagues from Harvard
on fashion allocation.
So there, we're not so worried
if the end user doesn't understand.
They can just go with it, fine,
that's not gonna affect anyone's life chances.
In healthcare, it's a different situation,
so you need a different level of explainability.
One thing that I've seen that's really interesting,
some work at MIT is during training,
giving the end users some simulation
so they can see where are the places that the AI solution
is very accurate and where is it less accurate?
So that as end users, they can get a feel
for where they should be overruling the tool.
But with that, Mark and Suresh,
maybe you could talk about what you guys
do at Duke around this?
- Yeah, please.
- So I can start.
On a similar spectrum.
So Kate, I'm from Northern California,
have a lot of friends who work in big tech,
and there are industries where most algorithm development
is done on embeddings of the raw data,
where nobody on the engineering team
can tell you what any one individual feature even is
because it's some mapping to many different transformations
of many different raw data elements.
And that was completely foreign to me.
So even talking about the explainability or interpretability
of a model input, I would say that, for us,
is almost non-negotiable,
where we have to be able to tell clinicians
what is used in the algorithm.
What are the discreet data elements?
Here's a list of them.
Here's what their distributions look like in the population
that the data was trained on.
So that's one piece.
The other piece, and we're actually gonna be working
with Kate on this in the upcoming year,
is doing extensive interviews
and prototyping of documentation for different types
of stakeholders involved in the adoption process.
So an end user may want to be able to, per se,
double-click and see additional information
about the algorithm, what the indicated use is,
what the population demographics are for validation
of the tool, whereas a business unit leader
who's making the decision,
"Do I move forward with adoption or not?"
they may need much more extensive validation studies.
And the other thing too is just professionally,
there's norms in healthcare for how to validate
and disseminate literature related to new innovations.
So I think some of this has to map to the norms
within your own industry for how you build credibility
in a tool that's gonna be adopted by an organization.
- Got it. Thank you.
Well, we have just a few minutes left,
so I wanted to give you a chance for some parting thoughts.
Kate, do you wanna put a cap on this for us?
- Yeah. (laughs)
Okay, I'll try and tie this up.
I think the reason why
we're so excited about doing this research
is AI is such an important space,
and it's so important that you're all working in this area
because it has huge opportunity to impact the world
in a positive way.
The reality is, right now, the performance
is not matching where we want it to be.
And so we all need to be learning from one another,
taking on new ideas, iterating.
Developers, for you guys,
I'm sure it feels like every day,
you feel like you solve something.
The next day, you wake up
and you get hit by a ton of bricks
and you have to start over.
So like, try, fail, dust yourselves off, iterate.
C-suite leaders, do what you can to support
the development teams, remove any roadblocks you can.
Researchers, share your findings.
Funders, keep funding.
But as we do all that,
I think what's really important is not to forget
about the people on the front lines
because they're the ones
that are going to bring these AI solutions
into the real world.
Thank you.
- Absolutely. Thank you, Kate.
Well, that was great.
Thank you, Kate, Mark, and Suresh for sharing your insights.
This has been been a wonderful
and really informative hour for us.
And I also wanted to thank Five9, our sponsors today.
For our audience, thank you very much for joining us today,
and hope you'll join us again for another SMR webinar.
Browse More Related Video
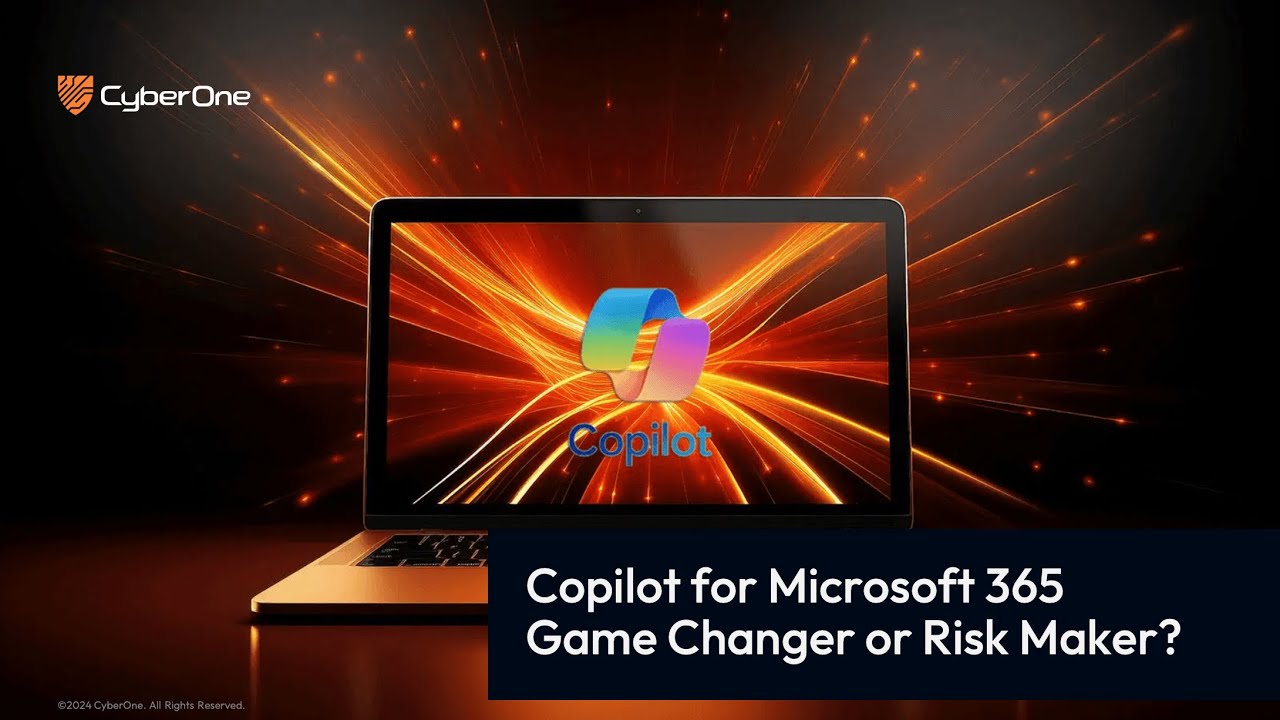
Copilot for Microsoft 365 β Game Changer or Risk Maker?
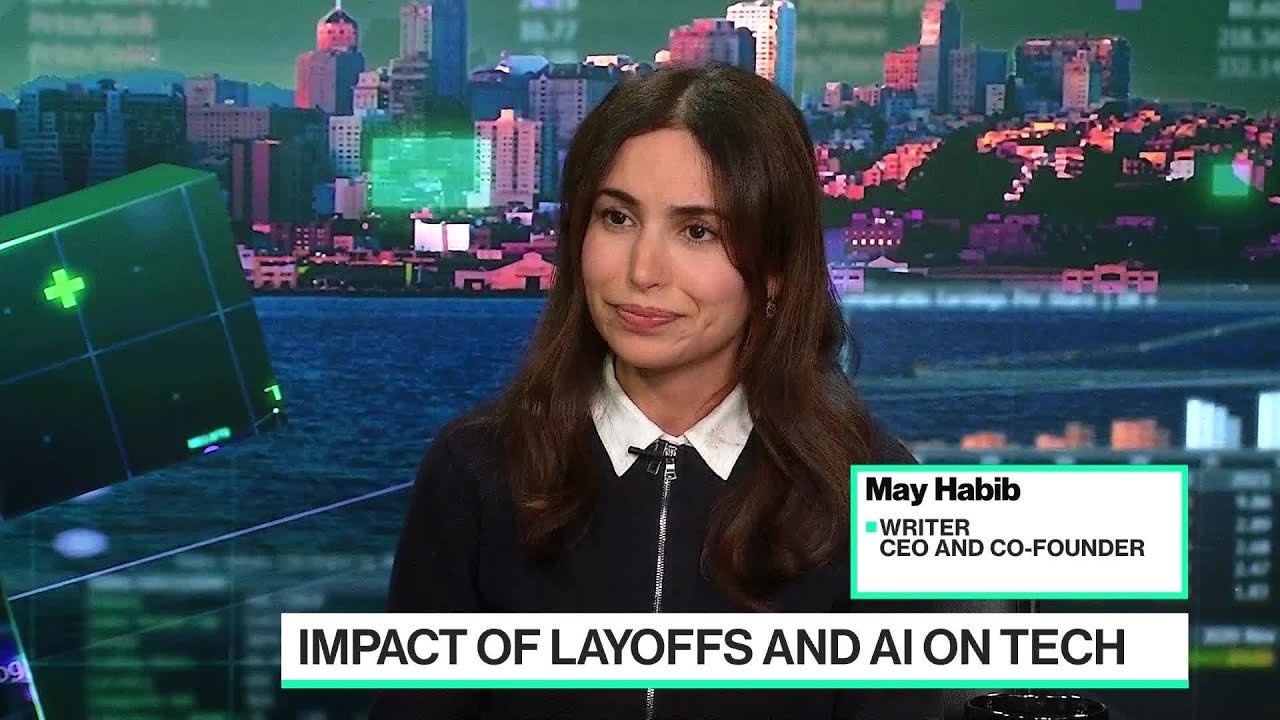
Writer CEO: Bringing AI and People Together
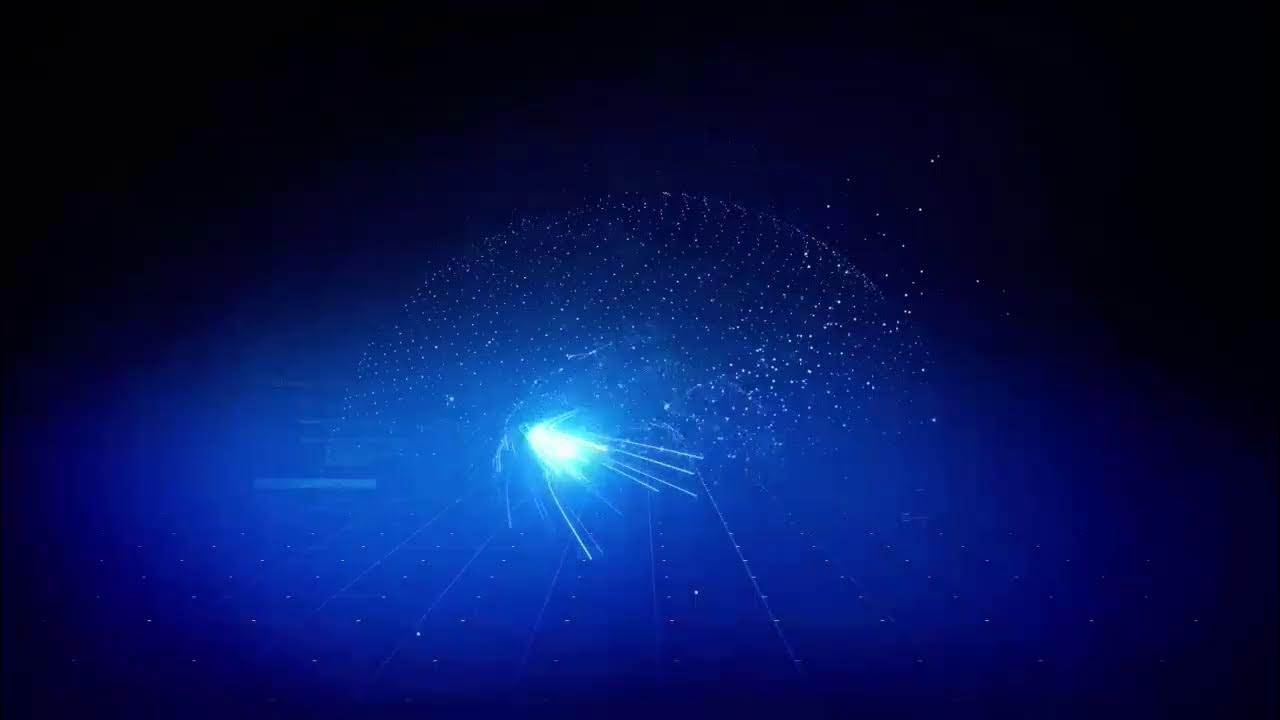
SOCIETAS DEL 12 APRILE 2024
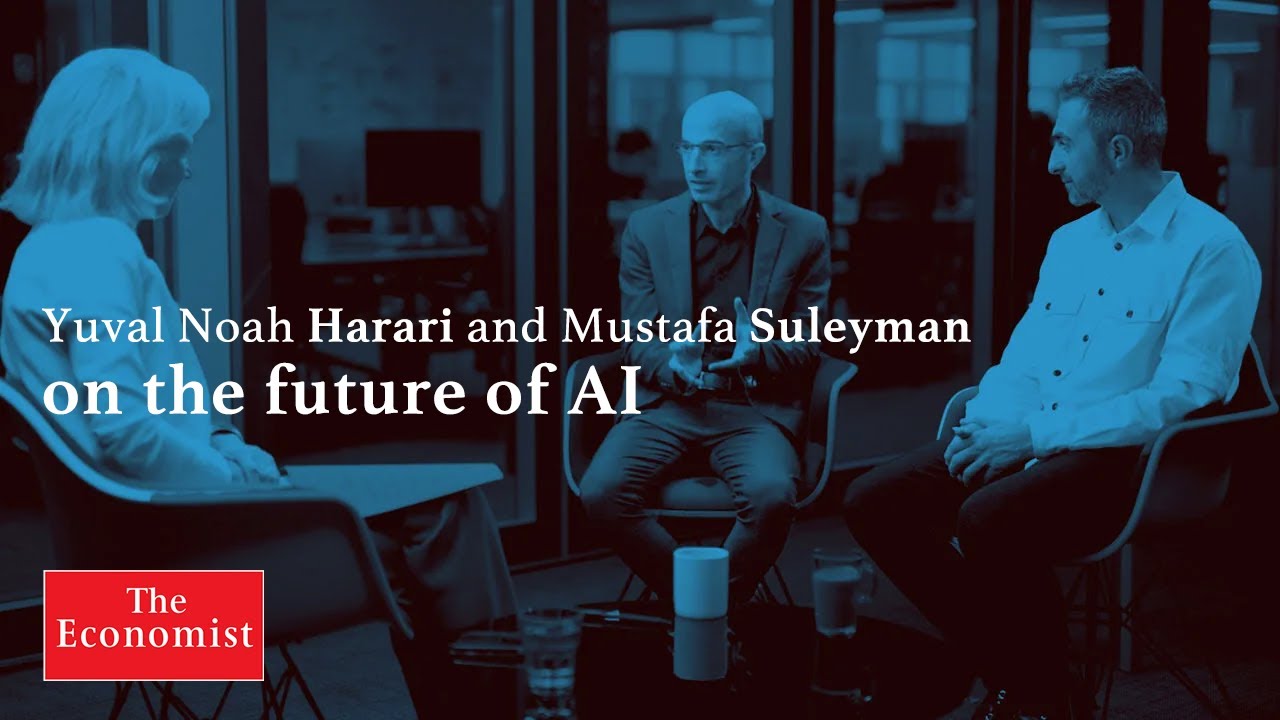
Mustafa Suleyman & Yuval Noah Harari -FULL DEBATE- What does the AI revolution mean for our future?
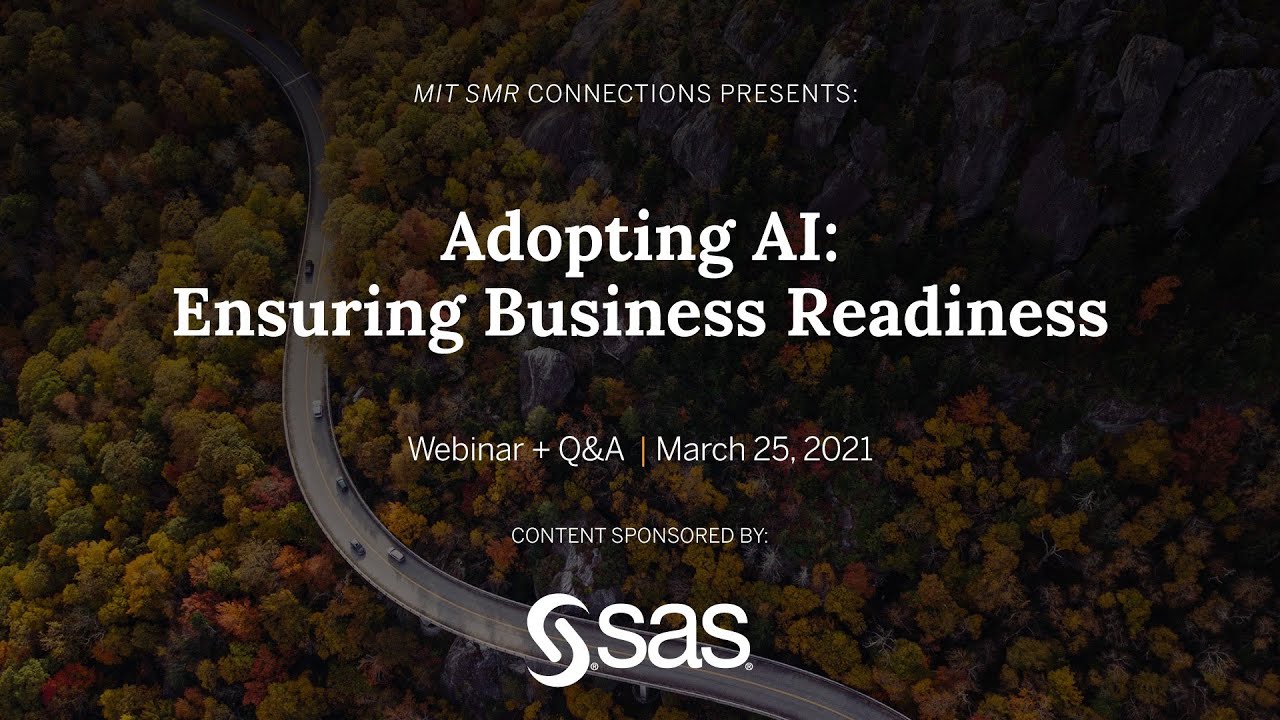
Adopting AI: Ensuring Business Readiness

MENGAPA PARA PAKAR AI MULAI KETAKUTAN DENGAN AI??
5.0 / 5 (0 votes)