What is Symbolic Artificial Intelligence? Prediction: ChatGPT + Symbolic AI = Mind Blowing
Summary
TLDRThis video provides an overview of symbolic artificial intelligence (AI), a subfield of AI focused on using symbols, logic, and formal reasoning to represent and manipulate knowledge. It covers the history of symbolic AI, beginning in the 1950s with rule-based systems for tasks like chess and problem-solving. Key applications include expert systems, which mimic human decision-making, and natural language processing systems, used for tasks such as translation. Though the AI field has shifted towards data-driven approaches, symbolic AI remains vital in areas like knowledge representation and reasoning.
Takeaways
- 🤖 Symbolic AI is a subfield of artificial intelligence that focuses on using symbols, logic, and formal reasoning to represent and manipulate knowledge.
- 🧠 Symbolic AI involves creating explicit rule-based systems for tasks like problem-solving and decision-making.
- 📅 The history of symbolic AI dates back to the 1950s, with early research aimed at mimicking human intelligence through rule-based systems.
- ♟️ Early symbolic AI systems were designed to perform tasks such as playing chess and solving mathematical problems.
- 🔢 Symbolic AI uses a symbolic representation of knowledge, where information is represented as symbols (e.g., words or numbers) and manipulated using logical rules.
- 📊 Symbolic AI contrasts with data-driven AI approaches, such as machine learning, which use statistical algorithms to learn from examples.
- 👨⚕️ Expert systems are a notable example of symbolic AI, designed to mimic the decision-making abilities of human experts in specific domains.
- 🗣️ Another example of symbolic AI is natural language processing systems, which use formal grammars to understand and generate human language.
- 🔄 Despite the rise of data-driven AI approaches, symbolic AI continues to play an important role in areas like knowledge representation and reasoning.
- 🎯 Symbolic AI has led to practical applications such as expert systems for diagnostics and natural language processing tools for language translation.
Q & A
What is symbolic AI?
-Symbolic AI is a subfield of artificial intelligence that focuses on using symbols, logic, and formal reasoning to represent and manipulate knowledge. It involves creating rule-based systems that can perform tasks like problem solving and decision making.
How does symbolic AI represent knowledge?
-Symbolic AI represents knowledge using symbols, such as words or numbers, and applies logical rules to manipulate and reason about this information.
What distinguishes symbolic AI from machine learning?
-Symbolic AI uses explicit rules and logical reasoning to process knowledge, whereas machine learning relies on data and statistical algorithms to learn from examples.
When did symbolic AI first emerge?
-The history of symbolic AI can be traced back to the 1950s when researchers began exploring the idea of creating machines that could mimic human intelligence.
What were early applications of symbolic AI?
-Early efforts in symbolic AI focused on creating rule-based systems that could perform tasks like playing chess and solving mathematical problems.
What are expert systems in symbolic AI?
-Expert systems are a well-known example of symbolic AI. These are computer programs designed to mimic the decision-making abilities of a human expert in a specific domain, such as diagnosing medical conditions.
How is symbolic AI used in natural language processing?
-In natural language processing (NLP), symbolic AI uses formal grammars to understand and generate human language, aiding in tasks such as language translation and text summarization.
What is the difference between symbolic AI and modern data-driven AI approaches?
-Modern data-driven approaches like machine learning focus on analyzing large datasets and deriving patterns, while symbolic AI focuses on logic, rules, and symbolic manipulation of knowledge.
Why is symbolic AI still important despite the rise of machine learning?
-Symbolic AI remains important in areas like knowledge representation and reasoning, where formal logic and rules are essential to making accurate decisions.
What are some practical applications of symbolic AI?
-Some practical applications of symbolic AI include expert systems for decision-making, as well as natural language processing systems used for tasks like translation and text summarization.
Outlines
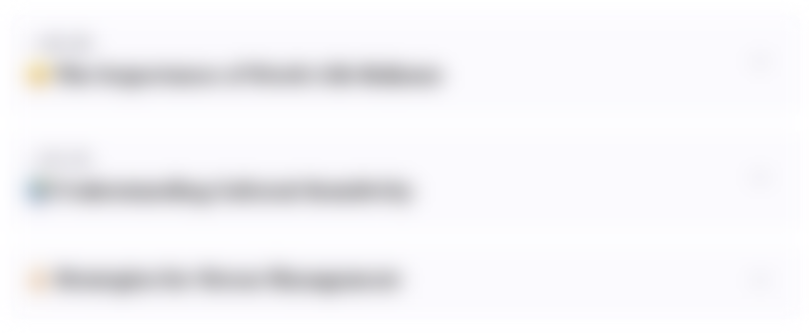
This section is available to paid users only. Please upgrade to access this part.
Upgrade NowMindmap
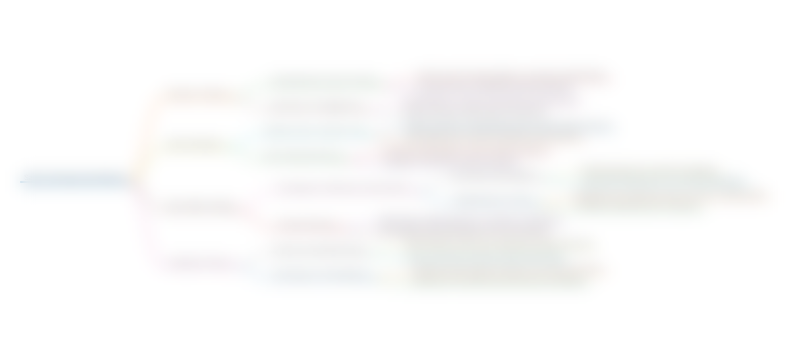
This section is available to paid users only. Please upgrade to access this part.
Upgrade NowKeywords
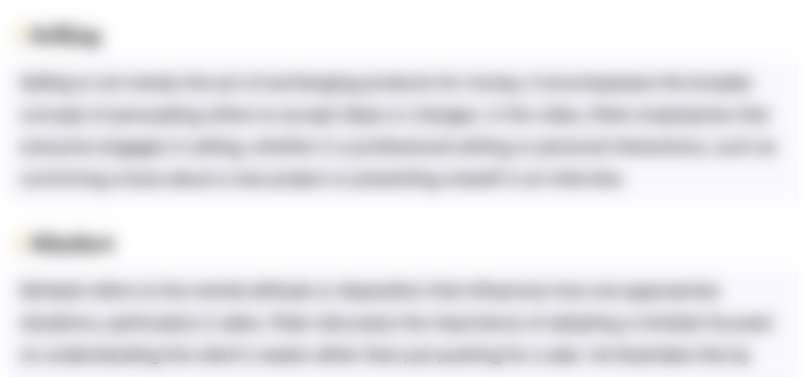
This section is available to paid users only. Please upgrade to access this part.
Upgrade NowHighlights
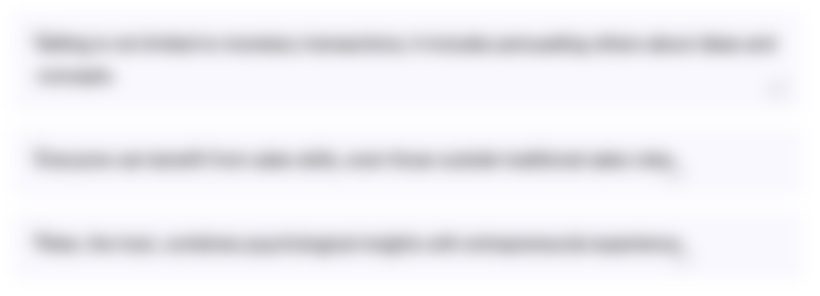
This section is available to paid users only. Please upgrade to access this part.
Upgrade NowTranscripts
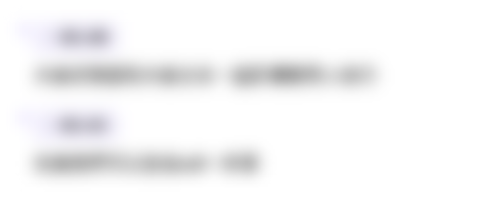
This section is available to paid users only. Please upgrade to access this part.
Upgrade NowBrowse More Related Video
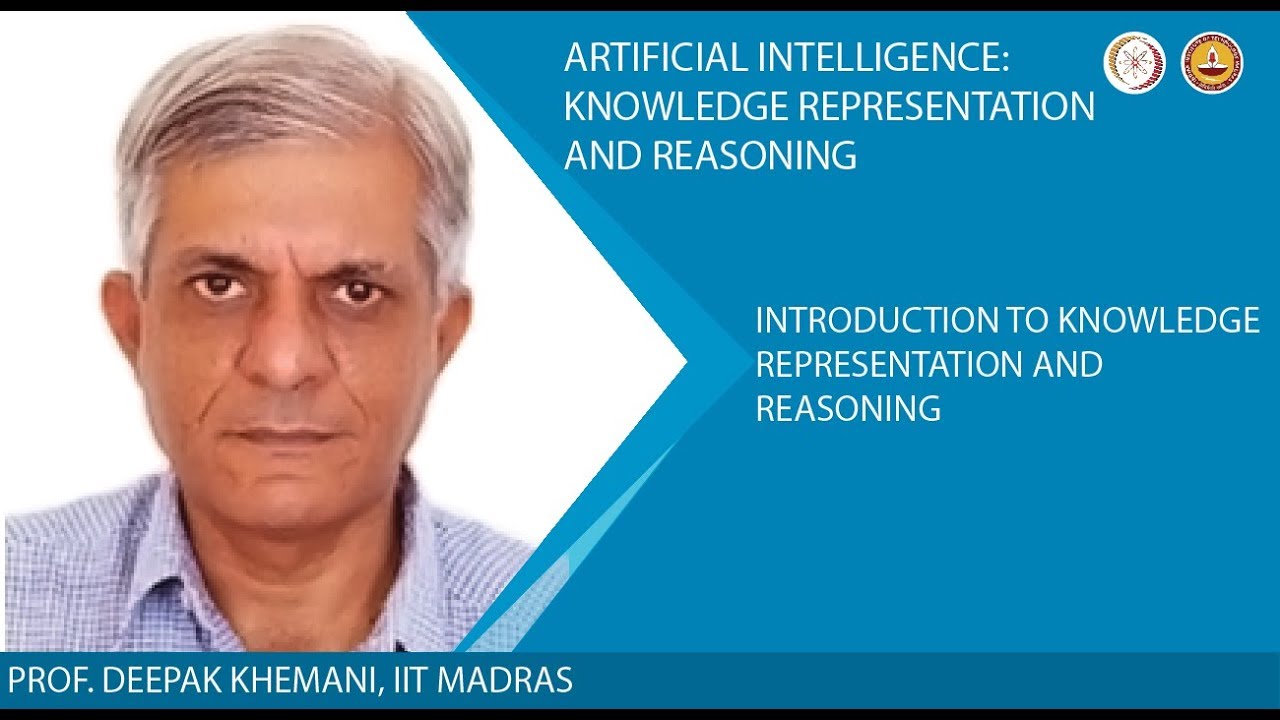
Introduction to Knowledge Representation and Reasoning
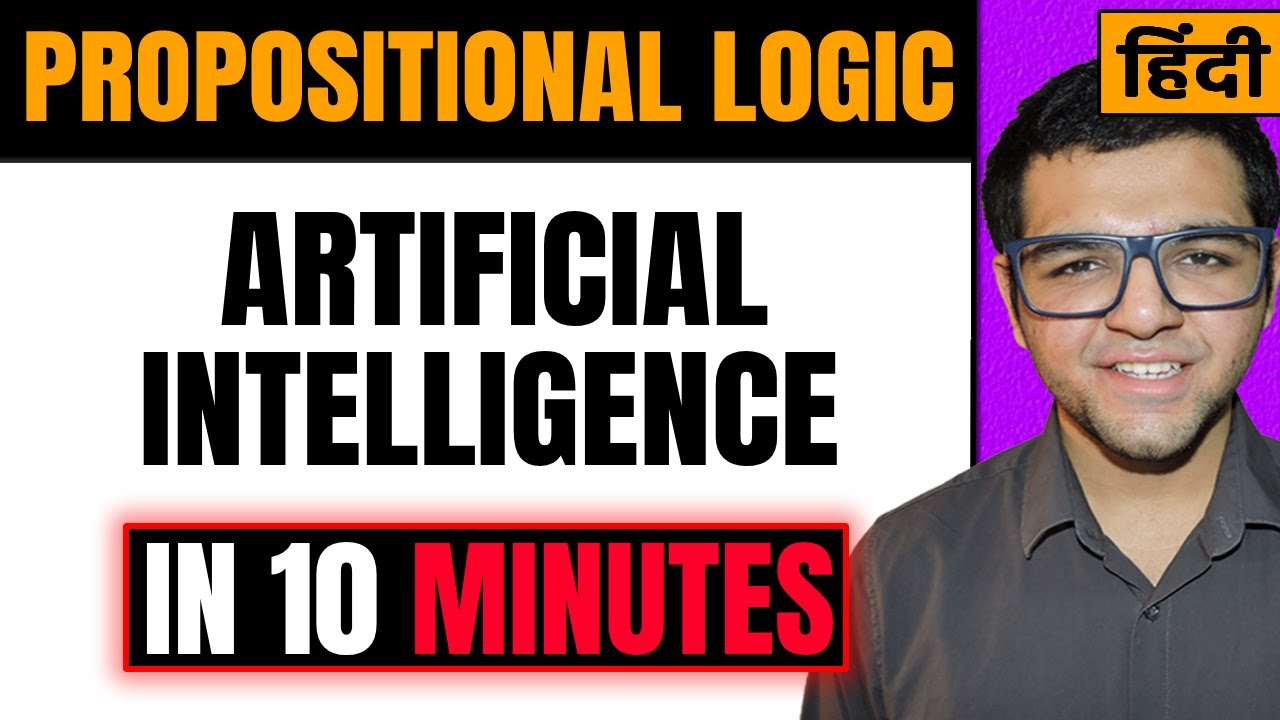
Propositional Logic in Artificial Intelligence in Hindi | Knowledge Representation

REPRESENTASI PENGETAHUAN KECERDASAN BUATAN | Logika | Prosedural | Semantic Network | Frame
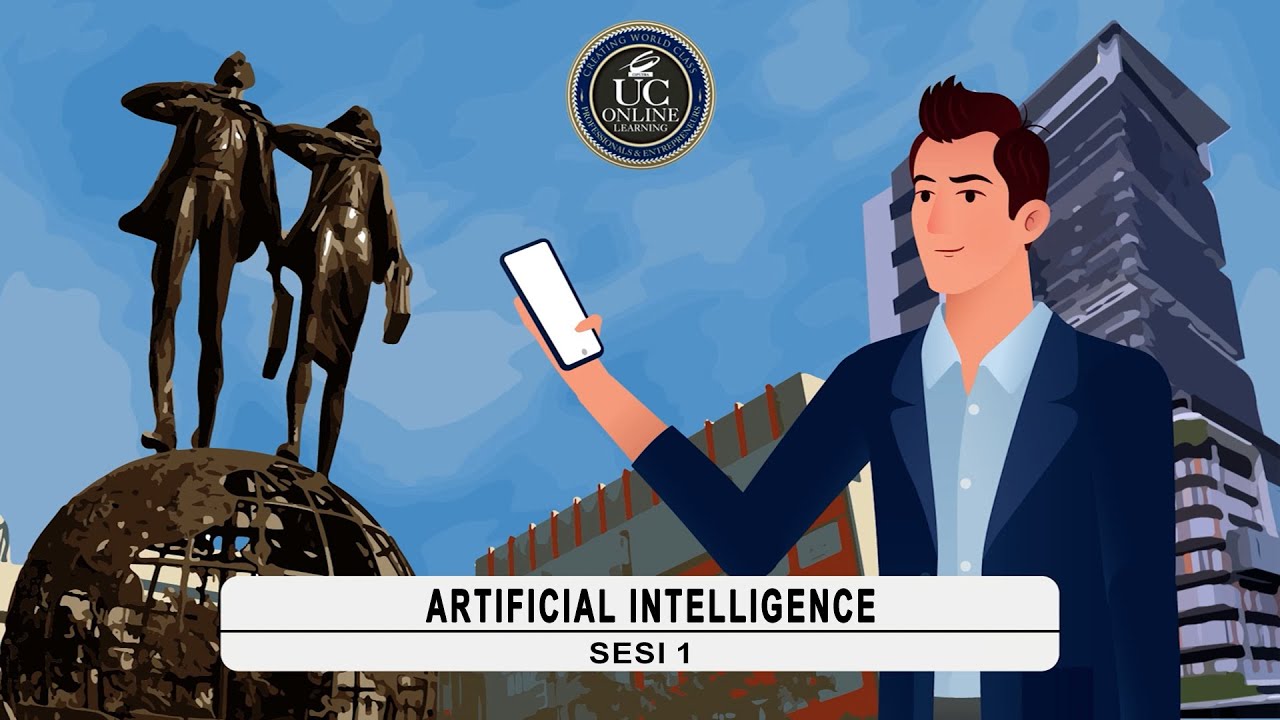
IMT - AI (1) - Apa itu AI?, Sejarah, dan Penerapan AI
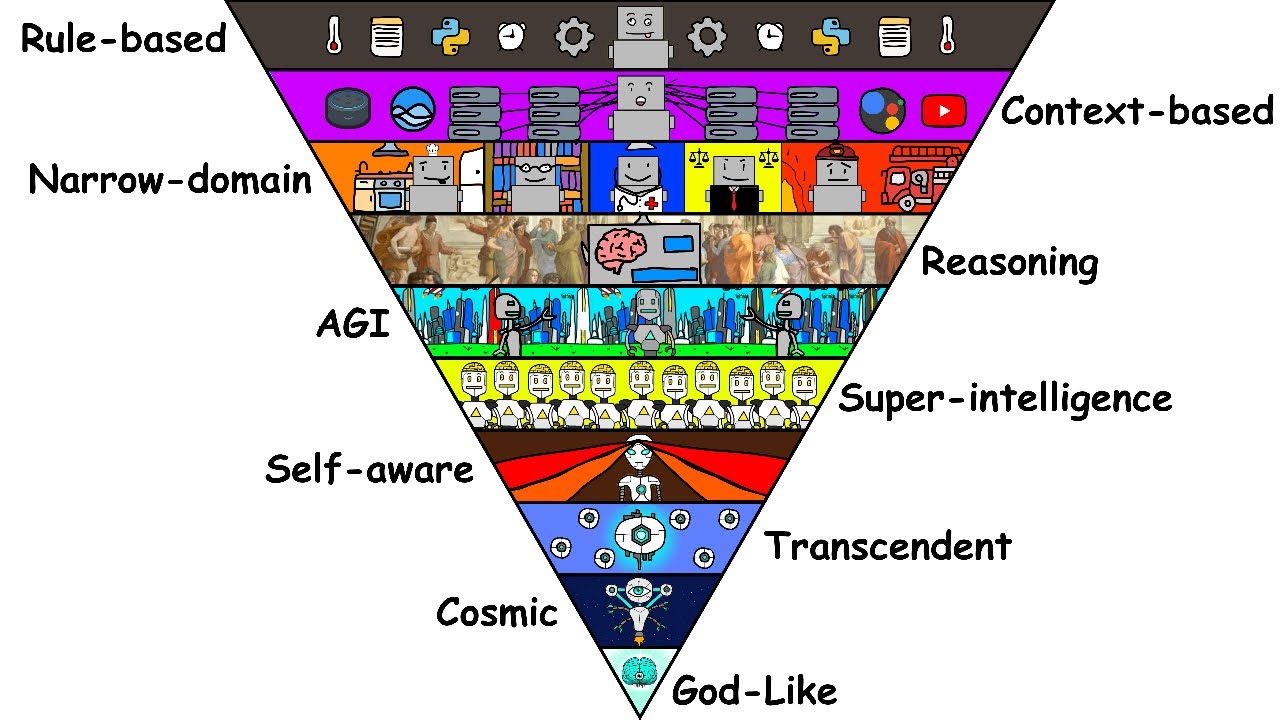
The 10 Stages of AI Explained in 10 Minutes

Introduction
5.0 / 5 (0 votes)