Artificial Intelligence in Gastroenterology (AI for GI)
Summary
TLDRDr. Brennan Spiegel, a gastroenterologist at Cedars-Sinai, discusses the impact of artificial intelligence (AI) in gastroenterology. He explores how AI, particularly natural language processing (NLP) and image recognition, is transforming diagnosis and treatment in the field. Topics include automated polyp detection in colonoscopies, AI-assisted diagnosis of conditions like Barrett's esophagus and ulcerative colitis, and the potential for personalized medicine. Spiegel also touches on the limitations of AI, emphasizing the importance of human judgment and the collaboration between machines and doctors for better patient outcomes.
Takeaways
- 🤖 AI in gastroenterology is rapidly advancing, focusing on how human intelligence and AI can complement each other, termed as 'augmented intelligence'.
- 📚 Artificial Intelligence (AI) includes components like machine learning, natural language processing (NLP), and image recognition, which are relevant to medical applications like gastroenterology.
- 💡 AI systems are being developed to assist doctors by automating tasks such as polyp detection in colonoscopies, improving accuracy and efficiency in diagnosis.
- 🏥 AI can augment doctors' abilities rather than replace them, allowing more time for patient consultation, decision-making, and empathy, as discussed in the book 'Deep Medicine'.
- 🔬 AI has proven useful in areas like image analysis in gastroenterology, where it can accurately identify cancerous or precancerous conditions like Barrett’s esophagus and gastric cancer.
- 📈 In clinical trials, AI-assisted reviews increased the diagnosis rate of high-grade dysplasia and cancer by over 14%, showing the potential impact on patient outcomes.
- 👁️ AI systems in colonoscopy can increase the adenoma detection rate, improving overall outcomes in screening and diagnosis of colorectal conditions.
- 🔍 AI's application in capsule endoscopy helps detect ulcers and erosions with high accuracy, reducing the burden on clinicians sifting through large image sets.
- 🧪 AI can analyze blood work (CBC) to predict colon cancer risk, offering a non-invasive way to screen for potential issues before using more invasive procedures like colonoscopies.
- 🧠 The adoption of AI in healthcare is in its early stages, and its future success will depend on more prospective validation studies, cost-effectiveness evaluations, and integration into daily clinical workflows.
Q & A
What is the main focus of Dr. Brennan Spiegel's presentation?
-The main focus of Dr. Brennan Spiegel's presentation is the exciting and rapidly evolving role of artificial intelligence (AI) in gastroenterology, with an emphasis on how AI can augment human abilities in clinical settings.
What is the difference between artificial intelligence (AI) and augmented intelligence as described by Dr. Spiegel?
-According to Dr. Spiegel, artificial intelligence (AI) refers to computer systems simulating human behavior, while augmented intelligence emphasizes the collaboration between human intelligence and machines to enhance natural human capabilities.
What are some components of AI that Dr. Spiegel highlights in the presentation?
-Dr. Spiegel highlights several components of AI, including machine learning, natural language processing (NLP), and vision systems, with a focus on how they apply to gastroenterology.
How has AI shown its capabilities in gastroenterology according to the presentation?
-AI has demonstrated its capabilities in gastroenterology through natural language processing (NLP) for diagnostic support, machine learning for risk prediction, and vision systems for detecting abnormalities such as polyps during colonoscopy.
What is an example of AI being used in real-time during colonoscopy as mentioned by Dr. Spiegel?
-An example is the use of AI to detect polyps in real-time during colonoscopy by placing boxes around potential polyps, improving adenoma detection rates and assisting gastroenterologists in identifying abnormalities that might otherwise be missed.
What concerns are raised regarding the use of AI in gastroenterology?
-Dr. Spiegel mentions concerns such as selection bias in the images used for training AI models, the varying quality of real-world images, and the need for prospective validation studies to ensure the efficacy and reliability of AI tools in clinical practice.
How has AI improved the detection of gastric cancer and Barrett's esophagus?
-AI has shown promise in detecting early gastric cancer and Barrett's esophagus through convolutional neural networks that analyze endoscopic images, achieving high accuracy in identifying dysplasia and cancerous changes with strong area under the curve (AUC) scores.
What potential role does AI have in diagnosing conditions like ulcerative colitis and IBS?
-AI can aid in diagnosing ulcerative colitis by distinguishing active from healing inflammation using endocytoscope images, and it may also be able to identify imperceptible abnormalities in IBS, potentially leading to faster and more accurate diagnosis.
What is Dr. Spiegel's perspective on using AI in routine gastroenterology practice?
-Dr. Spiegel believes AI has the potential to significantly enhance gastroenterology by assisting with polyp detection, reducing radiation exposure, and identifying microscopic abnormalities. However, he stresses that more research and real-world validation are necessary before widespread adoption.
What are some future challenges for AI in gastroenterology, as discussed by Dr. Spiegel?
-Future challenges include conducting more prospective validation studies, proving the impact of AI on patient outcomes, assessing cost-effectiveness, and figuring out how to integrate AI tools into routine workflow without disrupting the clinical process.
Outlines
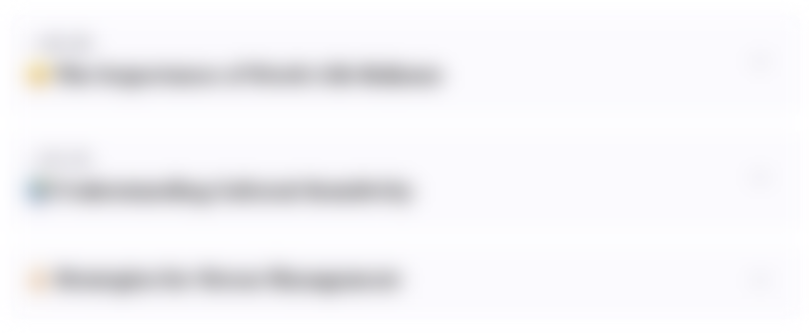
This section is available to paid users only. Please upgrade to access this part.
Upgrade NowMindmap
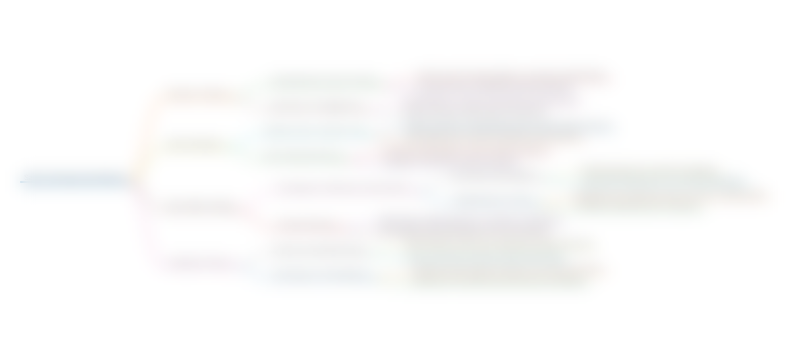
This section is available to paid users only. Please upgrade to access this part.
Upgrade NowKeywords
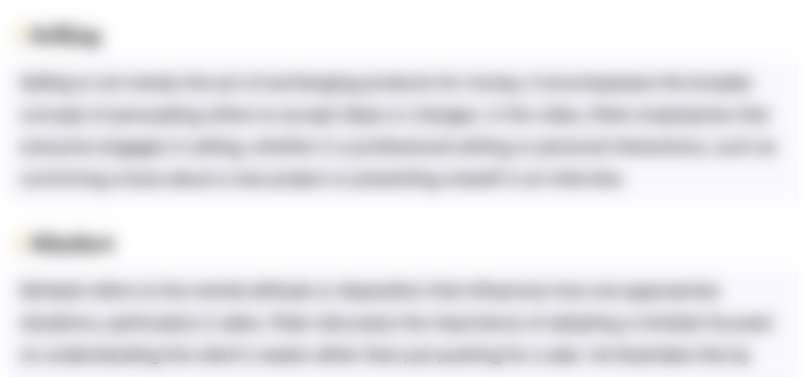
This section is available to paid users only. Please upgrade to access this part.
Upgrade NowHighlights
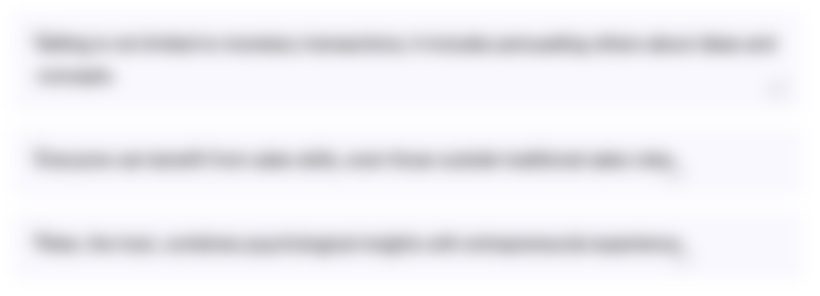
This section is available to paid users only. Please upgrade to access this part.
Upgrade NowTranscripts
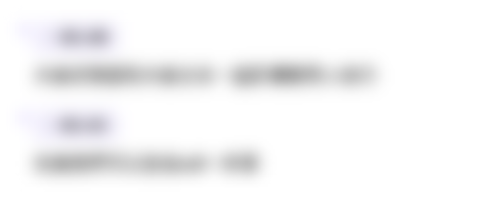
This section is available to paid users only. Please upgrade to access this part.
Upgrade NowBrowse More Related Video
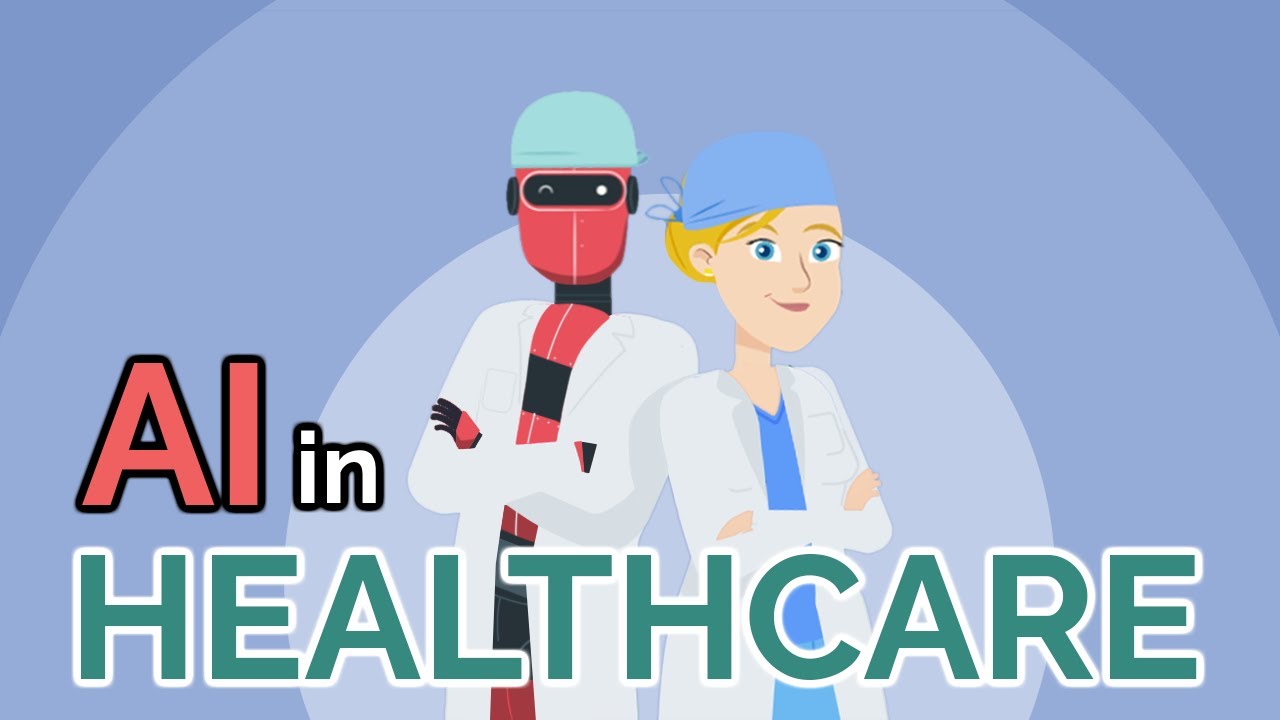
4 Ways Artificial Intelligence is Transforming Healthcare
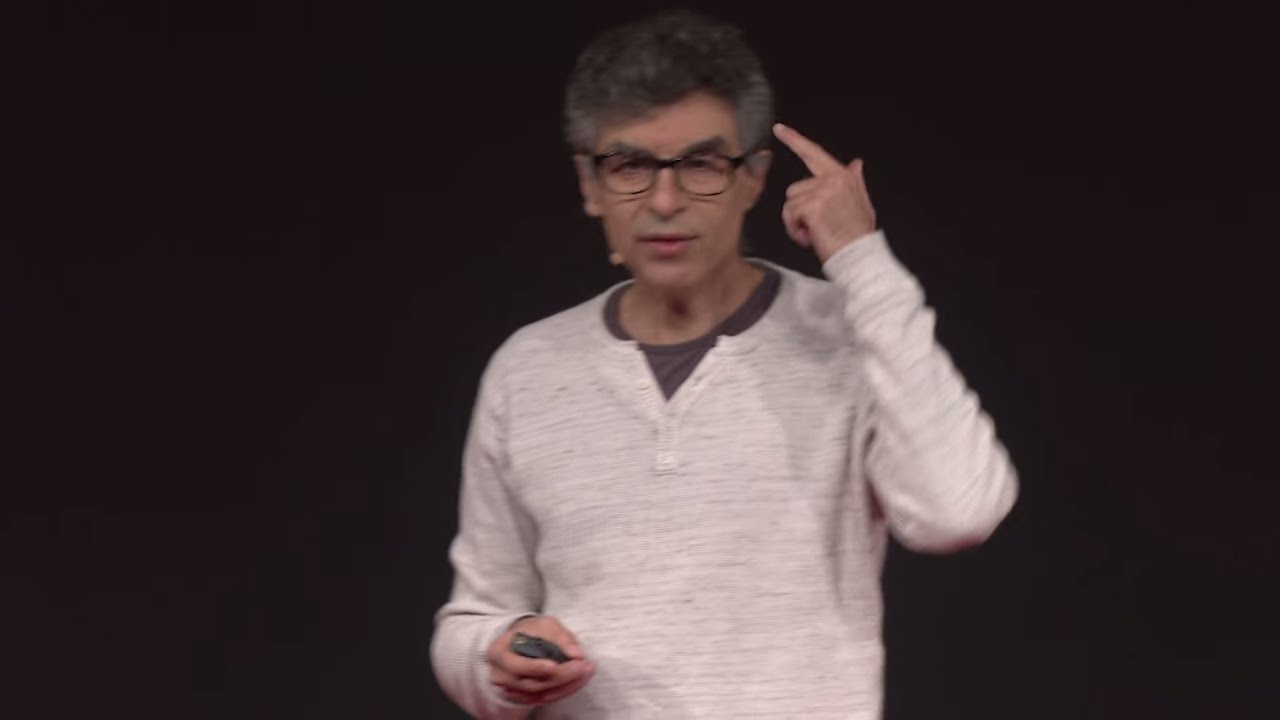
The Rise of Artificial Intelligence through Deep Learning | Yoshua Bengio | TEDxMontreal

Barbara Gallavotti | Che cosa pensa l'Intelligenza artificiale
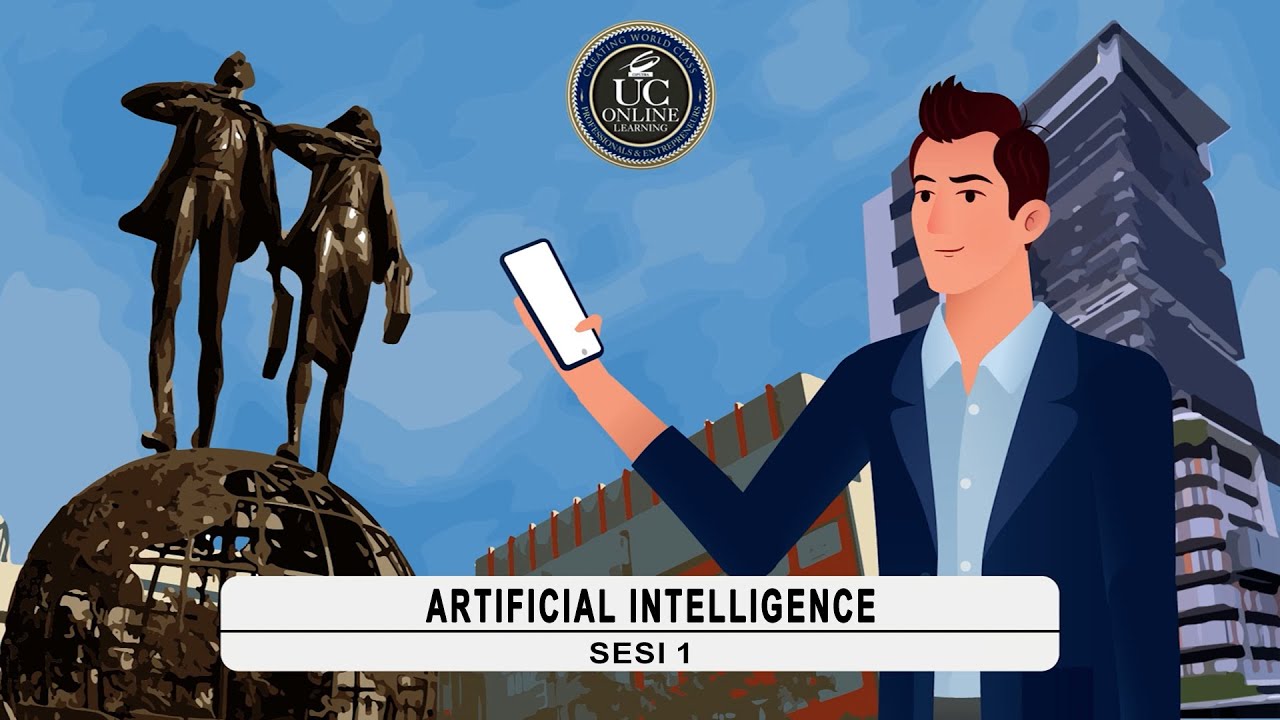
IMT - AI (1) - Apa itu AI?, Sejarah, dan Penerapan AI

Smart Nation 2.0: Professor Joseph Sung on artificial intelligence in science
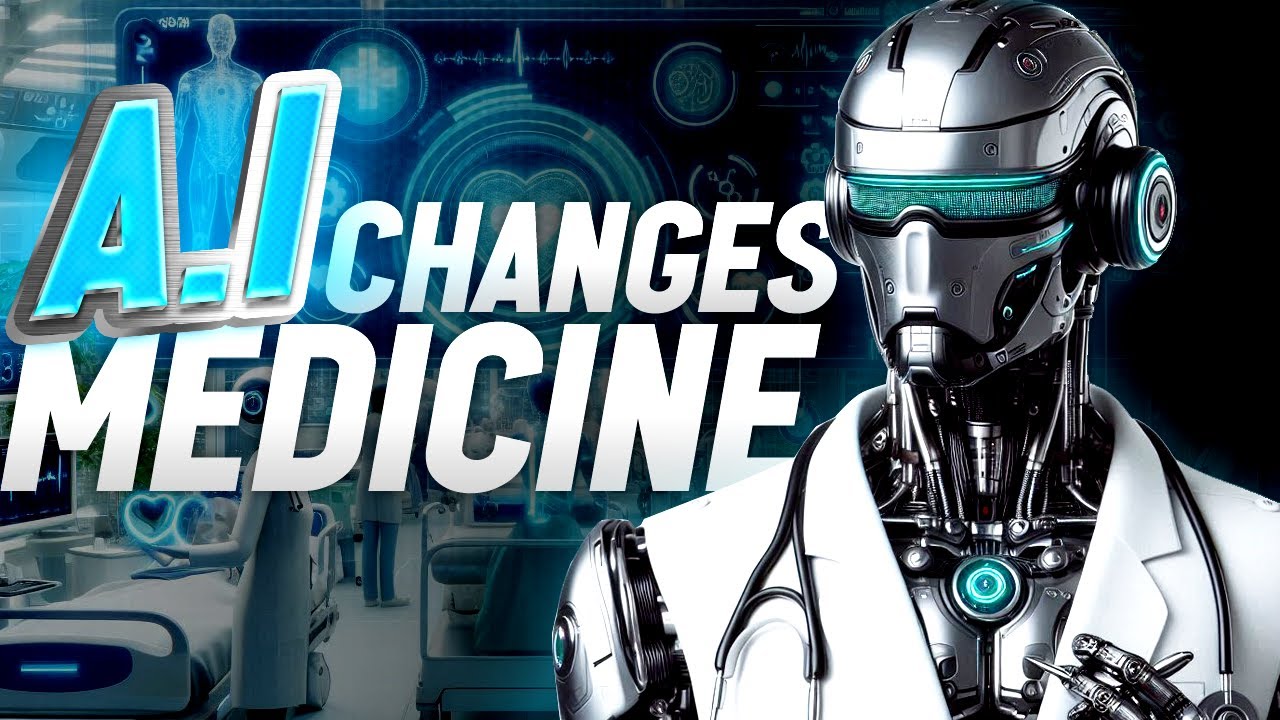
China's AI Hospitals Are More Advanced Than You Think
5.0 / 5 (0 votes)