Optics in AI Clusters - Meta Perspective
Summary
TLDRIn this presentation, the speaker discusses the growing challenges and innovations in AI clusters and optics from Meta's perspective. They highlight the rapid increase in AI model sizes, the demand for more compute power, and the growing gap between hardware capabilities and model requirements. The talk emphasizes the need for flexibility in AI cluster design and more efficient hardware to handle the immense data and computational demands. The importance of innovation across the entire stack, including software, memory architectures, and IO, is stressed, with a focus on meeting future scalability needs for artificial general intelligence (AGI).
Takeaways
- 😀 The growth of AI models is continuing at an accelerating pace, with larger models leading to better results and requiring significantly more compute power.
- 😀 AI and machine learning (AIML) model sizes are increasing at a faster rate than hardware capabilities, creating a gap between industry needs and hardware capacity.
- 😀 Larger models demand more parameters and data, which results in a need for more compute, higher bandwidth, and efficient hardware and optical infrastructure.
- 😀 The requirement for larger AI models is driving the need for innovation across the entire technology stack, including hardware, software, and optical components.
- 😀 PCI over Optics is evolving, but it is still not increasing quickly enough to meet the demands of AI model scaling.
- 😀 Increasing AI model sizes and compute demands push the need for higher data throughput across clusters, driving the need for faster interconnects like Infiniband, NVLink, and optical connections.
- 😀 The training of large language models (LLMs) is just one portion of the AI stack; inference, query consumption, and response generation also require significant computational resources.
- 😀 AI cluster designs need flexibility to accommodate diverse workloads across the training and inference phases, ensuring scalability and efficiency.
- 😀 Meta's design for large AI clusters, which involve thousands of GPUs, pushes the limits of bandwidth, requiring innovations in network designs, especially around IO fabric and interconnects.
- 😀 Future AI models will need more memory (HBM stacks) and faster GPU-to-GPU, GPU-to-CPU, and GPU-to-HBM connections to meet growing demand, with the latest announcements predicting 16 terabytes per second of memory bandwidth per accelerator.
- 😀 Meta is committed to the open community and innovation in AI hardware and infrastructure, collaborating through the Open Compute Project (OCP) to build the next-generation clusters and address the rapid advancements in AI capabilities.
Q & A
What is the main topic of the presentation?
-The main topic of the presentation is about the growth of AI models, their impact on optics and IO requirements, and how the hardware industry needs to innovate to keep up with these demands, focusing on AI clusters and the evolving need for more compute power.
Why is the growth of AI model size important for the optical industry?
-As AI models grow larger, they require significantly more computational resources, especially in terms of IO bandwidth and hardware innovation. This puts pressure on the optical industry to meet the increasing demand for faster, more efficient interconnects and data transfer capabilities.
How fast are AI models growing compared to hardware capabilities?
-AI models are growing at a much faster rate than hardware capabilities. AI models are doubling in size every 4 months, whereas hardware advancements like GPU flops are doubling every 24 months, leading to a gap between the need for more compute power and the industry's ability to provide it.
What is the significance of flops (floating-point operations per second) in AI training?
-Flops are critical in AI training because they measure the computational power needed to process large models and datasets. More flops enable faster model training and better results, which is why AI clusters require higher flops to meet the demands of growing AI models.
What challenges arise from training larger AI models?
-Larger AI models require more compute resources, including more GPU power and more IO bandwidth. This makes training these models slower and more complex, as the data and parameters don't fit on a single GPU, requiring parallelization and distributed computing to handle the workload.
What role does IO play in the design of AI clusters?
-IO plays a crucial role in AI cluster design because larger models and datasets require more bandwidth to communicate between GPUs and other hardware. The IO fabric, including both scale-up and scale-out networks, needs to be able to handle the increased demand for data transfer, ensuring efficient training and inference processes.
How does Meta approach AI cluster design to meet these demands?
-Meta approaches AI cluster design by focusing on flexibility across the entire hardware stack, from software to hardware. They aim for reconfigurable hardware to meet diverse workloads, which includes training large language models and running AI inference tasks efficiently. They also prioritize collaboration within the open community to share innovations.
What are some of the key components in a modern AI cluster design?
-Key components in modern AI cluster design include GPUs, high-speed interconnects like Infiniband and NVLink, memory architecture such as HBM (high bandwidth memory), and the use of both scale-up and scale-out networks. These components work together to provide the necessary compute power and bandwidth for training and inference tasks.
What challenges exist with the current state of optical interconnects in AI clusters?
-Current optical interconnects are not yet fast enough to keep up with the rapidly increasing demand for compute power and IO bandwidth in AI clusters. The technology is still in development, and there is a significant gap between where optical technologies are today and where they need to be to support next-generation AI models.
How does Meta address the gap in optical technology for AI clusters?
-Meta addresses the gap in optical technology by investing in early-stage developments, ensuring that optical solutions are ready ahead of schedule. They emphasize the need for co-investment and close collaboration within the community to speed up the maturity of optical technologies to meet the growing demands of large-scale AI clusters.
Outlines
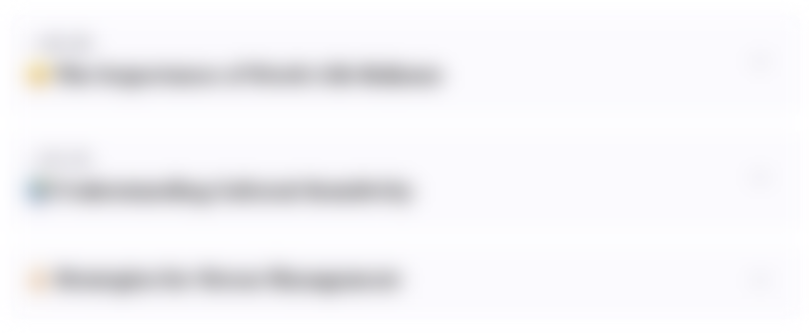
This section is available to paid users only. Please upgrade to access this part.
Upgrade NowMindmap
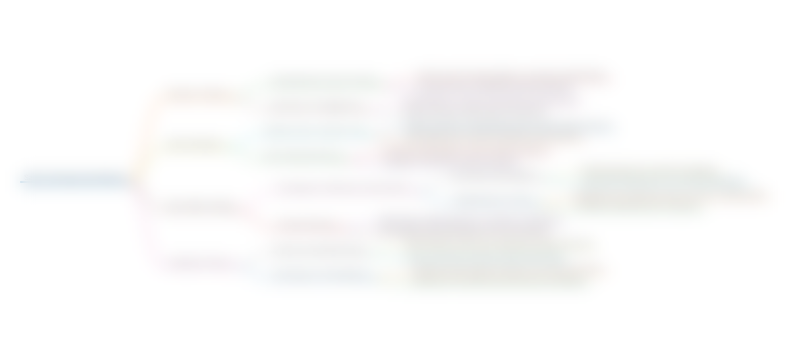
This section is available to paid users only. Please upgrade to access this part.
Upgrade NowKeywords
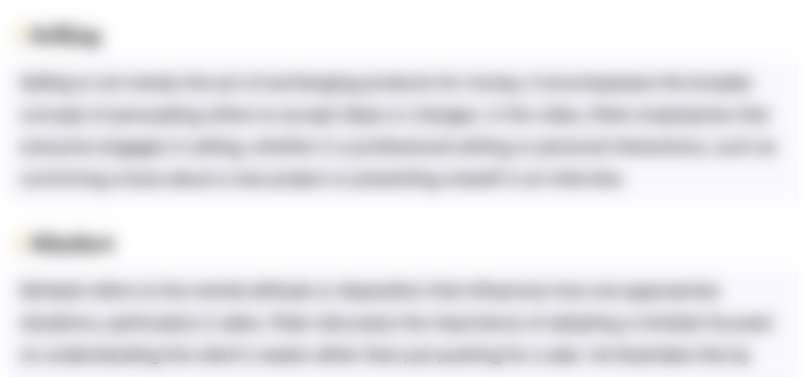
This section is available to paid users only. Please upgrade to access this part.
Upgrade NowHighlights
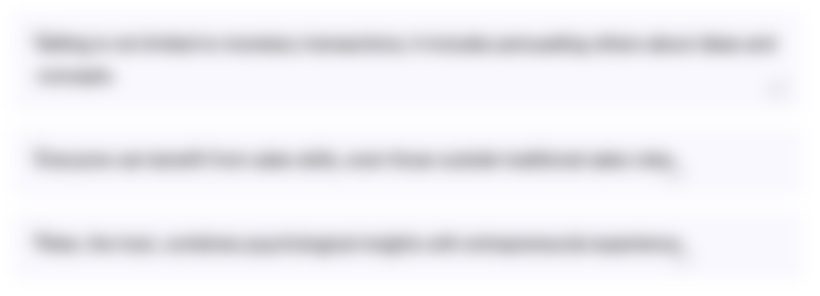
This section is available to paid users only. Please upgrade to access this part.
Upgrade NowTranscripts
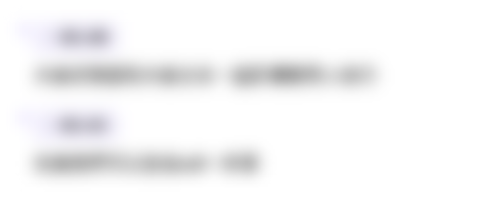
This section is available to paid users only. Please upgrade to access this part.
Upgrade NowBrowse More Related Video
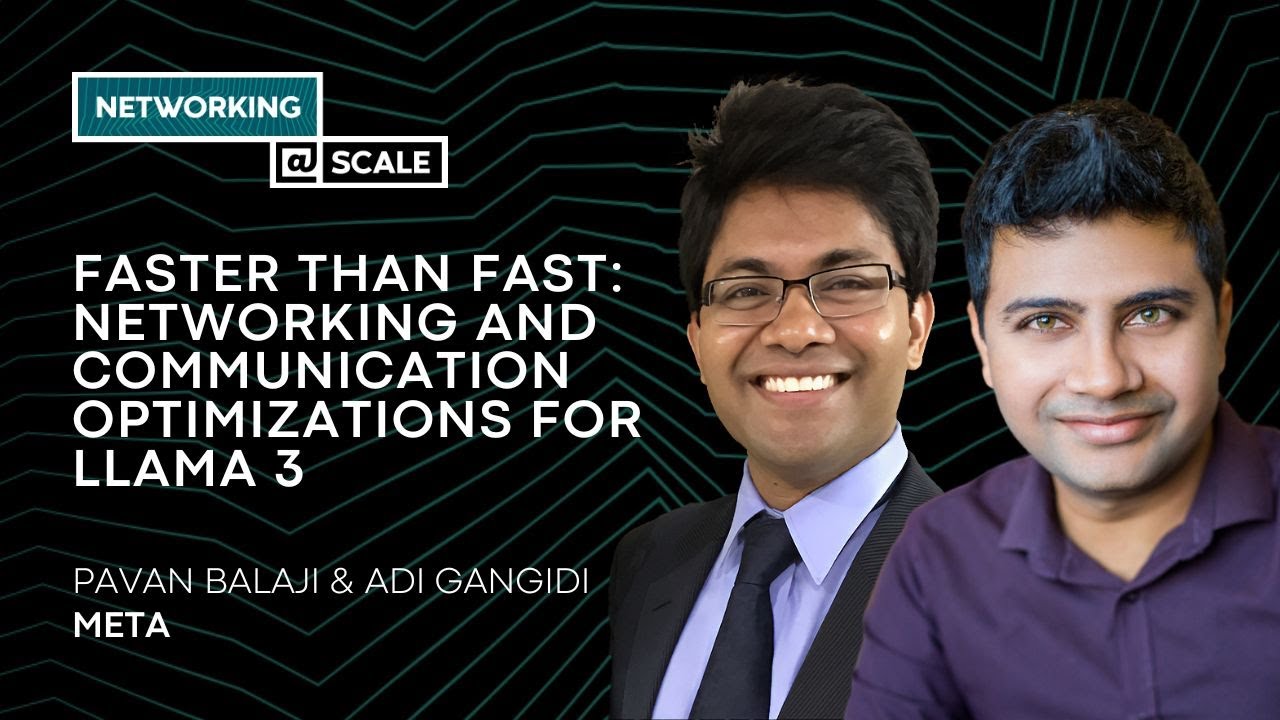
Faster Than Fast: Networking and Communication Optimizations for Llama 3
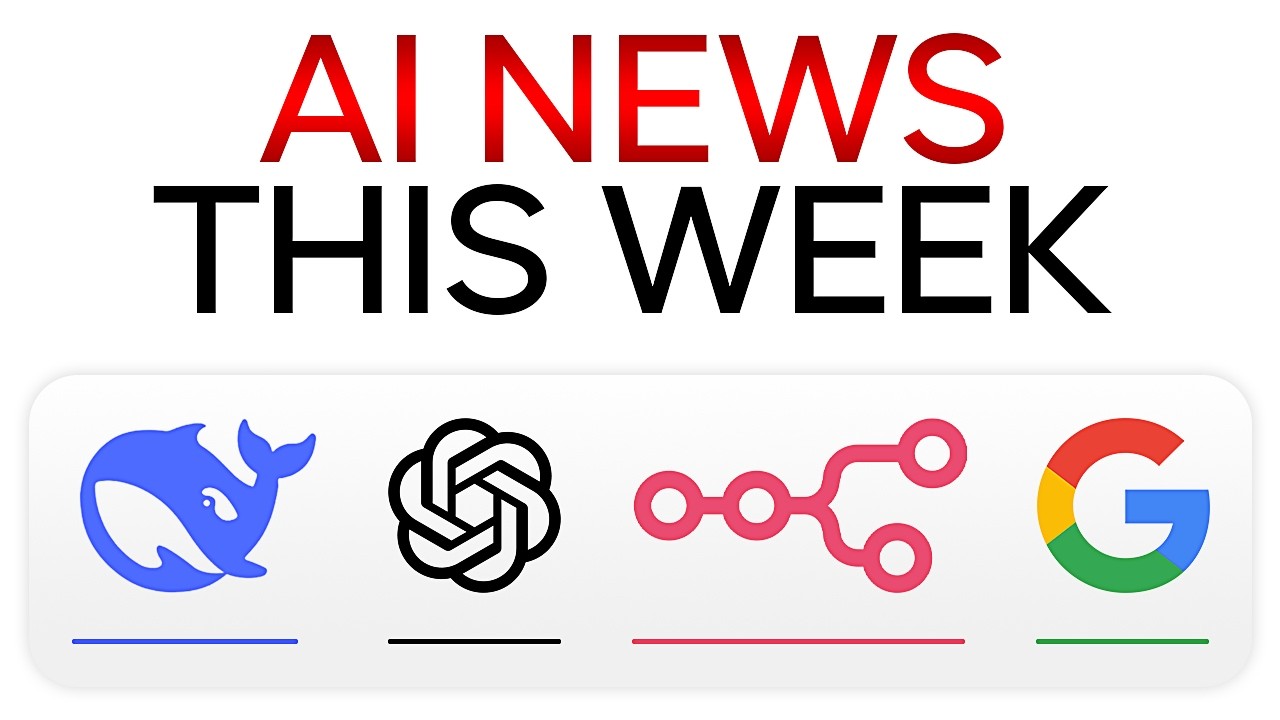
The Most INSANE AI News This Week! 🤯

Technology and the Future of Dental Practice | Dr. Yara Oweis | TEDxPSUT
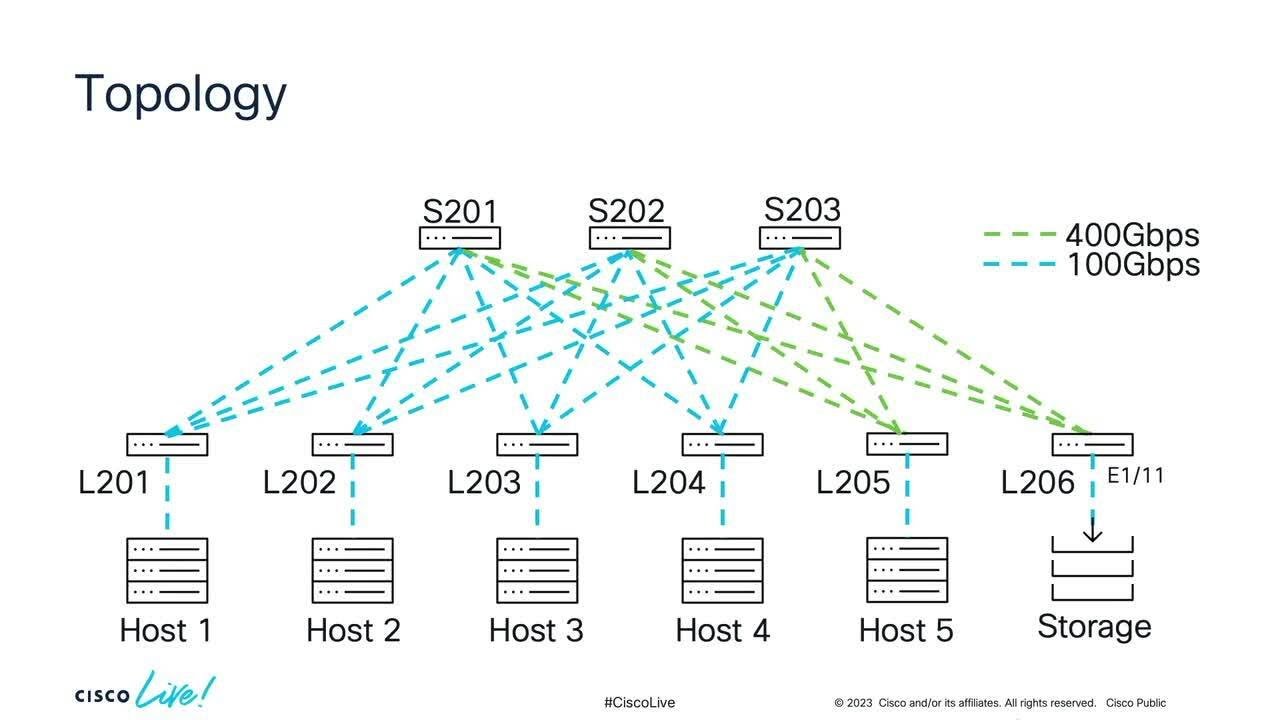
Cisco Artificial Intelligence and Machine Learning Data Center Networking Blueprint
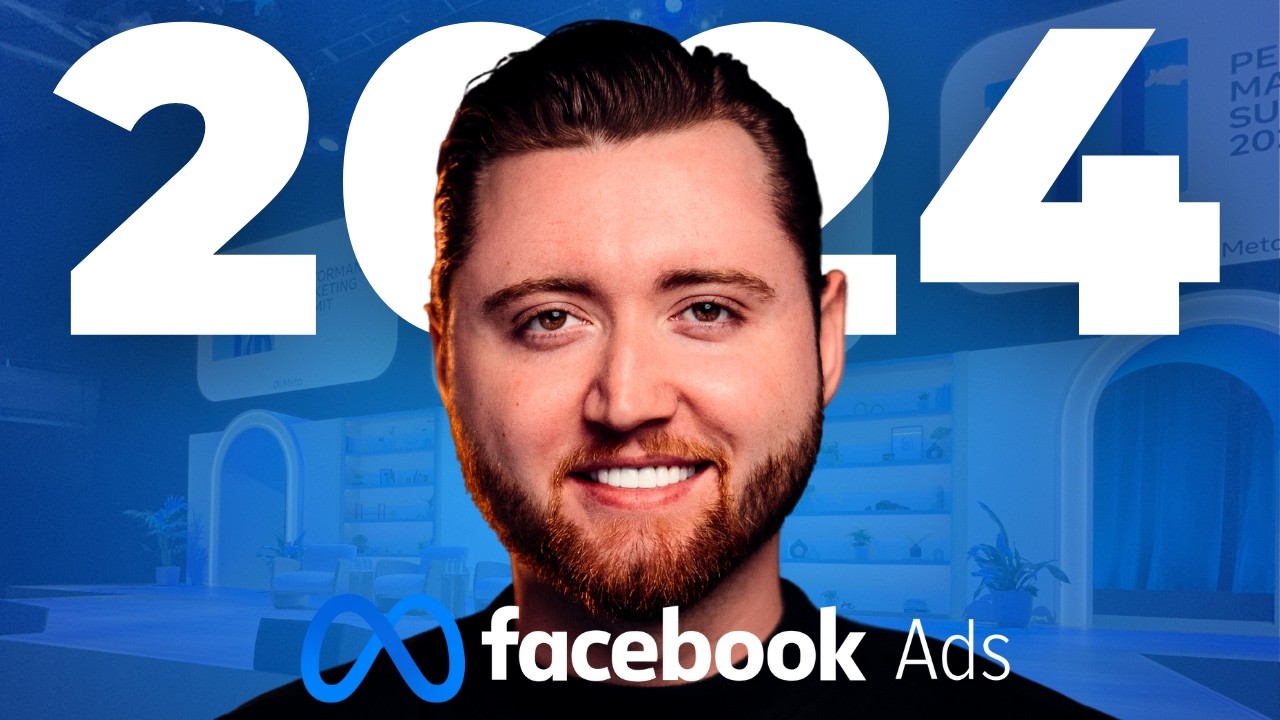
The LATEST Facebook Ad Updates in 2024

Giải ThÃch AI Agent Là Gì Như Thể Bạn Má»›i 5 Tuổi (CÆ¡ Há»™i Má»›i 2025)
5.0 / 5 (0 votes)