Natural Language Processing in Artificial Intelligence in Hindi | NLP with Demo and Examples
Summary
TLDRThis video delves into the realm of Natural Language Processing (NLP), a pivotal branch of artificial intelligence that enables computers to understand, interpret, and generate human language. With practical examples, the speaker elucidates the significance of NLP in various applications such as speech recognition, sentiment analysis, machine translation, and chatbots. The video also explores the intricacies of NLU (Natural Language Understanding), highlighting the challenges of ambiguity in language interpretation and the importance of context. It concludes with insights into NLG (Natural Language Generation), emphasizing the need for intelligent and contextually relevant responses in AI communication.
Takeaways
- 💡 Natural Language Processing (NLP) is a vital branch of artificial intelligence that enables computers to understand, interpret, and generate human language.
- 🗣️ Human communication relies on natural language, and NLP aims to replicate this ability in machines, allowing them to communicate effectively with humans.
- 📈 NLP has a wide range of applications, including speech recognition, sentiment analysis, machine translation, and chatbots, which are utilized by major tech companies like Google and Amazon.
- 🔍 Speech recognition technology allows devices like Google Assistant and Siri to interpret spoken language and provide appropriate responses.
- 📊 Sentiment analysis is used to gauge public opinion on social media platforms, helping to analyze the positive or negative sentiments towards various topics, such as movie ratings or political stances.
- 🌐 Machine translation services, like Google Translate, facilitate communication by instantly translating text from one language to another, breaking down language barriers.
- 🤖 Chatbots are AI-powered programs that simulate conversation with users, providing automated responses based on their knowledge base, enhancing customer service and user engagement.
- 🧠 The process of NLP involves several stages: Natural Language Understanding (NLU), where the machine interprets the user's intent, and Natural Language Generation (NLG), where the machine formulates a response.
- 🔎 NLU faces challenges such as lexical, syntactic, semantic, and pragmatic ambiguity, which require sophisticated algorithms to resolve and ensure accurate interpretation of language.
- 💬 NLG focuses on creating contextually relevant and intelligent responses that are structured and meaningful, ensuring the machine's reply aligns with the user's query and maintains a natural conversation flow.
Q & A
What is Natural Language Processing (NLP)?
-Natural Language Processing is a sub-field of computer science, information engineering, and artificial intelligence that is concerned with the interactions between computers and human languages, aiming to enable computers to understand, interpret, and generate human language in a way that is both meaningful and useful.
Why is NLP important for competitive exams and interviews?
-NLP is important for competitive exams and interviews because it is a rapidly growing field in artificial intelligence with numerous applications in technology and business. Understanding NLP can give candidates an edge in exams and interviews, showcasing their knowledge of current technological trends and their potential to work with cutting-edge AI systems.
What are the primary applications of NLP mentioned in the script?
-The primary applications of NLP mentioned in the script include speech recognition, sentiment analysis, machine translation, and chatbots. These applications are used in various industries such as IT, customer service, social media, and language translation services.
Can you provide an example of speech recognition technology?
-An example of speech recognition technology is Google Assistant and Siri by Apple. These systems can interpret spoken language in various languages, understand the user's query, and provide a relevant response.
How does sentiment analysis work on social media platforms?
-Sentiment analysis on social media platforms like Facebook and Twitter involves analyzing text from posts or tweets to determine the sentiment or opinion expressed, such as whether the comments are positive or negative. This can be used for gauging public opinion on various topics, like movie ratings or political stances.
What is machine translation and how does it help in communication?
-Machine translation is the process by which a computer program translates text or speech from one language to another. It helps in communication by allowing people who speak different languages to understand each other without needing a human interpreter, making it easier to communicate across language barriers.
What is a chatbot and how does it function?
-A chatbot is a software application that uses AI to conduct conversations with users in natural language. It functions by processing user inputs, understanding the intent behind the queries, and generating appropriate responses from its knowledge base to simulate a conversation with a human.
What are the challenges faced by NLP in understanding human language?
-The challenges faced by NLP in understanding human language include lexical ambiguity, syntactic ambiguity, semantic ambiguity, and pragmatic ambiguity. These challenges arise from the multiple meanings of words, sentence structures, contextual meanings, and the interpretation of phrases based on the situational context.
How does NLP handle ambiguity in language?
-NLP handles ambiguity in language through various techniques such as tokenization, parsing, lemmatization, stemming, and named entity recognition. These methods help in breaking down the text, understanding the context, and differentiating between various meanings to provide a more accurate interpretation.
What is the role of Natural Language Generation (NLG) in NLP?
-Natural Language Generation (NLG) in NLP is responsible for generating human-like text based on the understanding achieved through Natural Language Understanding (NLU). It involves text and syntax planning, using a knowledge base to construct coherent and contextually relevant responses to user queries.
How does structured data play a role in NLG?
-Structured data in NLG plays a role by providing a well-organized and easily accessible knowledge base from which the system can select and arrange information to generate responses. This ensures that the generated text is not only coherent but also relevant and structured in a way that is easy for users to understand and utilize.
Outlines
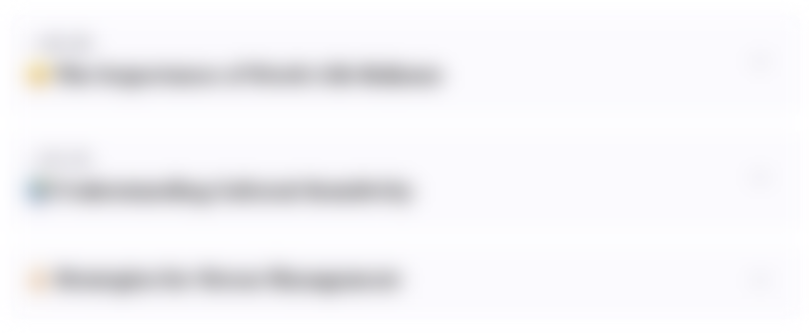
This section is available to paid users only. Please upgrade to access this part.
Upgrade NowMindmap
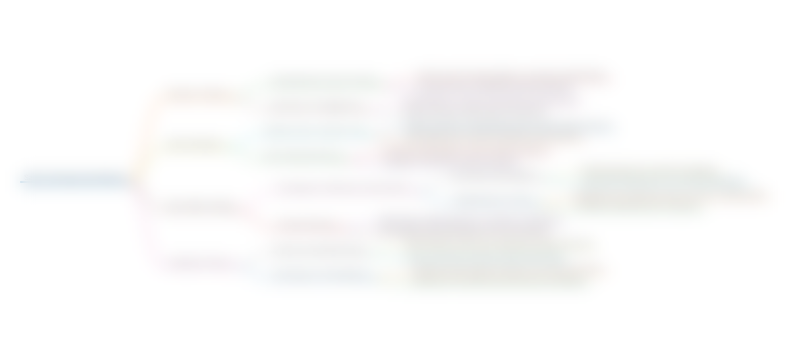
This section is available to paid users only. Please upgrade to access this part.
Upgrade NowKeywords
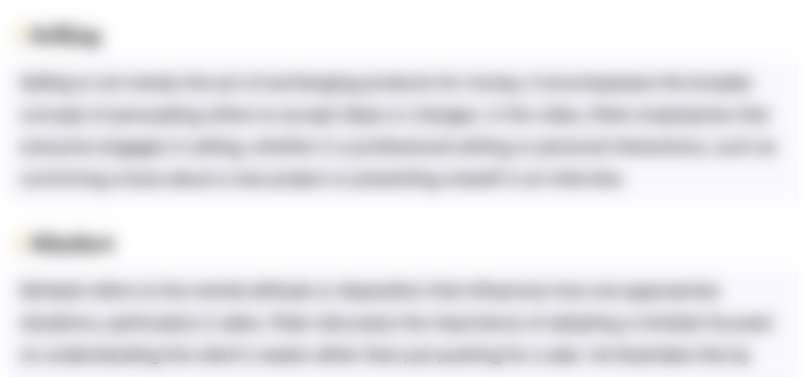
This section is available to paid users only. Please upgrade to access this part.
Upgrade NowHighlights
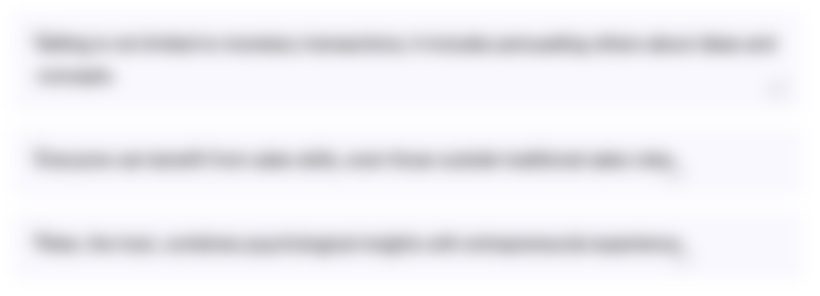
This section is available to paid users only. Please upgrade to access this part.
Upgrade NowTranscripts
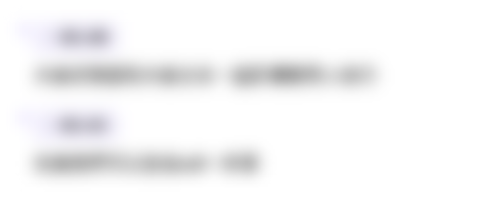
This section is available to paid users only. Please upgrade to access this part.
Upgrade NowBrowse More Related Video
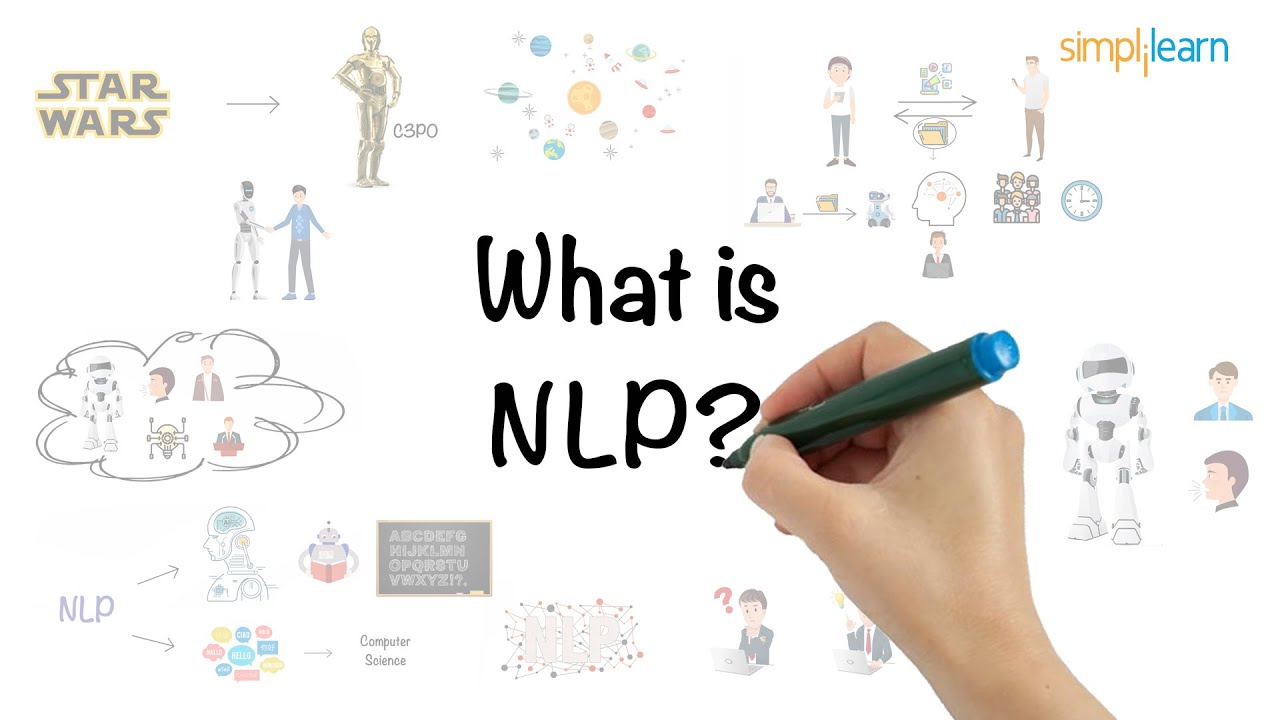
Natural Language Processing In 5 Minutes | What Is NLP And How Does It Work? | Simplilearn
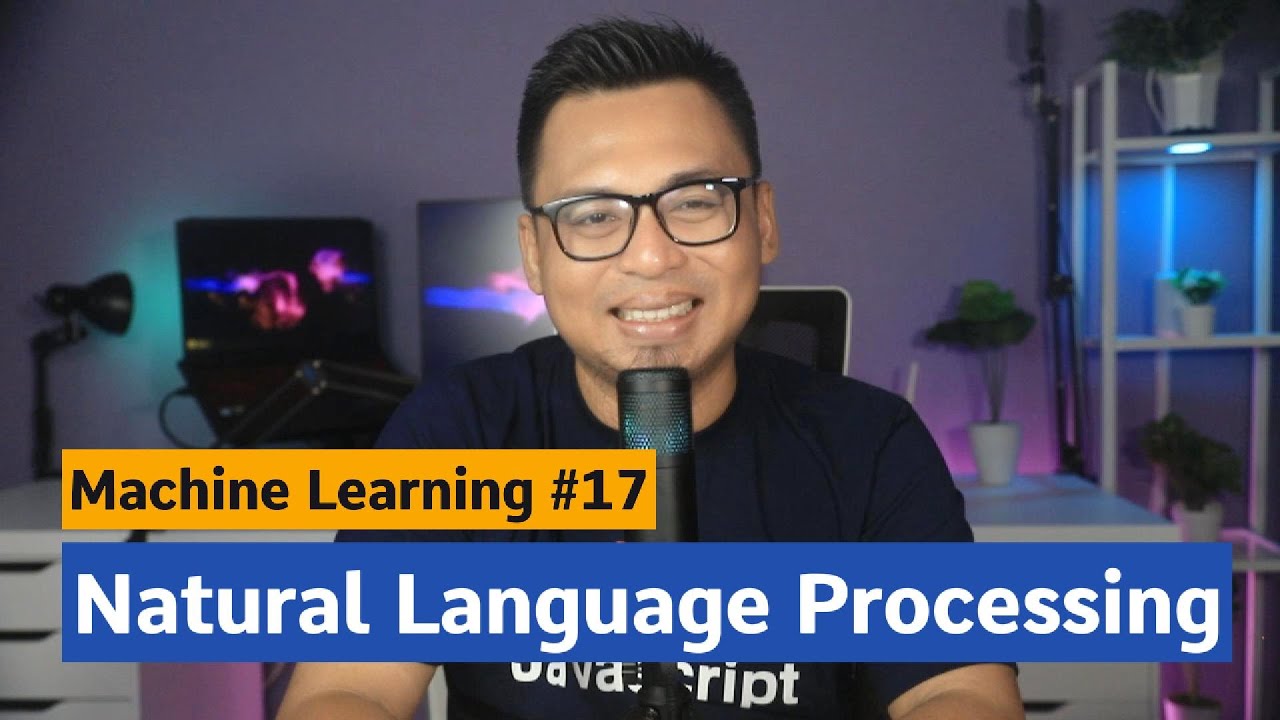
Machine Learning #17 - Natural Language Processing (Pemrosesan Bahasa Alami)
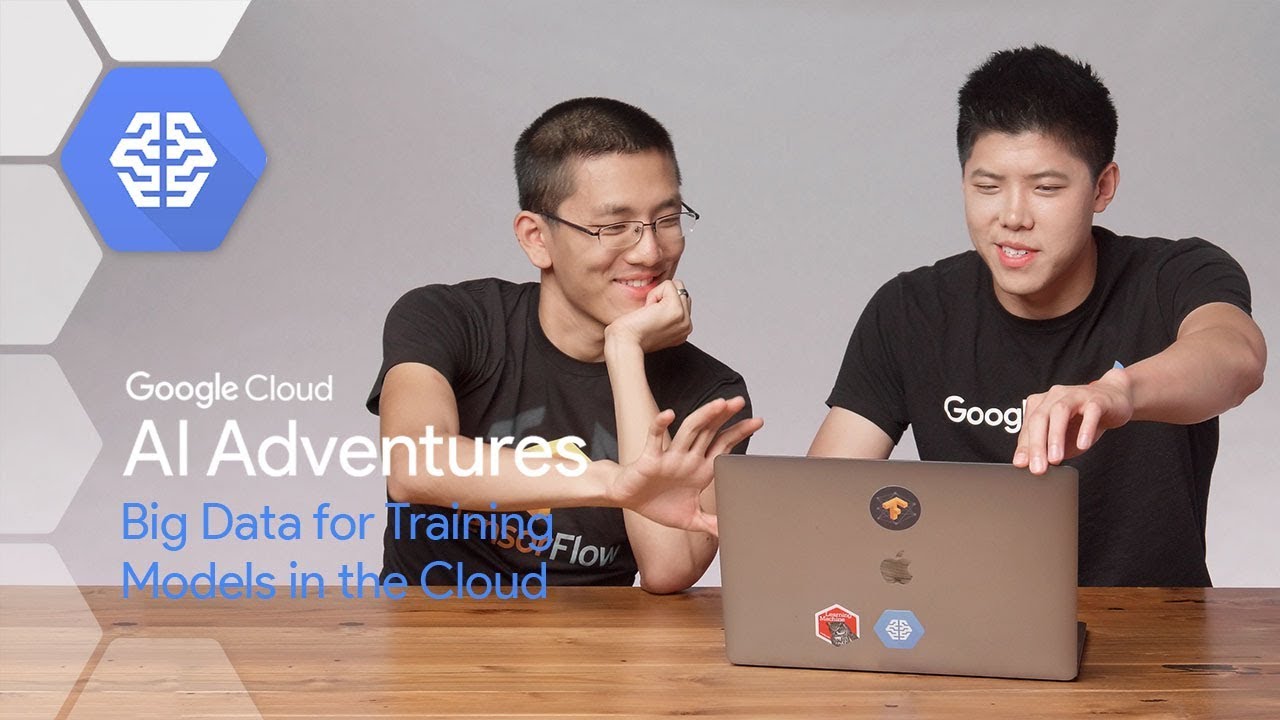
Natural Language Generation at Google Research
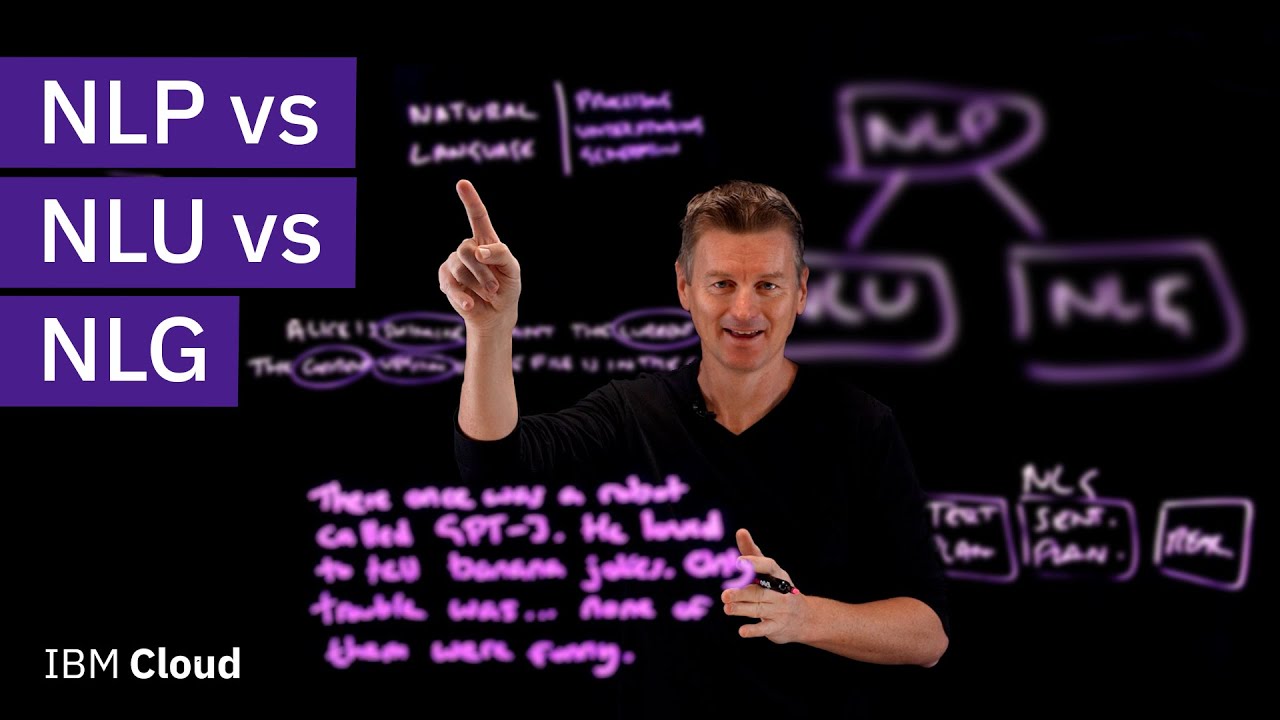
NLP vs NLU vs NLG

WML और MLM में अंतर क्या है? जानिए आसान भाषा में @Directsellingsuccessguaranty
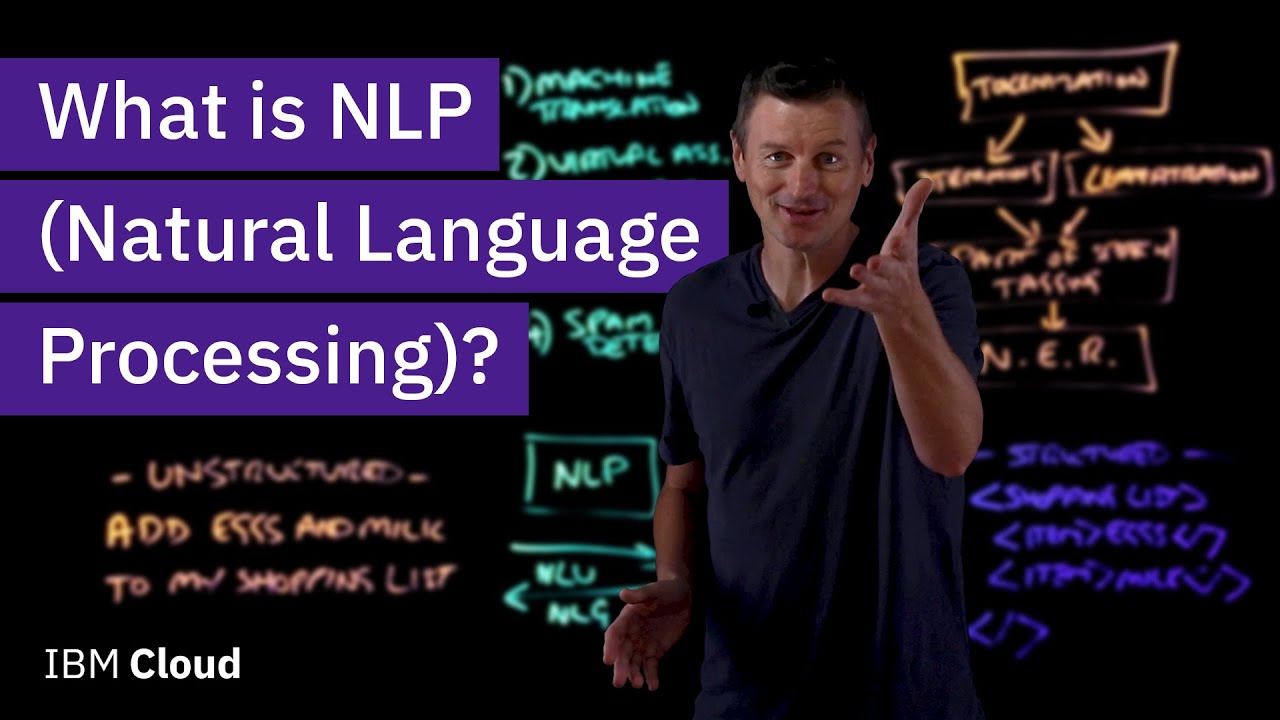
What is NLP (Natural Language Processing)?
5.0 / 5 (0 votes)