Webinar: The Skeptic's Guide to Data by Deliveroo Sr PM
Summary
TLDRスクリプトの要点を簡潔にまとめ、ユーザーの興味を引き出す魅力的な概要を提供する。
Takeaways
- 🗣️ データは製品マネージャーが現実世界と製品がどのように相互作用するかを理解するために必要な真実の反映として扱われています。
- 🤔 データは単なるツールであり、適切に使用され、目的に応じて扱われなければなりません。
- 💡 データの能力と限界を理解し、他のツールと組み合わせて使用することが重要です。
- 📈 データはオペラ指揮者のように、オーケストラのさまざまなセクションを統合して豊かな音の景色を作り出す必要があります。
- ❓ データの収集、処理、提示の動機を理解することが、データの最大限の活用と正しい意思決定を確保するための鍵です。
- 🎯 正しいメトリックスを測定しているかどうかを確認し、問題を理解し、影響を測定するために必要なものであることを確認します。
- 🚫 データが常に正しいわけではなく、誤解されることがあるため、常に批判的であり、自分の前提を疑問にすることが大切です。
- 🔍 データの生成方法を理解し、その正確性と信頼性を確認することが、データの品質を向上させるために不可欠です。
- 🤝 ユーザー研究とデータ分析は互いに補完し、製品発見のための聖三一です。
- 🛫 データとユーザー洞察を組み合わせることで、美しくて実用的なものを作成することができます。
- 🥳 データに愛を注ぎ、それを批判的に検証し、理解し、全力で活用することで、素晴らしい製品を作り上げることができます。
Q & A
W Perkinsが話すデータ分析の目的は何ですか?
-W Perkinsは、データ分析が製品マネージャーが現実世界と製品がそれとの相互作用を理解するために必要な、信頼性の高い理解を得るための重要なツールであると述べています。
トニー・ベンの5つの質問とは何ですか?
-トニー・ベンの5つの質問は、「何の力を持っているのですか」「その力はどこから得たのですか」「その力を誰の利益のために使うのですか」「誰に対して責任を負うのですか」「どうやって私たちから取り除くのですか」という連続的な質問です。
データが「嘘をつかない」という考えに対して、W Perkinsはどのような立場を取ていますか?
-W Perkinsは、データが「嘘をつかない」との考えに対して批判的な視点を持ち、データは誤解されることがあるため、製品マネージャーはその情報を扱い的时候就には批判的な考えを持っていることが重要だと述べています。
データの収集、処理、提示の動機を理解するために、W Perkinsはどのようなアプローチを提案していますか?
-W Perkinsは、データの収集、処理、提示の動機を理解するために、データが提示される背景や目的、そしてデータがどのように生成されたかを掘り下げることが重要だと提案しています。
W Perkinsが指摘する、「正しいメトリックス」とは何を意味しますか?
-「正しいメトリックス」とは、製品マネージャーが問題を理解し、影響を測定するために必要な、適切なデータ指標を意味しています。重要なのは、データが実際に必要な情報を正確に反映しているかどうかを確認することです。
データの生成方法を理解することは、なぜ重要ですか?
-データの生成方法を理解することは、データの完全性や信頼性を評価し、データが意図したメッセージを正確に伝えているかどうかを確認するために重要です。また、ユーザーの動機や製品との相互作用を理解することも重要です。
W Perkinsはデータの検証に関してどのようなアドバイスをしていますか?
-W Perkinsは、データの検証においてユーザー洞察を利用することが重要であると述べています。データだけを頼りにせず、ユーザー研究や顧客洞察を組み合わせることで、より正確な理解を得ることができます。
データとユーザー洞察の違いは何ですか?
-データは製品のユーザーとの相互作用を記録し、詳細が豊富な技術的なドローイングに似ていますが、ユーザー洞察はより印象主義的な絵画に似ており、世界に対する鮮やかなイメージを提供します。しかし、データは構造的な表現であり、ユーザー洞察は直接的な測定が困難です。
W Perkinsは製品マネジメントにおいて、どのようにデータを活用することを提案していますか?
-W Perkinsは、製品マネジメントにおいてデータを愛し、批判的に取り扱い、理解し、完全な潜在力を発揮させることを提案しています。また、データとユーザー洞察を組み合わせることで、素晴らしい製品を構築し、製品マネジメントの良いニュースを広めることも重要だと述べています。
W Perkinsが提案するデータ分析の5つの質問は何ですか?
-W Perkinsが提案するデータ分析の5つの質問は、「データセットの収集、処理、提示の動機は何ですか」「私が見るメトリックスは私が気にしているものであり、正しいことを測定しているのですか」「データはどのように生成されており、高い完全性を持っていますか」「どのようにしてそのデータを検証することができますか」「データを使用して意思決定をサポートする人たちをどのように参加させますか」です。
W Perkinsはなぜ、製品マネージャーには批判的な思考が必要だと述べていますか?
-W Perkinsは、製品マネージャーは最終的に意思決定について責任を持つため、情報を扱う的时候就は批判的な思考が必要です。データが誤解されることがあるため、製品マネージャーは決断を下す前に、その情報を厳密に検証する必要があります。
Outlines
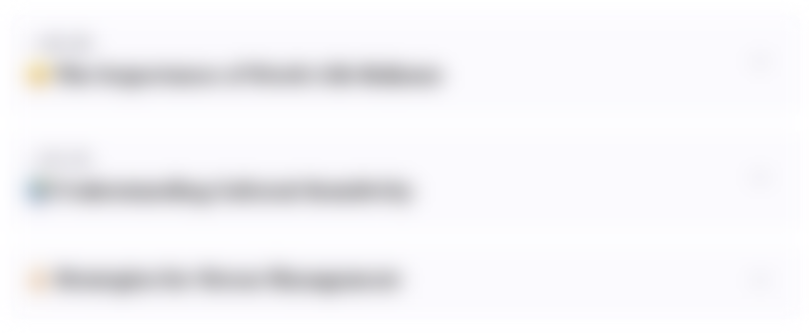
This section is available to paid users only. Please upgrade to access this part.
Upgrade NowMindmap
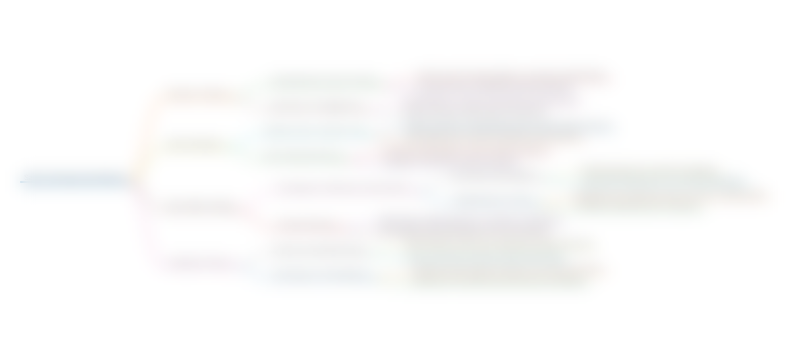
This section is available to paid users only. Please upgrade to access this part.
Upgrade NowKeywords
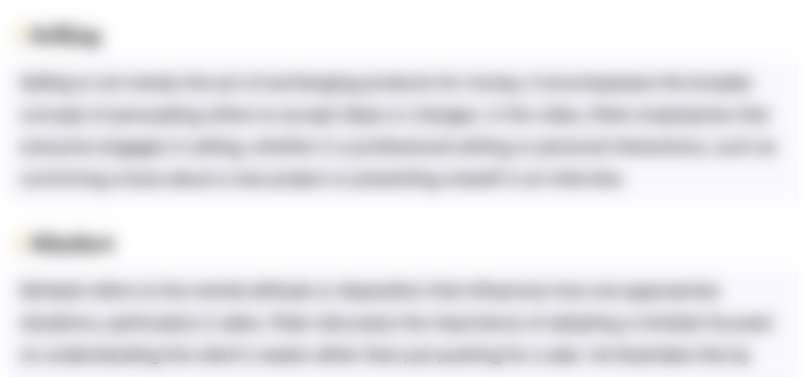
This section is available to paid users only. Please upgrade to access this part.
Upgrade NowHighlights
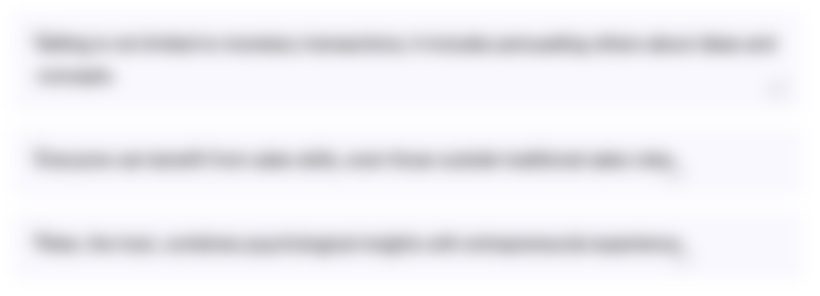
This section is available to paid users only. Please upgrade to access this part.
Upgrade NowTranscripts
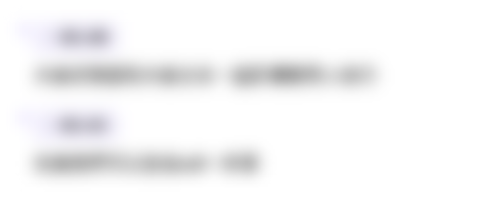
This section is available to paid users only. Please upgrade to access this part.
Upgrade NowBrowse More Related Video
5.0 / 5 (0 votes)