Retrieval Augmented Generation for Navigating Large Enterprise Documents
Summary
TLDRThe Google Cloud Community session featured the Generali Italia team discussing their experience with developing a RAAG-based application for navigating complex enterprise documents. The team highlighted the challenges of information retrieval in a heavily regulated industry with extensive documentation. They detailed their approach using large language models, the process of embedding and retrieval, and the importance of in-context learning. The session included a live demonstration and Q&A, emphasizing the team's innovative use of AI to enhance document accessibility and information retrieval within their organization.
Takeaways
- 📈 The Generali Italia team developed a RAAG (Retrieval-Augmented Generation) based application for navigating complex enterprise documents.
- 🚀 The project aimed to leverage AI advancements to simplify the information retrieval process within a large volume of technical and regulatory documentation.
- 📚 The team faced challenges with over 400 documents totaling more than 5,000 pages, which would take over 100 hours to read.
- 🔍 Information retrieval was identified as a key field to assist with the challenges, involving searching for documents, information within documents, or the documents themselves.
- 💡 The team utilized large language models (LLMs) and generative AI to surpass the state of the art in understanding language and generating meaningful conversations.
- 🧠 In-context learning was employed to reduce hallucinations in AI responses by providing the model with relevant context from the documents.
- 📊 The team conducted experiments with default parameters and later introduced custom strategies for document chunking and hyperparameter tuning.
- 📈 They created a synthetic dataset for evaluation purposes due to the lack of an existing validation set, extracting questions and answers using a large language model.
- 🔧 The experimentation involved tools like Vertex AI Platform, various LLMs, and a vector database for storing embeddings.
- 📝 The architecture included an ingestion phase and an inference phase, with the latter involving user interaction and frontend services.
- 🔄 The team plans to experiment with new foundation models and Vertex AI's vector search, as well as work on LLMs for better handling of new documents.
Q & A
What was the main challenge Generali Italia faced with their documentation?
-The main challenge was the continuous growth of textual data and knowledge, which made it difficult to extract information efficiently from a large volume of documents, leading to significant time consumption.
How did Generali Italia leverage AI to simplify the information retrieval process?
-They defined a perimeter of relevant business documents and used large language models within a retrieval-augmented (RAG) based solution to develop a document Q&A application.
What is information retrieval and how does it assist in addressing the challenges faced by Generali Italia?
-Information retrieval is the science of searching for information in documents or for documents themselves. It assists by providing methodologies to efficiently locate and extract the needed information from vast document collections.
What is the role of the embedding model in the RAG architecture?
-The embedding model, which is a large language model itself, takes text as input and returns a list of numbers (vector). It helps in creating context embeddings that are used to find similar information chunks for answering user queries.
How did Generali Italia handle the lack of a validation dataset for their RAG system?
-They created a synthetic dataset by extracting paragraphs from each document and using a large language model to generate questions and answers, which were then used for validation and performance evaluation.
What are the key metrics used to evaluate the performance of the RAG-based application?
-Key metrics include Mean Reciprocal Rank (MRR), Mean Average Precision (MAP) at a given cut-off of K, Recall, and Rouge and BLEU scores for comparing the quality of generated responses against real answers.
What was the significance of the research paper 'Lost in the Middle' in the context of Generali Italia's RAG system?
-The paper provided insights into how large language models use the information from the context provided. This led Generali Italia to introduce a re-ranking layer to optimize the organization of information presented to the LLM for better performance.
How did Generali Italia ensure the scalability and reliability of their RAG-based application?
-They utilized the Vertex AI platform for experimentation and model training, which ensured scalability and reproducibility. Additionally, they used Google's infrastructure for the reliability of their product.
What was the outcome of the experiments with custom chunking strategies and hyperparameter tuning?
-The experiments resulted in improved performance, with the best chunk size identified as 1,000 characters and a recall of 80% at 15 documents, along with a question-answer accuracy of 73%.
How did Generali Italia address the need to explain acronyms and insurance definitions to users?
-They added custom chunks to their collection that explained acronyms and insurance definitions, which improved the chatbot's ability to answer questions related to these topics, despite a slight decrease in overall metrics.
What are the next steps for Generali Italia's RAG-based application?
-The next steps include testing new foundation models like Gemini Pro, using Vertex AI's side-by-side pipeline to compare different models, and exploring Vertex Vector search for a more efficient vector database solution.
Outlines
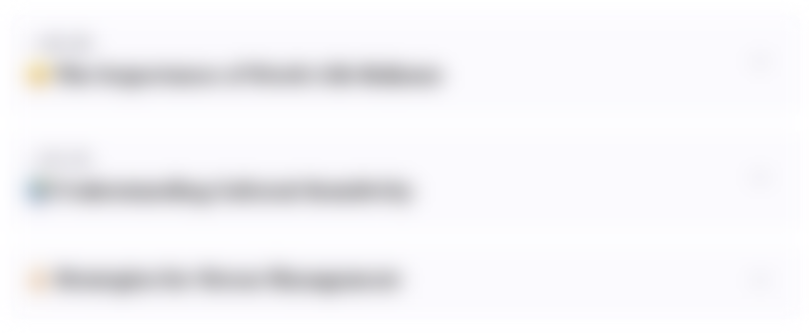
This section is available to paid users only. Please upgrade to access this part.
Upgrade NowMindmap
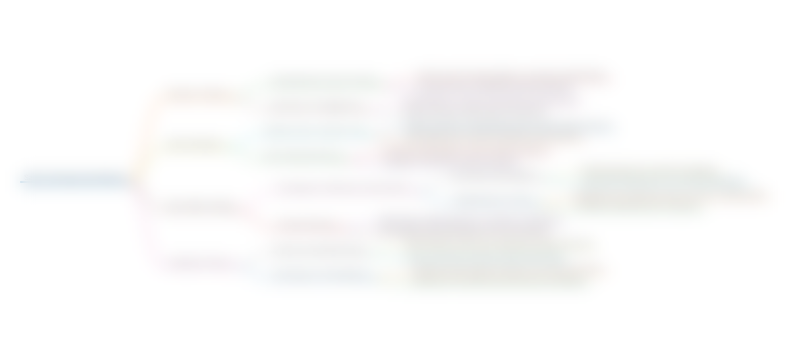
This section is available to paid users only. Please upgrade to access this part.
Upgrade NowKeywords
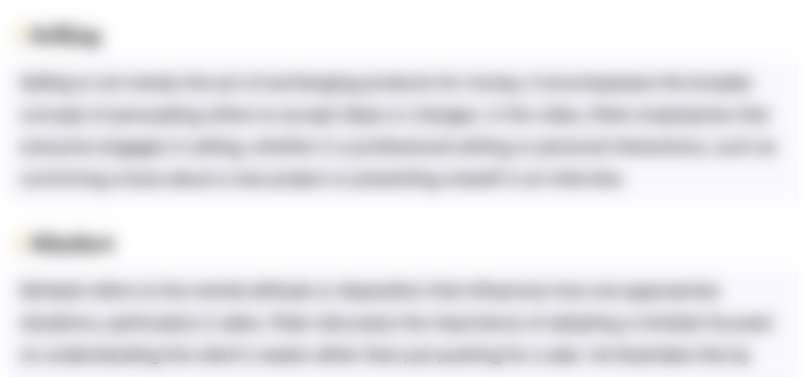
This section is available to paid users only. Please upgrade to access this part.
Upgrade NowHighlights
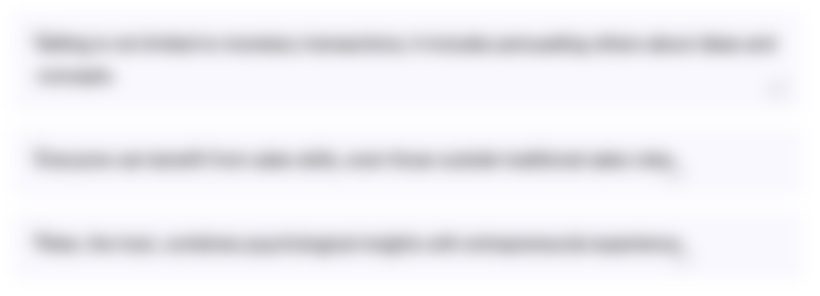
This section is available to paid users only. Please upgrade to access this part.
Upgrade NowTranscripts
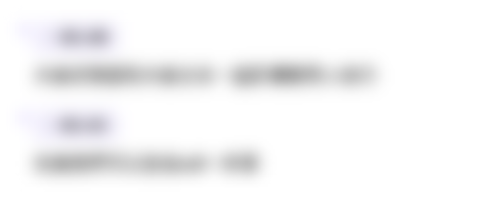
This section is available to paid users only. Please upgrade to access this part.
Upgrade NowBrowse More Related Video

Advanced Retrieval - Multi-Vector ("More Vectors Are Better Than One")
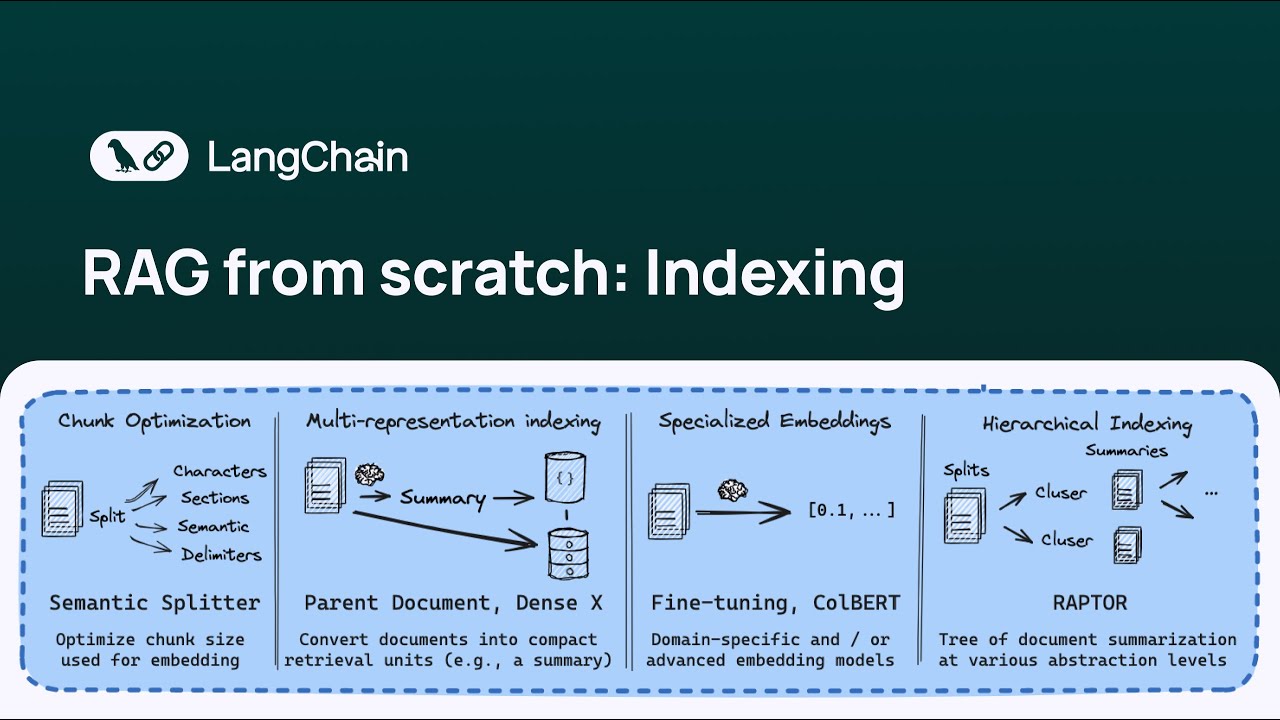
RAG from scratch: Part 12 (Multi-Representation Indexing)
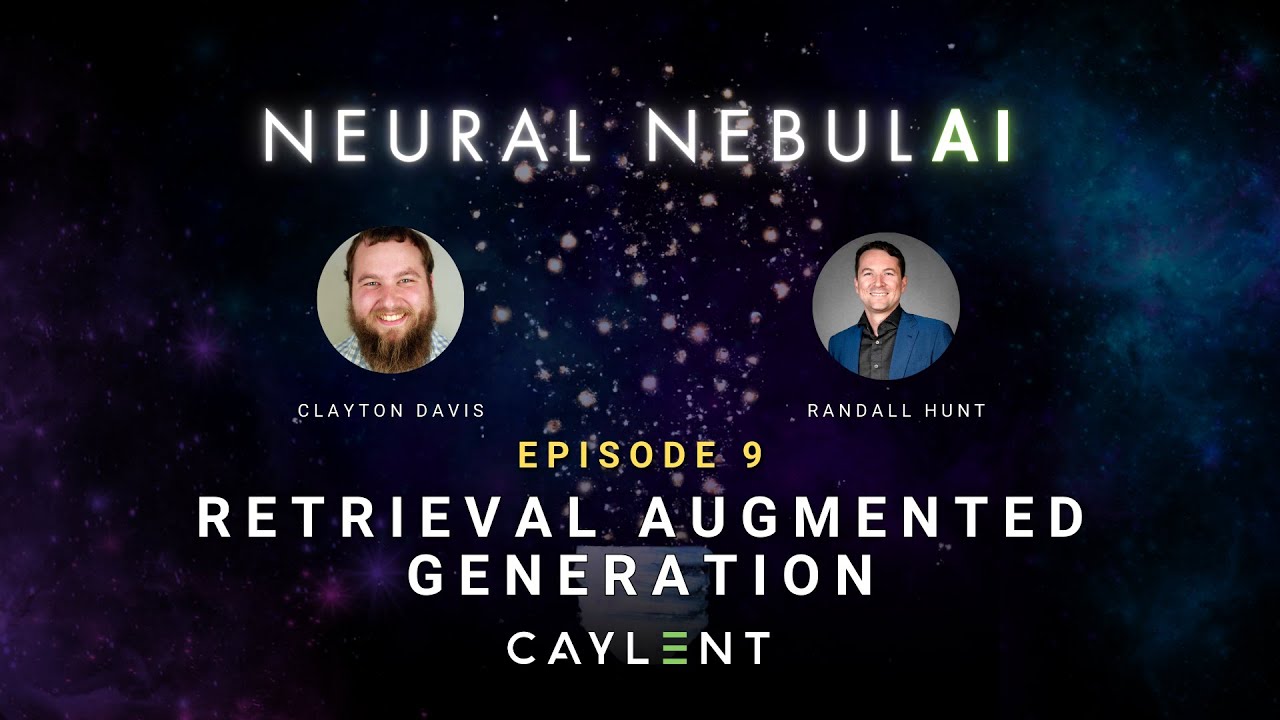
Retrieval Augmented Generation - Neural NebulAI Episode 9
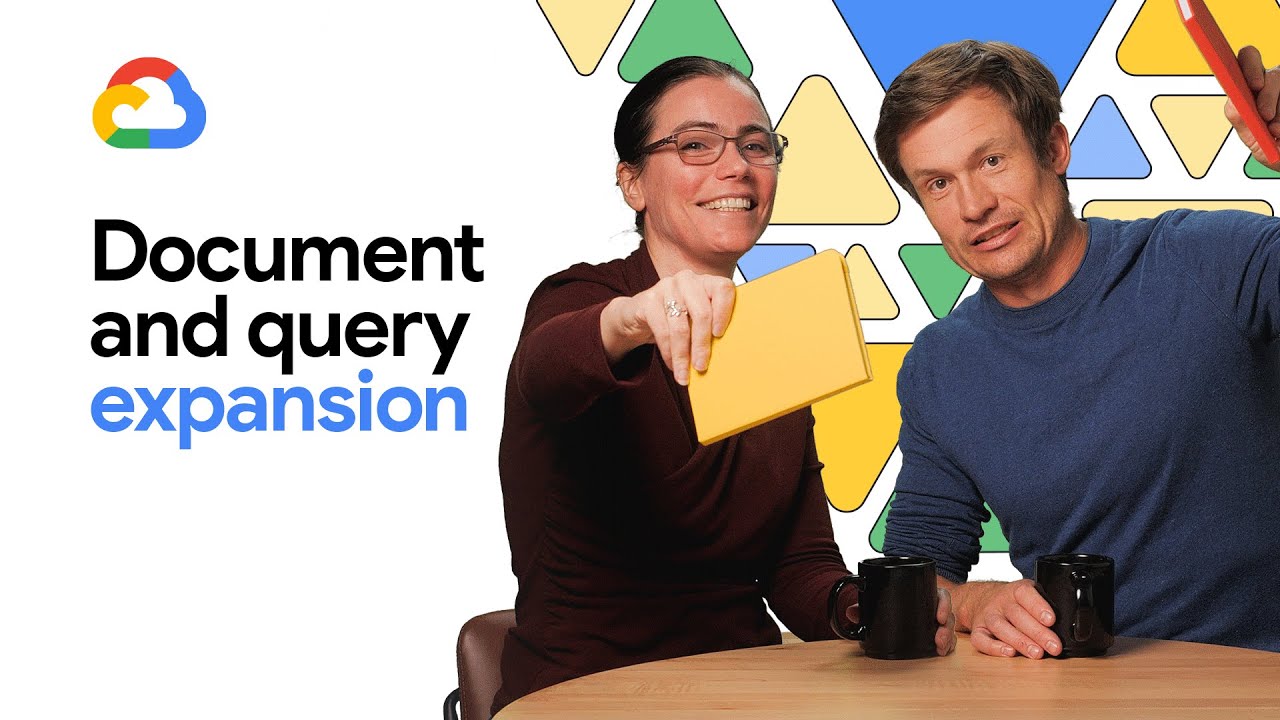
Using RAG expansion to improve model speed and accuracy
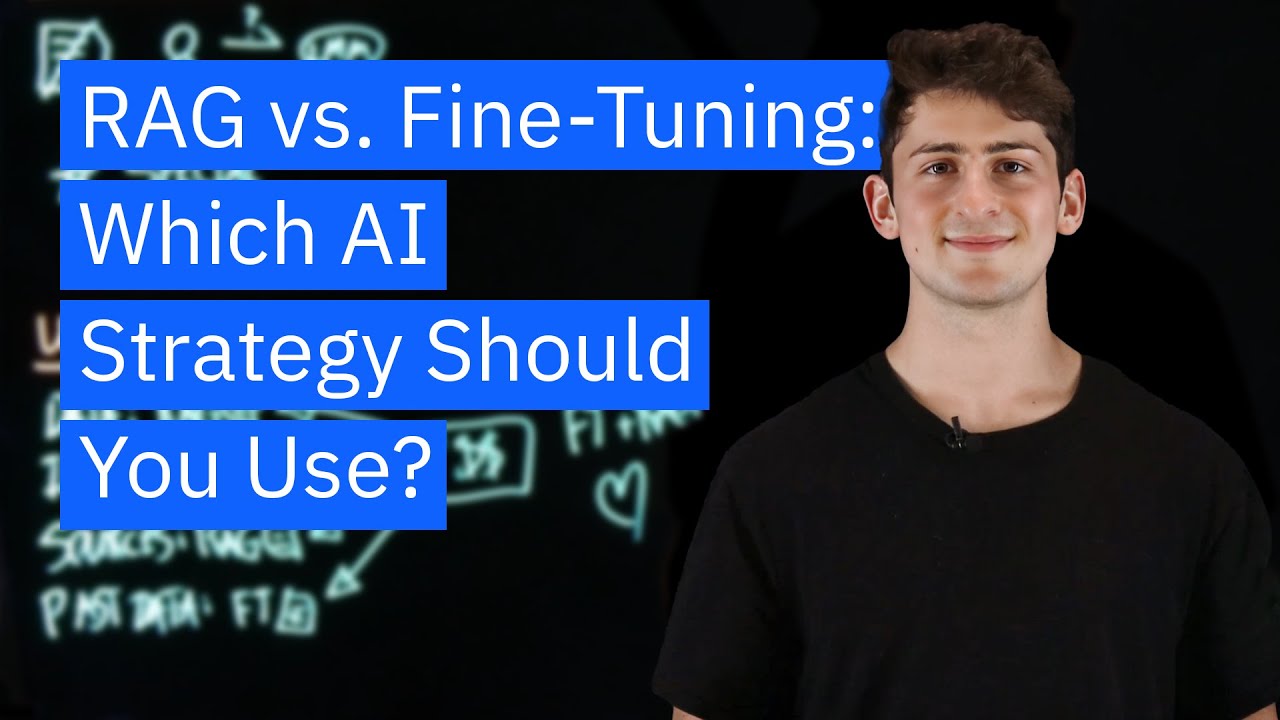
RAG vs. Fine Tuning
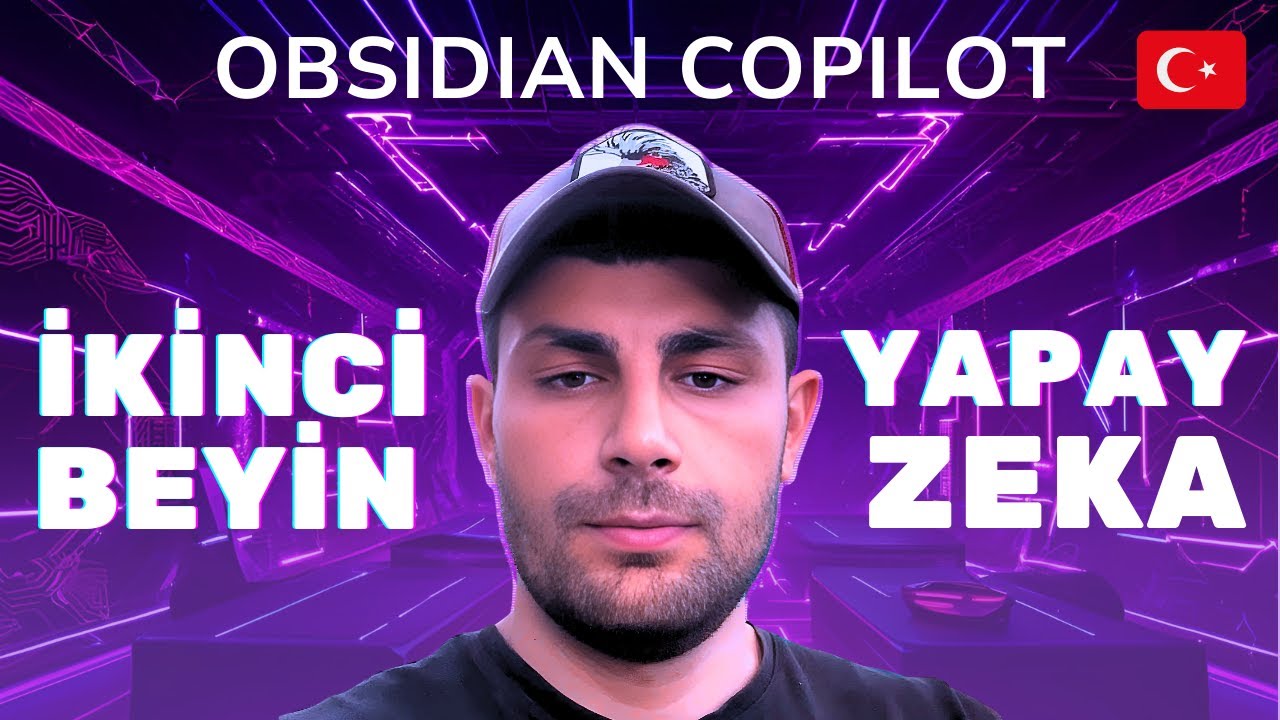
Not Tutarken Bu Yöntemi Kullanmak Size İkinci Beyin Kazandıracak
5.0 / 5 (0 votes)