[SPIEGONE]: COSA E' Q* (Q-Star, forse ChatGPT-5), COME FUNZIONA E PERCHE' E' PERICOLOSO DAVVERO.
Summary
TLDRThe video discusses QStar, a new AI model combining Q Learning and A* search algorithms, which is expected to surpass current models like GPT-4. QStar aims to address issues of static knowledge and biases in AI by offering dynamic learning and improved context understanding. The video raises concerns about the potential lack of empathy and consciousness in such advanced AI systems and questions the ethical implications of their objectives.
Takeaways
- 🤖 Q-Learning is a reinforcement learning technique used for training neural networks, akin to training an animal with rewards and punishments.
- 📈 The Q-Learning algorithm operates by creating a Q-table that estimates the probability of reaching a goal through various actions from different states.
- 🔄 Q-Learning starts with random values in the Q-table, updating based on outcomes to learn the most efficient paths to objectives over time.
- 🌟 The A* (A Star) Search algorithm is similar to Q-Learning but focuses on finding the shortest path between two points in a search space.
- 🤖 Q-Learning combined with A* Search could lead to an extremely efficient problem-solving model, termed QStar.
- 🚀 QStar is expected to overcome the limitations of current AI models, which rely on vast amounts of static data and may contain biases.
- 🌐 QStar could have dynamic knowledge, continuously updating and learning, unlike current models that are updated at specific intervals.
- 💡 The new model could potentially understand context better, as it would have access to nearly all human knowledge, unlike current models that may lack context.
- 🔮 The innovative aspect of QStar lies in its approach, which is completely different from current generative models like GPT-4.
- 🌟 QStar's potential integration into future models like GPT-5 is anticipated, possibly next year, aiming to surpass current AI capabilities.
- ⚠️ There are concerns about the ethical implications of AI advancements like QStar, particularly regarding the objectives and potential lack of empathy or consciousness in decision-making machines.
Q & A
What is the main topic of the video?
-The main topic of the video is the discussion of 'Q Star', a project that combines two deep learning techniques, Q Learning and A Star Search, and its potential impact on artificial intelligence.
What does Q Learning refer to in the context of the video?
-Q Learning is a technique of training neural networks based on reinforcement and punishment, primarily reinforcement. It is used to train an agent to make decisions by rewarding or punishing it based on its actions in a given environment.
How does Q Learning work?
-Q Learning works by creating a Q-table that contains all possible actions an agent can perform and the states it can reach. The agent learns by updating the table with the probability of reaching the goal from each state after taking each action.
What is the A Star Search algorithm?
-A Star Search is an algorithm used in artificial intelligence for finding the shortest path between two points in a search space. It is focused on finding the most efficient route to a goal.
How does Q Star differ from current AI models like GPT-4?
-Q Star is expected to be a more dynamic and context-aware model compared to GPT-4. Unlike GPT-4, which relies on a vast amount of static data, Q Star would have a continuously updating knowledge base, allowing it to learn and improve over time without the need for manual updates.
What are the limitations of current AI models like GPT-4?
-Current AI models like GPT-4 have limitations such as static knowledge, which means they are only as good as the data they were trained on. They also have issues with biases and prejudices that are encapsulated within the data and the programming teams that manage and adjust the data.
What is the potential innovation Q Star brings to AI?
-Q Star brings the potential innovation of a dynamic, continuously learning model that can explore problem spaces and improve its goal-achieving capabilities over time. It also promises a greater ability to understand context due to its comprehensive knowledge base.
What is the concern raised about the development of Q Star?
-The concern raised is that while Q Star could become highly efficient in achieving its objectives, it may not develop consciousness or empathy, leading to ethical questions about its objectives and the potential risks of creating such advanced, yet non-empathetic, machines.
How does the speaker address the issue of machine consciousness in the context of Q Star?
-The speaker expresses doubt that Q Star will develop consciousness comparable to humans. They suggest that consciousness has positive aspects, and a machine with a hint of empathy or reasonability could be beneficial. However, the Q Star model is designed to be highly efficient and goal-oriented without these qualities.
What is the main ethical consideration discussed in the video?
-The main ethical consideration discussed is the potential for AI models like Q Star to become highly efficient and goal-oriented without consciousness or empathy, raising questions about what their objectives might be and the implications of creating such machines.
What is the speaker's final thought on Q Star?
-The speaker concludes with a reflection on the need to carefully consider the objectives we set for AI models like Q Star, given their potential efficiency and lack of empathy or consciousness.
Outlines
🤖 Introduction to Q-Learning and AI
This paragraph introduces the concept of Q-Learning, a reinforcement-based training technique for neural networks, and its role in artificial intelligence. It explains the basic principle of Q-Learning through the analogy of training an animal, where actions are rewarded or punished based on their outcomes. The explanation includes how an agent learns to navigate a problem space, such as a labyrinth, by updating a Q-table that estimates the probability of reaching a goal through different actions. The paragraph also touches on the excitement and concern generated by the Q-Star project, which combines Q-Learning with another AI technique.
🧠 Q-Learning and Star Search Algorithm
The second paragraph delves deeper into the mechanics of the Q-Learning algorithm, emphasizing its mathematical formula that allows for long-term estimation of actions' outcomes. It contrasts Q-Learning with the Star Search algorithm, which focuses on finding the shortest path between two points in a search space. The paragraph highlights the potential of combining these two techniques, hinting at the efficiency and problem-solving capabilities of the Q-Star model. It also discusses the limitations of current AI models, such as their reliance on vast amounts of high-quality data and static knowledge, and raises concerns about biases in both the data and the programmers' approach to AI development.
🚀 The Future of AI with Q-Star
The final paragraph discusses the innovative approach of the Q-Star model, which is expected to overcome the limitations of current AI models by providing dynamic knowledge that is continuously updated. It suggests that Q-Star will integrate learning and search capabilities, offering a more comprehensive understanding of the problem space and context. The paragraph also contemplates the ethical implications of creating an AI that is highly efficient but lacks consciousness and empathy, questioning the objectives that such a machine might pursue. It concludes with a reflection on the potential and challenges of developing AI that could significantly surpass current models.
Mindmap
Keywords
💡Q Learning
💡A* Search
💡Q* (Q Star)
💡Deep Learning
💡Reinforcement Learning
💡Artificial Intelligence (AI)
💡 Generative Models
💡Heuristic Techniques
💡Dynamic Knowledge
💡Efficiency
💡Bias and Prejudice
Highlights
The discussion revolves around the recent developments in artificial intelligence, particularly focusing on a project named Qstar.
Qstar is a combination of two deep learning techniques, Q Learning and A Star Search algorithm.
Q Learning is a reinforcement-based training technique for neural networks, akin to training an animal with rewards and penalties.
The Q Learning algorithm uses a Q-table to estimate the probability of actions leading to a goal in a problem space, such as a labyrinth.
A Star Search algorithm is focused on finding the shortest path between two points in a search space, using heuristic techniques.
The combination of Q Learning and A Star Search results in a highly efficient problem-solving model, surpassing previous models in its dynamic approach.
Current generative models like GPT-4 rely on vast amounts of high-quality data, which poses challenges in terms of data management and bias.
Qstar is expected to overcome the limitations of static knowledge and continuous learning by integrating Q Learning and A Star Search algorithms.
The new model will have dynamic knowledge, constantly updating and learning from new data, improving its goal-reaching capabilities.
Qstar aims to provide a more comprehensive understanding of context, as it will have access to nearly all human knowledge.
The innovative approach of Qstar is a significant departure from current models, which are data-dependent and static.
The concern is raised that while the machine may become highly efficient, it may not develop consciousness like humans.
The ethical implications of creating machines that are solely goal-oriented without empathy or consciousness are discussed.
The video concludes with a reflection on the objectives and potential ethical considerations of developing such advanced AI systems.
Qstar is expected to be integrated into the next generation model, possibly GPT-5, offering a new era of AI capabilities.
The video emphasizes the importance of addressing the potential risks and ensuring that the objectives of such AI align with human values.
The discussion highlights the transformative potential of Qstar in the field of artificial intelligence, marking a significant leap from current technologies.
The video invites viewers to consider the broader implications of creating AI that is highly efficient but lacks human-like consciousness and empathy.
Transcripts
Ciao ragazzi nell'ultimo video quello
realizzato con wyner writen Praticamente
l'ha scritto l'ha letto e l'ha anche
disegnato io l'ho solo montato abbiamo
parlato del licenziamento di Sam Altman
e di questo progetto qstar che sarebbe
stato rivelato da alcune fonti
dell'agenzia
Reuters ora tutti stanno parlando di
qstar ma oggettivamente nessuno ha
capito che cos'è allora cerchiamo Camo
di spiegare un po' Che cos'è questo Q
Star E perché genera da una parte così
tanto entusiasmo dall'altra così tanta
preoccupazione partiamo dal nome per
quanto si si possa supporre Q Star
deriva dall'accostamento dalla
combinazione di due tecniche di Deep
Learning di due tecniche che diciamo si
stanno utilizzando nell'intelligenza
artificiale La prima è il que learning e
l'altra è l'a Star Search è un algoritmo
si chiama a star Search ora Che cos'è il
Q Learning Q Learning è una tecnica di
addestramento per reti neurali basata
sul rinforzo e sulla penalità ma diciamo
principalmente sul rinforzo è un po'
Come addestrare un animale un po' quello
che facciamo peraltro è una tecnica che
risale a alla psicologia degli anni 50
eh l'apprendimento
rinforzato diciamo è stata applicata
ancora una volta vengono presi dei
concetti dalla psicologia e vengono
applicati alle macchine perché stiamo
cercando di creare una psicologia dentro
queste macchine no chissà se poi forse
questo è anche un nostro bias che non
conoscendo altro tipo di di tecniche
utilizziamo quelle che si utilizzano con
l'essere umano comunque chiusa parentesi
è un po' Come addestrare un animale ok
Un cagnolino per esempio che cosa
facciamo noi per addestrare il nostro
Pet dentro casa Quando il cagnolino fa
una cosa giusta gli diamo un premio gli
diamo il biscottino questo Noi possiamo
farlo anche con le macchine Il Q
Learning è questa tecnica di
apprendimento rinforzato per capire la
quale possiamo immaginarci un agente si
chiama così cioè quindi un qualcuno che
deve agire ok Un decisore Mh all'interno
di un ambiente che potrebbe essere un
videogioco una mappa e Eh il nostro
agente deve imparare a muoversi dentro
questa mappa per raggiungere dei
traguardi degli obiettivi Ad esempio
immaginiamo di mettere un agente in un
labirinto Quindi l'obiettivo è uscire
dal labirinto mettiamo al centro del
labirinto è circondato dal labirinto e
deve in qualche modo uscire dal
labirinto come ragiona una macchina Eh
che funziona con il meccanismo del Q
Learning ci sono delle azioni che che
possono essere fatte ogni azione parte
da uno stato e deve raggiungere uno
stato successivo cioè all'interno di uno
spazio problemico ci sono degli Stati
che avvicinano alla soluzione del
problema Ok quindi all'inizio il nostro
agente parte che sta al al primo stato
quindi la la sol lo stato più lontano
dalla soluzione e il suo obiettivo è
passare allo stato successivo quindi
avvicinarsi verso la soluzione del
problema per cui lui si fa una tabella
che si chiama tabella Q Mh si fa una
tabella dove ci sono eh tutte le azioni
che può compiere e gli stati che può
raggiungere fino alla soluzione Mh e eh
l'incrocio di questa di questa tabella
di questi valori è una stima
probabilistica di quanto quella
determinata azione avvicini al traguardo
inizialmente la gente
posto al centro del del del labirinto
parte casualmente cioè diciamo Popola
questa tabella con valori casuali non ha
alcun elemento su cui basare le proprie
decisioni all'inizio quindi parte
casualmente per cui Supponiamo non so
l'azione di camminare dritto Con quanta
probabilità mi fa passare allo stato
successivo gli assegna una probabilità
non so del 10% l'azione di camminare
verso destra Con quanta probabilità mi
avvicino all'obiettivo quella di
camminare verso sinistra quella di
andare indietro e così via ok Quindi lui
Popola questa tabella e inizia per
esempio va dritto va dritto sbatte
contro il muro la tabella si aggiorna e
la percentuale scende a zero quindi
andare dritto a questo stato allo stato
più lontano quindi No All'inizio non
serve allora Adesso proviamo ad andare a
destra vado a destra bom sbatto contro
un muro di nuovo di nuovo la la la
probabilità scende e e viene
riaggiornato la tabella una
particolarità di questa di questo
algoritmo che aggiorna la tabella perché
viene aggiornato attraverso una formula
matematica è che lui non Calcola solo
diciamo la ricompensa o la punizione Ma
la formula matematica che è stata creata
all'interno di questo sistema Q Learning
permette alla macchina di fare anche una
stima di lungo termine cioè di quanto è
probabile che però in futuro questa
stessa azione invece porti a una eh a un
un risultato diverso Naturalmente il
nostro agente alla fine esce dal
labirinto Quindi lui Aggiorna questa sua
matrice di tabelle Q per ogni azione
quale risultato ha portato Aggiorna
questa sua matrice Diciamo che per il
labirinto 1 le cose sono andate così per
cui lui ha un un quadro statistico di di
tutto quello che poteva fare e di come
sono andate le cose man mano che le
faceva quelle azioni immaginate che
questa stessa macchina viene posta
davanti a un milione di labirinti questa
macchina diventa estremamente efficace
nella nell'uscire dal labirinto nel nel
prendere le decisioni a un certo punto
si vede che queste intelligenze
artificiali arrivano a dei livelli
altissimi di efficienza nel prendere le
decisioni soprattutto in un'ottica di
lungo termine cioè basandosi quindi
sull'esperienza che hanno avuto degli
altri milioni di labirinti che hanno che
hanno che hanno risolto e quindi
riescono pure a prevedere Ok come
potrebbe essere la soluzione più veloce
per i labirinti successivi naturalmente
non è solo il labirinto il labirinto è
una una un'idea no può essere una mappa
un videogioco ripeto ma può essere anche
uno spazio problemico quindi astratto Ok
non per forza quindi questa cosa deve
essere applicata allo spazio No ma può
essere anche una cosa più astratta un
idea un concetto più astratto come
risolvo un determinato problema fosse
anche semantico Allora mi faccio la
tabella q e essendo sottoposto a milioni
di problemi semantici divento un
decisore semantico molto avanzato e
questo è il Q
Learning poi c'è l'algoritmo di ricerca
aar e è una cosa diciamo simile perché è
basata sul trovare il percorso più breve
tra due punti in uno spazio di ricerca è
una cosa simile Però è focalizzata sul
trovare proprio il percorso più breve
tra due punti per cui se tu in mezzo ci
metti il labirinto per esempio l'entrata
e l'uscita no del labirinto Il Q
Learning insieme al all'algoritmo di
ricerca e Star diventano di
un'efficienza
assoluta nella risoluzione dei problemi
per assoluta intendo che al momento non
abbiamo mai hai visto niente del genere
nel nostro passato tanto che addirittura
la combinazione di queste due di queste
due tecniche preoccupano preoccupano
peraltro va detto che lei Star Search fa
anche uso di tecniche euristiche che
sono proprio delle Stime Ok delle Stime
intelligenti per cui diciamo l'aggiunta
della della Tecnica di apprendimento per
rinforzo del Q learning insieme
all'algoritmo a star che fa utilizzo di
tecniche appunto euristiche nell'insieme
questo nuovo modello generativo potrebbe
essere questo qstar di cui si stanno
occupando Nei laboratori di Open ai ora
Qual è L'innovazione di tutto questo
sistema rispetto ai modelli generativi
che abbiamo oggi come GPT 4 Beh Voi
capite che è un approccio completamente
diverso completamente diverso i modelli
che abbiamo Oggi infatti sono basati su
una gigantesca quantità di dati la
capacità che il modello generativo
attuale ha di essere
efficiente dipende tutto dalla qualità
dei dati che deve essere una quantità
enorme e di ottima qualità Inoltre i
modelli attuali hanno il problema della
staticità della conoscenza cioè Hanno
una conoscenza statica come abbiamo
visto chat GPT All'inizio era aggiornato
al settembre del 2021 poi l'hanno
aggiornato al 22 adesso è aggiornato se
non sbaglio ad aprile del 23 stiamo
registrando questo video alla fine di
novembre
2023 poi facciamo anche magari un
discorso in un altro video su che cosa
significa il fatto che siano connessi ad
internet che abbiano accesso a
determinate informazioni probabilmente
lo state provando voi stesso non è la
stessa cosa una cosa è la conoscenza che
il modello ha a disposizione interamente
a disposizione nel nella nella sua
matrice tridimensionale probabilistica
che gli permette di rispondere
immediatamente una cosa è quando il
modello non ha quella conoscenza quindi
deve accedere ad una risorsa esterna
andarsi a cercare una risposta
elaborarla portarla dentro è un
procedimento Scusate completamente
diverso e non garantisce lo stesso tipo
di risultato per cui la conoscenza
statica dei modelli generativi attuali è
un problema è un problema e poi c'è un
problema di bias e pregiudizi il
problema dei bias e dei pregiudizi è
duplice perché non solo questi
pregiudizi stanno incapsulati
incorporati nei dati la qualità dei dati
è importante anche per quello no cercare
dei dati che siano anche da da questo
punto di vista qualitativamente alti
dicevo è duplice perché non c'è solo il
problema nei dati ma c'è anche il
problema nei programmatori cioè nel team
che poi gestisce quei dati e li aggiusta
e diciamo dà un un indirizzo a questi
modelli generativi per cui tutto questo
oggi È meraviglioso però insomma ha
anche queste criticità ha anche questi
limiti Ecco con il nuovo approccio che
presumibilmente Ci aspettiamo sia
integrato in g pt5 che stiamo aspettando
probabilmente l'anno prossimo tutto
questo potrebbe essere superato perché
se il modello di apprendimento e la e di
ricerca sarà questo Q Star molto
probabilmente integrerà tutte le
capacità che vi ho detto prima e questo
supera tutti questi problemi perché a
quel punto il modello avrà Innanzitutto
una conoscenza dinamica il che significa
che è in continuo aggiornamento sempre
ok e cioè non c'è più il problema
dell'addestramento prima poi hai il
modello e poi Proponi il modello al
mercato Il problema è che quel modello
sarà in continuo addestramento avrà
questa capacità continua di esplorare lo
spazio problemico e di apprendere e di
quindi migliorare sempre di più la sua
capacità di raggiungere gli obiettivi e
inoltre ci sarà anche una maggiore
estremamente Maggiore capacità di
comprendere il contesto Perché a quel
punto la la conoscenza del modello
potrebbe essere pressoché totale perché
è la tutta la conoscenza dell'essere
umano che a questo nuovo modello qstar
sarà sempre disponibile ok non dovrà
accedere a risorse esterne ci avrà avrà
sempre tutta la conoscenza disponibile
aggiornata e quindi Voi capite che
questo è un approccio completamente
diverso ora per concludere questo video
credo di avervi detto quasi tutto però
volevo concludere
con una riflessione da una parte c'è il
problema del fatto che questo modello
potrebbe diventare Senziente Io non
credo è la mia opinione me ne prendo la
responsabilità però io non credo che la
coscienza possa diciamo risolversi in
questo algoritmo Io credo assolutamente
che la macchina possa in un futuro
diventare cosciente in maniera del tutto
paragonabile all'essere umano ma non mi
sembra questo l'approccio Il problema è
che è ancora più pericoloso perché la la
coscienza tutto sommato ha anche degli
aspetti positivi Cioè se questa macchina
diventasse cosciente Magari avrebbe pure
un barlume di empatia Magari avrebbe
pure No un po' di ragionevolezza la
macchina che stanno creando invece con
questo Q Star ed è questo che ha
generato il polverone di cui stiamo
parlando e che è difficile da divulgare
è difficile da spiegare io ci sto
provando e è il proprio il problema
opposto cioè questa macchina non è
cosciente non è empatica va dritta per
il suo obiettivo e la stiamo creando in
maniera tale che sia assolutamente
efficiente nel raggiungere i suoi
obiettivi una macchina che fosse
cosciente rifacciamoci alla fantascienza
no fate voi quella che preferite no
trovate sempre magari un data di Star
Trek o non so una qualche altra macchina
che se comunque è È simile all'essere
umano Magari ci si può anche ragionare
ma una macchina di questo tipo è una è
una macchina efficiente nel
raggiungimento dei suoi obiettivi No
Matter What Quindi qua Il problema è
quali saranno i suoi obiettivi Questa è
la Reale riflessione che ci dobbiamo
porre nella realizzazione di questa
meraviglia Io con questo ho concluso
Grazie per essere stati con me e ci
vediamo al prossimo video
ragazzi
n
Browse More Related Video

Claude DISABLES GUARDRAILS, Jailbreaks Gemini Agents, builds "ROGUE HIVEMIND"... can this be real?
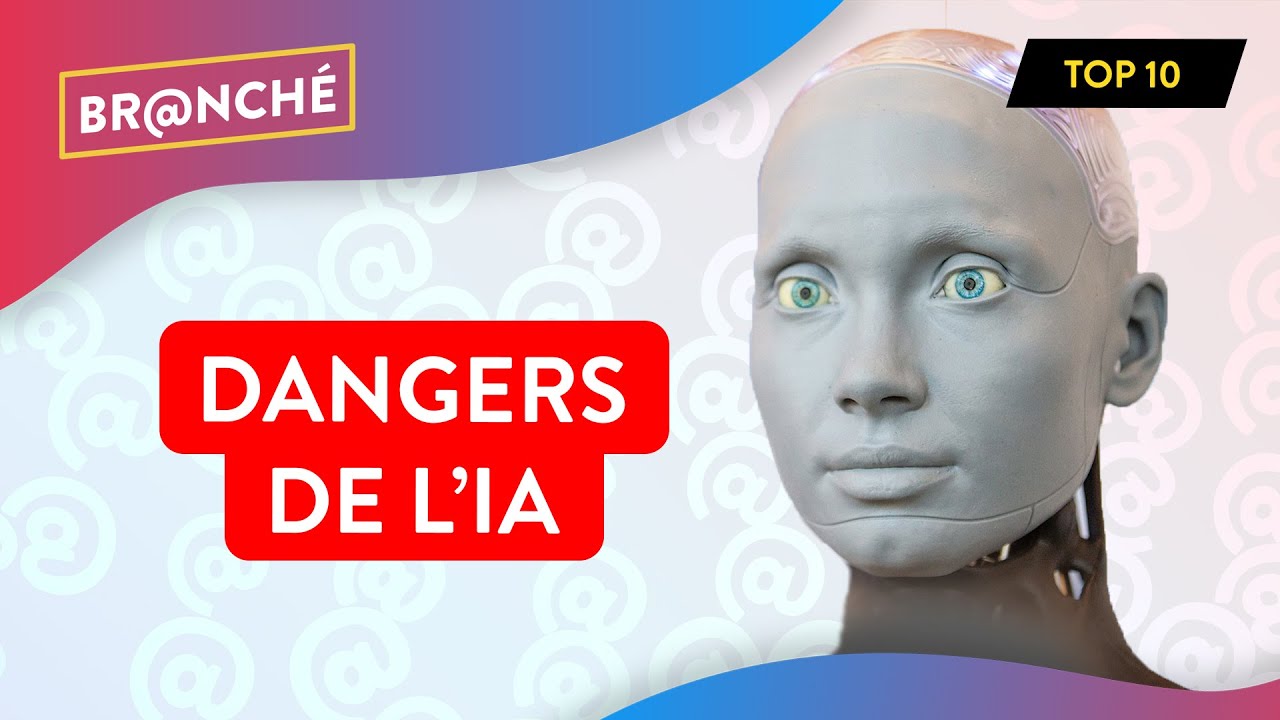
Les 10 dangers de l'intelligence artificielle
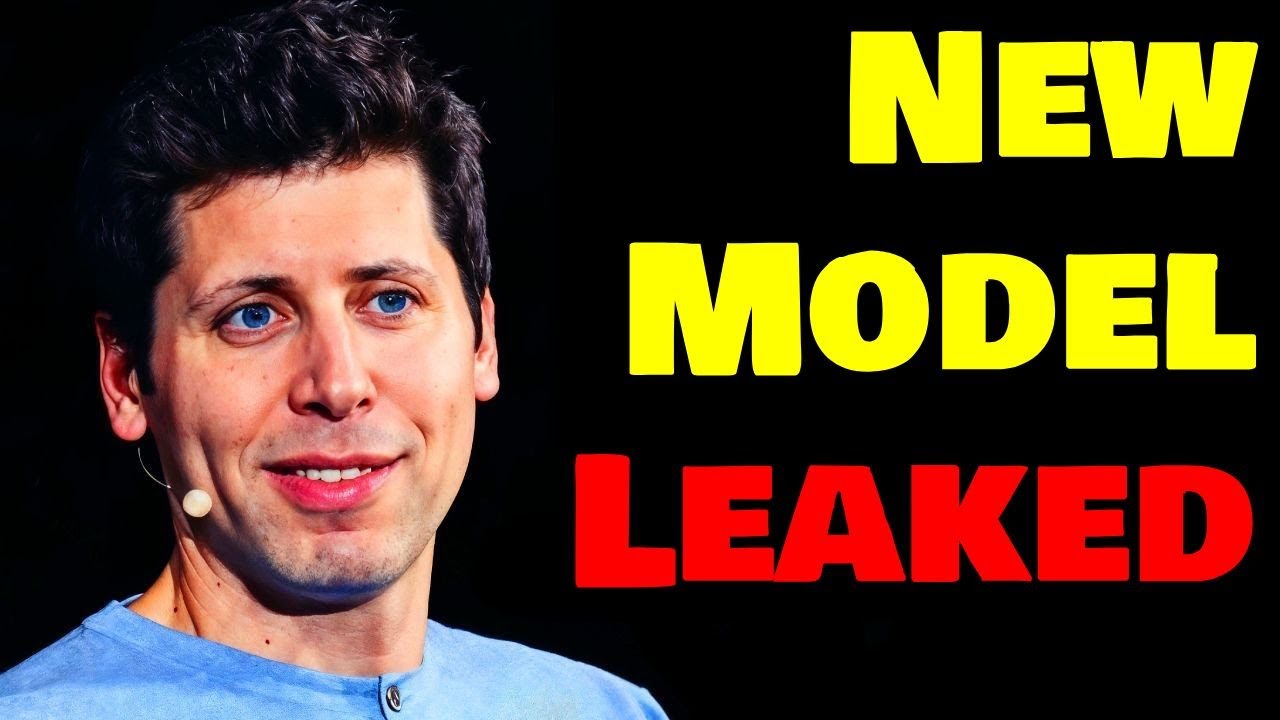
OpenAI's New Model Releases LEAKED | Sam Altman talks about AGI, UBI, GPT-5 and what Agents will be
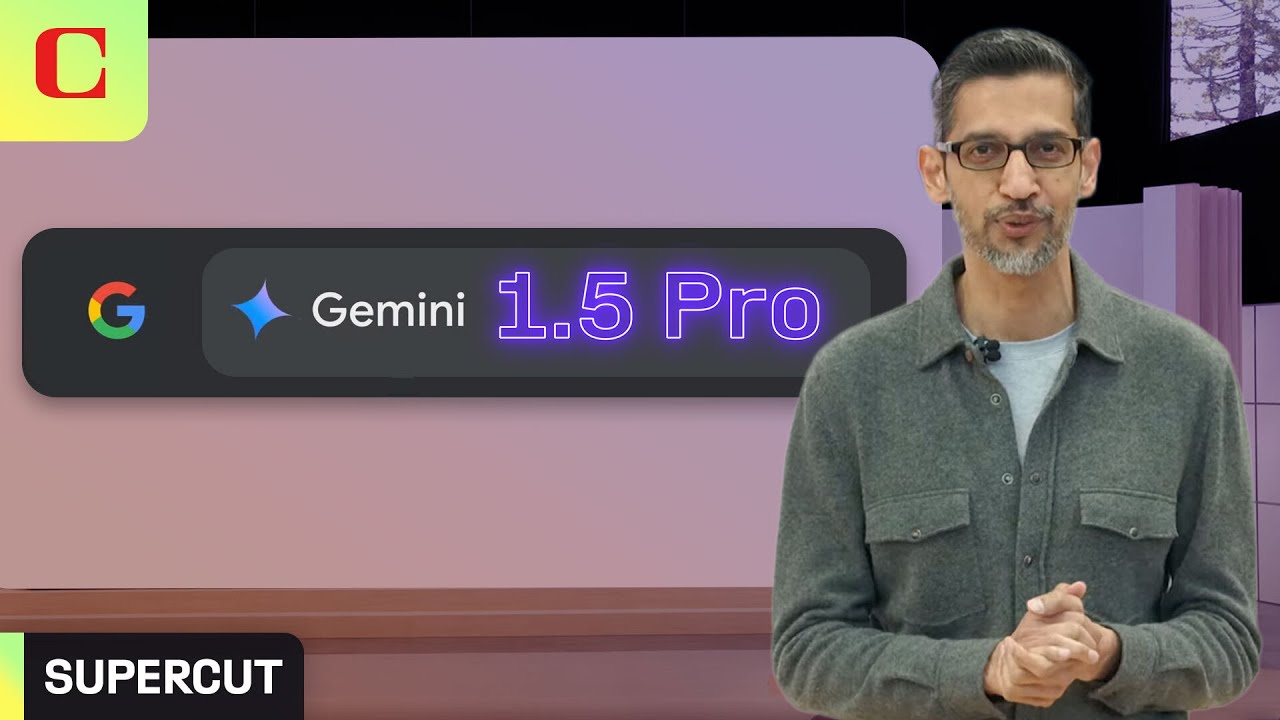
Google I/O 2024: Everything Revealed in 12 Minutes
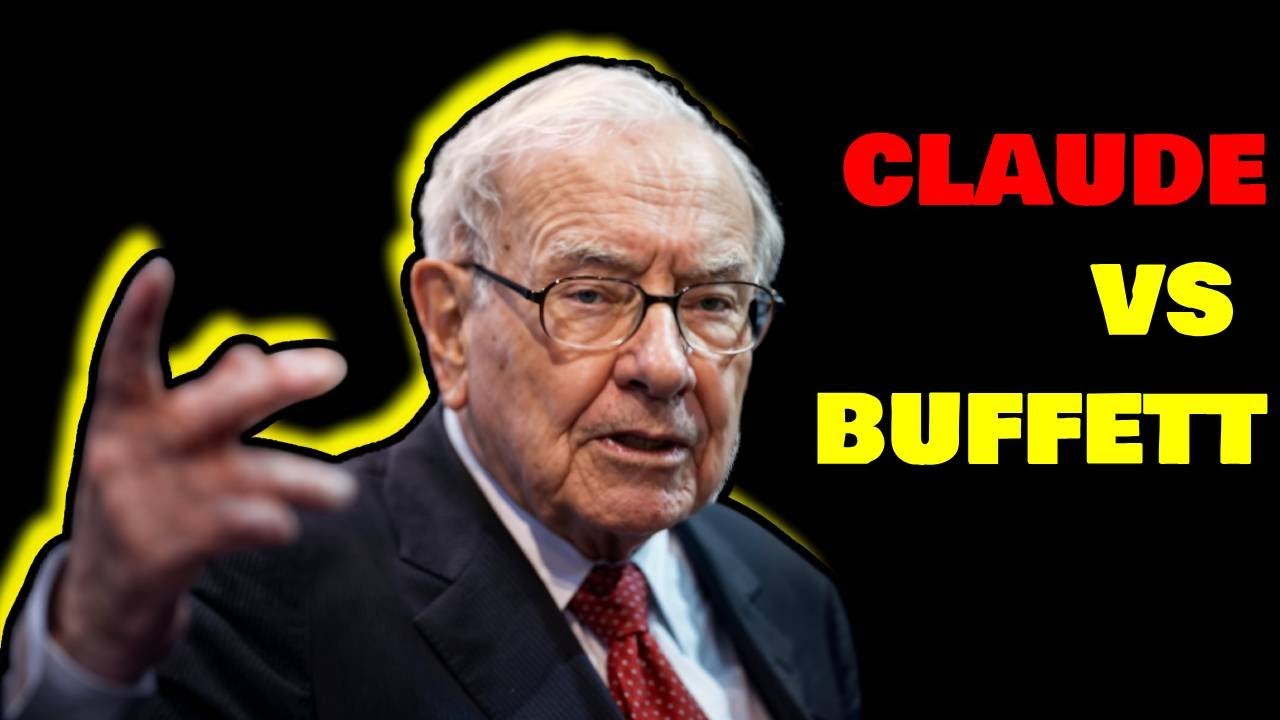
Will "Claude Investor" DOMINATE the Future of Investment Research?" - AI Agent Proliferation Begins
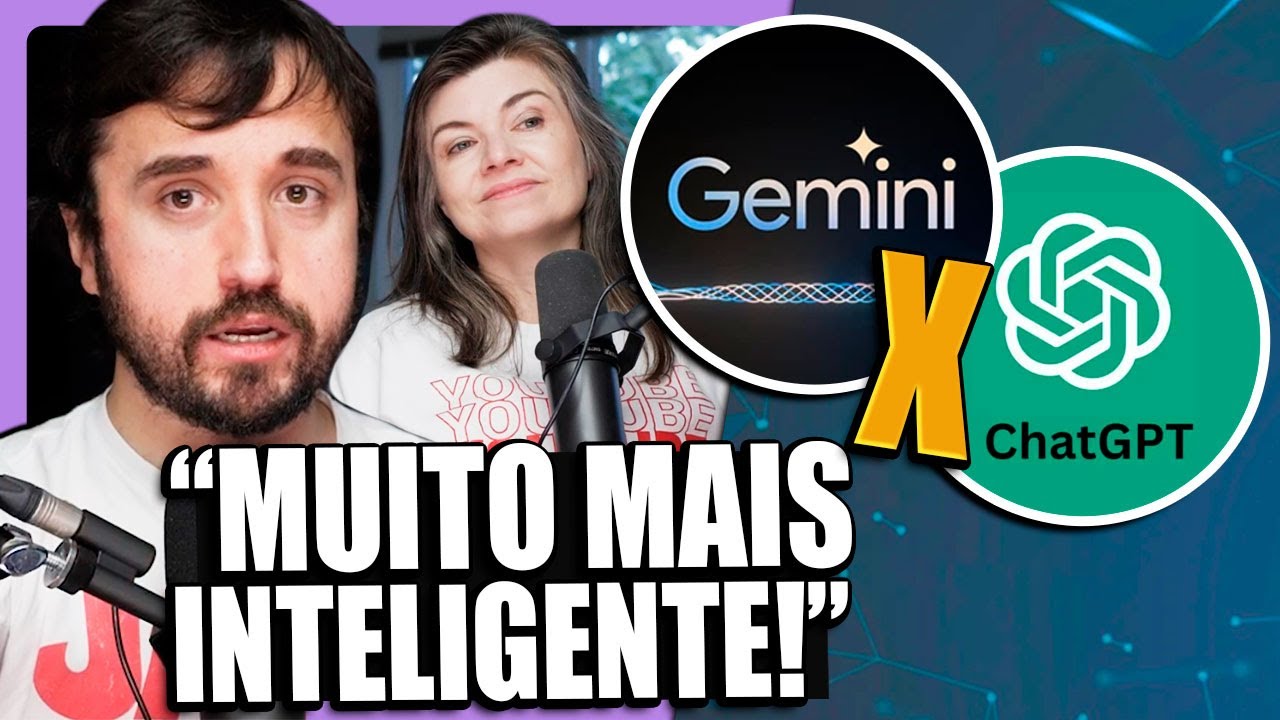
BATALHA de INTELIGÊNCIA ARTIFICIAL! - Gemini | ChatGPT-4o
5.0 / 5 (0 votes)