Teach Algorithms To Give You MILLIONS OF STREAMS While You Sleep
Summary
TLDRThis video script offers musicians insight into how algorithms on platforms like Instagram and Spotify work, explaining user modeling and music genome analysis. It advises artists to understand these algorithms to grow their audience, suggesting strategies like engaging with similar artists, using effective hashtags, and collaborating to build algorithmic connections. The script also touches on the importance of recency in connections and the power of features and collaborations for algorithmic growth.
Takeaways
- 🎶 Algorithms on platforms like Instagram and Spotify recommend artists based on user modeling and music analysis.
- 🔍 User modeling involves creating profiles of users and their interests to suggest similar artists.
- 🎵 Music genomes analyze songs for sound and rate them on various markers to match user moods and preferences.
- 🚫 'Algorithmic jail' refers to being stuck without algorithmic recommendations due to lack of user engagement or connections.
- 🔗 Building connections with other artists through collaborations, features, and remixes can boost algorithmic visibility.
- 📈 Regularly engaging with similar artists and using relevant hashtags can signal to algorithms that you belong to a particular community.
- 📊 Recency of connections matters; consistently making new connections helps maintain algorithmic relevance.
- 📈 Clout bombing, where multiple artists are featured together, can create a surge in algorithmic connections and fan engagement.
- 🎧 Platforms analyze user behavior to recommend artists, so engaging with content from similar artists can influence recommendations.
- 📝 Understanding your micro-genre and niche is crucial for effectively targeting and connecting with the right audience and artists.
Q & A
What is 'algorithmic jail' mentioned in the script?
-Algorithmic jail refers to the situation where an artist's music is not being recommended or discovered by algorithms on platforms like Instagram, TikTok, and Spotify, despite the artist creating good music and engaging content.
How do algorithms recommend artists to users?
-Algorithms recommend artists through two main methods: user modeling, where they create profiles of users and their interests to suggest similar artists, and music genomes, which analyze songs for sound and rate them on various markers to match with users' moods and preferences.
Why is it important for musicians to understand how algorithms work?
-Understanding algorithms is important for musicians because it helps them strategize how to be discovered by more users. It allows them to create content that aligns with what algorithms look for in terms of user engagement and music characteristics.
What is the role of hashtags in helping an artist's music get discovered?
-Hashtags play a crucial role in helping an artist's music get discovered as they provide context to algorithms about the content and its potential audience. Using relevant and popular hashtags can increase the visibility of an artist's content within the algorithm.
Why should musicians collaborate with other artists?
-Collaborating with other artists helps musicians create stronger algorithmic connections. When artists collaborate, they appear on each other's pages, which can lead to mutual fans and increased recommendations by the algorithm.
How can musicians build connections with other artists on platforms?
-Musicians can build connections by engaging with similar artists on social media, using relevant hashtags, collaborating on projects, and participating in community activities. This engagement signals to algorithms that the artists are part of the same community.
What is the significance of 'clout bombing' in the context of algorithmic growth?
-Clout bombing is when multiple artists or influencers come together in a single post or event, which can lead to increased sharing and tagging, thus deepening algorithmic connections and potentially ramping up new followers for all involved.
How can musicians ensure their music is recommended in algorithmic playlists?
-To be recommended in algorithmic playlists, musicians should focus on creating music that fits well within specific moods or themes, collaborate with popular artists, and consistently engage with their community to build strong algorithmic ties.
What is the impact of recency on algorithmic recommendations?
-Recency matters because algorithms prioritize recent connections and interactions. Artists need to continually create new content and engage with their community to maintain and grow their presence in algorithmic recommendations.
How can musicians leverage their presence on short-form platforms like TikTok?
-Musicians can leverage their presence on short-form platforms by creating engaging content that includes their music, using trending hashtags, and interacting with other artists and users. This helps in building a strong community and getting recommended by algorithms.
Outlines
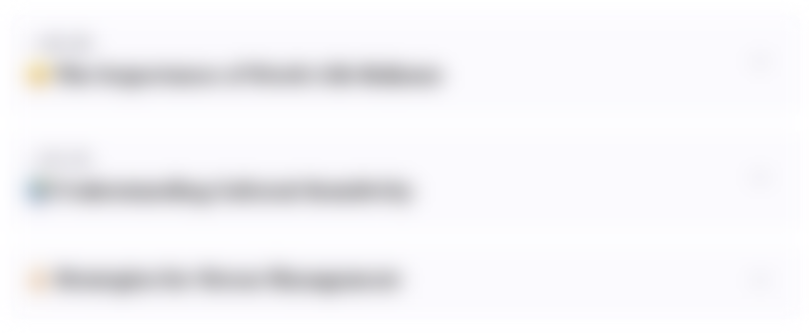
This section is available to paid users only. Please upgrade to access this part.
Upgrade NowMindmap
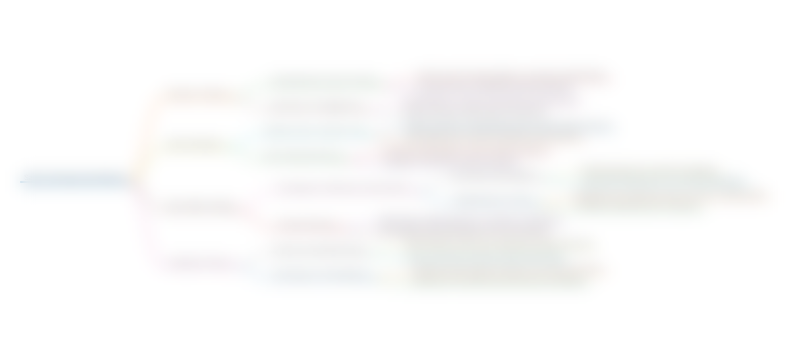
This section is available to paid users only. Please upgrade to access this part.
Upgrade NowKeywords
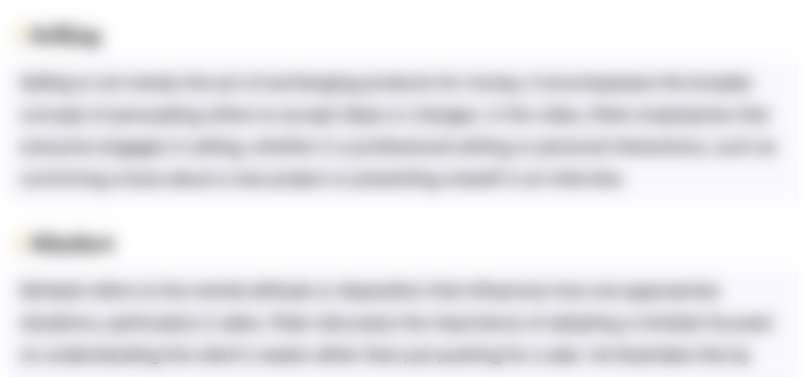
This section is available to paid users only. Please upgrade to access this part.
Upgrade NowHighlights
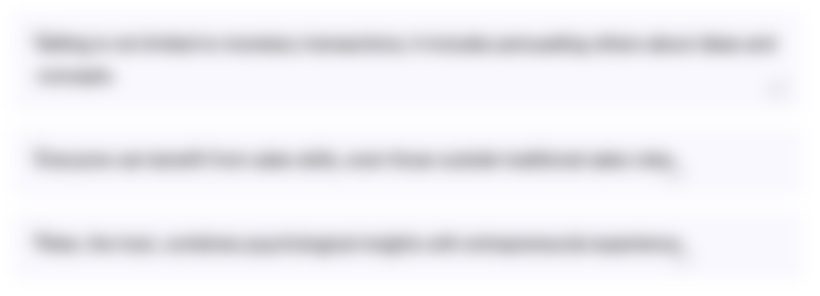
This section is available to paid users only. Please upgrade to access this part.
Upgrade NowTranscripts
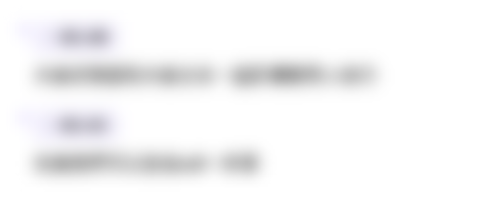
This section is available to paid users only. Please upgrade to access this part.
Upgrade NowBrowse More Related Video
5.0 / 5 (0 votes)