Truth in Data Science | Jaya Tripathi | TEDxYouth@BHS
Summary
TLDRJaya Tripathi, a principal scientist at Mitel, discusses her role as a data scientist, emphasizing the interdisciplinary nature of the field. She highlights the importance of domain expertise, statistical methods, and machine learning in extracting knowledge from data. Tripathi shares her experience with hypothesis testing in medical research, where she predicts prescription fraud by analyzing data from the Prescription Drug Monitoring Program. She also explores data through visualization techniques like histograms, clustering, and geospatial analysis, leading to significant findings such as the identification of potential fraud and the prediction of an HIV epidemic in Indiana. She concludes by advocating for passion in research, the importance of asking questions, and the scientific method in data analysis.
Takeaways
- 🔬 Jaya Tripathi is a principal scientist at Mitel, focusing on data science and innovation programs.
- 📊 Data science involves interdisciplinary approaches, including domain expertise, math, statistics, visualization, and graph analytics.
- 🔍 The concept of absolute truth does not exist in data science, especially in medical research, due to the variability of observable properties.
- ⚖️ Hypothesis testing is a key method in scientific research, where one begins with a hypothesis, makes assumptions, and tests them through models and data analysis.
- 💊 Tripathi's research involved predicting prescription fraud by analyzing data elements like travel distance and drug combinations.
- 📈 The CRISP-DM process was used for data mining, emphasizing the importance of understanding and preparing data before modeling.
- 📊 Visualization techniques, such as histograms and clustering, were used to explore data and identify patterns that could inform machine learning models.
- 🗺 Geospatial analysis, including heat maps, was used to identify hotspots for drug use, which led to significant findings and interventions.
- 🔎 The importance of not just accepting the obvious explanation in data analysis was highlighted, encouraging deeper exploration to uncover underlying issues.
- 📚 Tripathi suggests further reading on the Simpsons paradox, UC-Berkeley gender bias study, and the man in the cave allegory to understand data science concepts better.
Q & A
What is Jaya Tripathi's profession and where does she work?
-Jaya Tripathi is a Principal Scientist at Mitel Corporation in Bedford, Massachusetts.
What is the purpose of the innovation program at Mitel Corporation?
-The innovation program at Mitel Corporation is an internal research program that fosters creativity and is funded to support research initiatives.
What does Jaya Tripathi consider as the 'sexiest job' of the 21st century?
-Jaya Tripathi refers to a quote from the Harvard Business Review, which states that a data scientist has the 'sexiest job' of the 21st century.
What interdisciplinary techniques does a data scientist like Jaya Tripathi use?
-Data scientists use a combination of domain expertise, math, statistics, visualization techniques, and graph analytics, among other scientific methods and algorithms, to extract knowledge from data.
Why does Jaya Tripathi believe the concept of absolute truth does not exist in data science, particularly in medical research?
-Jaya Tripathi explains that in medical research, data is based on observable properties that can vary over time or across different data sets, and the concept of absolute truth can introduce biases, which contradicts the scientific method.
What is the significance of hypothesis testing in Jaya Tripathi's research?
-Hypothesis testing is a method of scientific research where Jaya Tripathi starts with a question, makes assumptions, and creates tests to validate those assumptions. It helps in building models and determining the confidence of results, leading to the acceptance or rejection of initial assumptions.
Can you explain the CRISP-DM process Jaya Tripathi mentioned for data mining?
-The CRISP-DM process is a cross-industry standard for data mining that Jaya Tripathi follows. It involves understanding the data, preparing it by removing duplicates and correcting errors, modeling, and finally evaluating and validating the results.
What was Jaya Tripathi's hypothesis regarding prescription fraud by physicians?
-Jaya Tripathi hypothesized that she could predict prescription fraud by physicians based on data elements such as the distance traveled by the person, certain combinations of drugs prescribed, and other related factors.
How did Jaya Tripathi use geospatial analysis in her research?
-Jaya Tripathi used geospatial analysis, specifically heat maps, to visualize data on drug distribution and usage across different regions. This helped in identifying hotspots for further investigation, such as the HIV epidemic in Scott County, Indiana.
What is Jaya Tripathi's advice for conducting good research?
-Jaya Tripathi suggests that one must be passionate about the research topic, make observations, ask interesting questions, formulate hypotheses, gather the correct data, test predictions, and generalize findings with other datasets.
Outlines
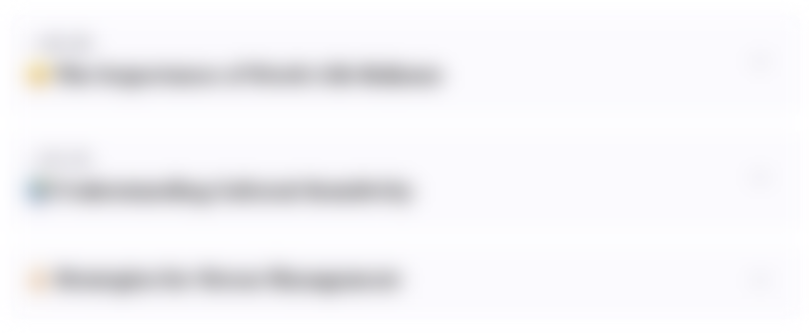
This section is available to paid users only. Please upgrade to access this part.
Upgrade NowMindmap
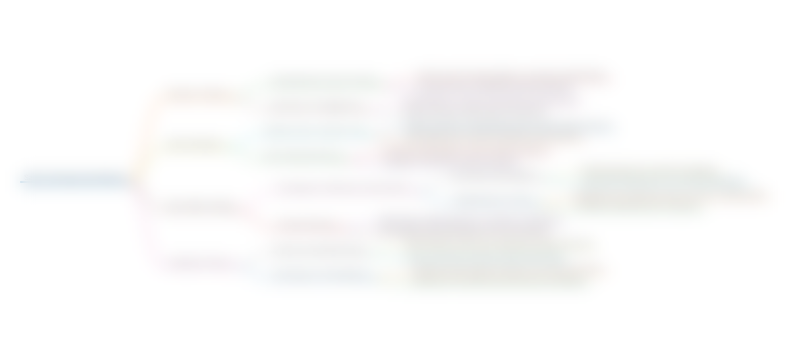
This section is available to paid users only. Please upgrade to access this part.
Upgrade NowKeywords
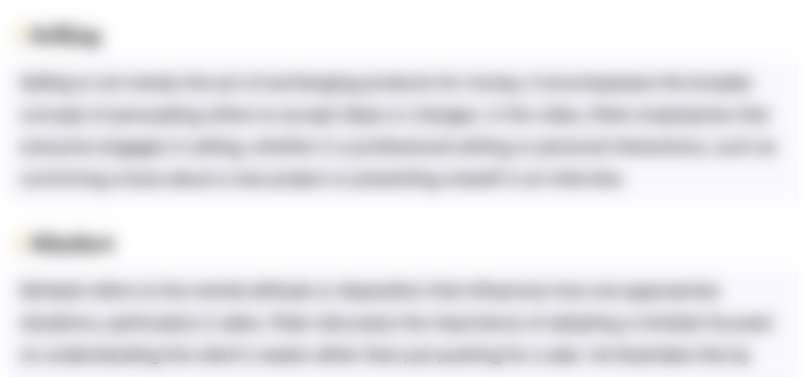
This section is available to paid users only. Please upgrade to access this part.
Upgrade NowHighlights
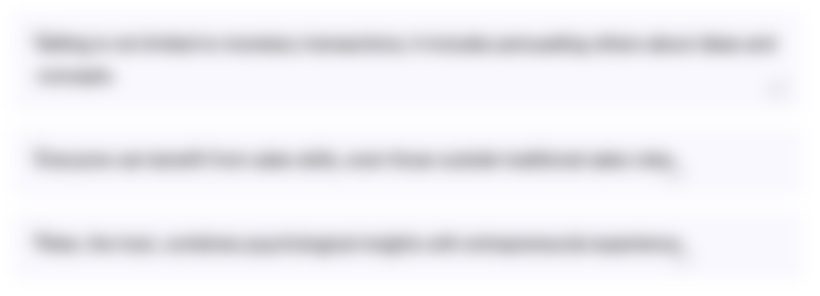
This section is available to paid users only. Please upgrade to access this part.
Upgrade NowTranscripts
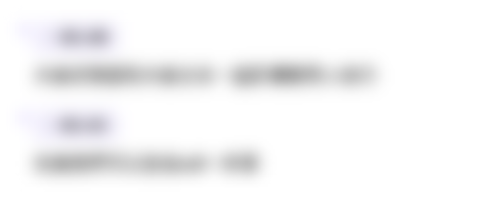
This section is available to paid users only. Please upgrade to access this part.
Upgrade NowBrowse More Related Video
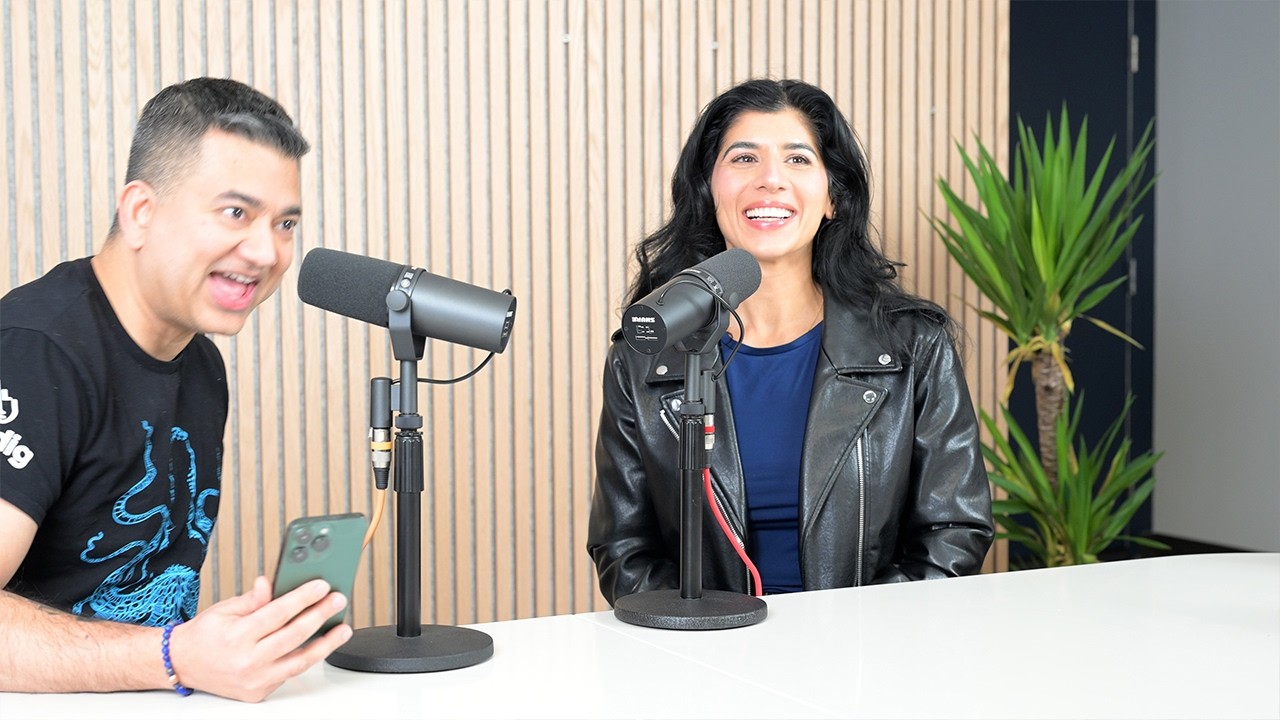
Should You Be A Data Analyst or Data Engineer in 2025?
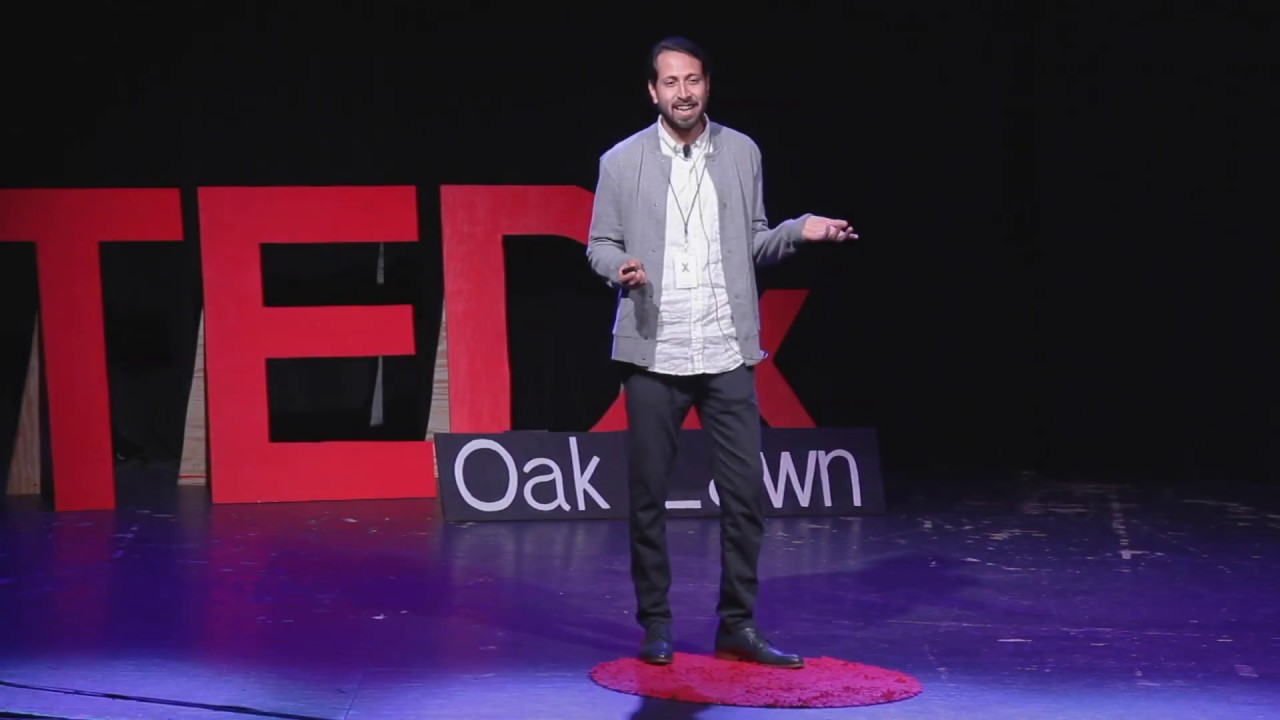
Demystifying Data Science | Mr.Asitang Mishra | TEDxOakLawn
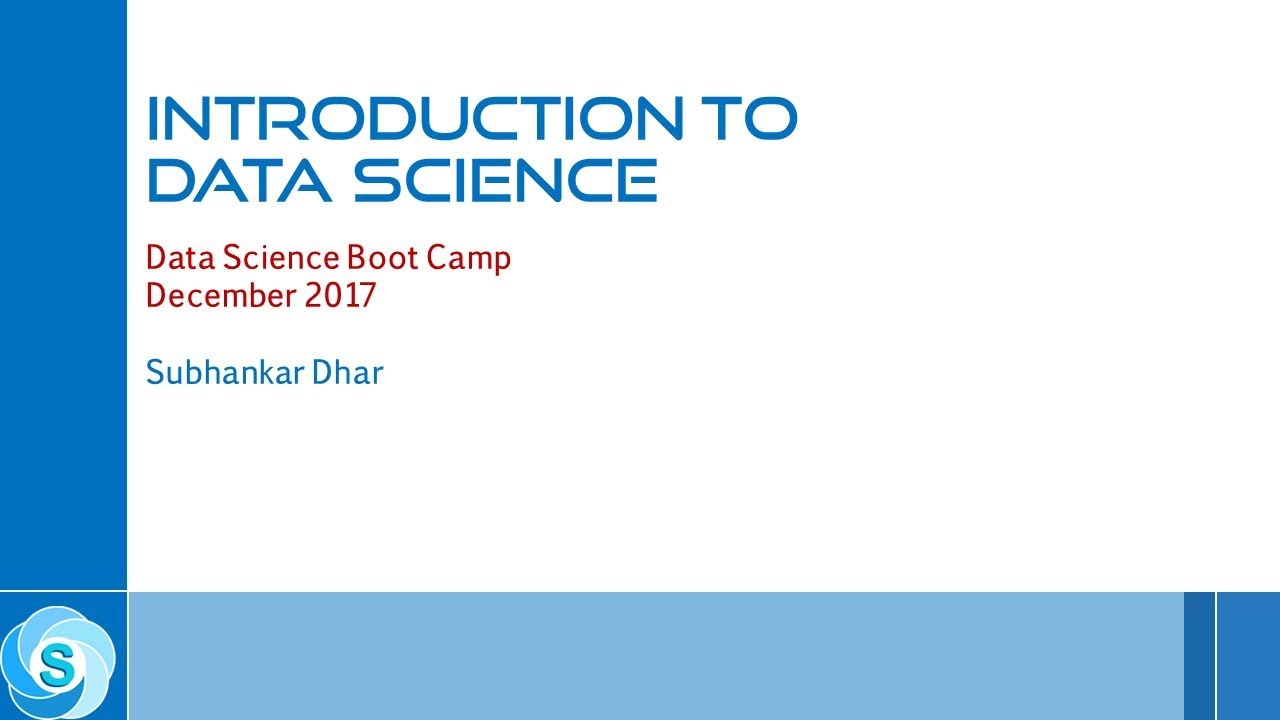
Introduction to Data Science - Fundamental Concepts

4 Skills You Need to Be a Full-Stack Data Scientist
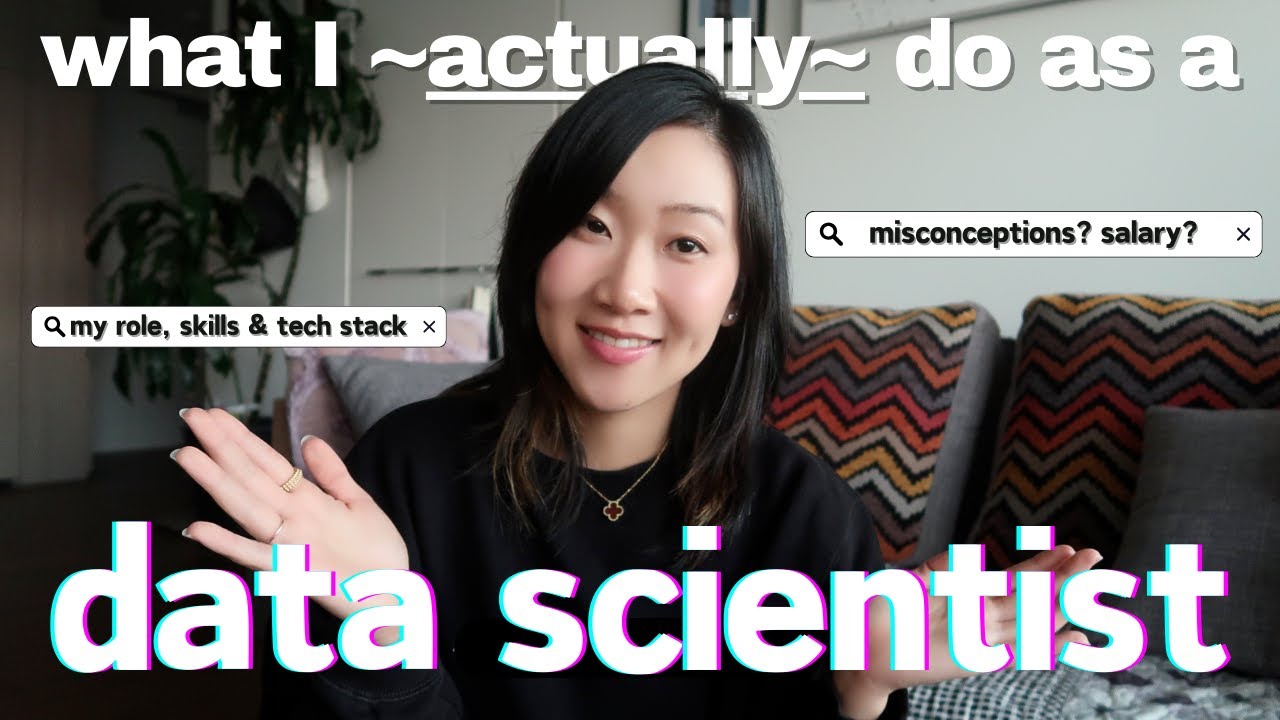
What I *actually* do as a Data Scientist in 2024 (everything you need to know)
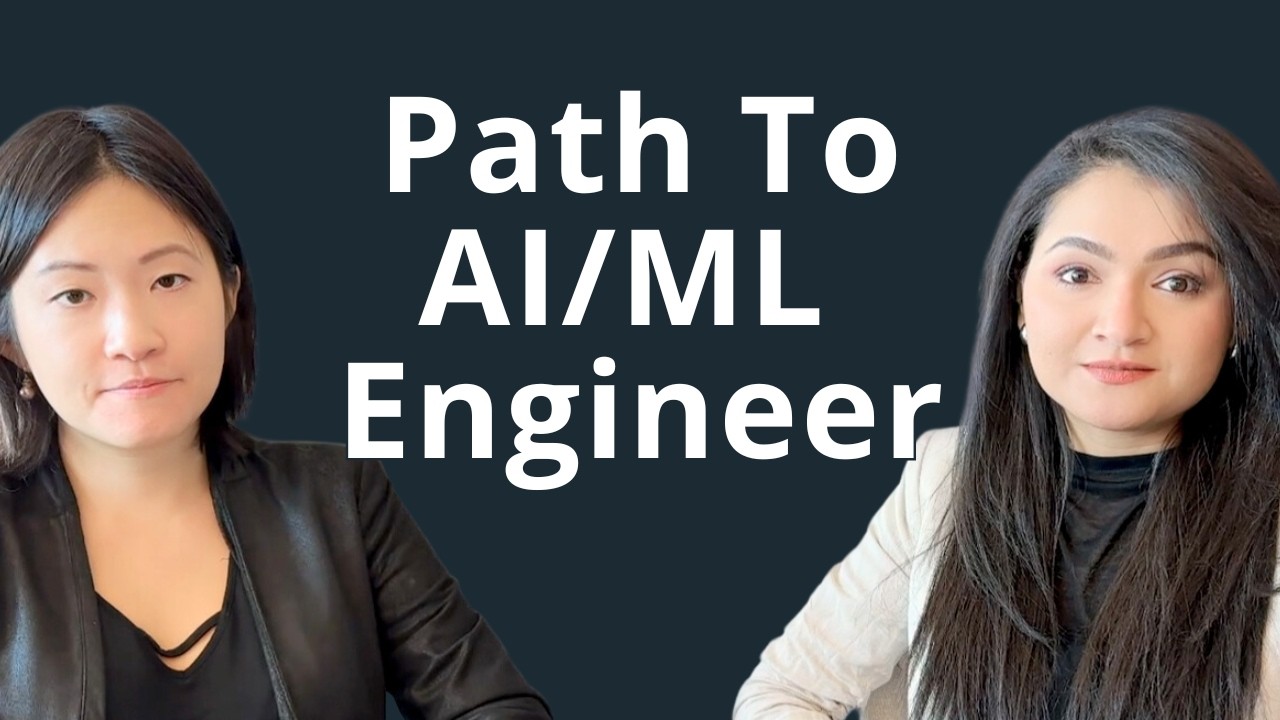
Advice From a Top 1% Machine Learning Engineer
5.0 / 5 (0 votes)