Navigating the Clinical AI landscape
Summary
TLDRIn this dynamic webinar, hosted by Endpoints News and sponsored by Mendle, we delve into the evolving landscape of clinical AI and its pivotal role in advancing precision medicine. The discussion features insights from Mendle's co-founder and CEO, Karim Galil, and Unlearn AI's founder, Charles Fisher. Both leaders share their vision on harnessing AI to revolutionize healthcare, from making medicine more objective to enhancing clinical trials. The conversation navigates through challenges, innovations, and the potential of AI in healthcare, aiming to bridge the gap between technology and medical advancements for a more objective and efficient future in medicine.
Takeaways
- π The webinar, sponsored by Mendle, focuses on navigating the clinical AI landscape and charting the path to precision medicine.
- π¨ββοΈ Speakers include Karim Galil, MD, co-founder and CEO of Mle AI, and Charles Fisher, CEO and founder of Unlearn AI, both sharing insights into the integration of AI in healthcare.
- π§ͺ Mle AI's mission is to make medicine objective by creating the world's largest index of patient journeys, leveraging AI that understands medicine like a physician.
- π Unlearn AI focuses on solving AI for medicine, particularly in making clinical trials more efficient and accurate through the creation of digital twins.
- π₯ Both companies emphasize the importance of AI innovation specifically tailored to the clinical domain, beyond general AI applications.
- π The discussion covers the AI market's segmentation into innovators, tool builders, and wrappers, highlighting the roles and impacts of each in advancing clinical AI.
- π The critical role of data in AI development is underscored, especially the challenge of training AI models with complex, real-world clinical data to avoid inaccuracies and biases.
- π» The webinar explores the potential of AI to revolutionize clinical trials, with Unlearn's digital twins exemplifying how AI can simulate patient outcomes to streamline trials.
- π’ Talent and culture within AI companies are deemed crucial, with a focus on the blend of engineering and research expertise necessary to drive genuine innovation.
- π° The dialogue encourages skepticism towards overhyped AI claims, advocating for a grounded understanding of AI capabilities and limitations in healthcare applications.
Q & A
What is the mission of MLE AI as described by Karim Galil?
-MLE AI's mission is to make medicine objective by enabling the world's largest index of patient journeys, leveraging AI that understands medicine like a physician.
How does Charles Fisher's background contribute to his work at Unlearn AI?
-Charles Fisher's background as a scientist with interests at the intersection of physics, machine learning, and computational biology, combined with his experience in machine learning engineering and computational biology, contributes to his work at Unlearn AI, focusing on inventing new AI technologies for medicine.
What distinguishes Mendle's approach to clinical AI from traditional methods?
-Mendle's approach is distinguished by its use of a clinical NLP platform that allows command over the full spectrum of data, integrating both structured and unstructured data into a computer-ready knowledge representation for sophisticated clinical reasoning queries.
How does Unlearn AI aim to transform clinical trials?
-Unlearn AI aims to transform clinical trials by using AI to create digital twins of individual patients, allowing for the forecasting of medical outcomes under various scenarios, thereby making trials more efficient and accurate.
What challenges do innovators face in the AI landscape according to the webinar?
-Innovators face challenges such as the need for long-term R&D investment before product development, the need to differentiate amidst a saturated market of AI companies, and the difficulty in educating potential clients on the nuances of AI technologies.
What is Hypercube, as mentioned in the webinar?
-Hypercube is a product launched by Mendle designed to enhance clinical analytics through an AI platform specifically built for the clinical domain, improving accuracy and scalability in clinical trial matching and other applications.
Why do both speakers emphasize the importance of publishing peer-reviewed papers in AI?
-Publishing peer-reviewed papers is emphasized as it provides scientific validation of the AI technology, helps differentiate genuine AI innovation, and facilitates engagement with scientific and regulatory communities.
According to the webinar, how does AI technology face limitations in healthcare applications?
-AI technology faces limitations in healthcare applications due to challenges like context window limitations, hallucination risks, and the need for explainability, especially in complex clinical reasoning tasks.
What is the significance of domain-specific AI technologies as discussed in the webinar?
-Domain-specific AI technologies are significant because they address unique challenges and requirements of specific fields like healthcare, potentially leading to breakthroughs and advancements beyond the capabilities of general domain AI.
How do the speakers view the future of AI in healthcare and clinical trials?
-The speakers view the future of AI in healthcare and clinical trials optimistically, believing that AI will continue to evolve and provide innovative solutions for previously unsolvable problems, though they acknowledge the current limitations and the need for continuous research and development.
Outlines
π’ Introduction to the Webinar on Clinical AI
The webinar, hosted by Car Bball from Endpoints News and sponsored by Mendle, focuses on navigating the clinical AI landscape towards precision medicine. It features two prominent panelists: Karim Galil, MD, the co-founder and CEO of Mle AI, who shares his vision of making medicine objective through AI-driven patient journey indexing, and Charles Fisher, CEO and founder of Unlearn AI, who brings a deep background in physics, machine learning, and computational biology. The introduction sets the stage for a discussion on how AI can bridge the gap between medicine's slow advancement and rapid technological progress, aiming to make clinical research scalable and medicine more objective.
π Exploring Mle AI's Mission and Innovations
Karim Galil delves into Mle AI's mission to objectify medicine by leveraging AI to create a comprehensive index of patient journeys. He outlines the challenges of integrating technology with the slower pace of medical advancement and highlights Mle AI's journey from its founding to the launch of its first product in mid-2023. Galil discusses the innovative clinical NLP platform developed by Mle AI, which aims to revolutionize clinical analytics by enabling sophisticated queries on consolidated patient data, thereby addressing the inaccuracies and inefficiencies in traditional clinical data analysis.
π Unlearn AI's Approach to Enhancing Clinical Trials
Charles Fisher introduces Unlearn AI's mission to pioneer AI solutions specifically for medicine, emphasizing the company's focus on creating AI technologies that tackle unsolved medical problems. He shares insights into Unlearn AI's digital twin concept, which forecasts individual patient outcomes in clinical trials to increase efficiency and accuracy. Fisher explains how this innovative approach can significantly impact the design and execution of clinical trials, particularly in neurology, by providing more precise and efficient methods for evaluating treatment effects.
π The State of AI in Healthcare and Clinical AI Innovations
The discussion shifts towards categorizing AI companies into innovators, tool builders, and wrappers, with a focus on how each contributes to the AI ecosystem. The conversation then pivots to the specific challenges and opportunities in applying AI within the clinical domain. Both speakers emphasize the importance of domain-specific AI technologies and discuss how companies like Mle AI and Unlearn AI are pushing the boundaries of clinical AI by addressing unique challenges in healthcare, such as the need for sophisticated reasoning and knowledge representation in AI systems.
π§ Debating the Capabilities and Limitations of AI in Medicine
The dialogue deepens into a philosophical and technical exploration of AI's capabilities and limitations, especially in complex clinical reasoning. Both speakers share their views on the distinction between machine learning and deeper AI functionalities, such as reasoning and knowledge representation. They discuss the current state of AI, including the challenges of data context, hallucination, and explainability in clinical applications. The conversation highlights the importance of integrating machine learning with structured knowledge to address the nuances of medical data analysis.
π‘ Challenges and Opportunities in Commercializing AI for Healthcare
The conversation turns towards the practical aspects of commercializing AI in healthcare, discussing the discernment problem faced by buyers in a saturated market and the importance of distinguishing genuine AI innovation from mere tool use. Both speakers share their experiences with regulatory approvals, the importance of publishing peer-reviewed research to establish credibility, and the strategic focus on innovation to navigate the complexities of the healthcare industry. They stress the need for AI companies to demonstrate real-world efficacy and ROI to survive and thrive in the competitive landscape.
π The Future of AI in Clinical Trials and Ethical Considerations
The final part of the discussion focuses on the potential impact of AI on clinical trials, specifically the concept of digital twins and how they can streamline the trial process by reducing the need for control groups. The conversation then addresses criticisms of current AI methodologies, such as those by Gary Marcus, and debates the need for new AI theories to advance the field. The speakers also touch on the ethical implications of AI in healthcare, emphasizing the need for transparency, accountability, and rigorous validation in deploying AI technologies in clinical settings.
Mindmap
Keywords
π‘Clinical AI
π‘Precision Medicine
π‘Innovators
π‘Digital Twin
π‘AI Ethics
π‘Machine Learning
π‘Knowledge Representation
π‘AI Hallucination
π‘AI Adoption in Healthcare
π‘AI Talent
Highlights
Introduction of webinar on clinical AI and precision medicine, sponsored by Mendle.
Karim Galil, MD, discusses his mission with mle AI to make medicine objective using AI to index patient journeys.
Charles Fisher's background in machine learning, computational biology, and his company Unlearn AI's goal to invent new AI technologies for medicine.
Discussion on the distinction between innovators, tool builders, and wrappers in the AI landscape.
Insight into Mendal's AI platform for clinical analytics and the challenges of bridging technology and medicine.
Unlearn AI's approach to creating digital twins for more efficient clinical trials.
Debate on the definition of AI and its implications for healthcare.
The importance of domain-specific AI technologies in advancing clinical fields.
The challenges of AI adoption in healthcare and distinguishing between genuine AI innovation and superficial applications.
The significance of peer-reviewed publications as a metric for assessing AI companies.
Discussion on the ethical and practical challenges of AI in medicine, including data biases and model explainability.
The critical role of talent in the success of AI companies, emphasizing the need for a mix of domain experts and engineers.
Perspectives on the future of AI in healthcare, emphasizing continuous innovation and ethical considerations.
The potential of digital twins to revolutionize clinical trials by reducing the need for control groups.
The importance of maintaining an open dialogue and collaboration within the AI and healthcare communities to foster innovation.
Transcripts
hi everyone I'm car bball with end
points news I'm your moderator for
today's webinar navigating the clinical
AI landscape and charting your path to
Precision medicine we're sponsored by
mendle and I'm excited to welcome our
panelists joining us we have Karim Galil
MD co-founder and CEO of mle AI mle's
mission is to make medicine objective by
enabling the world's largest index of
patient Journeys leveraging AI that
understands medicine like a physician Dr
Gil's experience as a physician
demonstrated that medicine does not
Advance at the same rate as technology
with mendal he aims to bridge this Gap
facilitate clinical research at scale
and make medicine truly objective Kareem
is an entrepreneur by Spirit his first
company Krypton Works LED healthtech in
the Mina region with customers including
Fortune 500 companies we're also joined
by Charles Fisher CEO and founder of
unlearn AI Charles is a scientist with
interest at the intersection of physics
physics machine learning and
computational biology previously he
worked as a machine learning engineer at
leap motion and a computational
biologist at fiser he was a Philipe my
fellow in theoretical physics at a CO
normal superier in Paris France and a
post-doctoral scientist in biophysics at
Boston University Charles holds a PhD in
biophysics from Harvard University and a
BS and biophysics from the University of
Michigan if you have any questions
during today's webinar our panelists
have reserved some time at the end so
please hit the Q&A button at the bottom
of your screen when you think of your
question this webinar will be available
on demand tomorrow to re-watch or share
with colleagues and now I'll pass it off
to Kareem to get us started
Kareem um thanks Gary and um Charles and
I met through an investor like few weeks
ago and uh we started talking about the
state of AI in healthcare and we found
that we have a lot of uh common pain
points and we thought like the
conversation
should get extended so we decided to do
an endpoints webinar so today is more of
a casual conversation about how we view
the state of AI in general state of AI
in health care what are the challenges
and we both have a two startups who are
in the space of AI but not necessarily
competing to the same product so it's
going to be interesting to see two
points of view from two angles um uh
around the clinical AI space but before
we get started we thought we give quick
intro about Manda quick intro about
unlearn um mandal started in 2017 but
actually we launched our first actual
product in around mid 2023 and
essentially the rest of the journey has
been around R&D and we see that as the
Journey of almost AI company today it's
different than building a software
company you have to kind of have
long-term greed re-invest in a lot of
R&D before you can actually have a
product we were very fortunate to have
um investors who believed in the mission
from the very goodg go the company is
the most funded clinical NLP company
today um but around um end of 2023 we
were very excited to launch hypercube
and this year we are growing our
customer base uh we're going to share a
couple of use cases maybe today um what
we do at mandal is if we can go to the
next slide is we view the state of um
clinical analysis today like everyone is
using standard stack think of like the
snowflake the big queries of the world
keyword search we do standard ETL work
and that process is is is is pretty
difficult it cost a lot of money but it
is very prone to inaccuracies we've seen
a lot of cases where someone would come
and say we have 10,000 callon cancer
patients um running our platform we only
find 2,000 um but if we go to the next
slide what we have done here is we've
built a clinical NLP platform that
allows you to command the full spectrum
of your data so it's not only your
structured data that being said we're
also able to ingest structured data like
claims and and and fire and XL7 we
consolidate all of that in a computer
ready knowledge representation something
that the computer can reason over and
then we provide a platform that allows
you with no code to query this data and
do very sophisticated uh clinical
reasoning type of questions like find me
patients who have X Y and Z but has
never went through a treatment for
example um
we're very excited about the early
results there is a paper that we just
submitted uh for the chil conference and
uh we see that the product if we also go
to the next slide
um um next slide
please has been uh taken to test against
uh Chad llama for a use case like
clinical trial matching so group of
researchers at tupen has been
interrogating three systems for certain
uh number of clinical trials and we're
able to see that our uh approach has
been uh showing better accuracy but also
has been significantly better and
designed to actually scale in the
clinical domain from a cost and and from
a processing time so that's essentially
what mle does we are U clinical
analytics 2.0 through an AI platform
that has been built for for the clinical
domain um that being said I'll let you
Charles introduce un
learn
yeah thanks thanks Kareem um so unlearn
is uh basically an AI research company
um who uh we we're focused on maybe if I
had to say it really broadly I'd say
solving AI for medicine that would be
the mission of of the company um also
founded in 2017 uh now raised about $135
million um uh totally about 75 people
and you know as I said I think that our
Focus has always been that we're we're
an AI research company who's focused on
really inventing new kinds of of AI
technologies that allow us to solve
previously unsolved problems
particularly in medicine that's that's
our real goal as a company so we end up
on a lot of these kind of like AI uh AI
lists um but our main focus today is uh
in terms of our goto Market strategy is
that that we work with biotech and
pharmaceutical companies to help them
run uh more efficient um and more
accurate clinical trials so if you go to
the next
slide um and yeah just click just click
to the animations animations but you
might as well just click them so it's uh
effectively what we what we aim to be
able to do to create is a digital twin
of an individual person and what that
means is uh let's imagine that that
Charles participates in the clinical
trial I'll take data from myself uh
collected before the clinical trial
input that into some uh
uh AI train pre-trained model and I
wanted them to be able to forecast what
would happen to me in the future um and
we'd like to be able to do this for
effectively any measurement that you
want to forecast it at any point in the
future for anything you want under any
scenario for any person uh so it's a
very challenging problem uh but that's
the type of type of thing that we work
on and then we we partner with biotech
and pharmaceutical companies mostly in
phase two and three clinical trials
starting in neurology uh to to uh
include patients digital twins to to
make those trials
better and next slide I think goes back
to you Kareem but I could be wrong nope
it does um so we we thought just to set
the stage today um kind of uh we put
together this slide so just kind of see
like set the stage around how we see the
state of AI in general and specifically
around the clinical domain and we
essentially see there's three big
buckets of of AI related companies you
have folks who are actually building
foundational models or foundational
approaches towards U an AI problem and
we like to call those the innovators and
those are essentially folks who would
hire mathematicians PhD and computer
science more like a R&D sophisticated
approach to again an AI problem that
hasn't been solved before and needs a
different unique approach um but then
there is also a lot of companies to
today that are coming in the tooling
space and those make the job of the
innovators easier uh they provide you
with compute power they would provide
you with marketplaces for models they
would provide you with u uh uh levels of
abstraction that allows you to build AI
faster or to deploy AI faster then we
also see that there is almost now um we
were making the joke almost every couple
of hours there is a new AI company and
bunch of those come and sit into the
wrapping space and and those would
essentially leverage technologies that
are built by the innovators using tools
built by the tool Builders to put an
interface on top of a foundational
technology and essentially build a a use
case or or a product so there wouldn't
be essentially um a breakthrough in AI
it is more of like a productization or a
layer of of of ux on top of a model
built with the innovators on the
innovators side the challenge that we're
seeing is uh most of the work and the
attraction has been happening around
General domain Ai and became a term and
the idea is you want to build an AI that
can do everything under the sun and
there are companies that have been on
the Forefront of that such as open AI C
here anthropic Google deep mind but what
the premises of today's webinar is about
is more that there is a breed of
companies now that are doing the same
type of innovation they're actually
solving very hard AI problems but
specifically for the clinical domain or
specifically for a clinical use case um
and we believe that this is going to be
beyond the hype of AI this is going to
be actually the state where you're going
to find a lot of domain specific AI
technologies that are built say for
healthcare for fintech for for defense
kind of applications and we see Mandel
and unlearn to be one of those companies
that are in the clinical domain yet kind
of innovating and building breakthrough
type of Technologies and one question we
thought we could start off today with is
like what is AI to begin with I I think
there is a lot of uh there isn't really
like a strong definition there is a lot
of debate actually how would you how
would you describe how would you define
AI but again to set the stage here is
how we think of the market this also set
the stage maybe we can start with you
Charles kind of how would you define an
AI what's the difference between Ai and
just a piece of software sure um so I
actually take a very narrow view of what
AI is uh it in in in my view today um so
historically if you look back AI was
sort of a field of research about
creating computers that are able to
learn and act on their own without any
human oversight effectively and that was
what AI was and there's lots of ways
that people can do that um but what
really has become the dominant Paradigm
in the last
decade has been things that I would call
connectionist connectionist and so
connectionist models are these are a
sort of class of models that are really
inspired by the way the brain works I
might some of us might say Loosely in
Loosely inspired by the way the brain
works but that's effectively that's
effectively the concept so to me today
I'm actually primar I primarily Define
ai as as the use of algorithms that are
inspired by the way the brain works
uh to to solve problems without uh a
tremendous amount of human oversight of
course there's still some human human is
the teacher effectively but that's
that's the way that I Define it so it's
actually relatively narrow so I exclude
like classical machine learning
algorithms like random forest or spms I
actually exclude those uh from from from
the definition of what would be AI in my
mind and I I I agree with that
definition I uh I think the simplest
definition again I I unlike Charles I
don't come from a technical background I
come more from a clinical background but
the definition that made most sense to
me coming into that space it's a
clinical model of the brain it's a
software sorry a computer model of the
brain it's essentially you're trying to
get the computer to model a function of
the brain or multiple functions of the
brain but to that extent it has been
interesting because uh the way our brain
works and there's this really
interesting book think fast and slow you
have two systems right you have a very
intuitive system that doesn't think much
that doesn't take take time to make
decisions but it would essentially like
when you're when you swerve your car
when you're driving when you're
breathing you're essentially using that
system but you also have another system
that is slower more arithmetic that's
the system that you will use to solve an
algorithm or to think of a big idea and
machine learning in many different ways
mimics that intuitive fast pattern
recognition system but there isn't a lot
of Technologies or companies that has
also been working on uh on on on the on
system on on system one the more
arithmetic slower ones and essentially
the way I view machine learning large
language models neural networks it's
it's like a parrot in many different
ways that is looking at a pattern and
repeating it and when it comes into text
it makes you to it makes you confuse
fluency with intelligence but very very
few technologies have also system one
what what's what's your take on that and
how does that affect the use case that
you are working on
yeah so
um I don't know where to start start on
the use Cas so I'll start on the use
case and now back into the to the
broader question um you know what we
focus on as our use case is really
different I think from what everybody
else focuses on um kind of in in
actually most of the AI research
Community um because we're not we use
some stuff that that involves like
language processing but actually we use
that more internally uh for our our own
like internal data cleaning efforts
that's not like our products are not
built off of those kinds of things
externally um we actually use a totally
different style of of neural network
architecture that no one else in the
world uses um and so the original idea
for what we were thinking about was um
not so much how do you create AI to
solve problems that humans are good at
but how do you create AI to solve
problems that humans don't know how to
solve and so I'm thinking about like to
me the the pro the the the one like
prototypical example it's not from us
where
where somebody did this is Alpha fold
right if you give me the sequence of a
protein if you write it down in front of
me and you say Charles what's the
threedimensional structure of that
protein my brain cannot compute that for
you I don't know how to do that um and
so but those are the kind of problems
that I'm really interested in it's like
how do we create new kinds of AI system
to solve the problems humans don't know
how to so basically solve scientific
problems um and but I I'd say that a lot
of the work that's been going that
that's gone on over the last decade has
been kind of more around how do we
create AI That's able to automate
problems humans are good at um and so
those would be things like language Tas
or same thing with images right you
think about uh recognizing objects in
images or now even generating images
those are things that humans are really
good at and we're trying to create
systems that automate that so um anyway
so so to me like our our use case is
super duper different but Kem I forgot
the beginning of your
question beginning of the question that
most of the like people basically use
machine learning and AI as if it's one
word it's like synonyms each other when
essentially machine learning is more of
like if we use the early discussion
about AI is a model of the brain the
brain has two systems there's the
learning type of a system and there is
more the arithmetic more system um well
yeah so so for the work that we are
doing today I so I agree with you I I
think that all AI right now all AI
methods are sort of um relatively simple
input output systems um and uh all of
them uh so so my view is you said kind
of at the beginning I I wouldn't go so
so um far as to say that things are just
parrots I think that they're
interpolators uh they're very good at it
um but I don't think that that the kinds
of things that we've worked at today are
are by themselves going to get us to
True sort of general or super
intelligence I think that there are
breakthroughs that are required to get
to that and um I know people are working
on it uh you know reasoning systems and
other kinds of systems like that but I I
think that today they're primarily going
to give you when I say they they're
interpolating what I mean by that is
that they they give you similar answers
to similar things they've seen before if
you ask it to create some brand new
thing that like it's never seen anything
like that before uh and you're
extrapolating to some really truly brand
new situation today's AI systems don't
don't know how to do that yeah um in in
our
use case here at Mandel our our number
one job is to synthesize a patient
Journey from complex data so we would
take thousand PDFs coming from an
oncologist some claims data coming from
a provide from an insurance company and
our job is to synthesize um coherent
patient Journey from many conflicting
facts so you would find in one page the
doctor saying the patient is uh
metastatic uh but then you look at the
pathologist saying the patient is stage
one and now you have two conflicting
pieces of information that you need to
reconcile which requires a lot of uh
clinical reasoning and our experience
with machine learning only approach or a
large language model only approach that
those systems as you said are really
good at pattern recognition and
repeating something that humans are good
at if they are fed with the right data
but it cannot do complex clinical
reasoning like a physician or or a
clinical expert would would would would
would be able to and the only way around
it is to couple it with some sort of a
knowledge representation or something to
ground the rules of medicine or the
concepts of medicine or ground the
machine learning model into it and it's
so interesting because um Yan laun which
is one of obviously the few people that
won the touring award for for neural
network newer architectures was saying
the only the neural network today is not
even at the level of a brain of a cat
and the only way we can come closer is
if we can couple it with symbolic Ai and
with knowledge representations and we
don't know how um so that's where we
have been spending a lot of time how can
you do complex reasoning with an AI
model
essentially
um yeah I was just gonna ask maybe um
and maybe this is too technical of a
question
but could you explain a little bit as to
how how you would View kind of that as
being different from fine-tuning just an
existing model on like say met text or
something like that like what how is
that going to be different so when when
when you're looking at like a lot of the
models today have limitations around the
context window and um a clinical record
by default will exceed that context
window easily which increases the
chances of of hallucinating the other
thing essentially how how those models
would work is it would bring terms that
are related to each other closer to each
other so an adverse event and fatigue
would probably be closer to each each
other to each other in the model but
once you move to another area you move
from uh oncology to Immunology fatigue
becomes more of a symptom rather than an
adverse event and how can you teach the
model to start understanding the nuan
differences between those two things um
I would say the other thing is no matter
what you do when you're are using a a a
especially a larger larger language
model the idea of hallucination becomes
incredibly dangerous when you are making
some sort of a clinical synthesis of a
patient journey and having like almost a
filtering layer that would tell you you
cannot have headache in your knees like
headache is probably not going to happen
in your knees ever and there is
impossible that you can have a stage one
patient who's also metastatic at the
same time and it's like kind of giving
you those like those things are
impossible to happen it's just like it's
against the the laws of medicine helps a
lot and decreasing the hallucination I
would say the third thing is
explainability in specifically in our
use case folks want to know why did the
AI why did the AI conclude that this
patient has adenocarcinoma and not any
other type of of of hisy and you have to
be able to trace back the logic and it
becomes incredibly hard when you're
having a machine learning only approach
to the problem I would say those are the
three things explainability
hallucination and the context window of
of of medicine in general is generally
High you know that's that's an
interesting idea because um I would bet
that those th well I would bet the um
those unallowable things if you actually
just trained a model on the raw data
like to just train a model on Raw data
that you would actually learn those
things because the data do have mistakes
I I absolutely worked on a project I
remember where a patient had had uh like
a leg amputation at some point and but
their medical records later on have them
having problems with that leg which was
just
amputated so if you're just training
entirely on the medical records you're
actually learning some of the mistakes
that are in the Raw data themselves yep
uh but then you still have to deal with
some Al some of the other problems like
the hallucinations and and and and
explainability but it's also very
interesting because the way folks
practice medicine in general is is has a
lot of intuition into it so if you start
training on like the only way you can
get to that is you have to train almost
on every medical record in the US coming
in from different states with different
regulations with different ways of of
approaching things to like take out the
bias but the other approach would be is
like hey no matter how you practice
medicine these are the like the Periodic
Table of medicine those are the laws
that would govern how we would how we
would approach um problem
um I think one one one topic we talked
about is as like both of us are
obviously commercializing an AI product
into the health care space and this is
not an easy space to commercialize
anything in general let alone an AI
product so H H how is your experience
with adoption what how are you seeing
the buyers today on the heal everyone
wants to buy an AI product but do you
think everyone have the right criteria
can you can you talk more about that no
I mean obviously like you said it's a
difficult space uh to to work into um I
think for us we have found
um I think I I'm going to go with the
two the two things that have that have
been positive and I've for us and maybe
two things that I would say that are
that are barrier um uh so I'll start
with the positives um one of the biggest
things for us is is that I think when
you're deploying sort of AI based
systems into into any sort of medical
context um there's usually some kind of
extra layer of validation that people
are looking for um and so in our case we
work in phase two and three clinical
trials like we we are working as part of
a study protocol actually like our our
AI is like written into the protocol of
these studies and so we wanted to be
able to go through um and get regulatory
buying um and so going and getting uh we
got a regulatory qualification for one
of our methods uh in from the EMA in
2022 uh got a letter from the FDA at the
end of last year saying that they concur
with that and so um working with The
Regulators has actually been really easy
uh I would say um me I wouldn't say it's
fast like you know on a startup time
scale working with The Regulators hasn't
been fast but it has been a really
positive experience and that I think I
think the regulatory agencies in my
they're were thinking about this
correctly and that they're not actually
that focused on like you know what is
the underlying algorithm and and how
does it work and that they're much more
focused on the context of use and making
sure that however whatever AI system
you're creating when you deploy it into
some use case that it really work works
for that and so I think that that's
really good and then you know getting
those qualifications has really helped a
lot with uh with our sort of go to
market the other piece though that I'd
say has been a positive is just finding
Innovative people uh there are people in
the industry like uh who are uh they
want to do things differently they want
to see technology get adopted or they're
frustrated with the current you know e
rooms law situation where truck
development is just getting slower and
more expensive and they want to do
something different
I think finding those people has been
great now the barriers are um one of the
big barriers is I I've coined this
actually back my friend Austin Austin
Juan coin this back when we were working
at fiser together but he called it the
discernment problem and the discernment
problem is that uh there's a bajillion
companies that that say they are all
using
AI um and the people who we're trying to
sell to are not experts in AI um and
they have uh they to them all of these
companies look exactly the
same so how do you actually stand out uh
amongst all of these companies uh many
of whom are are not maybe doing anything
particularly Innovative but but you know
everyone's using the same language and
that would get to my second point which
is even inside the Pharma
companies uh I at today I feel like the
my definition of AI which I described as
very narrow which is like connectionist
based models that like neural networks
has been replaced by uh what I would say
the industry uses which is anything that
uses a computer this is ai ai so like
you ask like what's biostatistics AI
what's bioinformatics AI like all of
those things have just gotten rebranded
as AI um I hear lots of people for
example say they're like oh well we are
doing AI a we've been doing AI for 50
years but that doesn't make any
sense because the algorithms that people
are using for like modern AI systems
have been all like really developed in
the last 10 or 15 years so it doesn't
make sense if you say you've been doing
it for 50 years then you're doing you're
not doing uh you're not doing AI in my
opinion if you've been doing it 50 years
you're using other kinds of
computational methods so um so those are
that that's been my kind of broad
experience what what about you Karem um
I in my 's case we don't have uh I mean
there is pain in getting regulatory
approval and uh my heart goes to all the
effort that you had to go through that
uh but I think down the path to your
point it kind of gives you that stamp of
validation that can help a lot in your
goto Market in my use case we don't have
that so I have to only suffer from the
latter point that you made which is like
every AI come everything is an AI
company till proven opposite and uh to
your point also we are seeing the
innovators in in every org and those are
folks who are actually spending the time
um I always get a little bit
um I mean I'll put it lightly irritated
once I find someone with pure MBA
Investment Banking background who took
two AI corer courses coming in on a call
dcing and AI company um from on behalf
of a vendor and that essentially becomes
a big problem for us because um we're
not we're not looking at the same ground
bases and the example I always give my
team U when I wanted to buy a a a a ring
to my wife to propose I had no freaking
clue how to look to buy a diamond for me
it's a piece of glass still proven
opposite right everything just looks
shiny and it's only when I started
getting educated like here's the clarity
score here is this here is that it
actually started making sense to me why
would I pay five like 10K I'm not going
to say how much I paid for my wife's
ring but how much should I pay versus
like a 100 bucks right and I feel a lot
of the AI buyers today haven't went
through that exercise yet of kind of
understanding what are the criterias
that you should be looking at an AI
vendor what we are betting on is that a
lot of the pilots that are happening
today are going to fail and then
companies that actually did have true
technologies will will remain and that's
the state of every bubble that happens
you just raised money and we're seeing
in VCS saying the same thing they are
not betting big on a lot of AI companies
because they assume that most of those
Pilots will fail and those who are
actually going to pass 2020 for and show
clear Roi on the business or clear Roi
on the outcomes of for the patients are
going to be the AI companies that will
eventually survive that that that
wave um do you see the same do you see a
lot of Pilots happening in in around
your your use cases or is it like we're
either going to get in or not going to
even try the
product I mean you know we've been you
know in in our case I there are
definitely still companies that want to
run run like earlier stage like test
cases pilots and things um I would say
that in some cases we're a little bit
we're we we're we're some part beyond
that and that we are actually using our
methods in some phase two and and and
and we're uh working on some protocols
for some bigger phase three studies now
um so to a degree we're a little bit um
we're a little bit but I would say like
there's the innovators where we're just
beyond that pilot stage like like we're
deploying things now with some companies
where where there are some Forward
Thinking people um and then there are
other companies who we hear all the time
and I you want to know what makes me
irritated is this phrase which is I've
heard now multiple times that we want to
be your second customer in this disease
area or this indication whatever it's
like oh you want to be the second like
that is just like what's wrong in my
opinion that's like what's wrong with
medicine is that is that attitude uh um
so uh but yeah I mean I I certainly
think that there are lots and lots of
companies who are
um in in in the broader space I think
that there are a lot of companies who
are working or applying computational
techniques or applying various machine
learning Technologies to problems in
medicine or Healthcare I don't think
that there's a lot of companies that are
really trying to push the boundaries for
uh for how these things really work um I
think you know to me one of the biggest
challenges for for uh the thinking about
the way AI is moving right now for for
people in in this industry is they're
used to a really slow moving technology
um so you think about drug development
and Discovery right drug Discovery
development takes like 10 or 15 years to
develop and bring a new drug to Market
and so uh maybe it's a little faster for
a medical device I don't know five years
probably still seems fast though uh so
you're talking about you know five to 15
years is the pace o over which things
change um but in AI things are CH
changing right now every like every like
five to 15 days right and so part of
what I think is a challenge for example
is like you work you might work with
somebody if you're really an AI research
company then if you've done a pilot with
me three months ago you haven't piloted
my technology now you piloted my three
months ago my prior version we we have
the same problem where actually the
customer buys the product or gets into a
pilot and two weeks later we just
launched the newer model and the
customer is like two weeks be behind but
it's not only two weeks it's it's some
R&D work that has been happening for for
few but we talked before the webinar
about uh areas of like um when we were
setting this up we said look like we uh
areas of differences between both of us
would actually become would make this
webinar more exciting so here's one area
of difference that that that we can go
through when for example we were talking
about the idea of like uh headache in
the knee or something like that the like
what I've heard from you is what I've
hear a lot from from clients is like I
bet if you actually train a model on
more clinical data that essentially that
problem wouldn't be solved but when
mandal started 2016 essentially we have
a team of 100 plus clinical annotators
and we have tried every model under the
under the Sun from birth back in
2019 or something to like the latest and
the best today and it essentially does
not work it the systems that that amount
of data that we have um per patient is
too big where the model really gets
confused and starts itating nonsense
essentially around a patient Journey but
here we are like I I view you as a
clinical like as an AI expert but we
have to go through that debate back and
forth the problem is if you and like if
if if you and I are going through that
or someone on my team is going through
that there's some ground truth that we
can stick to it becomes 100x harder if
I'm talking to someone who has never
built an AI model who has never trained
an AI model it becomes a a losing battle
so what ends up happening is they do
follow their own path they come back six
months later or a year later and say you
know what we just lost whatever $5
million on that initiative and didn't
work one one actually true case that
happened with us is we've been working
with a big diagnostic company and they
have taken multiple approaches including
Google like approaches and there have
been voices in the company saying hey
like Google is always going to win over
a small company like Mel the approach of
machine learning is as an only approach
is good enough they went through that
they found say x number of patients that
they thought is eligible for a certain
project they had and using our approach
it was like 20% of that number and
essentially what happened was like well
if that's the case Mendel is wrong the
other company is right and we had to
push for manual error analysis of the
two sets of data and guess what ended up
happening is actually we were right but
this type of friction and like the proof
is on you type of type of uh type of a
headwinds is what I'm seeing today in
the market as uh the biggest challenge
for AI companies um including yours or
or or
mine yeah you know the other thing
though with sort of I would say the flip
side of some of these things that's
really good I I in my opinion about
working within kind of
space is that um the best marketing the
best marketing that any that any of us
AI companies can have and it's
absolutely been true for us it's just
publishing an actual research
paper just publish you publish research
paper and people at Pharma read it right
uh that's absolutely true um for us like
I've had people at the FDA uh ask us
questions about our papers on archive
not even peer-reviewed papers the FDA is
reading our archive papers so so I think
that that's one of the best parts about
working within a scientific field is
that if you engage not only not if you
actually really engage you be like here
are the algorithms that we're using
here's these things here's how they work
and here's examples of them working in
these areas that you get real scientific
engagement from the other side I like
1,000% agree with that and I think
actually talking about like how can an
AI buyer assess a vendor I would say if
you don't have any peer-reviewed
Publications that chances are you don't
have any sign on your team because it's
not only for marketing your team will
ask I want to publish about this I I
want to compare against the status quo I
think this is a really great point and
it's like uh easy easy to go through
that checklist if you don't find
peer-reviewed Publications com like
talking about the technology or
comparing the system to like status quo
that is for me is already a yellow flag
that that something is is not going well
um this year we've decided actually to
allocate around 70% of our marketing
budget towards publishing peer-reviewed
Publications rather than actually
spending on conferences and and and
things like that but I 100% agree with
that uh point so what's the most like U
provoking AI marketing message that kind
of made you feel like oh man that's come
on that's too much like something too
like like making a big deal of of an AI
capability that does not exist or
something that just foundationally
Incorrect and you felt like
that that is
outrageous
um that's actually a hard question I I
don't know if I have seen something
that's been like an outrageously
overhyped claim I don't know if I
actually don't really think that that is
that that is really what I have seen um
in fact I remember uh JP Morgan one of
the previous JP Morgans maybe like JP
Morgan early 2023 or something like that
I was on a panel with endpoints and I
said something about like by the end of
the year uh an AI system is going to
pass like the bar exam the medical the
the mcap the ls it's going to pass all
these things just one system that's true
right at the time people acted like it
was hype um so I actually think that at
least for my opinion AI within the
healthcare space is under hype I think
it is under hype because I think that
people focus so much on what has been
done they go what have you done and of
course that's very very important you
got to be able to get things into these
clinical trials you got to use them or
or whatever use Cas is um but again I
think that what people are missing is
that it's a rapidly advancing technology
um so I to to to me it's less around
like people's claims around what AI can
do or will be able to do I think that
that's largely underhyped to me the
stuff is that like when somebody's like
hey we use we use sophisticated Ai and
then you go what is it and it's like
it's it's like sklearn import PCA and
it's it's like well that's not that's
that's not really what I had in mind um
and so but that's actually often but
that's kind of kind of what I think is
challeng is that's really difficult to
tell sometimes uh what people really
really are doing um so you have to
really I'd say re I've seen papers like
this I'm not going to call out who
specifically but that I have like a
specific paper in mind for example where
the whole beginning of the paper talks
about some this is uh some deep neural
network based model or whatever I forget
it is and then halfway through the paper
it just switches to using a random Force
the title of the paper is like uh deep
learning for something something
something like this but the Deep
learning model loses to just the random
force in the in their paper and so the
whole rest the whole second half of the
paper just like and so we use this D of
forest for the rest of our analyses and
that's like a fairly well-known AI
startup in the Medical Healthcare space
that published that paper uh but again
they published at least they published
the paper so you can read the thing and
see what they really did
did so um talking about the you're
saying like well-known startup um coming
into the um we're seeing I'm seeing a
lot of the hype happening now on the m&a
side of things um so obviously every
company is trying to take an AI approach
that is going to boil down to like buy
or build and the buy is not only like
buy from a vendor it's actually bu the
vendor Al together and and use the AI
capability we're seeing a lot of that
happening um
but there is a fairly like uh also known
startup in in in in our field that has
been essentially using um apis from
another AI vendor which basically means
like you can just go buy your those apis
yourself they just have a cooler
interface than than what you you would
get from the original vendor and we just
got that just got to see that this
company acquired at like high high high
high dollar value and in my mind
man like for $5 million you could have
done exactly the same thing and you
could have just saved another like
hundred million or something um so I'm
seeing also some of that hype happening
on the m&a side where companies that
kind of got the right Banker or got the
right type of packaging um with some
papers that have very interesting titles
are and end up doing some interesting
transactions that wouldn't have happened
I guess had then their more rigor around
the AI uh criteria so what are the what
are the criteria like if you are buying
an AI company or if you are buying an AI
product in general what would you advise
people to think
of um well I honestly actually I might
go in a little bit of a different
direction on that on that Kem I even if
somebody wants to use like an
off-the-shelf wrapper they probably
still should buy an AI company to do it
um uh you know so so at least from my
own perspective I mean I can speak from
like you know I so I'm a PhD in
theoretical physics right um and I'm
really an AI researcher and I I
attempted to go and work at a big Pharma
company and I would describe my
experience there kind of as I I like my
colleagues and everything but I kind of
describe it as an organ rejection like I
like it was like there was a very clear
oil and water kind of situation um and I
I think that that's quite broadly that
that the the the culture of companies
who are really building these new AI
systems very different from the culture
of of a traditional company in in
healthcare medicine Pharma Life Sciences
um I don't think that they go together
um and so I I think that that that that
building these types of capabilities
internally uh having tried to do it
myself okay is probably impossible I
think it's probably impossible um and uh
that doesn't mean people are going to
try they're going to try uh but I but I
think it's impossible and so I actually
think that if people do want to have
these internal capabilities they they
should go to look for companies that
that uh for Acquisitions they should
leave those companies semi-independent I
mean so one of the examples of like what
and I guess I don't know too much I have
a few friends who are at it now but um
would be like preent design so so
Genentech uh in the drug Discovery Space
went out and acquired this this AI uh it
was a small a company it's actually
grown I think a lot since it's been part
of genetic but I think that type of
model in which if you're looking to
really do these things yourself that you
you do go out and acquire a company uh
for the culture and leave it independent
so that you don't mess its culture up I
actually think of as being important
actually yeah would you do the same if
so let's talk maybe this is the last
point and we can jump to questions let's
talk about Talent um in in my mind like
the biggest deterministic Factor around
an AI company is the talent uh I think
first Mar some VC said they assume
there's like lesser than 2,000 people in
in the world today who are capable of
actually doing a breakthrough in AI or
have the the right background for it but
anyways it's long way to say like talent
and we're seeing the talent war between
like Big T can even startups every every
capable AI scientist today is getting
multiple offers from from from multiple
places right so how how much weight
would you put on talent because to your
point even if company is just wrapping a
technology just acquired for for the
culture and I would agree with that but
I would say the rest of the statement
would be if they have ai talent because
if you're buying culture you want to buy
the AI culture but if you have a company
that mainly 100% are software Engineers
that has been wrangling apis those are
no different than the software Engineers
that you already have in in your company
and Ive seeing company like Mosaic AI
for example acquired by data bricks for
enormous amount of money but again it
boils down to the talent and even if the
company didn't have that much traction
so how much weight would you put on on
Talent or is your point that as long as
they have attempted some sort of
innovation they probably have the right
traits so still take the risk on that
but like how much weight would you put
on Talent essentially is my question uh
no I mean 100% although I wouldn't put
down I wouldn't necessarily put down the
software engineering talent I think
software Engineers are very important in
in in particularly in in ml um but like
you mentioned something earlier about
you know if your ml team is just a bunch
of NBAs
then that's that's not really it's not
really an ml team uh I do I agree with
that you know um I I think that you know
companies who but I I that's kind of
partly my that's that is my point though
effectively what I talk about that the
cultural difference which is that you
know at unlearn most of our employees
have PhD in physics or math or computer
science um and it's very very different
you know you go to to a life sciences
company and everyone also has phds but
there phds in biology or their MDS and
these are very different disciplines um
and and not only the different
disciplines because the different
backgrounds and stuff but I the there's
really there are cultural differences
between the between what people care
about who come go into those fields and
I think the challenge for a lot of the
companies uh who are trying to be sort
of this te new tech bio set of companies
it's actually how do you integrate these
cultures um like if you're a small
biotech and you're you know like say
you're recursion and you're trying to
like really simultaneously be a leader
in Ai and ML and in drug Discovery I
think it's hard probably uh to to to be
able to actually really truly have these
two separate cultures and get them
together so no I agree with you that
1,000% you want to be looking for
companies that are higher in math
physics computer scientists uh but I do
I I would say that engineering Talent is
as important as research Talent enormous
yeah an enormous amount of of of of the
progress in ml particularly the last
like five years has really been
engineering driven more than research
driven there is Yan Lun also said this
uh let's engineer the heck out of it uh
which is essentially if you look at open
eye it's like a lot of engineering that
happened on a technology like
Transformers that has already been there
before that but it's like if you a
clinical analogy it's like you cannot
have a hospital with nurses only or
Physicians only and I think it's the
same thing you cannot build an AI
company with Engineers only or with phds
in in math and physics only I think it's
a lot of collaboration between both and
it's a spectrum of how much the density
has to be between those um I know
endpoints want us to jump into questions
and we have a lot of questions here um I
think the most interesting question was
for you to say which medical startup
company has had that funny up the paper
not goingon to say that
yeah it's a published paper so people
can go look it up themselves yeah uh I
think one question for you that I
thought was interesting and maybe I can
take one for me is like for you was like
can AI cut down the time it takes to
carry out the clinical trials in
particular phase three oh yeah I mean a
thousand per this is this is exactly
what we're what our goal is to work on
um so the basic idea about what we do if
you think about so the applying this
kind of idea of a digital twin in in a
clinical trial so a clinical trial you
want to know what would happen to a
patient if they got a
drug in comparison to what would happen
if they don't and so what we're
effectively doing is we're using these
models to simulate one of those outcomes
for a patient um and if that model was
perfect you could actually run a
clinical trial that doesn't have a
control group so you could just say I'm
going to take each patient I'm going to
create their digital twin I'm going to
simulate what would happen if they were
if they were assigned to control group
and and just by doing that comparison
you would be able to to get an estimate
to the treatment effect
um like you were saying earlier about
hallucinations and other things today's
AI systems are not perfect uh so the the
types of things that we're working in
there's still randomized studies where
you do have some patients uh being
randomized to a real control group um
but it's fewer than an an typical study
um so you know we might work to say you
know half the size of a control group as
you would need in a typical study and
that cuts off you know potentially a
couple of hundred patients for a large
for a large trial makes a trial more
attractive this is why people are
participating in trials in the first
place is typically to get access to a
new experimental therapy so it's really
a win-win and that we're able to do
things that that speed up the trials for
sponsors but do it in a way that keeps
sort of uh the the science rigorous so
you get the same kind of rigorous
results out of these RCS as other ones
uh and aligns with What patients want
out of Clin iCal trials in the first
place I think one other question um for
both of us actually was um what do you
think um I know we're going to disagree
on this one so let's go through it what
do you think uh regarding the criticism
of deep learning by some known people
like Gary Marcus um that current AI are
in worrisome hands regarding AGI and how
would that impact medical AI in general
um and related more to both of your
companies how do you think another new
AI Theory could boost your companies you
want to take this
first sure uh I'm trying to decide how
mean to
be
um
so look I I'm sorry but like fun the if
you wanna if you want to to go out there
and think about like two today's AI
systems have have some limitations they
do they do that's why people like me
exists that's what my job is is to think
about how to overcome how to invent new
kinds of algorithms that that will
overcome these limitations and um do I
think that those new types of things
will with be within the realm of what we
call Deep learning I do I think that
there will be different architectures
and different training techniques and
different loss functions and things like
this than we use today but but I think
that they will fit with under the same
umbrella um I think that uh you know
again if you look at what we do at
unlearn we actually use a totally new
architecture we use a completely new
kind of architecture that no one else in
the world uses actually for our machine
learning approaches it's a deep neural
network but it's a really weird kind of
deep neural network super strange uh so
again we're inventing new kinds of new
kinds of machine learning like totally
new kinds of things I I think that um
the the criticism where people just say
like hey it won't work it's I I don't
understand I don't understand what what
Gary is doing frankly I like go out
there and try to build a neural network
then that that he has never ever
published anything in which he actually
trains a neural network that works well
or trains some other machine learning
technology that works well on something
go do it stop just telling everybody
they're gonna be wrong and go go show us
how to do it if you think you know so
well I don't understand I don't
understand so anyway uh it ended it
started nice and it got
[Laughter]
um but I I actually have um probably a
different point of view on that um I see
like sometimes it's that to your point
there is some extreme in in in how
shooting I feel like in trying to become
objective we're becoming extreme in
shooting down some other technology uh
but to be clear also it's like if you go
to Home Depot and you buy a hammer and
you say trust me I can solve every
problem in the world with that hammer
nobody's going to believe you and I
think the AI Community is doing the same
we're picking machine learning and
neural networks and we're saying every
single problem Under the Sun is going to
be solved with this and I feel like this
is also a failing approach folks like
Yan Lun again I'll keep coming back to
that is running an AI department at
Facebook and they are shipping models
they have shipped llama and they're also
talking about the limitations of of the
AI Technologies in certain areas
especially around reasoning um I've
personally attended
um uh presentation by a big AI company
that I'm also not going to mention their
name one of the most famous AI companies
that just were in the media because
their CEO left and they brought the CEO
back but I'm not going to mention their
name and they went through like a 20
minutes presentation around applications
of this technology in healthcare and
then someone comes in and asks but what
happens when hallucination goes on uh
does it ever hallucinate and the person
says yes it it does and says okay how
can I tell he said we actually don't
have a way yet but we warn our users to
not take the results as is but the
reality of the matter is he spent a 20
minutes presentation not talking about
it allog together he only brought it up
when he was asked so it is not that they
are telling their users that there is a
lot of downsides and there is a lot of
limitations for today's technology I
would say the second thing is once you
come into the area of machine learning
and neural networks essentially the
training data dictates a lot like
technology aside training data
dictates a lot and the fact that the
company owns what data the model is
going to be trained on and don't have to
always disclose what is this data can
create insane amount of biases that
literally boils down to the CEO and the
executive team deciding should we should
we do this more or not so in my in my
use case I can train the data on a
certain drug like I can basically show
the model patients responding really
well to lung cancer if they take a drug
that some Pharma company paid me more
money for and I can bias my training you
know like I can bias the training data
easy to do something like that and I can
always say sorry it's it's the model or
the model hallucinated um but there is a
lot of ethical considerations on what
data the models are using there's a lot
of ethical considerations on how we
present it that being said I also think
it's a beautiful piece of technology and
don't get me wrong 80% of our 70% of our
stack is boils down to machine learning
models it's a beautiful piece of work
but on it it on its own is again is like
a hammer that's going to solve every
every problem Under the Sun I know we
have two more minutes left but there was
an interesting question for you which is
what is it uh can and I'm excited about
digital twins so I'll I'll I'll give you
the last two minutes could you help us
understand in simple words what are the
biggest benefits of using digital twins
in clinical
trials oh um
sure I mean the big biggest benefit is
that you can run a clinical trial in
which fewer people have to be randomized
to
control so that allows you to run the
trial faster because you just you don't
if you have fewer patients to enroll
then your time to complete enrollment is
faster uh it's usually months faster um
it aligns better with the expectations
of patients so you get more people more
a larger fraction of people uh getting
access to the experimental treatment
which is usually why they participate at
all um and we can do this in a way in
which we make sure that the clinical
trial produces accurate and unbiased
estimates of how effective the treatment
is that's how we've been able to go in
and work work with the Regulatory
Agencies to to to get these methods
qualified for phase two and three
studies um how all of that works uh I
the simple terms in two minutes is maybe
a little to too hard uh but um uh but
we'd be happy if anyone wants to to know
more about about those things just reach
out to to us and uh and we'd be happy to
talk about it yeah I mean we have a
couple of minutes left so I don't know
if uh at least I'll offer that and I
know that uh Charles will do the same
but I would say like like some closing
notes Here is like if you look at
Charles's background it's celebrate or
die my background is Muhammad Ali
hitting down someone there is a lot of
uh people really trying to scratch the
surface here on what AI can do in
healthcare and we're we're essentially
not doing this only to build companies
and and make money I think there's both
of us we talked about this like uh both
of us come in from a lot of like like
there is no there is no motivation for
you to like go build Crazy Technologies
unless you truly believe in the outcome
of that so please reach out to us if you
have any questions around AI in general
if you're trying to to buy a AI
technology and you want some advice on
what considerations should should you
consider I think both of us would be
more than happy to like um be helpful as
much as we can um we hope those 60
Minutes were the start of the
conversation and not the end of of the
conversation and uh we wanted to bring
it to you as organic as it can be and as
casual as it can be also so thanks
Charles for for that and um for
endpoints also for helping us put this
together yes thank you thanks for
invting me fortunately that is all the
time that we do have for today I know it
was a great discussion lots of great
questions coming in so thank you
everybody for tuning in thank you Kareem
thank you Charles for sharing your time
and expertise and thank you to mendle
for sponsoring the discussion today and
points webinars if you'd like to rewatch
today's webinar or share it with
colleagues a link for on demand viewing
will be emailed to you tomorrow I'm
Carrie bball for endpoints news thanks
for joining and we hope to see you at
the next Take Care thank
you
Browse More Related Video
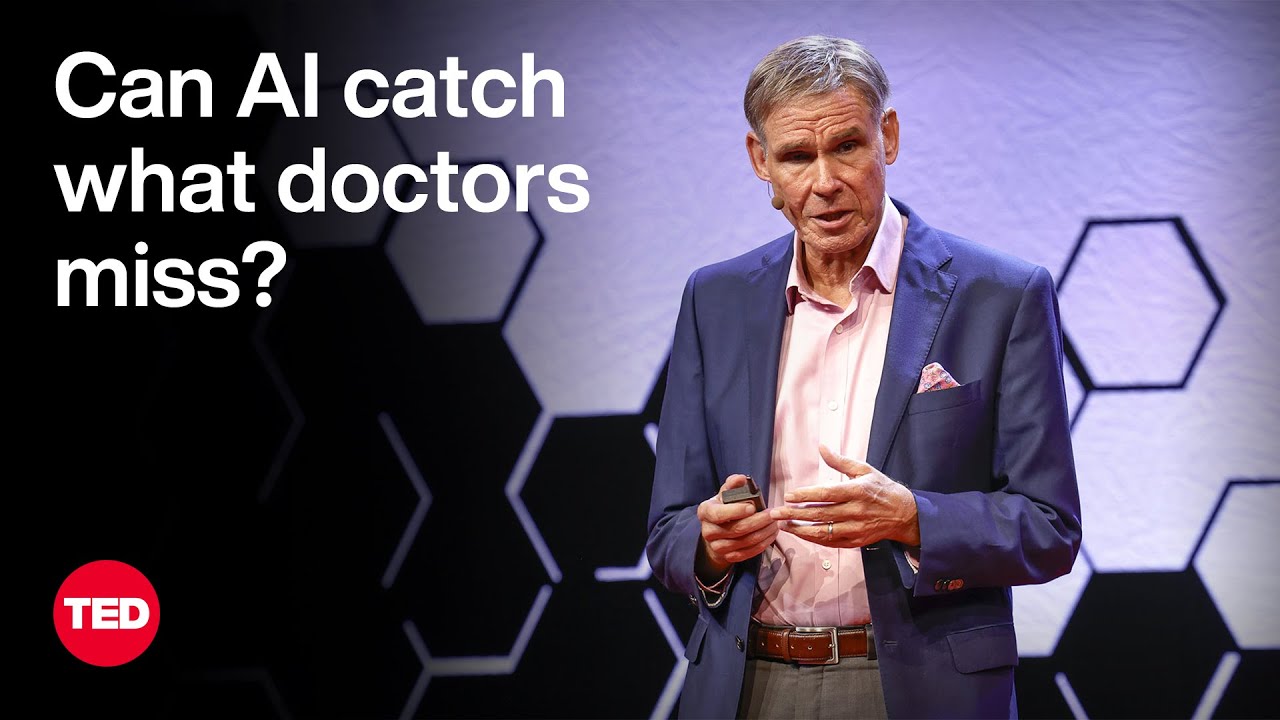
Can AI Catch What Doctors Miss? | Eric Topol | TED

How CROs and Sponsors Improve TMF Management with a Shared eTMF - Agatha eTMF software
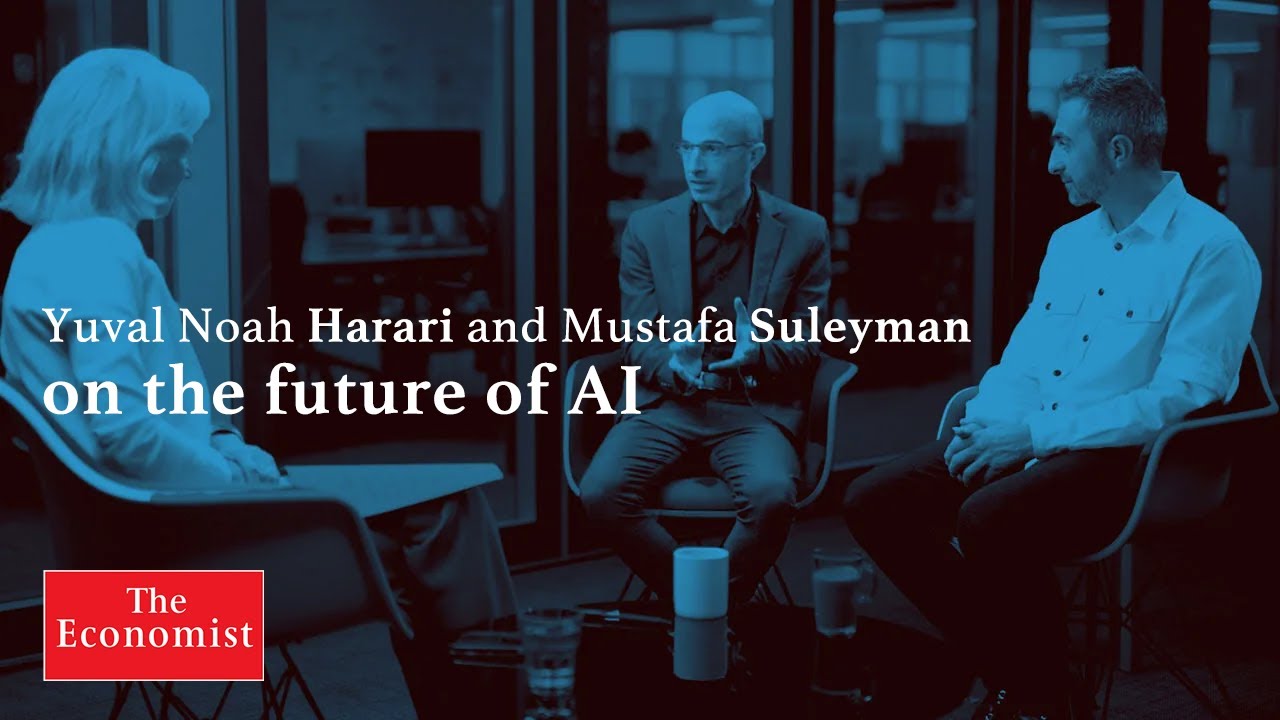
Mustafa Suleyman & Yuval Noah Harari -FULL DEBATE- What does the AI revolution mean for our future?
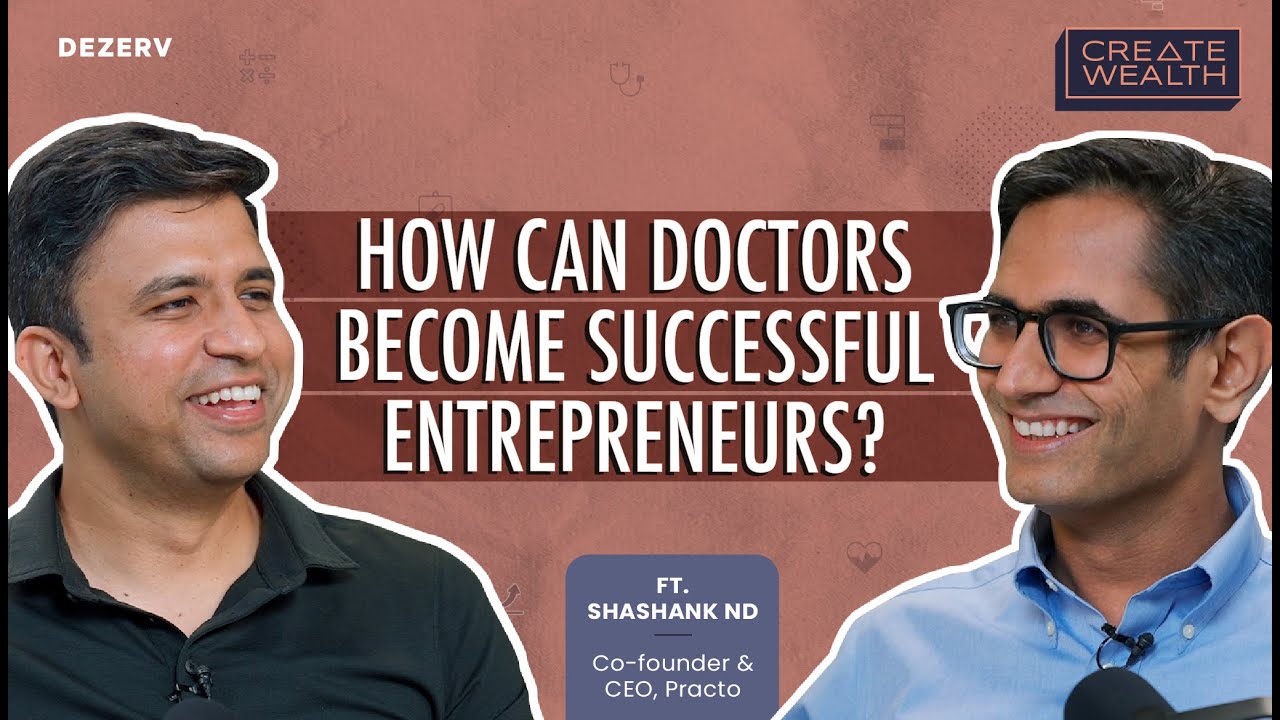
Insights for Doctors to Build Successful Businesses | Doctorβs Day | Ft. Shashank ND, CEO of Practo
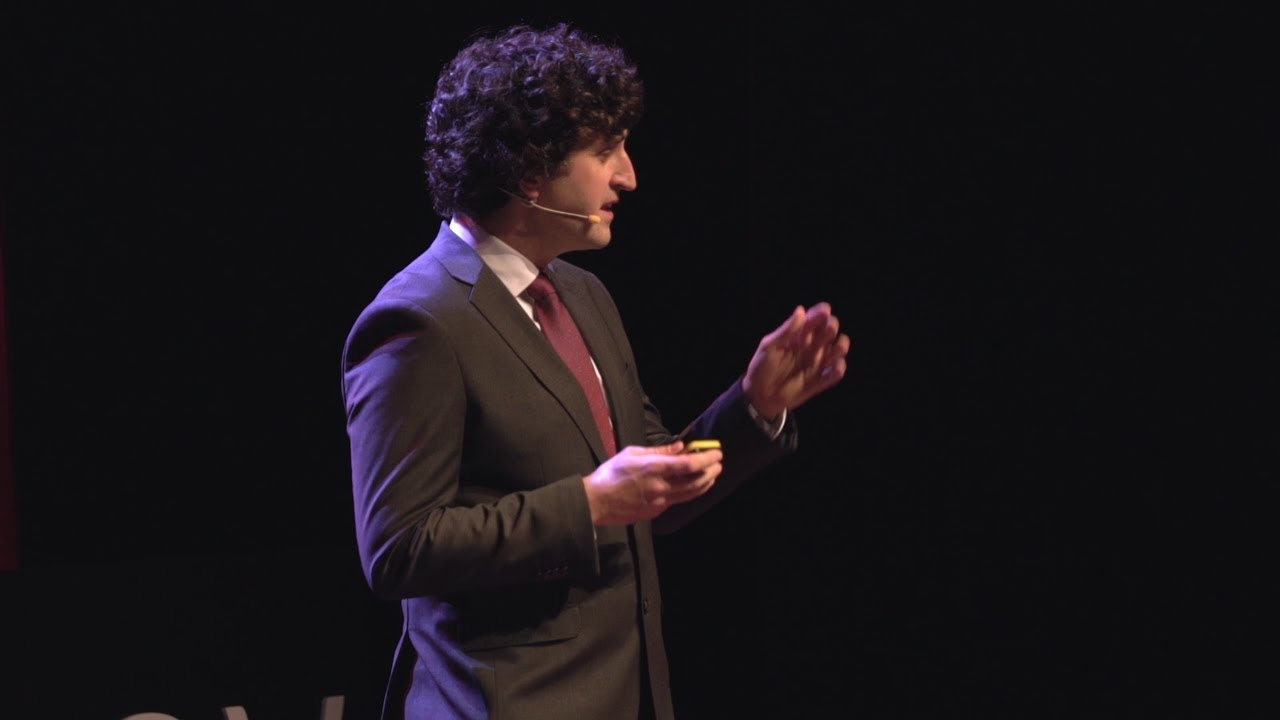
The future of AI in medicine | Conor Judge | TEDxGalway
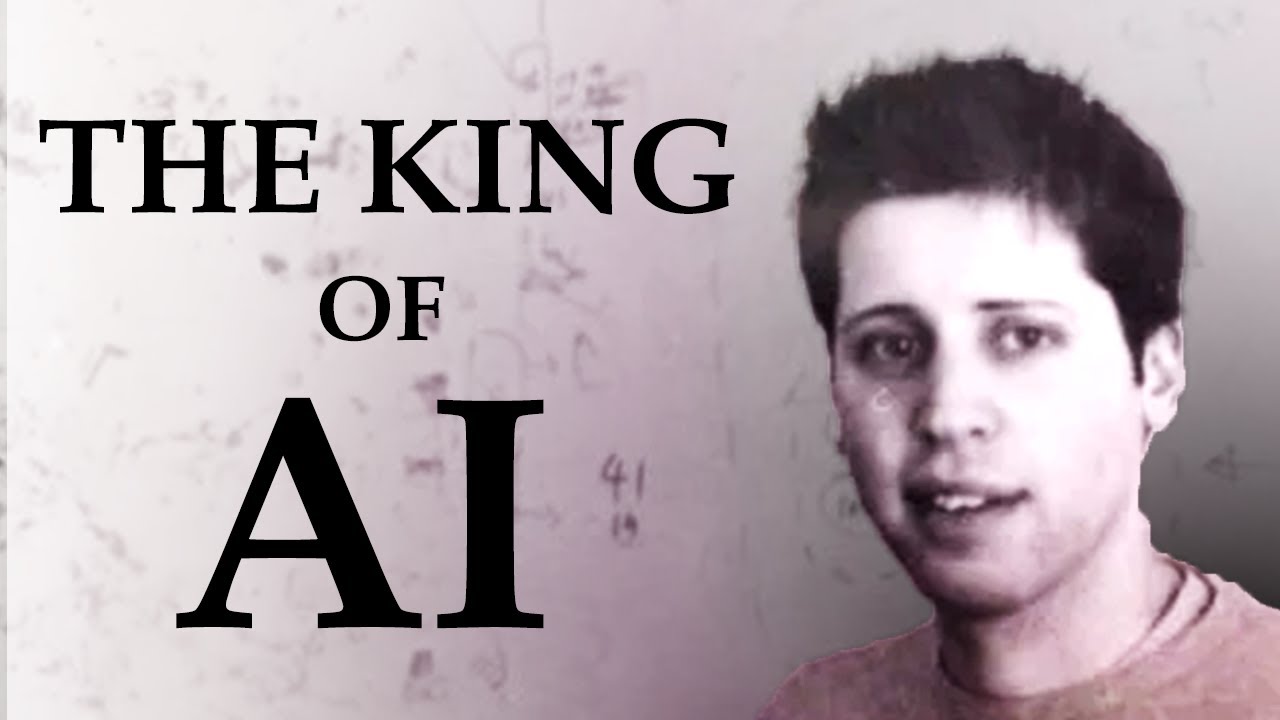
Sam Altman - The Man Who Owns Silicon Valley
5.0 / 5 (0 votes)