XAI-SA 2024, Opening talk
Summary
TLDRThis workshop delves into explainable machine learning and AI, addressing the shift from interpretable models to the black box nature of deep learning. It aims to make these models transparent through post-training interpretation methods or by designing inherently interpretable models. The event features presentations on various topics, including spectrum interpretability in music machine learning, hybrid deep neural audio processing, and the challenges of understanding AI models. With 20 paper presentations, oral talks, and a panel discussion, the workshop fosters collaboration and innovative ideas in the field of explainable AI.
Takeaways
- π The workshop is focused on explainable machine learning and AI, which has become a prominent field due to the rise of deep learning and the resulting black box models.
- π Explainable machine learning aims to make these complex models transparent, allowing for better understanding of their decision-making processes.
- π οΈ There are various solutions to achieve explainability, including post hoc interpretation methods and designing inherently interpretable models from the start.
- π The trade-offs between these methods will be explored during the workshop, with presentations and discussions on the latest research and applications.
- π The workshop features invited talks from experts in the field, including researchers from the speech and audio community and a renowned machine learning expert.
- π There will be 20 paper presentations, with four oral presentations and 16 poster presentations, covering a range of topics from model interpretation to application-specific uses.
- πΌ Specific applications discussed include music machine learning, speech and audio processing, and analysis of deep learning models for various purposes.
- ποΈ The workshop schedule includes a series of talks, paper presentations, and a panel discussion aimed at fostering collaboration and generating new ideas.
- πΉ The event is being recorded, and there is a YouTube channel for the workshop where invited talks and author videos will be posted.
- π₯ The organizers and reviewers are acknowledged for their efforts in ensuring a thorough review process, with each paper receiving at least three reviews.
- π Authors are encouraged to submit videos for their papers to the workshop's email for inclusion on the YouTube channel.
Q & A
What is the main focus of the workshop described in the transcript?
-The main focus of the workshop is on explainable machine learning and explainable AI, particularly in the context of deep learning models that are often considered black boxes.
Why has explainable machine learning become more important in recent years?
-Explainable machine learning has become more important due to the rise of deep learning, which has led to the creation of complex models that are not easily interpretable, thus making it a necessity to make these 'black box' models more transparent.
What are the two main approaches to making machine learning models more interpretable as mentioned in the transcript?
-The two main approaches are: 1) using post hoc interpretation methods where the original model is not altered but an attempt is made to understand its workings after training, and 2) designing an interpretable model from the start.
What is the trade-off that needs to be explored when choosing between the two approaches to explainability?
-The trade-off involves balancing the model's interpretability with its performance. Sometimes, more interpretable models may not perform as well as complex, less interpretable models.
What is the goal of the workshop in terms of the participants?
-The goal of the workshop is to bring together people working in the field of explainable AI to foster collaborations and come up with innovative ideas.
How many paper presentations are planned for the workshop?
-There are 20 paper presentations planned for the workshop, including four oral presentations.
What types of topics can be expected in the paper presentations and invited talks?
-The topics cover a range of areas including interpretable models, applications of post hoc explanation methods, analysis of deep learning models for music, and methodological approaches to explainable AI in speech and audio applications.
Who are some of the invited speakers mentioned in the transcript, and what are their areas of expertise?
-Some of the invited speakers include Ethan, who will talk about spectrum of interpretability for music machine learning; Cynthia Rudin from Duke University, who will discuss interpretable models versus post hoc interpretations; Professor G, who will cover hybrid and interpretable deep neural audio processing; and Gordon Wiinn, who will discuss understanding investigations into probing and training data memorization of AI models.
What is the schedule for the poster presentations at the workshop?
-The first poster session is at 10 AM, and presenters are asked to set up their posters by that time. All participants will present in all the sessions.
What is the role of the reviewers in the workshop, and how many reviews were written in total?
-The reviewers played a crucial role in the evaluation process, ensuring that each paper received at least three reviews, and in most cases, four. A total of 66 reviews were written for the workshop.
How can authors share their presentations or videos related to the workshop?
-Authors can share their videos by sending them to the workshop's email, and these will be featured on the workshop's YouTube channel, along with the invited talks.
Outlines
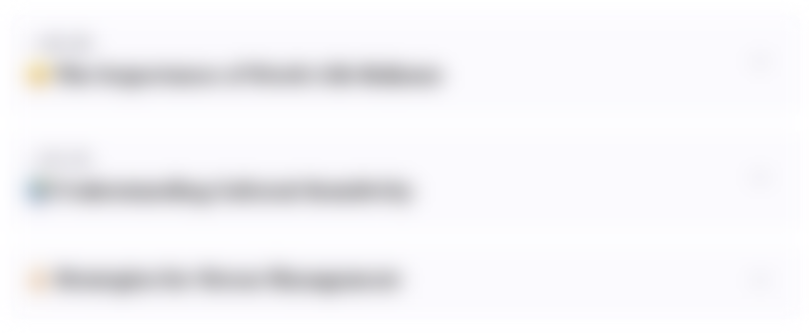
This section is available to paid users only. Please upgrade to access this part.
Upgrade NowMindmap
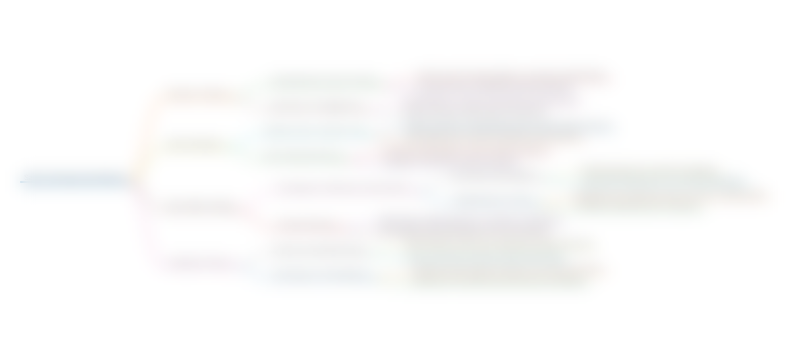
This section is available to paid users only. Please upgrade to access this part.
Upgrade NowKeywords
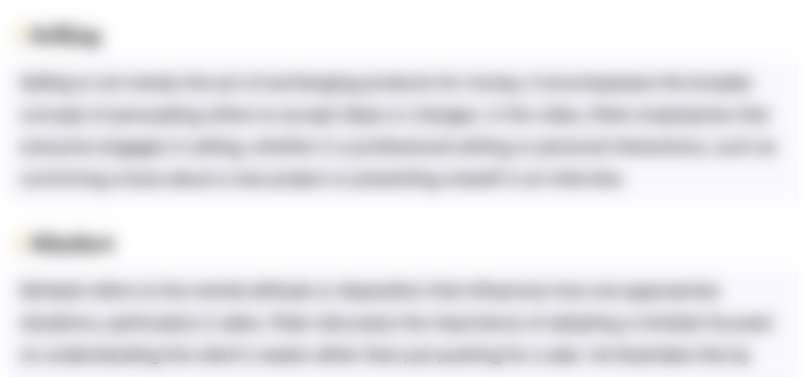
This section is available to paid users only. Please upgrade to access this part.
Upgrade NowHighlights
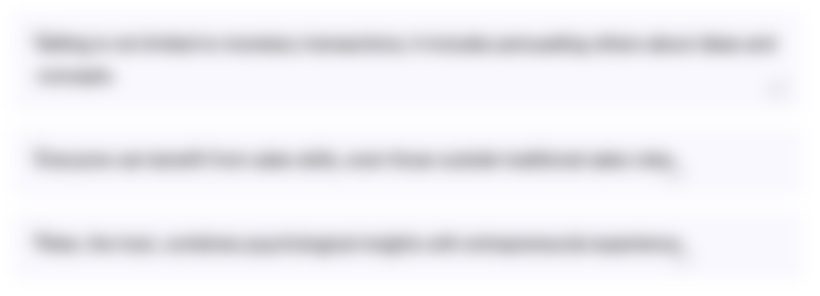
This section is available to paid users only. Please upgrade to access this part.
Upgrade NowTranscripts
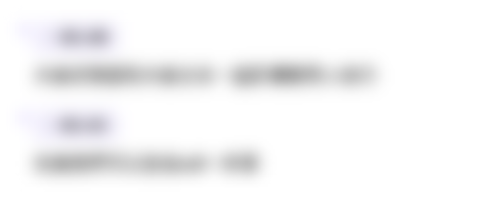
This section is available to paid users only. Please upgrade to access this part.
Upgrade NowBrowse More Related Video
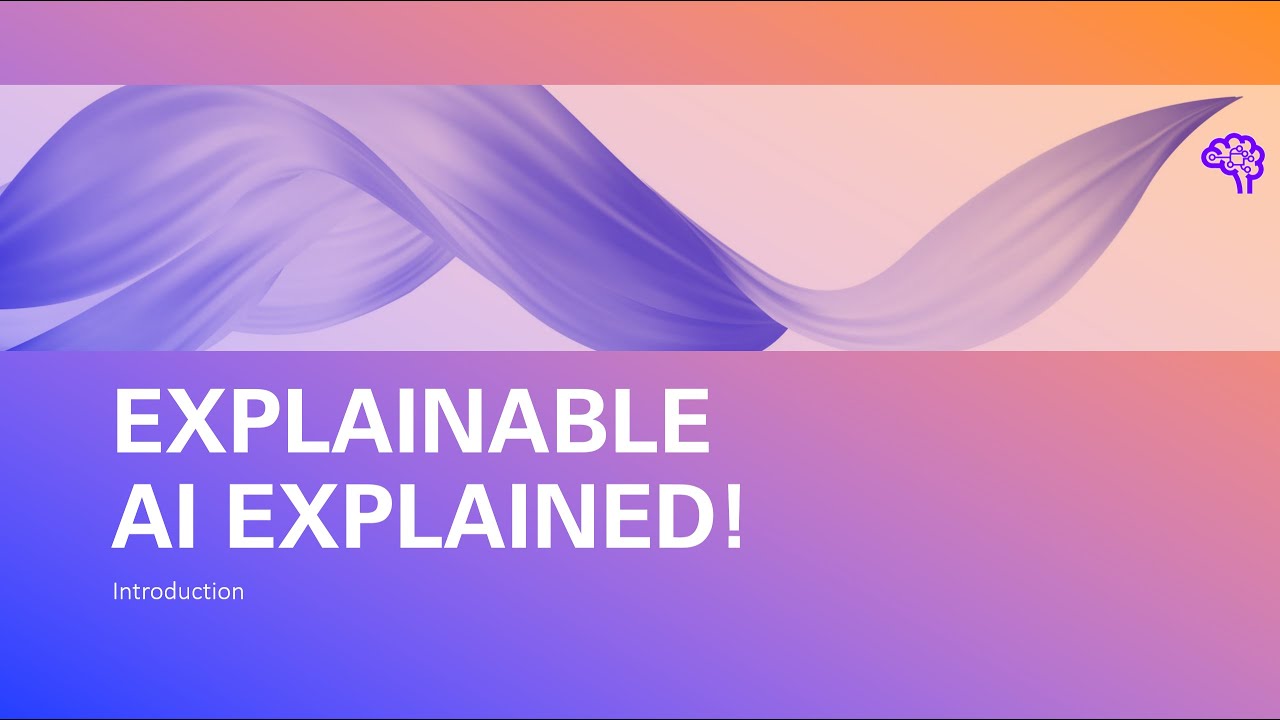
Explainable AI explained! | #1 Introduction

Introduction to Generative AI n explainable AI
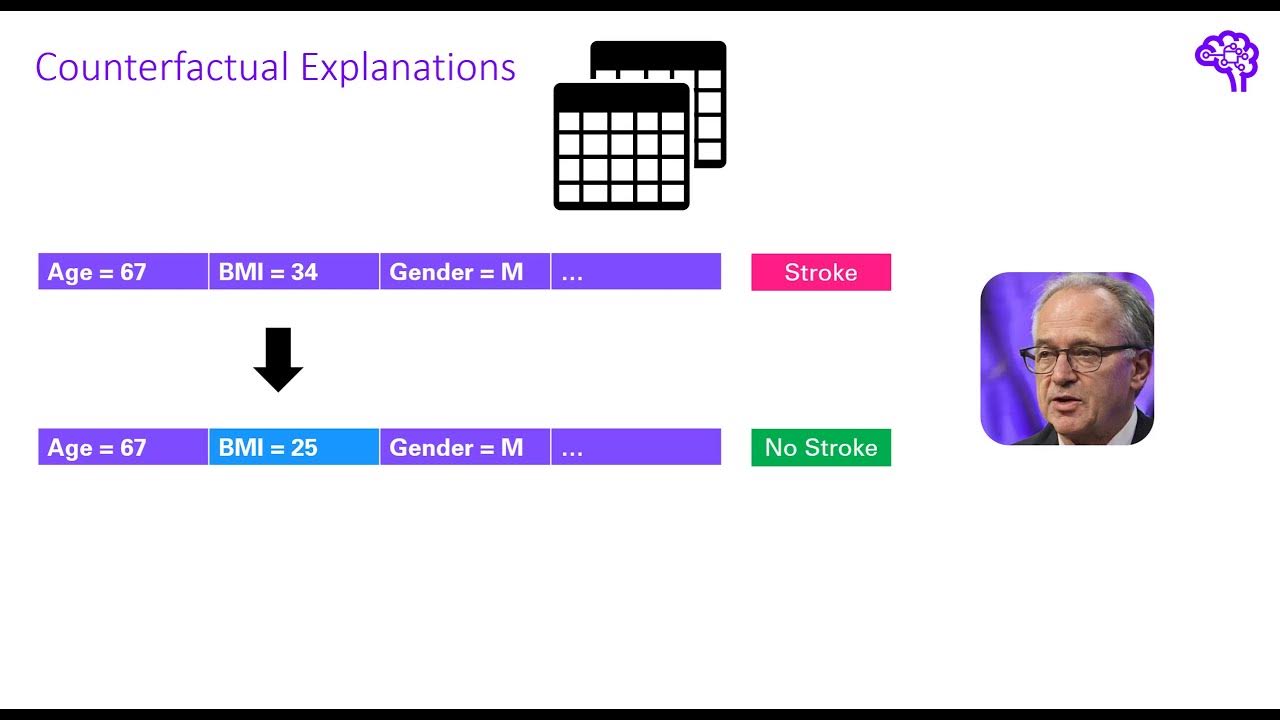
Explainable AI explained! | #5 Counterfactual explanations and adversarial attacks

Explainable AI Tutorial (XAI) | Part- 1 | Types of Machine Learning | Python
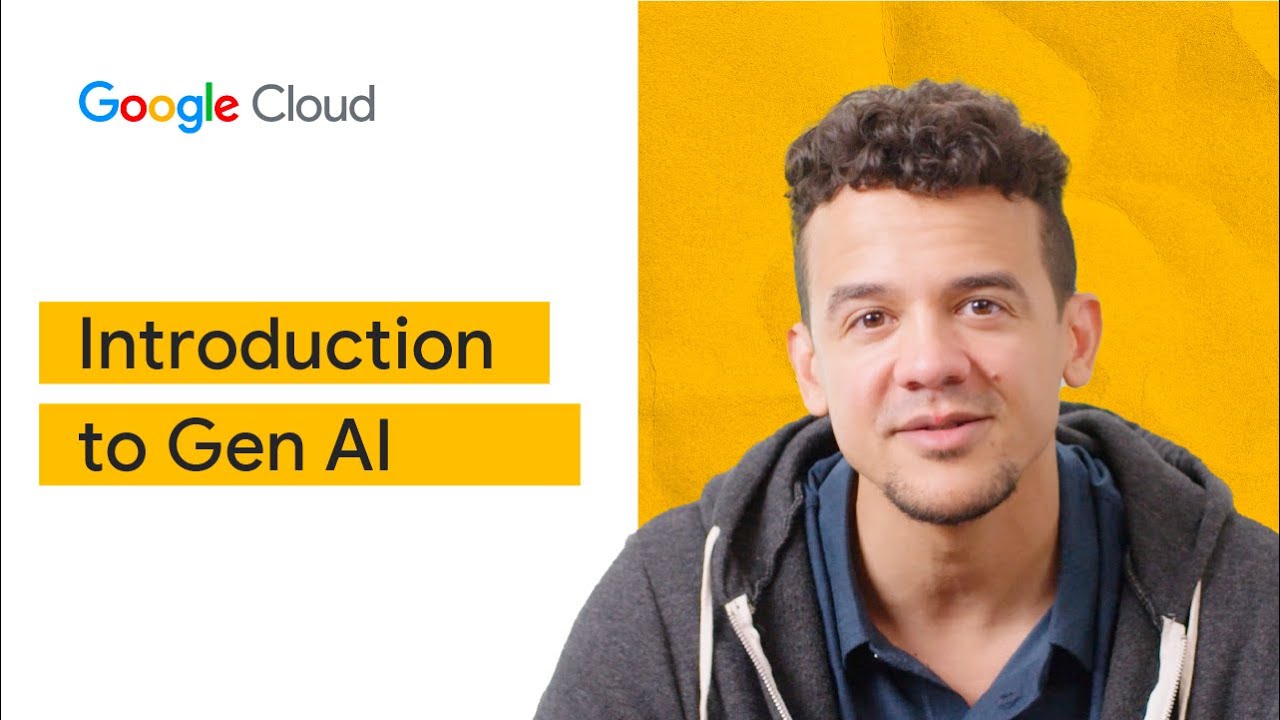
Introduction to Generative AI
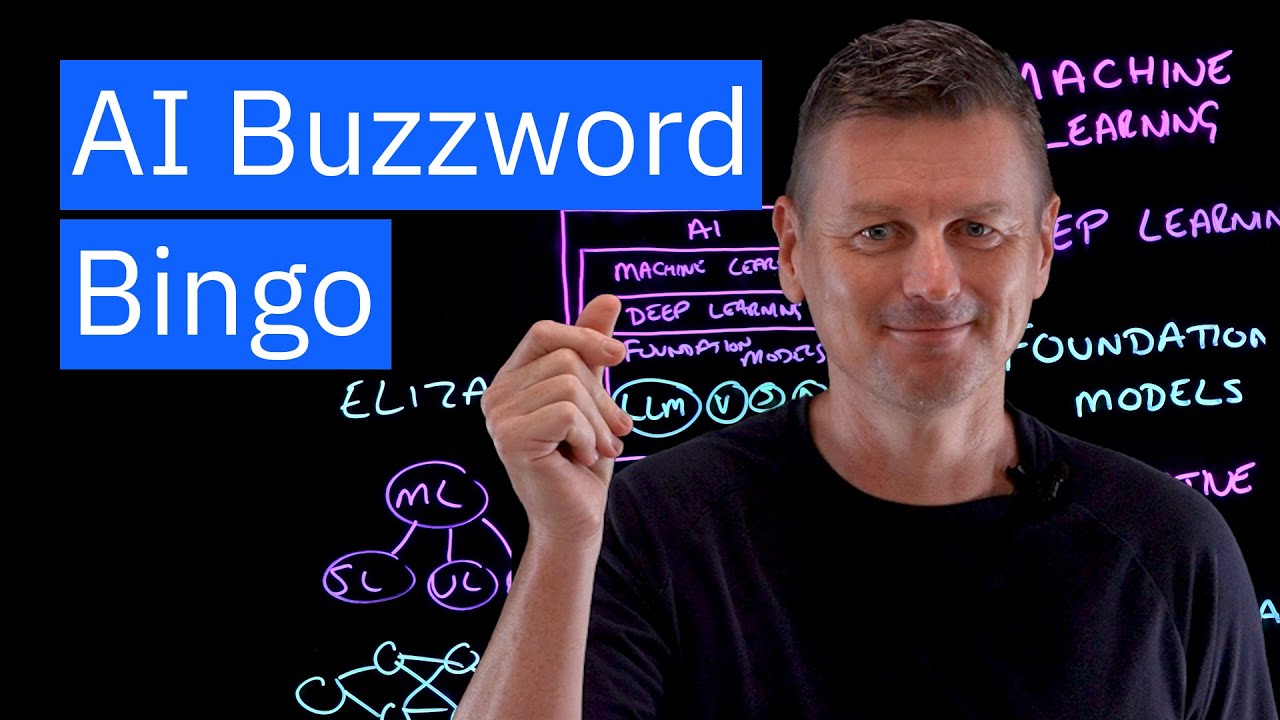
Machine Learning vs. Deep Learning vs. Foundation Models
5.0 / 5 (0 votes)