How to Get Ahead of 99% of Data Scientists with Streamlit (Tips from Tyler Richards)
Summary
TLDRIn this Data Science podcast episode, Tyler Richards shares his journey from election science to becoming a data scientist at Snowflake. He discusses his experience at Meta, focusing on content integrity and the challenges of moderating vast amounts of user-generated content. Richards also delves into his passion for Streamlit, an open-source library that simplifies the creation of data apps. His enthusiasm led him to write a book on Streamlit, which he continually updates to reflect the evolving tool. The conversation highlights how Streamlit can enhance job applications and the potential for data scientists to monetize their work, with Richards' recent creation of the 'stpaywall' component, aiming to facilitate online monetization for Streamlit app creators.
Takeaways
- 😀 Tyler Richards, a data scientist at Snowflake, co-hosts the podcast and discusses his journey from election science to working at Meta on content integrity, and eventually joining Streamlit.
- 📚 Tyler authored a book about Streamlit, which he revised and updated after joining the company, aiming to create the resource he wished existed when he started learning Streamlit.
- 💡 Streamlit is positioned as an effective tool for data scientists to stand out in the job application process by creating shareable, interactive apps that demonstrate their skills and projects.
- 🔑 Tyler's experience at Meta involved working on complex machine learning models for content moderation, emphasizing the importance of balancing free speech with platform integrity.
- ✍️ Writing a book was a method for Tyler to consolidate his learning and create a comprehensive guide for others to learn Streamlit effectively, focusing on deep understanding rather than fragmented knowledge.
- 🤝 The value of mentorship and feedback is highlighted as crucial for Tyler's growth in programming and data science, suggesting that pair programming and code reviews are beneficial practices.
- 💼 Tyler's transition from Meta to Streamlit was driven by a desire to be part of an early-stage company and to work more closely with a product he was passionate about.
- 🌐 Streamlit's open-source nature allows it to be used across various platforms, not just within Snowflake, providing flexibility for developers to host their apps in various environments.
- 🛠️ Tyler introduced 'streamlit_paywall', a component to facilitate monetization of Streamlit apps, with the goal of enabling data scientists to generate income from their work online.
- 💡 The importance of curiosity and kindness is emphasized as core values that Tyler would advise his younger self to embody, suggesting these traits are fundamental to personal and professional development.
Q & A
What is Tyler Richards' background and how did he become a data scientist?
-Tyler Richards has a background in election science, having worked in nonprofits and academia. He transitioned to data science and worked at Meta (formerly Facebook) focusing on integrity-related projects. His interest in data science was further fueled by his experience with the open-source library Streamlit, which he used extensively and eventually wrote a book about.
How did Tyler Richards and the host end up working at Streamlit?
-Both Tyler and the host were initially internet friends who connected through their shared interest in data science. They both joined Streamlit after recognizing its potential and enjoying their experiences with the platform, transitioning from being just friends to co-workers.
What was Tyler Richards' role at Meta focused on?
-At Meta, Tyler worked in the Integrity space, focusing on content moderation. His role involved developing machine learning models to efficiently identify and remove content that violated Meta's rules, balancing user experience, business interests, and free speech considerations.
What motivated Tyler Richards to write a book about Streamlit?
-Tyler was inspired to write a book about Streamlit after publishers reached out to him due to his online writings about the library. As an avid reader, he saw it as an opportunity to create a resource that he would have wanted to read, focusing on practical applications and experiences with Streamlit.
How did Tyler Richards' experience at Meta influence his decision to join Streamlit?
-Tyler's experience at Meta, where he worked on large-scale data science projects, made him appreciate the efficiency and capabilities of Streamlit. His desire to work in a more unpredictable and fast-paced environment, as well as his love for the product, led him to join Streamlit.
What is the significance of Streamlit in job applications according to Tyler Richards?
-Tyler believes that creating Streamlit apps can significantly enhance a job application by demonstrating practical skills and creativity. By building an app tailored to a specific job or company, applicants can stand out and showcase their ability to create tangible, impressive projects quickly.
How did Tyler Richards learn Python and what was his initial programming language?
-Tyler initially learned R during his stats classes and used it extensively. However, when he was offered a data science internship at Procter and Gamble, he had to quickly learn Python. He scrambled to learn Python in about three weeks and further honed his skills through pair programming with experienced engineers.
What advice does Tyler Richards have for finding a mentor?
-Tyler suggests that mentorship should be a two-way street where both parties benefit. He emphasizes the importance of showing earnestness, intellectual curiosity, and the potential to reciprocate the help in the future. He also highlights the value of hands-on learning and feedback from those more experienced.
What is the purpose of the 'stpwall' component Tyler Richards created?
-The 'stpwall' component is designed to simplify the process of monetizing Streamlit apps. It allows developers to easily implement a paywall in their apps, enabling them to charge users for access. Tyler's goal is to encourage more data scientists to create and sell their apps, potentially making a living from their work.
What advice would Tyler Richards give to his younger self?
-Tyler would advise his younger self to be more curious and more kind. He believes that embracing curiosity and treating others with kindness are essential qualities that have helped him in his personal and professional life.
How can listeners reach Tyler Richards and learn more about his work?
-Listeners can reach Tyler Richards through Twitter, where he is most active. His Twitter handle is Tyler J Richards, and his email can be found on his Twitter profile. He also mentions that the proceeds from his book are donated to charity, indicating his commitment to giving back.
Outlines
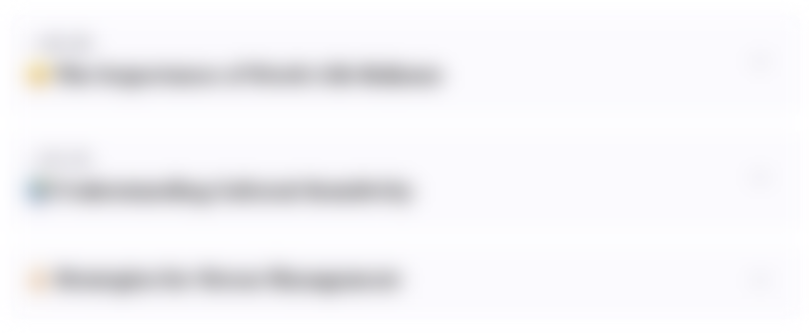
Этот раздел доступен только подписчикам платных тарифов. Пожалуйста, перейдите на платный тариф для доступа.
Перейти на платный тарифMindmap
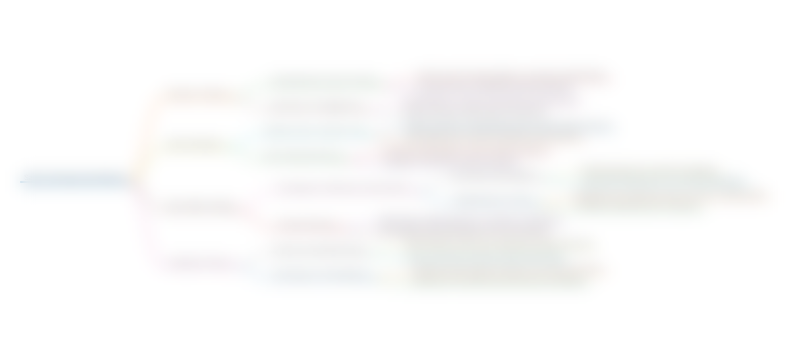
Этот раздел доступен только подписчикам платных тарифов. Пожалуйста, перейдите на платный тариф для доступа.
Перейти на платный тарифKeywords
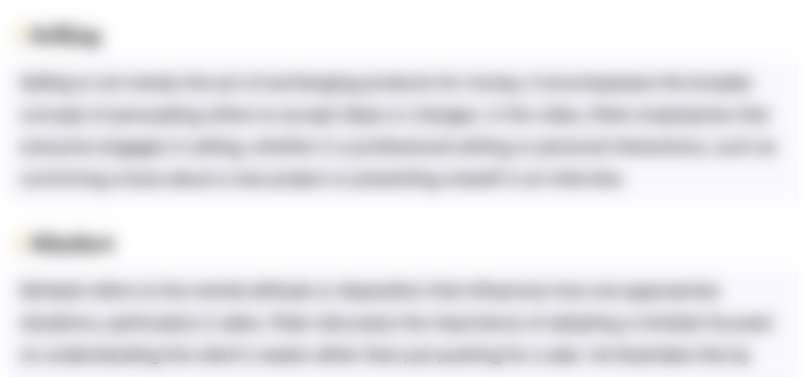
Этот раздел доступен только подписчикам платных тарифов. Пожалуйста, перейдите на платный тариф для доступа.
Перейти на платный тарифHighlights
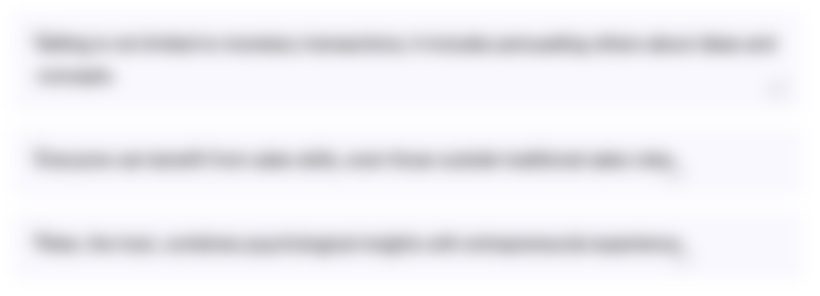
Этот раздел доступен только подписчикам платных тарифов. Пожалуйста, перейдите на платный тариф для доступа.
Перейти на платный тарифTranscripts
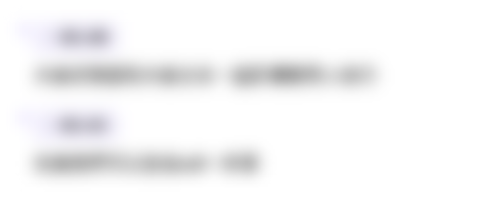
Этот раздел доступен только подписчикам платных тарифов. Пожалуйста, перейдите на платный тариф для доступа.
Перейти на платный тарифПосмотреть больше похожих видео

How I Became A Data Scientist (No CS Degree, No Bootcamp)

Exploring Data Science: How Political Campaigns Use Data and Analytics
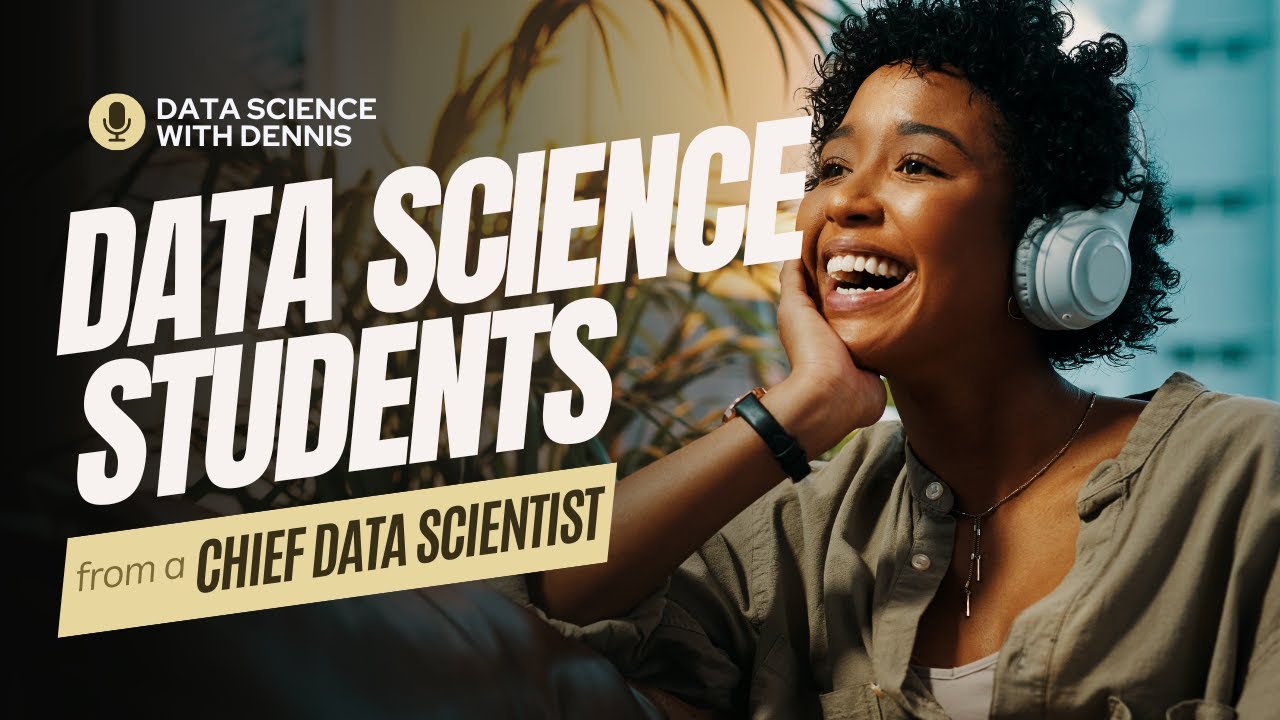
Data Science Blueprint for Students
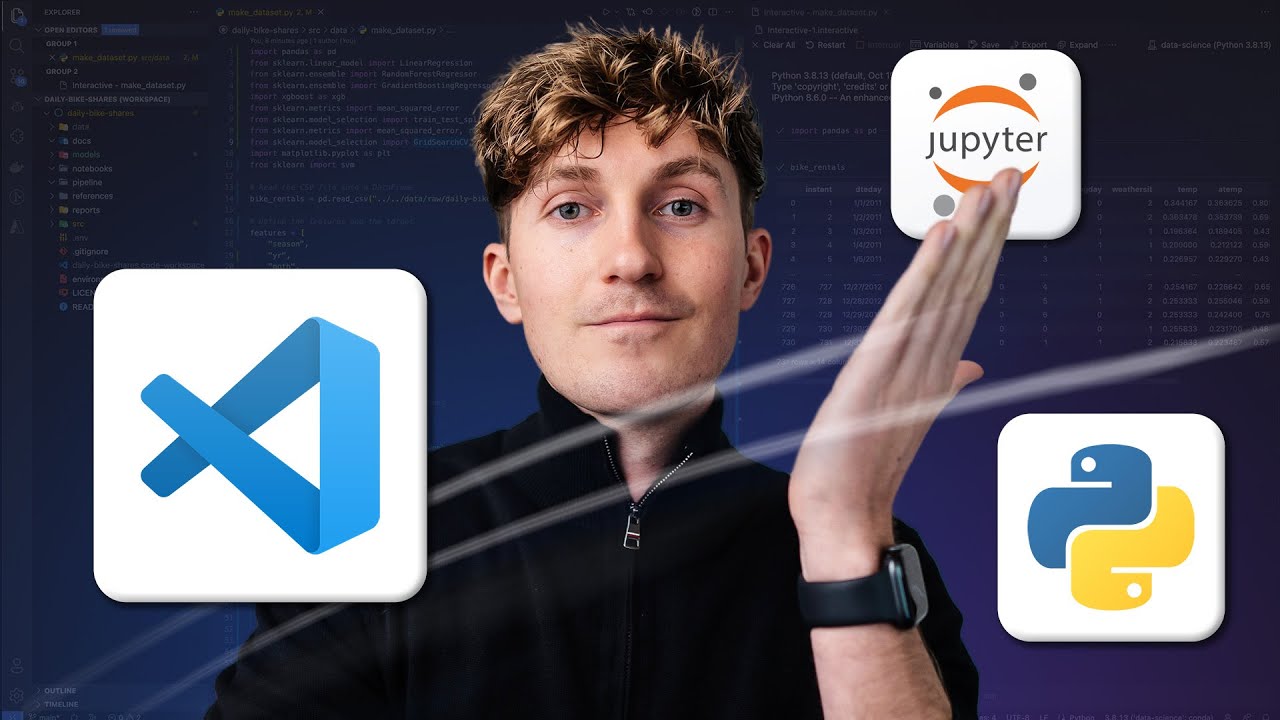
How to Set up VS Code for Data Science & AI
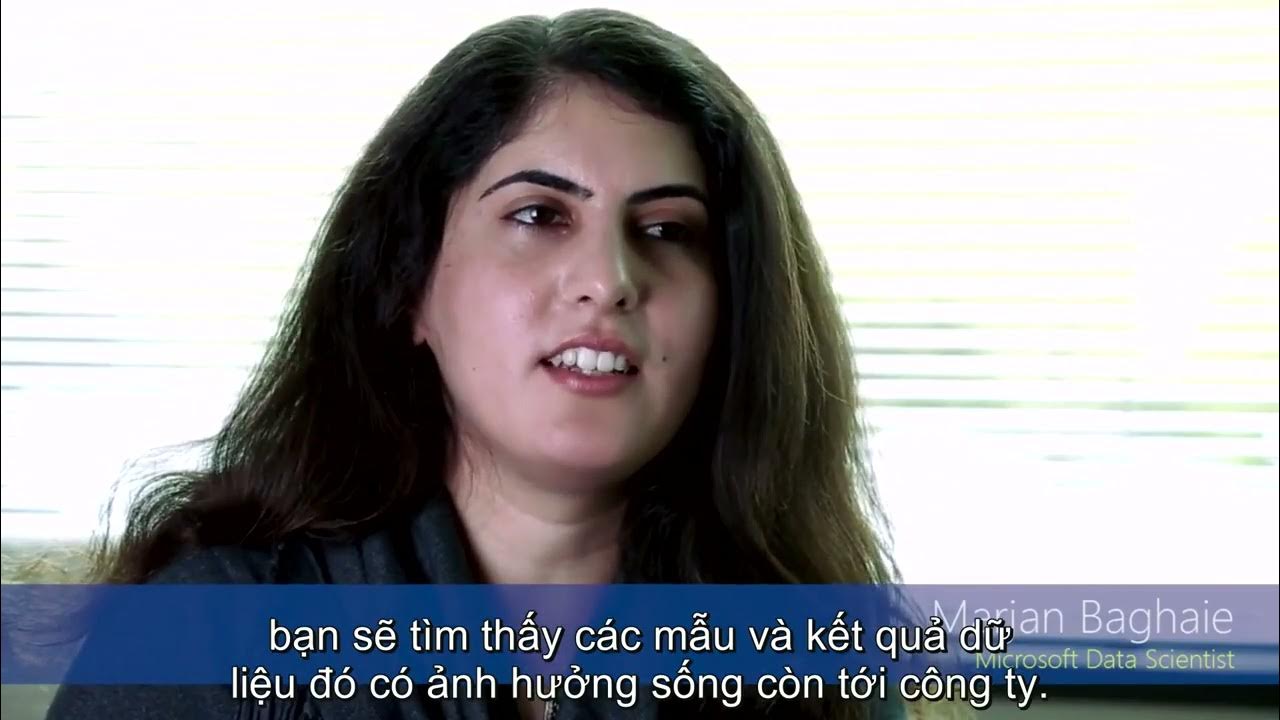
01 Điều gì khiến tôi mong muốn trở thành một nhà khoa học dữ liệu
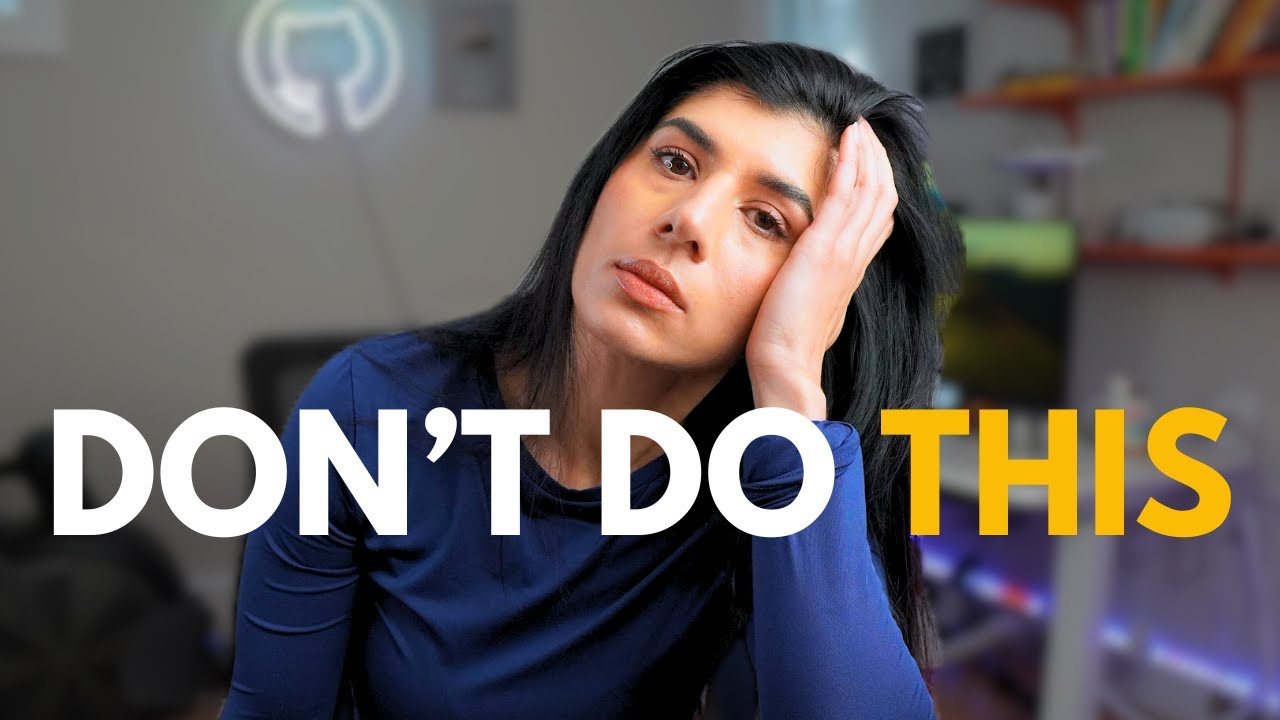
Starting a Career in Data Science (10 Thing I Wish I Knew…)
5.0 / 5 (0 votes)