Z-statistics vs. T-statistics | Inferential statistics | Probability and Statistics | Khan Academy
Summary
TLDRThis video explains the difference between Z-statistics and T-statistics in inferential statistics. It highlights how both statistics help determine the probability of obtaining a certain sample mean, with the Z-statistic used when sample sizes are large (over 30), assuming normal distribution. When sample sizes are small, the T-statistic is preferred, using a T-distribution instead. The video covers how to calculate these statistics and when to apply each one, providing clear distinctions between their use in normal and T-distribution contexts, and how sample size affects the choice of statistic.
Takeaways
- 😀 A Z-statistic is used to determine the probability of obtaining a sample mean at least as extreme as the one observed, assuming a large sample size.
- 😀 The Z-statistic is calculated by subtracting the population mean from the sample mean and dividing by the standard deviation of the sampling distribution.
- 😀 When the sample size is large (n > 30), we can use the Z-statistic to determine probabilities from a normal distribution.
- 😀 If the population standard deviation is unknown, the sample standard deviation can be used as an estimate when the sample size is large enough.
- 😀 The central limit theorem assures that for large sample sizes, the sampling distribution of the sample mean will be approximately normal, allowing us to use the Z-statistic.
- 😀 When the sample size is small (n < 30), the sampling distribution of the sample mean follows a T-distribution, not a normal distribution.
- 😀 For small samples, instead of the Z-statistic, the T-statistic is used, which follows a T-distribution with a mean of 0.
- 😀 The T-statistic is also calculated by subtracting the population mean from the sample mean, but it uses the sample standard deviation and the square root of the sample size in the denominator.
- 😀 A T-distribution is more spread out than the normal distribution and accounts for the greater variability expected in smaller samples.
- 😀 To determine the probability of getting a T-value at least as extreme as the observed value, we use a T-table, similar to how we use a Z-table for the Z-statistic.
- 😀 As a rule of thumb: for sample sizes larger than 30, the Z-statistic is appropriate; for smaller sample sizes, the T-statistic should be used, with the appropriate distribution table for each.
Q & A
What is the primary purpose of using the Z-statistic and T-statistic?
-The primary purpose of using the Z-statistic and T-statistic is to determine the probability of getting a sample mean as extreme as the one observed, given the assumed mean and the sample's standard deviation. The choice between the Z-statistic and T-statistic depends on the sample size and whether the population standard deviation is known.
How do you calculate the Z-statistic?
-The Z-statistic is calculated by subtracting the population mean from the sample mean and dividing the result by the standard deviation of the sampling distribution of the sample mean. This formula tells us how many standard deviations away the sample mean is from the population mean.
Why do we use the sample standard deviation in place of the population standard deviation when calculating the Z-statistic?
-We use the sample standard deviation as an estimate of the population standard deviation when the population standard deviation is unknown. This is valid when the sample size is large enough (typically n > 30), as the sample standard deviation is a good estimator of the population standard deviation in such cases.
When should you use the T-statistic instead of the Z-statistic?
-You should use the T-statistic when the sample size is small (n < 30) and when the population standard deviation is unknown. The T-statistic follows a T-distribution, which is wider and has heavier tails compared to the normal distribution used for Z-statistics.
What is the main difference between the Z-distribution and the T-distribution?
-The main difference is that the Z-distribution is a normal distribution, which has a fixed shape, while the T-distribution is more variable, especially with small sample sizes, and has heavier tails. As the sample size increases, the T-distribution approaches the normal distribution.
What does the central limit theorem tell us about the distribution of sample means?
-The central limit theorem states that if the sample size is sufficiently large, the distribution of the sample mean will be approximately normal, regardless of the original population's distribution. This is why we can use the Z-statistic for large sample sizes.
How do you interpret the result of a Z or T-statistic in terms of probability?
-The result of a Z or T-statistic gives the number of standard deviations the sample mean is from the assumed population mean. You can then use a Z-table or T-table to determine the probability of obtaining a result as extreme or more extreme than the observed result.
What happens when the sample size is small and we use a Z-statistic?
-When the sample size is small, using the Z-statistic becomes less reliable because the sampling distribution of the sample mean may not be normal. In such cases, the T-statistic is preferred as it adjusts for the uncertainty introduced by small sample sizes.
Why is a sample size of 30 considered a threshold for using the Z-statistic?
-A sample size of 30 is considered the threshold because, based on the central limit theorem, sample means from large enough samples (typically n > 30) are approximately normally distributed. Below this size, the sample mean may not follow a normal distribution, requiring the use of the T-statistic instead.
What is the rule of thumb for choosing between the Z-statistic and the T-statistic?
-The rule of thumb is: if the sample size is greater than 30, use the Z-statistic, and if the sample size is less than 30, use the T-statistic. Additionally, the T-statistic is used when the population standard deviation is unknown.
Outlines
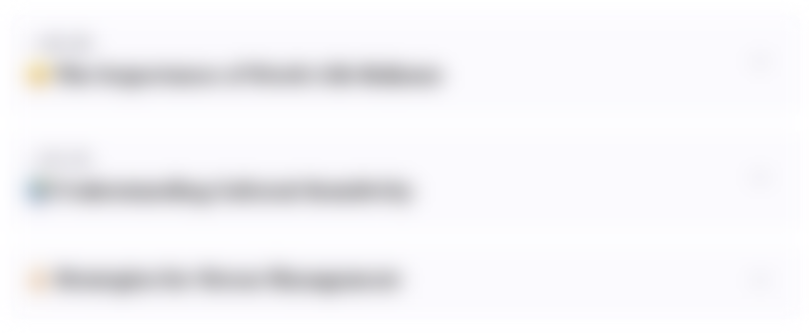
Этот раздел доступен только подписчикам платных тарифов. Пожалуйста, перейдите на платный тариф для доступа.
Перейти на платный тарифMindmap
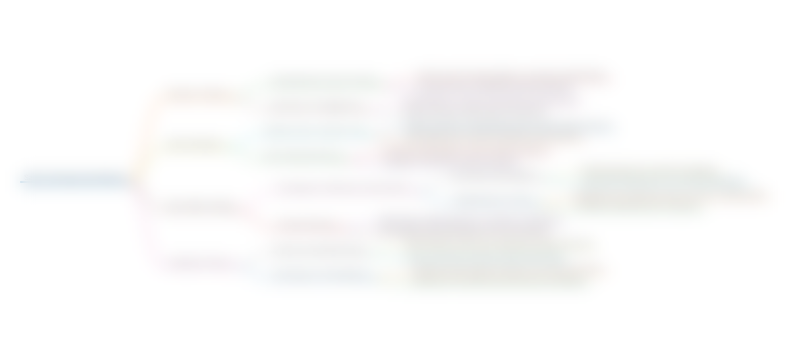
Этот раздел доступен только подписчикам платных тарифов. Пожалуйста, перейдите на платный тариф для доступа.
Перейти на платный тарифKeywords
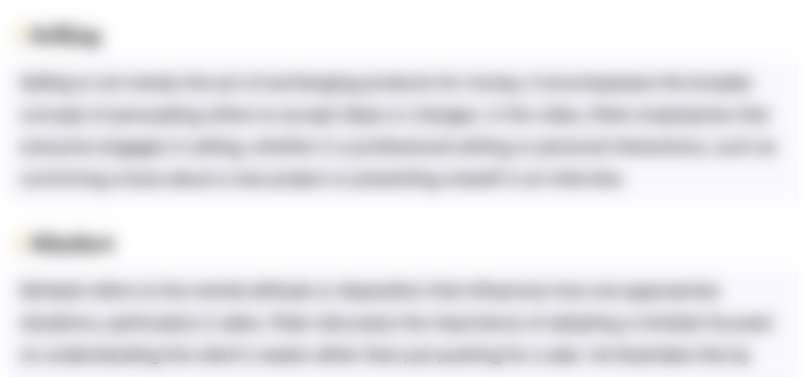
Этот раздел доступен только подписчикам платных тарифов. Пожалуйста, перейдите на платный тариф для доступа.
Перейти на платный тарифHighlights
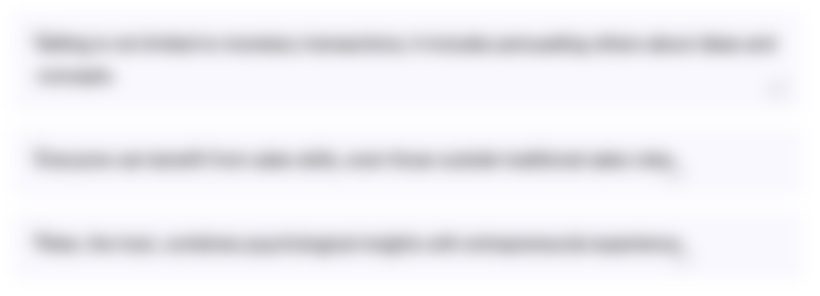
Этот раздел доступен только подписчикам платных тарифов. Пожалуйста, перейдите на платный тариф для доступа.
Перейти на платный тарифTranscripts
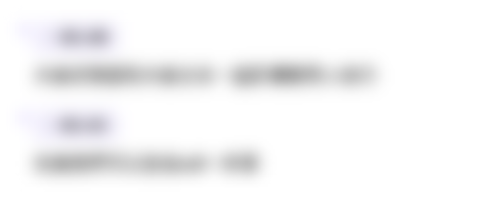
Этот раздел доступен только подписчикам платных тарифов. Пожалуйста, перейдите на платный тариф для доступа.
Перейти на платный тарифПосмотреть больше похожих видео
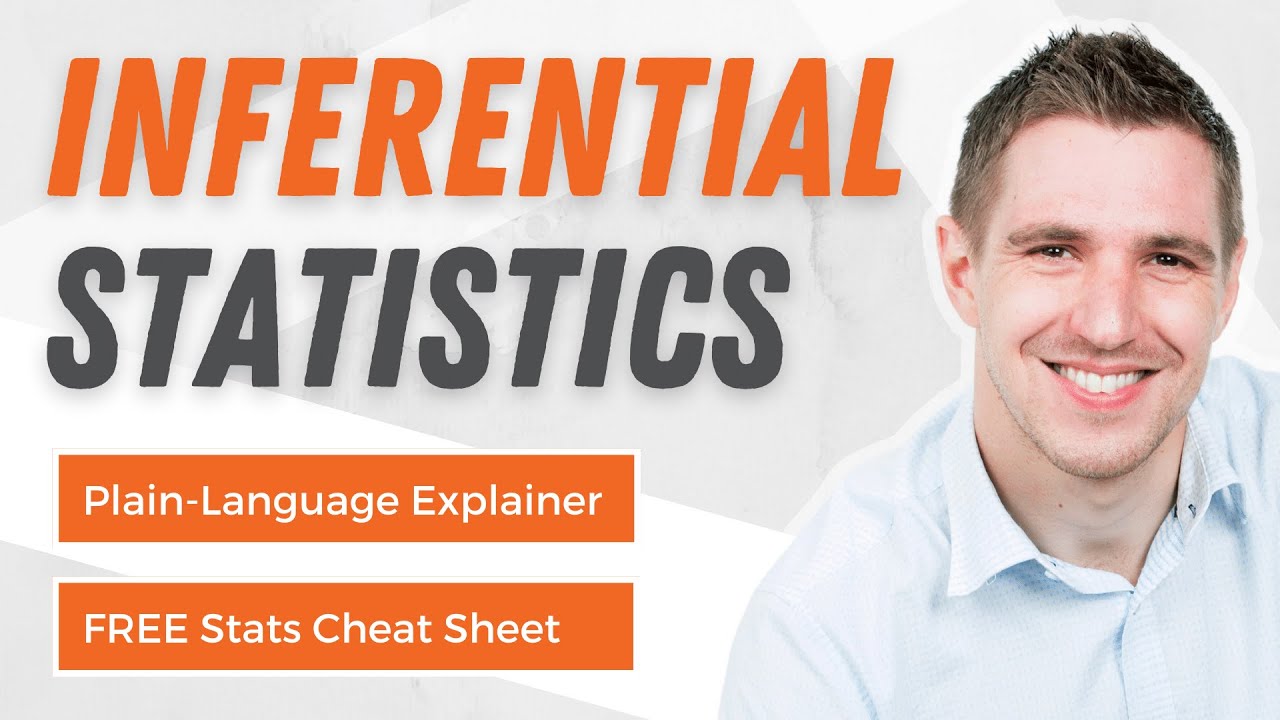
Inferential Statistics FULL Tutorial: T-Test, ANOVA, Chi-Square, Correlation & Regression Analysis
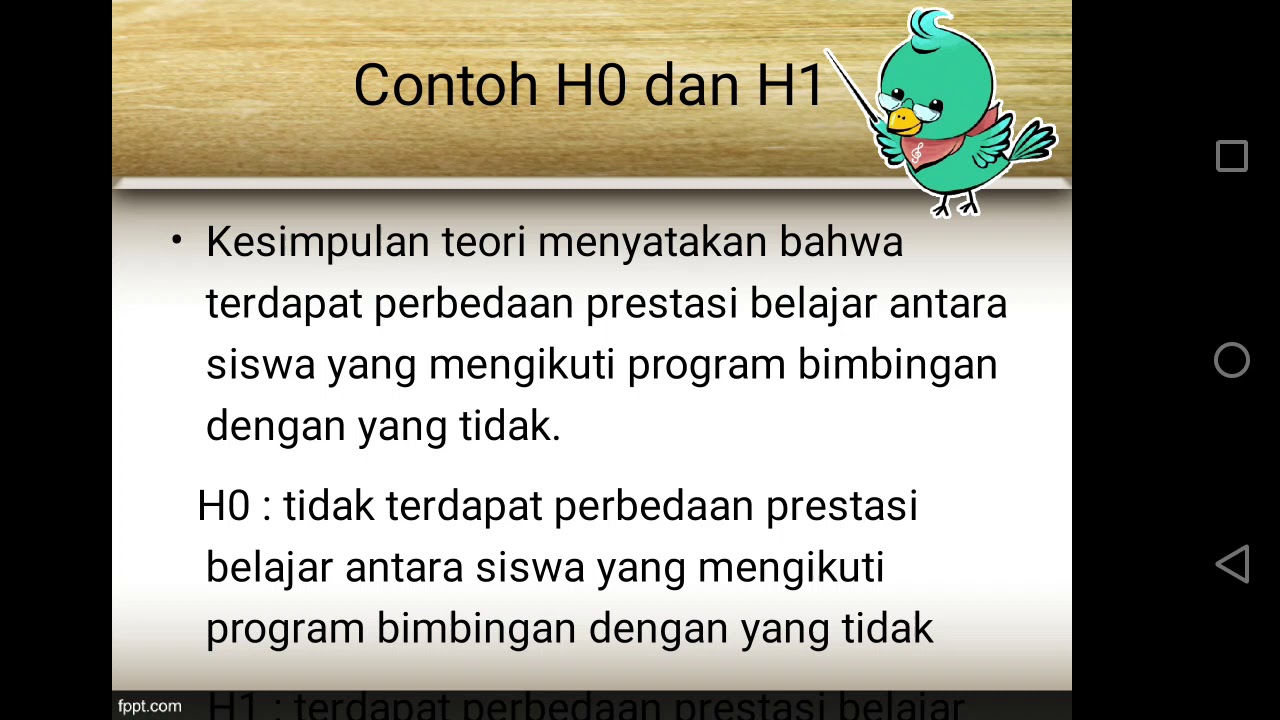
Statistik Inferensial & Hipotesis
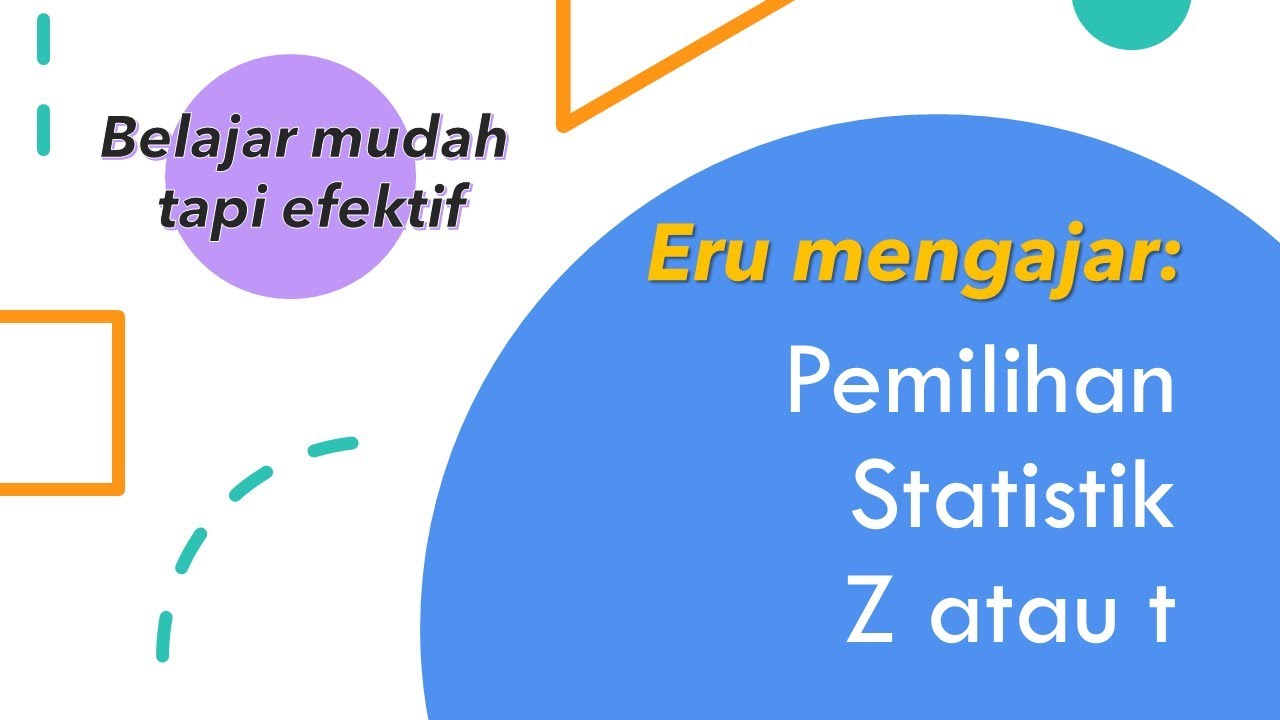
Pemilihan statistik Z atau t

KUPAS TUNTAS: Apakah Perbedaan Statistik Inferensial dengan Statistik Deskriptif ?
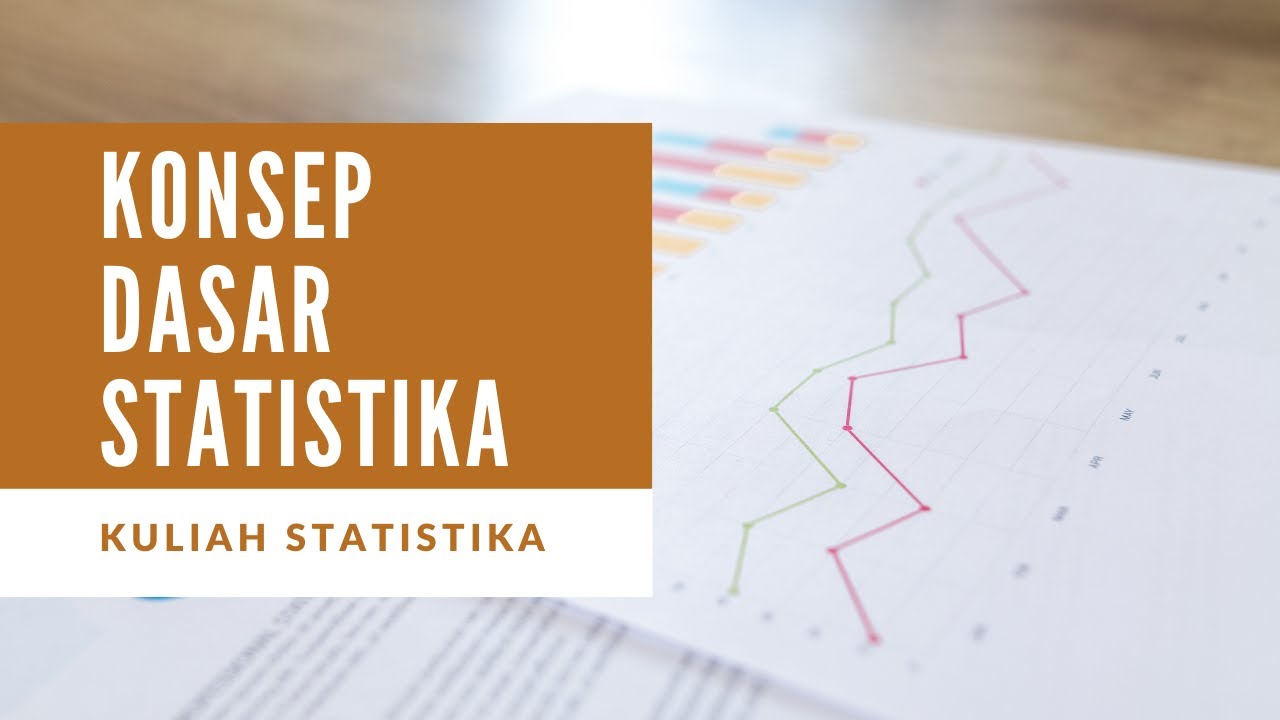
KULIAH STATISTIKA (1) - KONSEP DASAR STATISTIKA
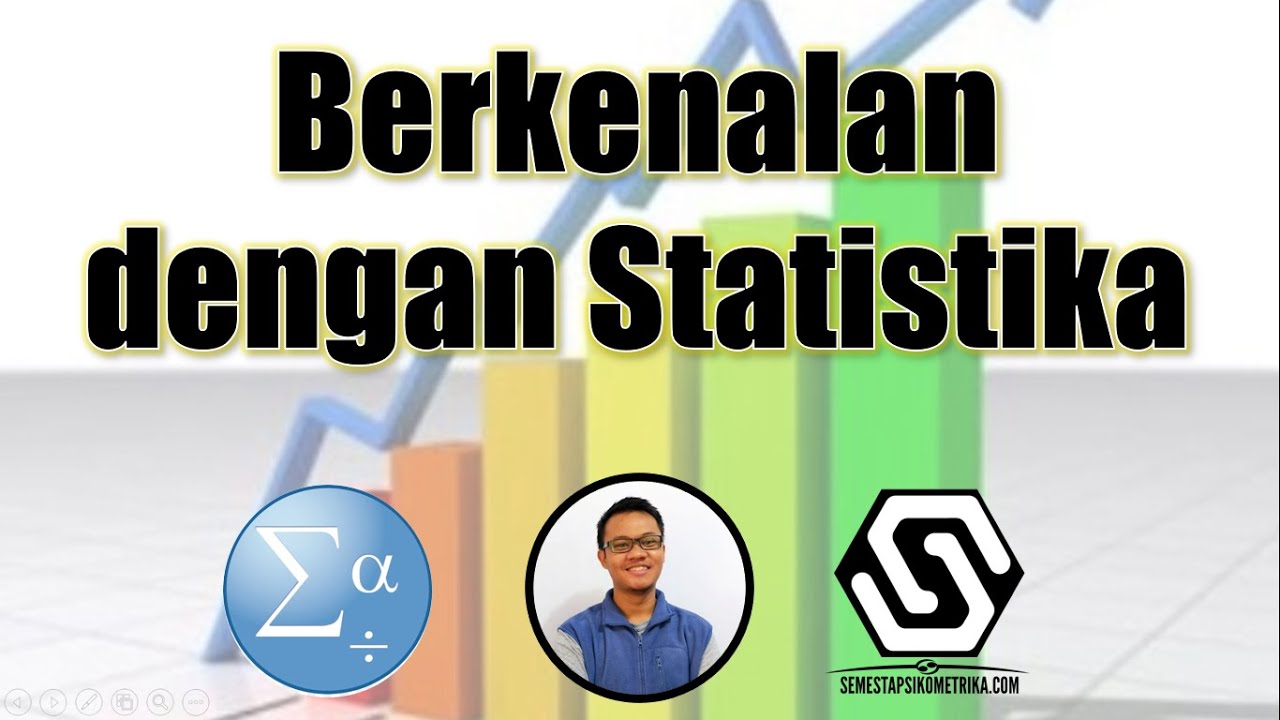
Berkenalan dengan Statistika
5.0 / 5 (0 votes)